Technology Automatically Delimits Areas of Brain in Medical Images
By MedImaging International staff writers Posted on 09 Oct 2013 |
Researchers have developed a way to enhance the delimitation of tumors in medical images.
As the investigators, from NUP/UPNA-Public University of Navarre (Spain), explained, “when the doctor decides where tumor tissue should be separated from healthy tissue, our algorithm ensures that he/she is never going choose the worst option because the best solution is automatically offered.”
The study’s findings were submitted by the Artificial Intelligence and Approximate Reasoning Group (GIARA) of the NUP/UPNA-Public University of Navarre and received an award from the European Association of Fuzzy Logic and Soft Computing (EUSFLAT) during its biennial meeting (EUSFLAT 2013), held in Milan (Italy), in September 2013.
The research has centered on the study of brain images obtained utilizing magnetic resonance imaging (MRI). Specifically, they have developed an algorithm to improve the process to segment the images. “By means of segmentation,” explained Dr. Aránzazu Jurío, one of the researchers of the study. “Each of the objects that make up the image is separated. Each pixel is analyzed so that all the ones sharing the same features are considered to form part of the same object.”
In the instance of medical images, this process is crucial for delimiting tumors where, if one is thinking about areas like those in the brain, 3 mm of difference can mean the difference between a cure and affecting areas controlling speech or vision.
To understand how the algorithm developed by the researchers works, Prof. Humberto Bustince, another investigator on the project, described the following parallel: “Imagine we have the image of a brain by means of magnetic resonance and seven doctors who have to decide how to delimit the tumor. From experience, we know that each one of them will separate the tumor differently. Now, with the proposed method, they will automatically be presented with a set of options, which, in any case, are always going to improve the choice that the worst of the seven may make. We’ve succeeding in improving on the worst: we’ve managed to ensure that even though they may go for the worst option, they will be wrong to the least extent possible.”
Another of the factors that specialists in artificial intelligence have to deal with is that of time variations, because medical images differ in time, and on occasions within a short space of time. “All these algorithms allow application in real time, because what may be useful for an image at a given moment may not be useful after some time,” explained Prof. Bustince.
In this respect, Dr. Jurío stressed that, “the algorithm we have developed produces a kind of consensus among the various functions that seek to obtain the best solution. We could say that it takes the process of choosing away from the expert because it is the algorithm that automatically selects the function.”
“The problem,” said coauthor of the study, Dr. Daniel Paternain, “is that for a specific image there are a number of functions that the expert may use, but if he or she gets the function wrong when doing the segmenting, the result could be devastating. What we tried to solve is this: if he/she gets the function wrong, the result may not be devastating.”
Related Links:
Artificial Intelligence and Approximate Reasoning Group
NUP/UPNA-Public University of Navarre
As the investigators, from NUP/UPNA-Public University of Navarre (Spain), explained, “when the doctor decides where tumor tissue should be separated from healthy tissue, our algorithm ensures that he/she is never going choose the worst option because the best solution is automatically offered.”
The study’s findings were submitted by the Artificial Intelligence and Approximate Reasoning Group (GIARA) of the NUP/UPNA-Public University of Navarre and received an award from the European Association of Fuzzy Logic and Soft Computing (EUSFLAT) during its biennial meeting (EUSFLAT 2013), held in Milan (Italy), in September 2013.
The research has centered on the study of brain images obtained utilizing magnetic resonance imaging (MRI). Specifically, they have developed an algorithm to improve the process to segment the images. “By means of segmentation,” explained Dr. Aránzazu Jurío, one of the researchers of the study. “Each of the objects that make up the image is separated. Each pixel is analyzed so that all the ones sharing the same features are considered to form part of the same object.”
In the instance of medical images, this process is crucial for delimiting tumors where, if one is thinking about areas like those in the brain, 3 mm of difference can mean the difference between a cure and affecting areas controlling speech or vision.
To understand how the algorithm developed by the researchers works, Prof. Humberto Bustince, another investigator on the project, described the following parallel: “Imagine we have the image of a brain by means of magnetic resonance and seven doctors who have to decide how to delimit the tumor. From experience, we know that each one of them will separate the tumor differently. Now, with the proposed method, they will automatically be presented with a set of options, which, in any case, are always going to improve the choice that the worst of the seven may make. We’ve succeeding in improving on the worst: we’ve managed to ensure that even though they may go for the worst option, they will be wrong to the least extent possible.”
Another of the factors that specialists in artificial intelligence have to deal with is that of time variations, because medical images differ in time, and on occasions within a short space of time. “All these algorithms allow application in real time, because what may be useful for an image at a given moment may not be useful after some time,” explained Prof. Bustince.
In this respect, Dr. Jurío stressed that, “the algorithm we have developed produces a kind of consensus among the various functions that seek to obtain the best solution. We could say that it takes the process of choosing away from the expert because it is the algorithm that automatically selects the function.”
“The problem,” said coauthor of the study, Dr. Daniel Paternain, “is that for a specific image there are a number of functions that the expert may use, but if he or she gets the function wrong when doing the segmenting, the result could be devastating. What we tried to solve is this: if he/she gets the function wrong, the result may not be devastating.”
Related Links:
Artificial Intelligence and Approximate Reasoning Group
NUP/UPNA-Public University of Navarre
Latest Imaging IT News
- New Google Cloud Medical Imaging Suite Makes Imaging Healthcare Data More Accessible
- Global AI in Medical Diagnostics Market to Be Driven by Demand for Image Recognition in Radiology
- AI-Based Mammography Triage Software Helps Dramatically Improve Interpretation Process
- Artificial Intelligence (AI) Program Accurately Predicts Lung Cancer Risk from CT Images
- Image Management Platform Streamlines Treatment Plans
- AI-Based Technology for Ultrasound Image Analysis Receives FDA Approval
- AI Technology for Detecting Breast Cancer Receives CE Mark Approval
- Digital Pathology Software Improves Workflow Efficiency
- Patient-Centric Portal Facilitates Direct Imaging Access
- New Workstation Supports Customer-Driven Imaging Workflow
Channels
Radiography
view channel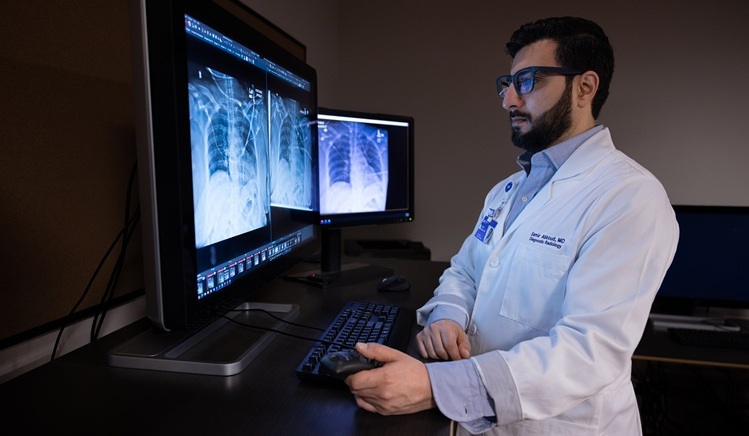
AI Radiology Tool Identifies Life-Threatening Conditions in Milliseconds
Radiology is emerging as one of healthcare’s most pressing bottlenecks. By 2033, the U.S. could face a shortage of up to 42,000 radiologists, even as imaging volumes grow by 5% annually.... Read more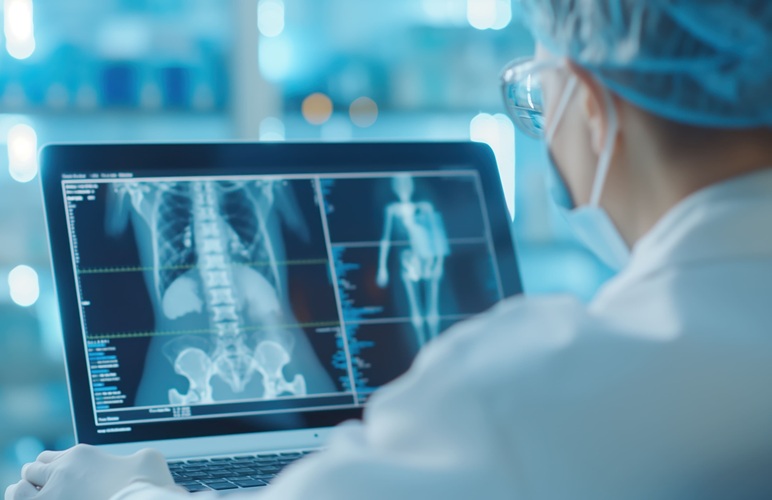
Machine Learning Algorithm Identifies Cardiovascular Risk from Routine Bone Density Scans
A new study published in the Journal of Bone and Mineral Research reveals that an automated machine learning program can predict the risk of cardiovascular events and falls or fractures by analyzing bone... Read more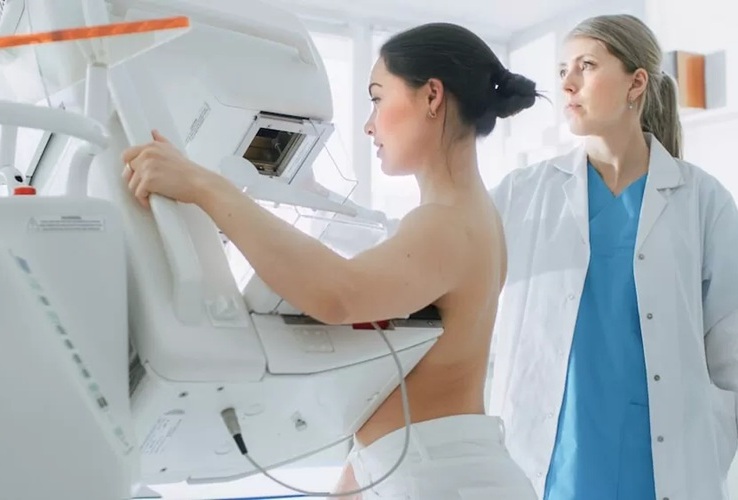
AI Improves Early Detection of Interval Breast Cancers
Interval breast cancers, which occur between routine screenings, are easier to treat when detected earlier. Early detection can reduce the need for aggressive treatments and improve the chances of better outcomes.... Read more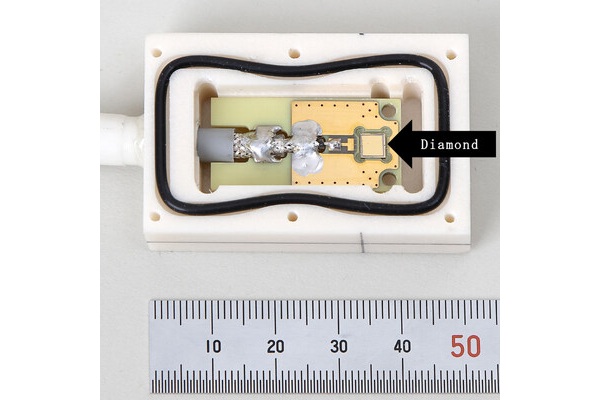
World's Largest Class Single Crystal Diamond Radiation Detector Opens New Possibilities for Diagnostic Imaging
Diamonds possess ideal physical properties for radiation detection, such as exceptional thermal and chemical stability along with a quick response time. Made of carbon with an atomic number of six, diamonds... Read moreMRI
view channel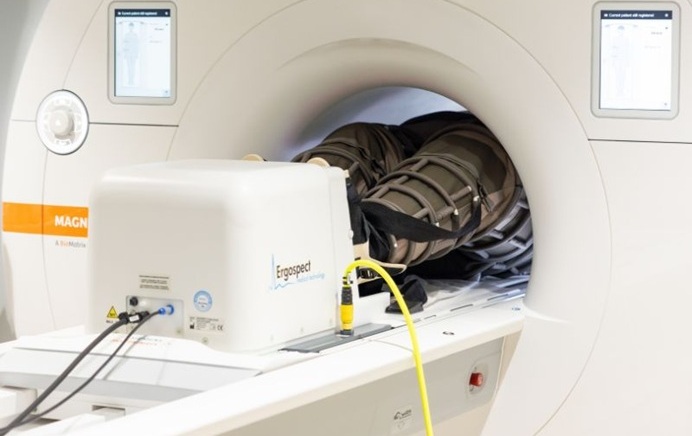
New MRI Technique Reveals Hidden Heart Issues
Traditional exercise stress tests conducted within an MRI machine require patients to lie flat, a position that artificially improves heart function by increasing stroke volume due to gravity-driven blood... Read more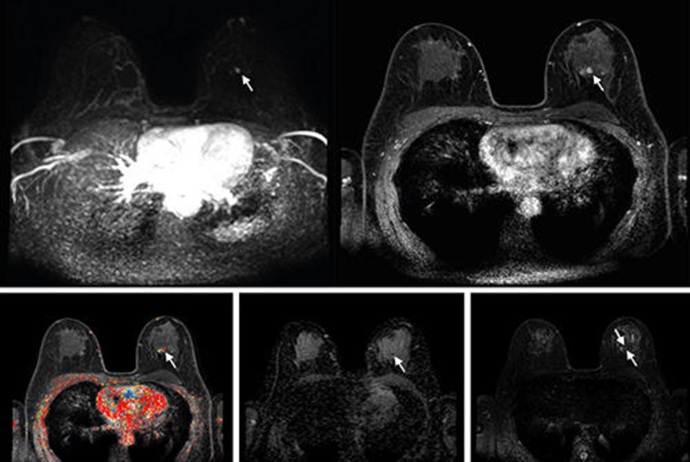
Shorter MRI Exam Effectively Detects Cancer in Dense Breasts
Women with extremely dense breasts face a higher risk of missed breast cancer diagnoses, as dense glandular and fibrous tissue can obscure tumors on mammograms. While breast MRI is recommended for supplemental... Read moreUltrasound
view channel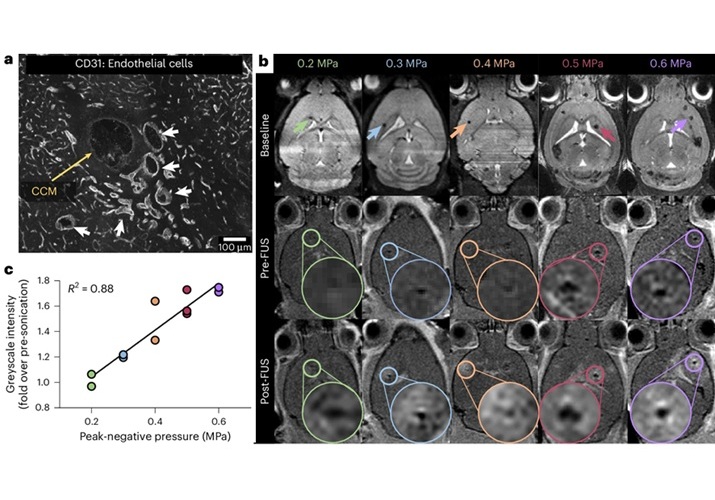
New Incision-Free Technique Halts Growth of Debilitating Brain Lesions
Cerebral cavernous malformations (CCMs), also known as cavernomas, are abnormal clusters of blood vessels that can grow in the brain, spinal cord, or other parts of the body. While most cases remain asymptomatic,... Read more.jpeg)
AI-Powered Lung Ultrasound Outperforms Human Experts in Tuberculosis Diagnosis
Despite global declines in tuberculosis (TB) rates in previous years, the incidence of TB rose by 4.6% from 2020 to 2023. Early screening and rapid diagnosis are essential elements of the World Health... Read moreNuclear Medicine
view channel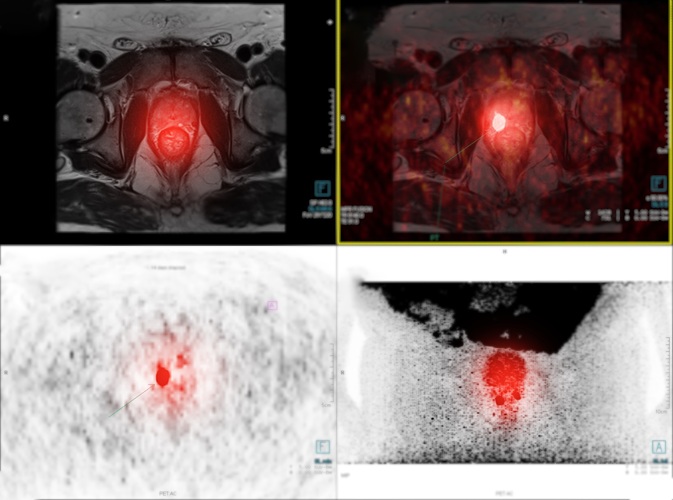
New Imaging Approach Could Reduce Need for Biopsies to Monitor Prostate Cancer
Prostate cancer is the second leading cause of cancer-related death among men in the United States. However, the majority of older men diagnosed with prostate cancer have slow-growing, low-risk forms of... Read more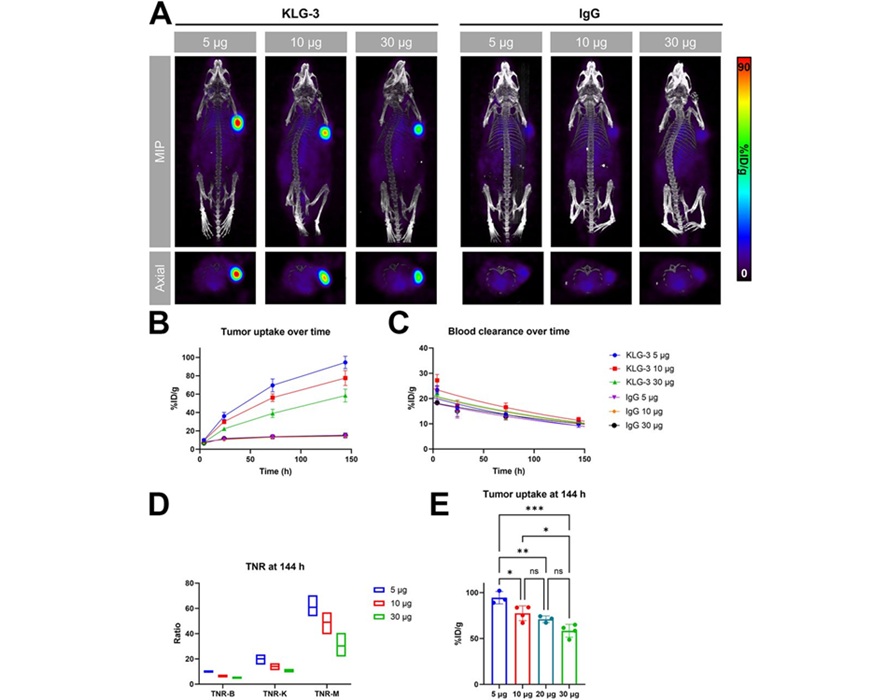
Novel Radiolabeled Antibody Improves Diagnosis and Treatment of Solid Tumors
Interleukin-13 receptor α-2 (IL13Rα2) is a cell surface receptor commonly found in solid tumors such as glioblastoma, melanoma, and breast cancer. It is minimally expressed in normal tissues, making it... Read moreGeneral/Advanced Imaging
view channel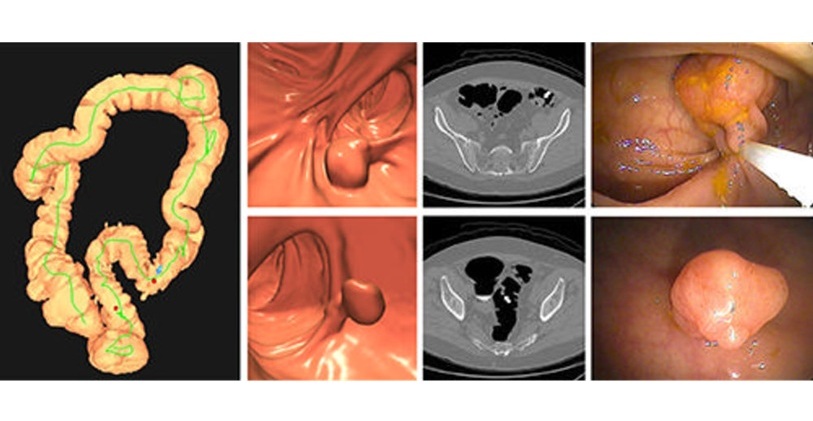
CT Colonography Beats Stool DNA Testing for Colon Cancer Screening
As colorectal cancer remains the second leading cause of cancer-related deaths worldwide, early detection through screening is vital to reduce advanced-stage treatments and associated costs.... Read more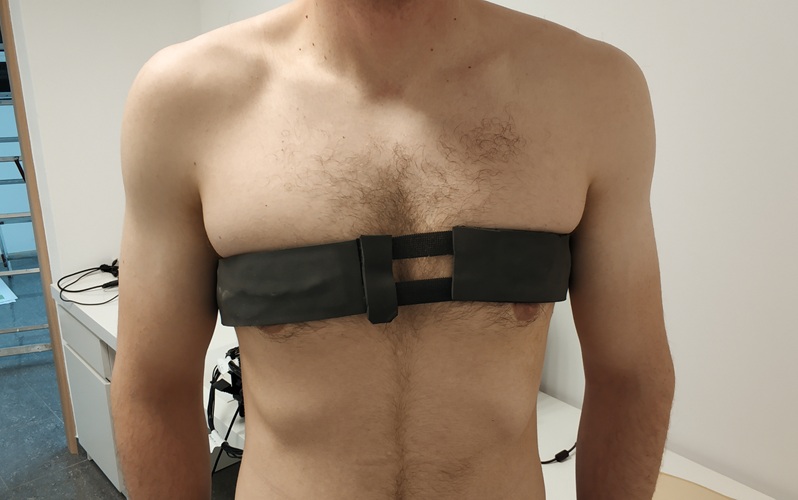
First-Of-Its-Kind Wearable Device Offers Revolutionary Alternative to CT Scans
Currently, patients with conditions such as heart failure, pneumonia, or respiratory distress often require multiple imaging procedures that are intermittent, disruptive, and involve high levels of radiation.... Read more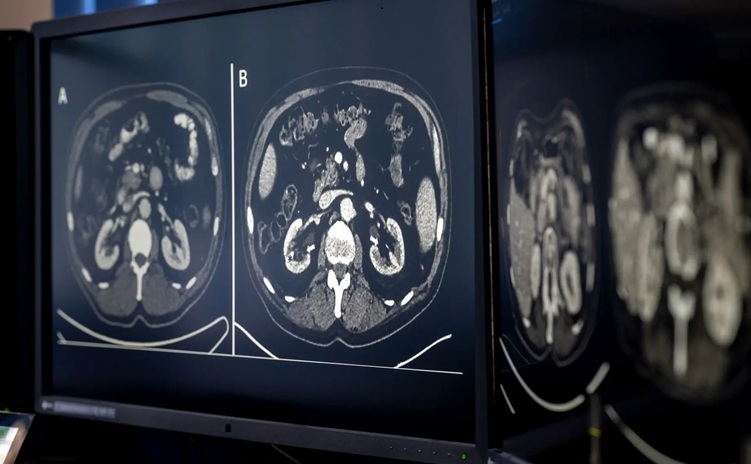
AI-Based CT Scan Analysis Predicts Early-Stage Kidney Damage Due to Cancer Treatments
Radioligand therapy, a form of targeted nuclear medicine, has recently gained attention for its potential in treating specific types of tumors. However, one of the potential side effects of this therapy... Read moreIndustry News
view channel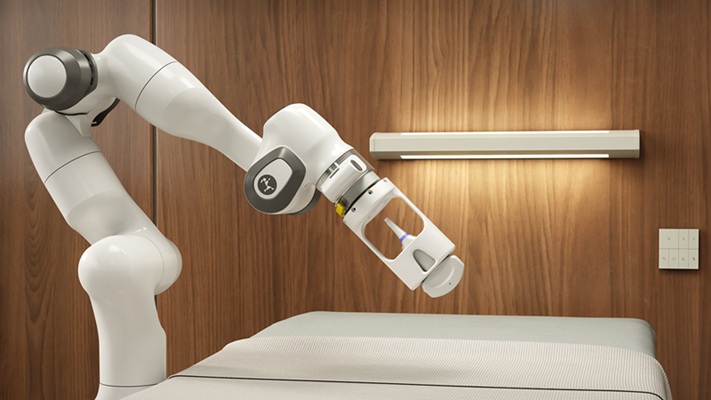
GE HealthCare and NVIDIA Collaboration to Reimagine Diagnostic Imaging
GE HealthCare (Chicago, IL, USA) has entered into a collaboration with NVIDIA (Santa Clara, CA, USA), expanding the existing relationship between the two companies to focus on pioneering innovation in... Read more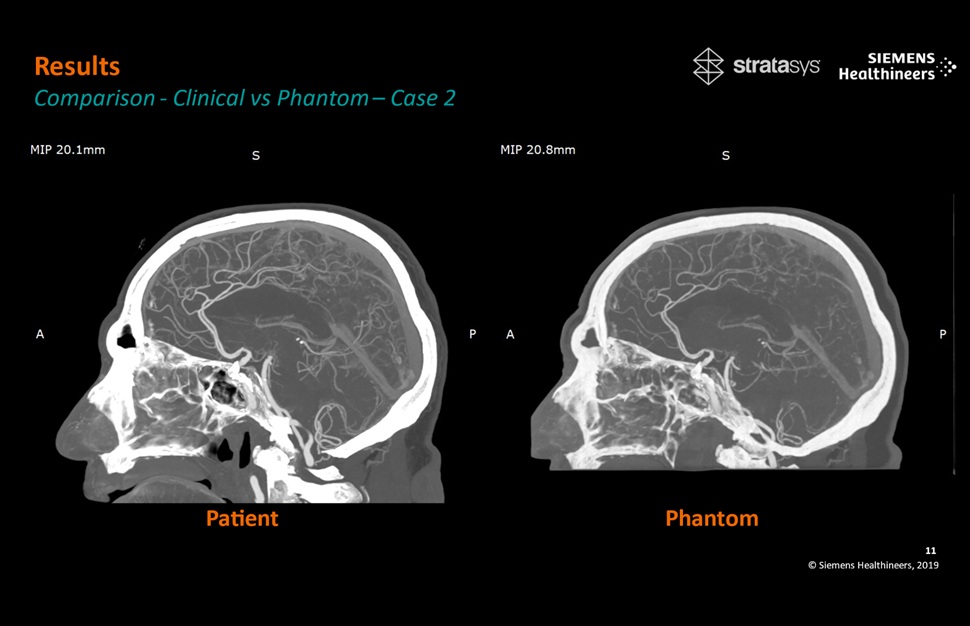
Patient-Specific 3D-Printed Phantoms Transform CT Imaging
New research has highlighted how anatomically precise, patient-specific 3D-printed phantoms are proving to be scalable, cost-effective, and efficient tools in the development of new CT scan algorithms... Read more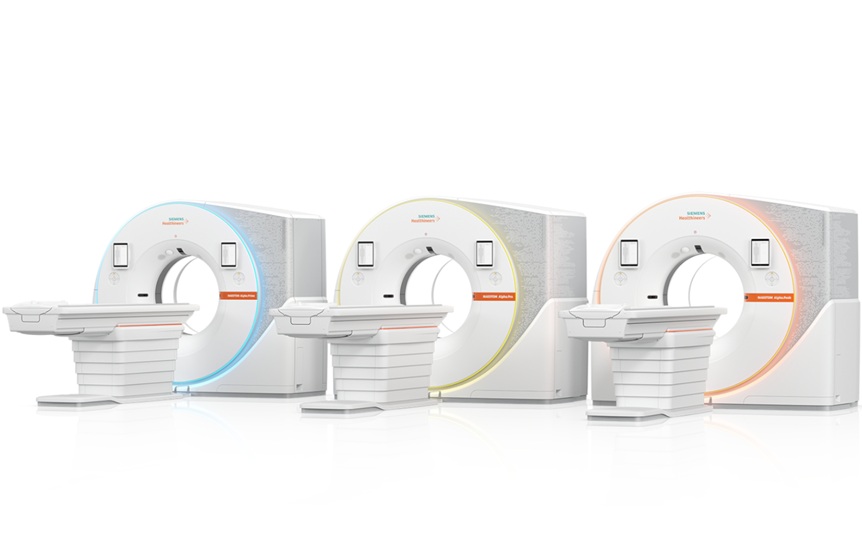
Siemens and Sectra Collaborate on Enhancing Radiology Workflows
Siemens Healthineers (Forchheim, Germany) and Sectra (Linköping, Sweden) have entered into a collaboration aimed at enhancing radiologists' diagnostic capabilities and, in turn, improving patient care... Read more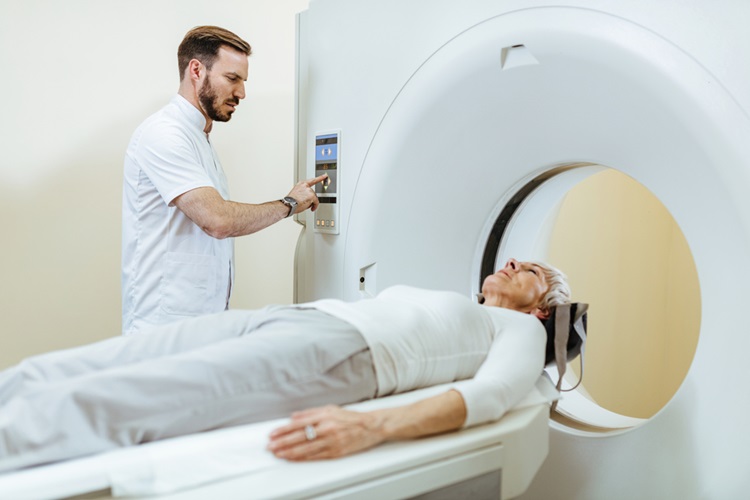