Machine Learning Algorithm Identifies Cardiovascular Risk from Routine Bone Density Scans
Posted on 16 May 2025
A new study published in the Journal of Bone and Mineral Research reveals that an automated machine learning program can predict the risk of cardiovascular events and falls or fractures by analyzing bone density scans taken during routine clinical tests.
In the study, researchers from Edith Cowan University (Joondalup, Western Australia), in collaboration with the University of Manitoba (Winnipeg, Canada), applied the machine learning algorithm to vertebral fracture assessment (VFA) images. These images are commonly obtained during routine bone density testing, often as part of osteoporosis treatment plans. The algorithm assessed the extent of abdominal aortic calcification (AAC) in the patients, which is an important marker for cardiovascular risk. The algorithm significantly reduced the time required for AAC screening, completing the task in less than a minute for thousands of images, compared to the five to six minutes it would take an experienced clinician to manually analyze a single image for the AAC score.
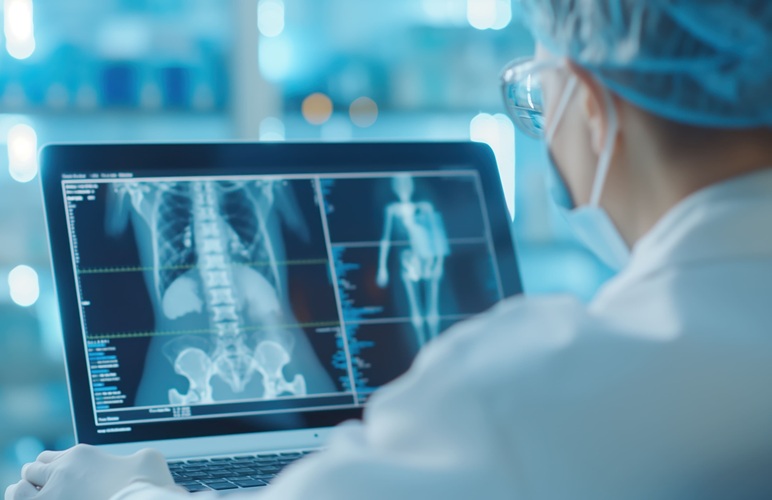
The study found that 58% of older individuals undergoing routine bone density testing exhibited moderate to high levels of AAC, with one in four of these individuals unaware of their condition, placing them at an elevated risk of heart attack and stroke. Additionally, the team discovered that patients with moderate to high AAC scores had an increased likelihood of being hospitalized due to falls or suffering fractures, compared to those with low AAC scores. The researchers suggest that applying this machine learning algorithm to bone density scans could provide clinicians with valuable insights into patients' vascular health, a key but often overlooked risk factor for falls and fractures.
“Women are recognized as being under screened and under-treated for cardiovascular disease. This study shows that we can use widely available, low radiation bone density machines to identify women at high risk of cardiovascular disease, which would allow them to seek treatment,” said ECU research fellow Dr. Cassandra Smith. “People who have AAC don’t present any symptoms, and without doing specific screening for AAC, this prognosis would often go unnoticed. By applying this algorithm during bone density scans, women have a much better chance of a diagnosis.”