Deep Learning Model Detects Lung Tumors on CT
By MedImaging International staff writers Posted on 27 Jan 2025 |
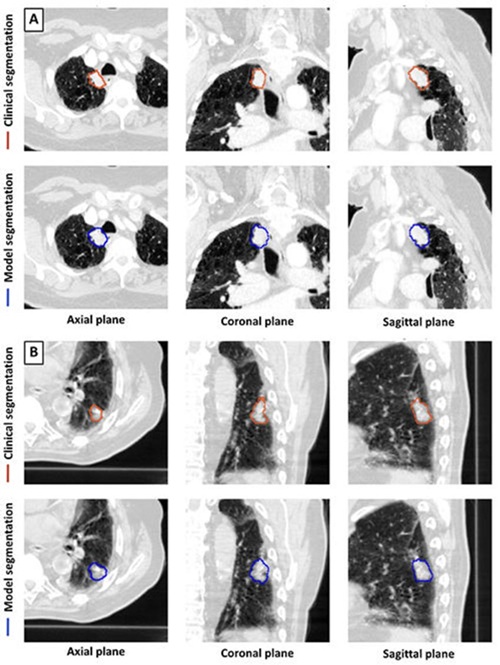
Lung cancer is the second most common cancer in the U.S. and the leading cause of cancer-related death. Accurately detecting and segmenting lung tumors on CT scans is essential for monitoring progression, evaluating treatment, and planning radiation therapy. Currently, this process is manually performed by clinicians, which is labor-intensive and prone to physician variability. While AI deep learning methods have been applied to tumor detection and segmentation, previous studies have been limited by small datasets, manual inputs, and a focus on single tumors. These limitations highlight the need for models that can provide robust, automated tumor delineation in various clinical settings. Now, a new study published in Radiology has shown promise for a new deep learning model capable of accurately detecting and segmenting lung tumors, positively impacting lung cancer treatment.
In this study, researchers at Stanford University School of Medicine (Stanford, CA, USA) used a large-scale dataset from routine pre-radiation treatment CT scans to develop a near-expert-level model for lung tumor detection and segmentation. Their aim was to create a model capable of identifying and segmenting lung tumors across different medical centers. The team used an ensemble 3D U-Net deep learning model trained on 1,504 CT scans with 1,828 segmented tumors and tested it on 150 CT scans. The model's predictions were compared with physician-delineated tumor volumes. Key performance metrics, such as sensitivity, specificity, false positive rate, and Dice similarity coefficient (DSC), were calculated to assess model accuracy. The model achieved 92% sensitivity and 82% specificity in detecting lung tumors. For scans with a single tumor, the median model-physician DSC was 0.77, and the physician-physician DSC was 0.80. The model also performed faster than physicians.
The researchers believe that the 3D U-Net architecture provides advantages over 2D models by capturing interslice information, which helps identify smaller lesions that may be confused with other structures. However, the model tended to underestimate tumor volume, especially for larger tumors, which could affect performance. The researchers recommend integrating this model into a physician-supervised workflow to allow clinicians to validate and correct any misidentified lesions. They also suggest future research to evaluate how the model can be applied to assess overall lung tumor burden and treatment responses over time, and whether it can predict clinical outcomes in combination with other prognostic models.
"To the best of our knowledge, our training dataset is the largest collection of CT scans and clinical tumor segmentations reported in the literature for constructing a lung tumor detection and segmentation model," said the study's lead author, Mehr Kashyap, M.D. "Our study represents an important step toward automating lung tumor identification and segmentation. This approach could have wide-ranging implications, including its incorporation in automated treatment planning, tumor burden quantification, treatment response assessment and other radiomic applications."
Related Links:
Stanford University School of Medicine
Latest General/Advanced Imaging News
- AI Reduces CT Lung Cancer Screening Workload by Almost 80%
- Cutting-Edge Technology Combines Light and Sound for Real-Time Stroke Monitoring
- AI System Detects Subtle Changes in Series of Medical Images Over Time
- New CT Scan Technique to Improve Prognosis and Treatments for Head and Neck Cancers
- World’s First Mobile Whole-Body CT Scanner to Provide Diagnostics at POC
- Comprehensive CT Scans Could Identify Atherosclerosis Among Lung Cancer Patients
- AI Improves Detection of Colorectal Cancer on Routine Abdominopelvic CT Scans
- Super-Resolution Technology Enhances Clinical Bone Imaging to Predict Osteoporotic Fracture Risk
- AI-Powered Abdomen Map Enables Early Cancer Detection
- AI Predicts Cardiovascular Risk from CT Scans
- Deep Learning Based Algorithms Improve Tumor Detection in PET/CT Scans
- New Technology Provides Coronary Artery Calcification Scoring on Ungated Chest CT Scans
- Deep Learning Model Accurately Diagnoses COPD Using Single Inhalation Lung CT Scan
- AI Model Reconstructs Sparse-View 3D CT Scan With Much Lower X-Ray Dose
- New Medical Scanner Identifies Brain Damage in Stroke Patients at Lower Magnetic Fields
- AI Tool Offers Opportunistic Screening for Heart Disease Using Repurposed CT Scans
Channels
Radiography
view channel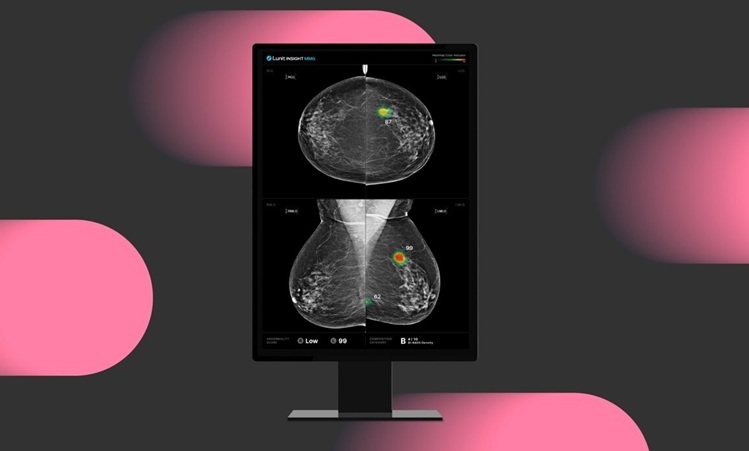
AI-Powered Mammography Screening Boosts Cancer Detection in Single-Reader Settings
A new study has revealed that an artificial intelligence (AI)-powered solution significantly improves cancer detection in single-reader mammography settings without increasing recall rates, offering a... Read more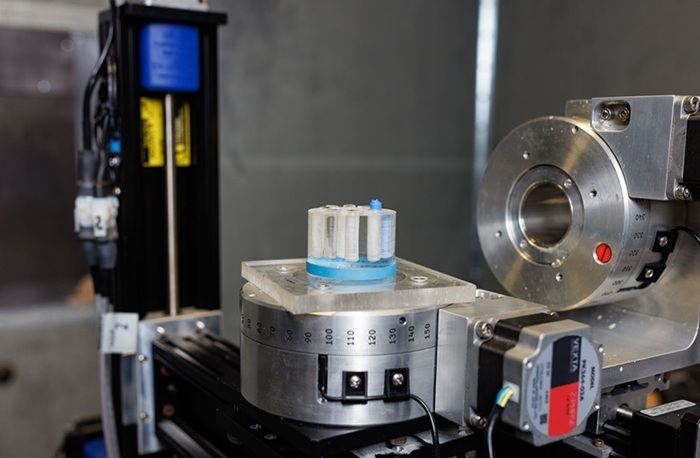
Photon Counting Detectors Promise Fast Color X-Ray Images
For many years, healthcare professionals have depended on traditional 2D X-rays to diagnose common bone fractures, though small fractures or soft tissue damage, such as cancers, can often be missed.... Read moreMRI
view channel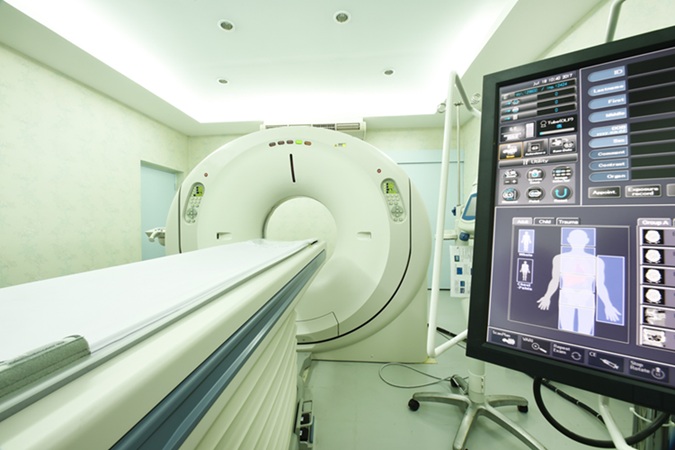
Biparametric MRI Combined with AI Enhances Detection of Clinically Significant Prostate Cancer
Artificial intelligence (AI) technologies are transforming the way medical images are analyzed, offering unprecedented capabilities in quantitatively extracting features that go beyond traditional visual... Read more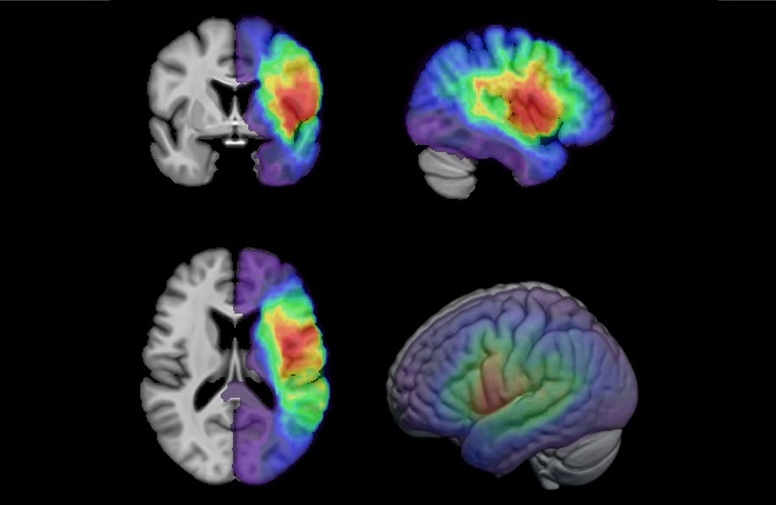
First-Of-Its-Kind AI-Driven Brain Imaging Platform to Better Guide Stroke Treatment Options
Each year, approximately 800,000 people in the U.S. experience strokes, with marginalized and minoritized groups being disproportionately affected. Strokes vary in terms of size and location within the... Read moreUltrasound
view channel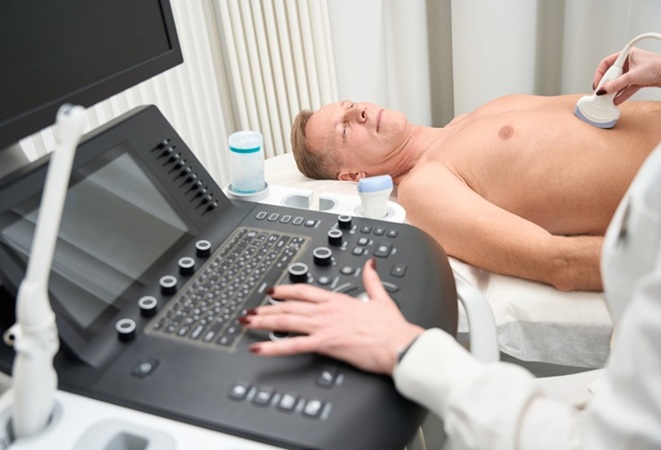
Artificial Intelligence Detects Undiagnosed Liver Disease from Echocardiograms
Echocardiography is a diagnostic procedure that uses ultrasound to visualize the heart and its associated structures. This imaging test is commonly used as an early screening method when doctors suspect... Read more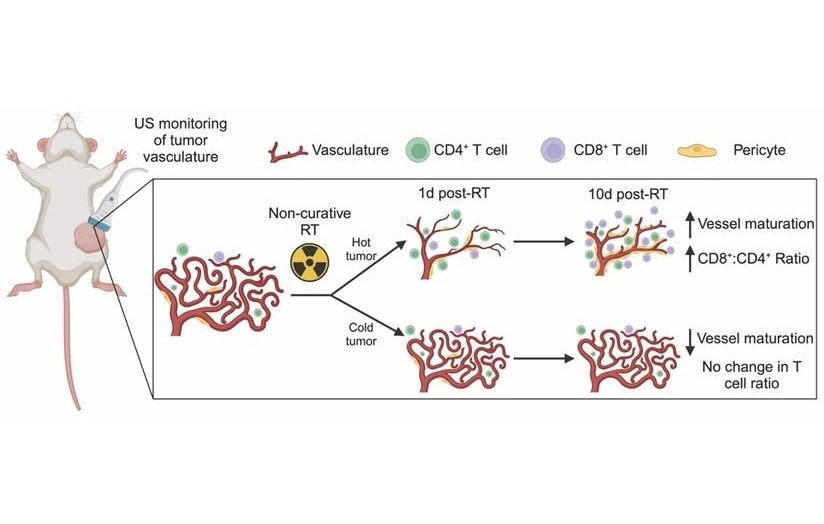
Ultrasound Imaging Non-Invasively Tracks Tumor Response to Radiation and Immunotherapy
While immunotherapy holds promise in the fight against triple-negative breast cancer, many patients fail to respond to current treatments. A major challenge has been predicting and monitoring how individual... Read moreNuclear Medicine
view channel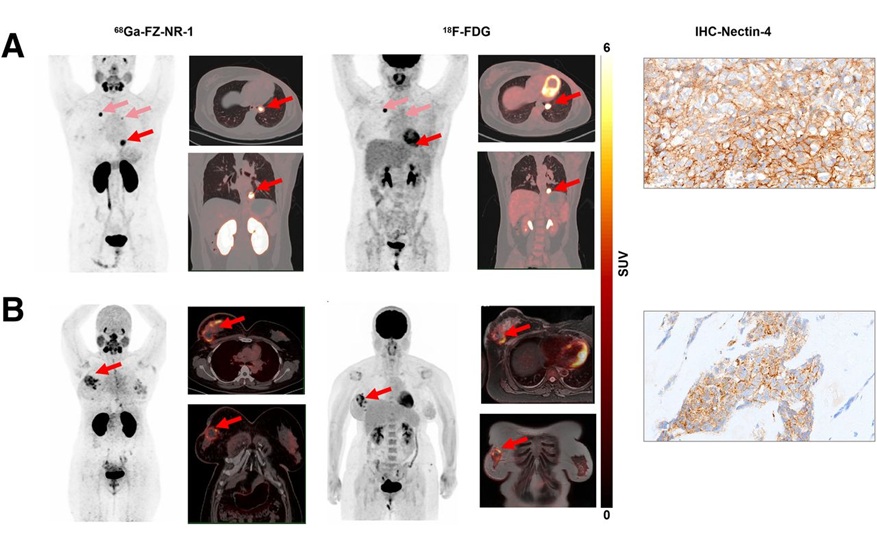
Novel Radiotracer Identifies Biomarker for Triple-Negative Breast Cancer
Triple-negative breast cancer (TNBC), which represents 15-20% of all breast cancer cases, is one of the most aggressive subtypes, with a five-year survival rate of about 40%. Due to its significant heterogeneity... Read more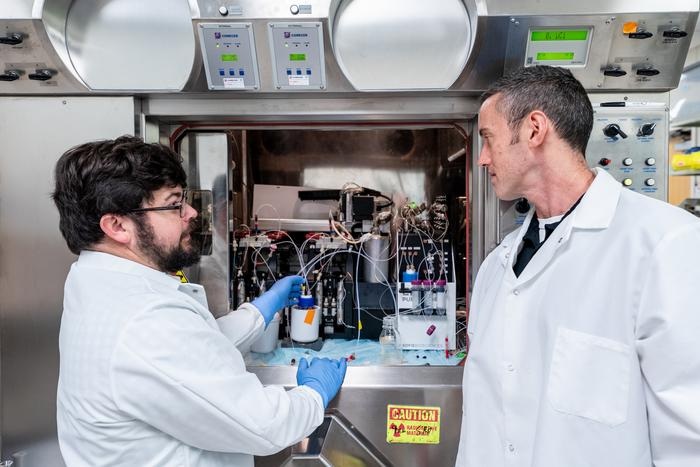
Innovative PET Imaging Technique to Help Diagnose Neurodegeneration
Neurodegenerative diseases, such as amyotrophic lateral sclerosis (ALS) and Alzheimer’s disease, are often diagnosed only after physical symptoms appear, by which time treatment may no longer be effective.... Read moreImaging IT
view channel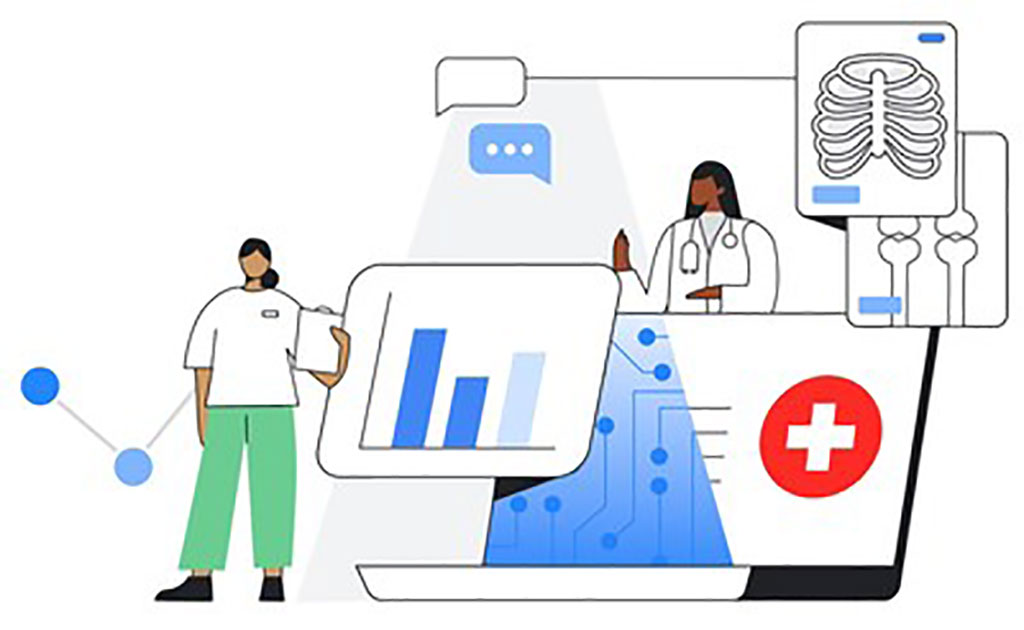
New Google Cloud Medical Imaging Suite Makes Imaging Healthcare Data More Accessible
Medical imaging is a critical tool used to diagnose patients, and there are billions of medical images scanned globally each year. Imaging data accounts for about 90% of all healthcare data1 and, until... Read more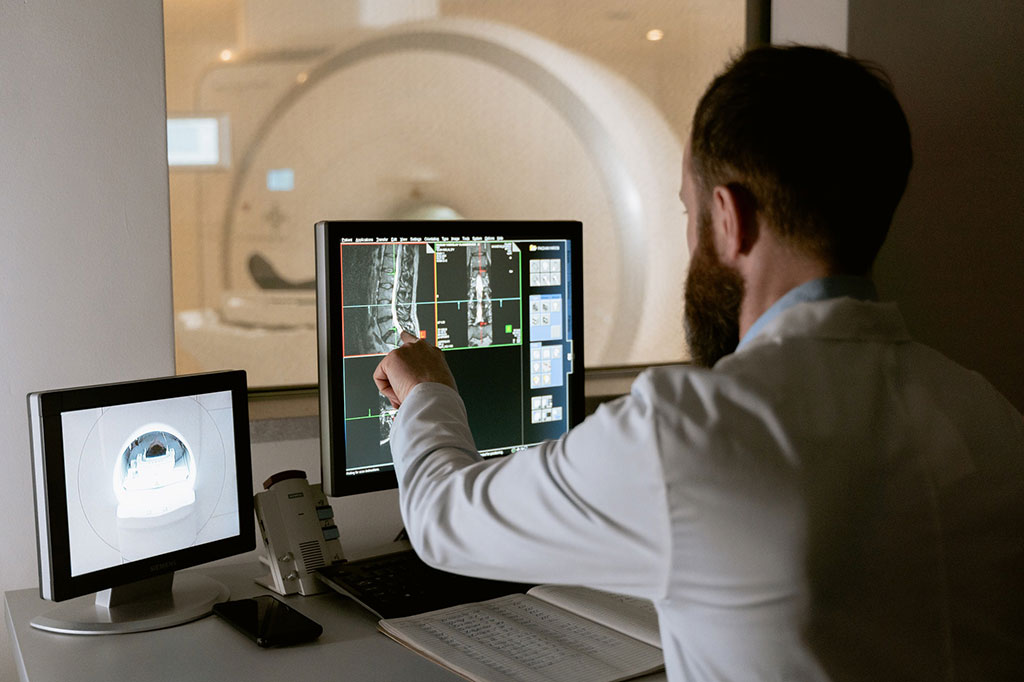
Global AI in Medical Diagnostics Market to Be Driven by Demand for Image Recognition in Radiology
The global artificial intelligence (AI) in medical diagnostics market is expanding with early disease detection being one of its key applications and image recognition becoming a compelling consumer proposition... Read moreIndustry News
view channel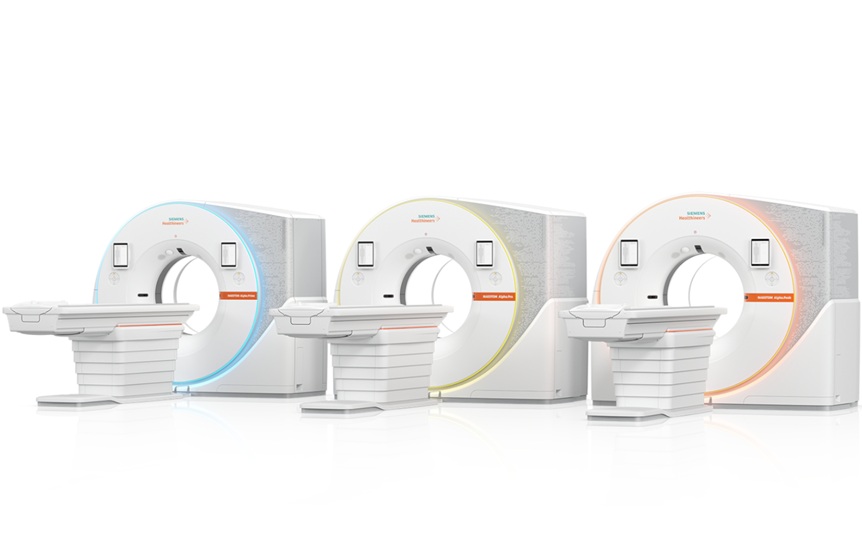
Siemens and Sectra Collaborate on Enhancing Radiology Workflows
Siemens Healthineers (Forchheim, Germany) and Sectra (Linköping, Sweden) have entered into a collaboration aimed at enhancing radiologists' diagnostic capabilities and, in turn, improving patient care... Read more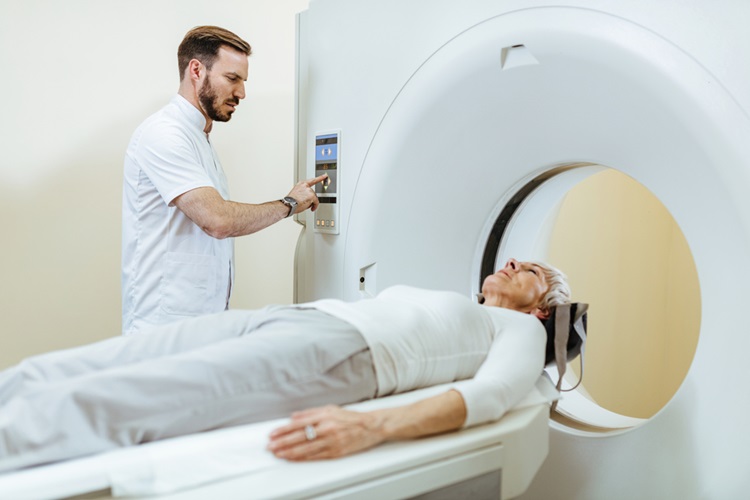