AI Improves Detection of Colorectal Cancer on Routine Abdominopelvic CT Scans
Posted on 17 Feb 2025
Radiologists often miss certain colorectal cancers (CRCs) during routine abdominopelvic CT scans, even when these cancers are visible in the images. Researchers have now developed and tested an artificial intelligence (AI) model designed to detect CRCs on routine abdominopelvic CT scans, even when no bowel preparation is used.
In a retrospective study conducted by researchers at Severance Hospital (Seoul, Korea), 3,945 patients were included. The study involved a training set of 2,662 patients with CRC who had undergone routine contrast-enhanced abdominopelvic CT before treatment. Additionally, test sets consisted of patients who had undergone routine contrast-enhanced abdominopelvic CT for various indications, along with colonoscopy within a two-month period between January and June 2018. A radiologist, using colonoscopy reports, identified which CRCs were visible on the CT scans and marked the lesions on all slices where CRC was detected, establishing the reference standard.
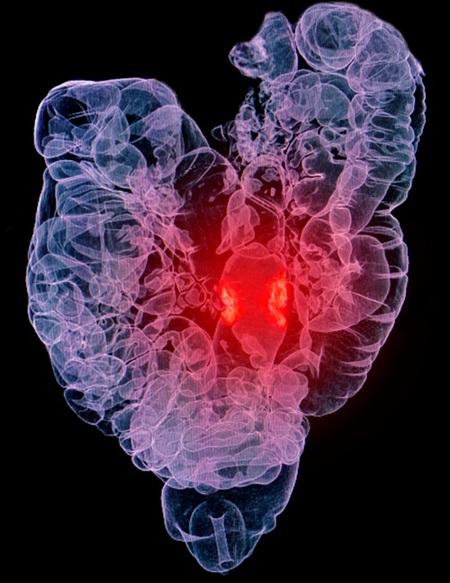
The researchers adapted and trained a modern transformer-based object detection network to develop an AI model that could automatically identify CRCs visible on unprocessed DICOM slices from CT scans. The AI model's performance was evaluated using alternative free-response ROC analysis, per-lesion sensitivity, and per-patient specificity, and its results in the external test set were compared to those of two radiologist readers. Clinical radiology reports were also reviewed. In both the internal test set (93 CT-visible CRCs in 92 patients) and the external test set (26 CT-visible CRCs in 26 patients), the AI model achieved an AUC of 0.867 and 0.808, sensitivity of 79.6% and 80.8%, and specificity of 91.2% and 90.9%, respectively.
In the external test set, the radiologists had sensitivities of 73.1% and 80.8%, and specificities of 98.3% and 98.6%. The AI model successfully detected five of the nine CRCs that were missed by at least one of the radiologists. Clinical radiology reports raised suspicion for 75.9% of CRCs in the external test set. The findings, published in the American Journal of Roentgenology, highlight the potential of the AI model for automating the detection of CRCs on routine abdominopelvic CT scans. Based on the results, the researchers concluded that the AI model could help reduce the number of missed CRCs during routine scans performed for purposes unrelated to CRC detection.