AI Model Significantly Enhances Low-Dose CT Capabilities
Posted on 28 Mar 2025
Lung cancer remains one of the most challenging diseases, making early diagnosis vital for effective treatment. Fortunately, advancements in artificial intelligence (AI) are revolutionizing lung cancer screening, enhancing both its accuracy and efficiency. While current screening methods, such as low-dose CT scans, assist in confirming the presence of lung cancer, they are often hampered by high false-positive rates and variability in detecting incidental but crucial findings, including those related to cardiovascular diseases. Furthermore, the global screening rate for low-dose CT remains under 10%, partly due to a shortage of radiologists. Now, a new study has presented a multimodal multitask foundation model that substantially improves the effectiveness of low-dose CT in lung cancer detection.
This innovative AI model, developed and tested by a team from Rensselaer Polytechnic Institute (RPI, Troy, NY, USA) in collaboration with other researchers, improves lung cancer risk prediction by 20% and cardiovascular risk prediction by 10%. It is the first model to tackle more than a dozen related tasks simultaneously, incorporating data from various sources, such as CT scans, radiology reports, patient risk factors, and other clinical findings. The study, published in Nature Communications, highlights the significant potential of this model in clinical settings. By integrating CT images with textual data, this model greatly improves the accuracy of lung cancer detection and prediction, which is crucial for enhancing patient outcomes. A key advantage of utilizing foundation models in medicine is their ability to improve performance on related tasks when trained with large-scale datasets, such as screening CT scans. For example, this model shows promise in advancing performance in oncology, a field where data specific to particular tasks is often scarce.
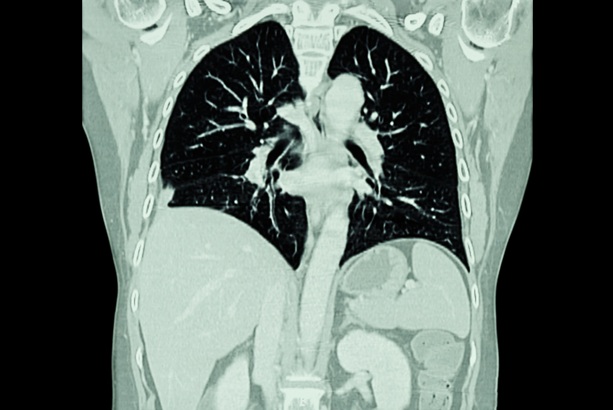
“This work has been significantly accelerated using RPI’s high-performance computing facility,” said Chuang Niu, Ph.D., research scientist at RPI and first author of the study. “Now, our multi-institutional team is further enhancing our foundation model on an increasing size of multimodal data, using both our own GPUs and New York State’s Empire AI high-performance computing facility. The collaboration across leading institutions underscores the growing synergy between artificial intelligence and medical research, with the potential to revolutionize how diseases are detected and treated.”
Related Links:
Rensselaer Polytechnic Institute