AI Method Accurately Predicts Breast Cancer Risk by Analyzing Multiple Mammograms
By MedImaging International staff writers Posted on 09 Dec 2024 |
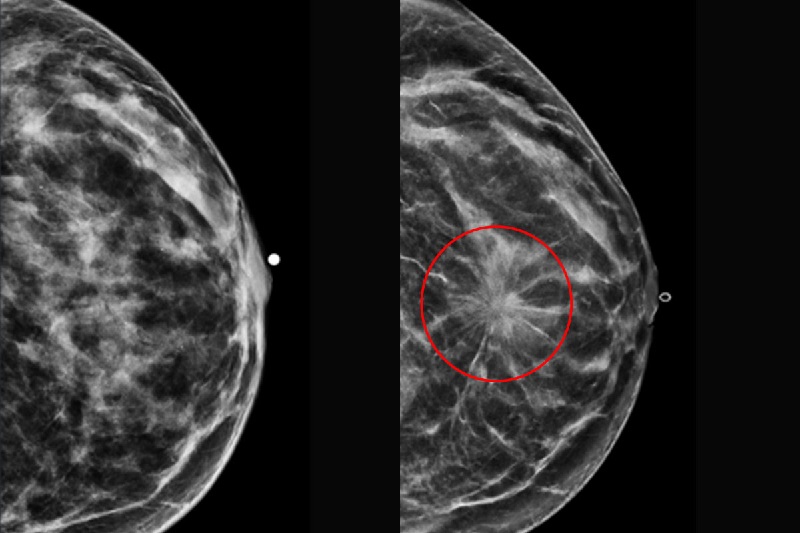
Currently, there is no way to predict who is at risk of developing breast cancer based on mammogram images alone. While some risk-reduction strategies are available, such as the use of tamoxifen, these treatments may cause unwanted side effects. Most high-risk women are offered more frequent screenings or the option to include additional imaging methods, like MRIs, to detect cancer early. Now, a new study published in JCO Clinical Cancer Informatics introduces a breakthrough approach to analyzing mammograms, significantly enhancing the accuracy of predicting a woman’s risk of developing breast cancer in the next five years. By incorporating up to three years of previous mammograms, this new method identified individuals at high risk of developing breast cancer 2.3 times more accurately than the standard method, which relies solely on questionnaires about clinical risk factors such as age, race, and family history.
This risk-prediction model builds upon previous research conducted by Washington University School of Medicine (St. Louis, MO, USA), which revealed that earlier mammograms contain valuable insights into the early stages of breast cancer that may go unnoticed by even well-trained professionals. These insights include subtle shifts in breast density, which measures the relative amounts of fibrous and fatty tissue in the breasts. In the latest study, the team developed an artificial intelligence-based algorithm that can detect minute differences in mammograms, helping to identify women at the highest risk of developing breast cancer over a specific time period. Apart from breast density, the algorithm also evaluates other patterns, such as changes in texture, calcification, and asymmetry in the images. The new method is capable of spotting small changes over time in repeated mammogram images that are not visible to the human eye but are rich in information crucial for identifying high-risk individuals.
The researchers trained their machine-learning algorithm using mammograms from more than 10,000 women who had breast cancer screenings between 2008 and 2012. These women were followed until 2020, with 478 diagnosed with breast cancer during that period. The team then applied their method to a separate dataset of over 18,000 women who had mammograms between 2013 and 2020, with 332 of these women being diagnosed with breast cancer during follow-up. According to the new prediction model, women identified as high-risk were 21 times more likely to be diagnosed with breast cancer in the next five years compared to those in the lowest-risk group. In the high-risk group, 53 out of every 1,000 women screened developed breast cancer, while in the low-risk group, only 2.6 women per 1,000 screened developed breast cancer. In comparison, the older, questionnaire-based method correctly identified only 23 women per 1,000 as high-risk, missing 30 breast cancer cases that the new method detected.
The mammograms were taken from both academic medical centers and community clinics, demonstrating that the accuracy of this method remains consistent across various healthcare settings. Importantly, the algorithm was designed with significant representation of Black women, a group often underrepresented in breast cancer risk models. The predictive accuracy was consistent across racial groups. Ongoing work includes testing the algorithm in women from diverse racial and ethnic backgrounds, including Asian, Southeast Asian, and Native American women, to ensure its effectiveness for all populations. The researchers are also working on securing patents and licensing for this new method, with plans to make it widely available wherever screening mammograms are provided.
“Today, we don’t have a way to know who is likely to develop breast cancer in the future based on their mammogram images,” said co-author Debbie L. Bennett, MD, an associate professor of radiology and chief of breast imaging for the Mallinckrodt Institute of Radiology at WashU Medicine. “What’s so exciting about this research is that it indicates that it is possible to glean this information from current and prior mammograms using this algorithm. The prediction is never going to be perfect, but this study suggests the new algorithm is much better than our current methods.”
Latest Radiography News
- World's Largest Class Single Crystal Diamond Radiation Detector Opens New Possibilities for Diagnostic Imaging
- AI-Powered Imaging Technique Shows Promise in Evaluating Patients for PCI
- Higher Chest X-Ray Usage Catches Lung Cancer Earlier and Improves Survival
- AI-Powered Mammograms Predict Cardiovascular Risk
- Generative AI Model Significantly Reduces Chest X-Ray Reading Time
- AI-Powered Mammography Screening Boosts Cancer Detection in Single-Reader Settings
- Photon Counting Detectors Promise Fast Color X-Ray Images
- AI Can Flag Mammograms for Supplemental MRI
- 3D CT Imaging from Single X-Ray Projection Reduces Radiation Exposure
- Printable Organic X-Ray Sensors Could Transform Treatment for Cancer Patients
- Highly Sensitive, Foldable Detector to Make X-Rays Safer
- Novel Breast Cancer Screening Technology Could Offer Superior Alternative to Mammogram
- Artificial Intelligence Accurately Predicts Breast Cancer Years Before Diagnosis
- AI-Powered Chest X-Ray Detects Pulmonary Nodules Three Years Before Lung Cancer Symptoms
- AI Model Identifies Vertebral Compression Fractures in Chest Radiographs
- Advanced 3D Mammography Detects More Breast Cancers
Channels
MRI
view channel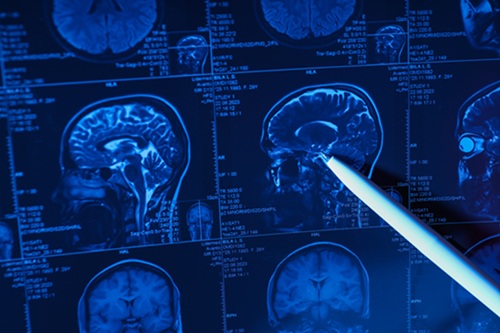
AI Tool Predicts Relapse of Pediatric Brain Cancer from Brain MRI Scans
Many pediatric gliomas are treatable with surgery alone, but relapses can be catastrophic. Predicting which patients are at risk for recurrence remains challenging, leading to frequent follow-ups with... Read more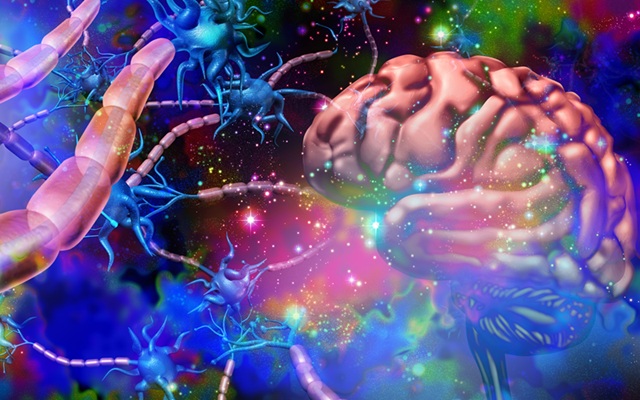
AI Tool Tracks Effectiveness of Multiple Sclerosis Treatments Using Brain MRI Scans
Multiple sclerosis (MS) is a condition in which the immune system attacks the brain and spinal cord, leading to impairments in movement, sensation, and cognition. Magnetic Resonance Imaging (MRI) markers... Read more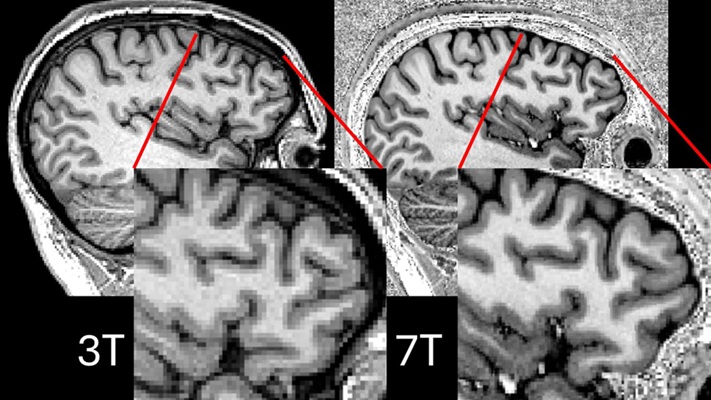
Ultra-Powerful MRI Scans Enable Life-Changing Surgery in Treatment-Resistant Epileptic Patients
Approximately 360,000 individuals in the UK suffer from focal epilepsy, a condition in which seizures spread from one part of the brain. Around a third of these patients experience persistent seizures... Read moreUltrasound
view channel.jpeg)
AI-Powered Lung Ultrasound Outperforms Human Experts in Tuberculosis Diagnosis
Despite global declines in tuberculosis (TB) rates in previous years, the incidence of TB rose by 4.6% from 2020 to 2023. Early screening and rapid diagnosis are essential elements of the World Health... Read more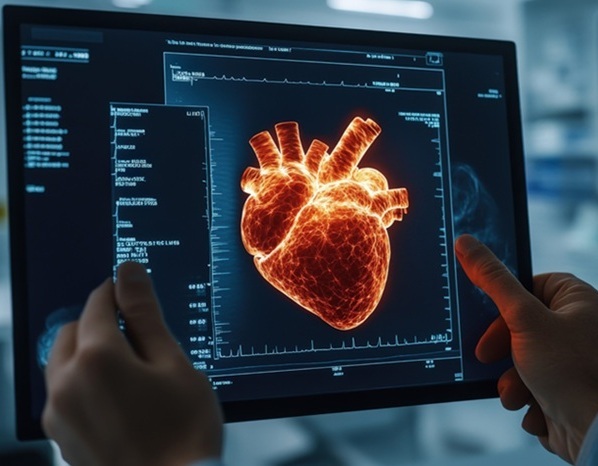
AI Identifies Heart Valve Disease from Common Imaging Test
Tricuspid regurgitation is a condition where the heart's tricuspid valve does not close completely during contraction, leading to backward blood flow, which can result in heart failure. A new artificial... Read moreNuclear Medicine
view channel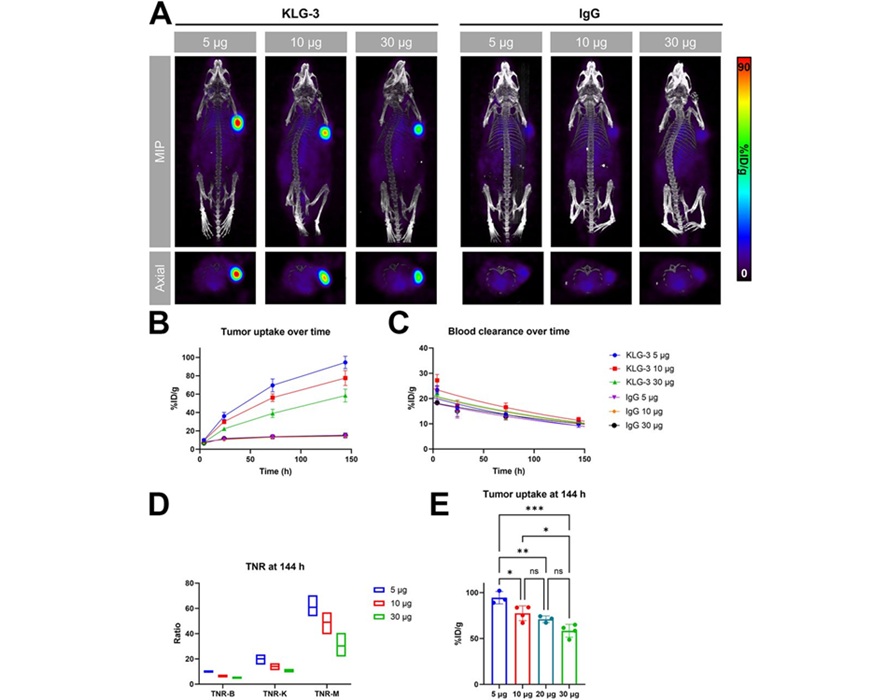
Novel Radiolabeled Antibody Improves Diagnosis and Treatment of Solid Tumors
Interleukin-13 receptor α-2 (IL13Rα2) is a cell surface receptor commonly found in solid tumors such as glioblastoma, melanoma, and breast cancer. It is minimally expressed in normal tissues, making it... Read more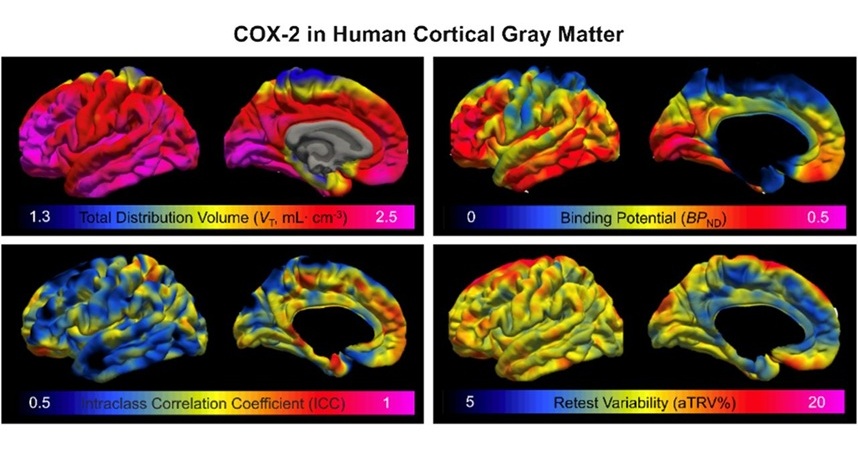
Novel PET Imaging Approach Offers Never-Before-Seen View of Neuroinflammation
COX-2, an enzyme that plays a key role in brain inflammation, can be significantly upregulated by inflammatory stimuli and neuroexcitation. Researchers suggest that COX-2 density in the brain could serve... Read moreGeneral/Advanced Imaging
view channel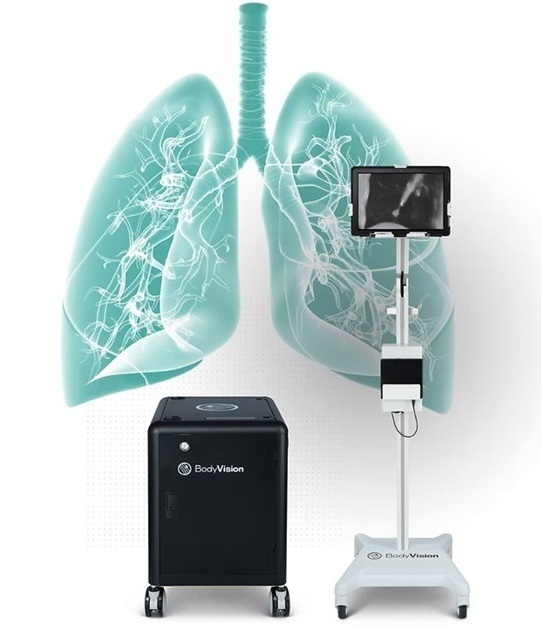
AI-Powered Imaging System Improves Lung Cancer Diagnosis
Given the need to detect lung cancer at earlier stages, there is an increasing need for a definitive diagnostic pathway for patients with suspicious pulmonary nodules. However, obtaining tissue samples... Read more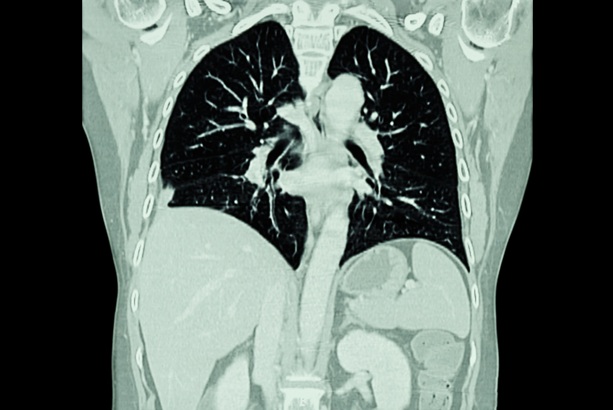
AI Model Significantly Enhances Low-Dose CT Capabilities
Lung cancer remains one of the most challenging diseases, making early diagnosis vital for effective treatment. Fortunately, advancements in artificial intelligence (AI) are revolutionizing lung cancer... Read moreImaging IT
view channel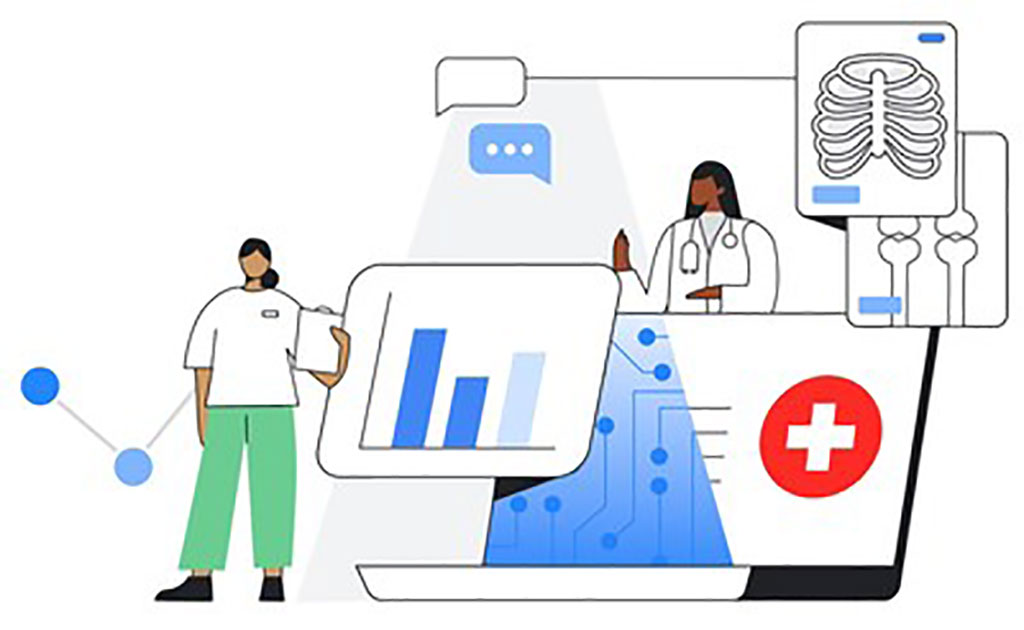
New Google Cloud Medical Imaging Suite Makes Imaging Healthcare Data More Accessible
Medical imaging is a critical tool used to diagnose patients, and there are billions of medical images scanned globally each year. Imaging data accounts for about 90% of all healthcare data1 and, until... Read more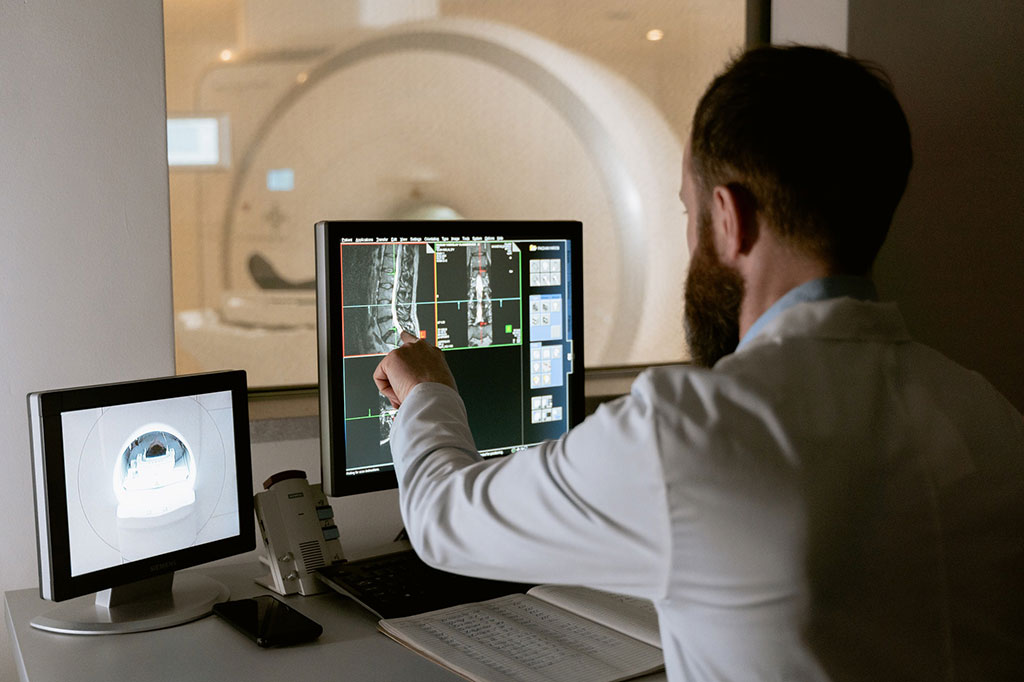
Global AI in Medical Diagnostics Market to Be Driven by Demand for Image Recognition in Radiology
The global artificial intelligence (AI) in medical diagnostics market is expanding with early disease detection being one of its key applications and image recognition becoming a compelling consumer proposition... Read moreIndustry News
view channel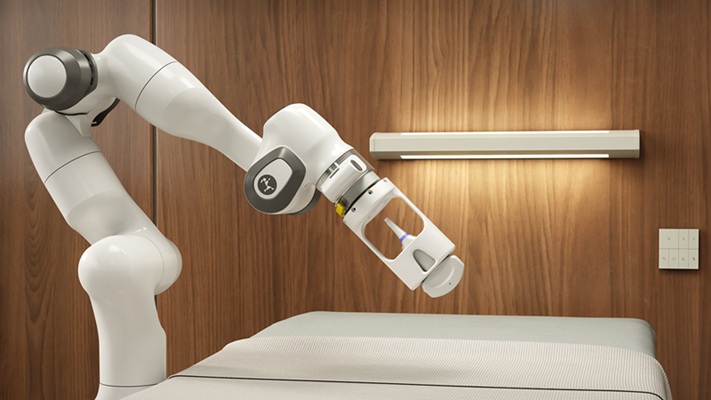
GE HealthCare and NVIDIA Collaboration to Reimagine Diagnostic Imaging
GE HealthCare (Chicago, IL, USA) has entered into a collaboration with NVIDIA (Santa Clara, CA, USA), expanding the existing relationship between the two companies to focus on pioneering innovation in... Read more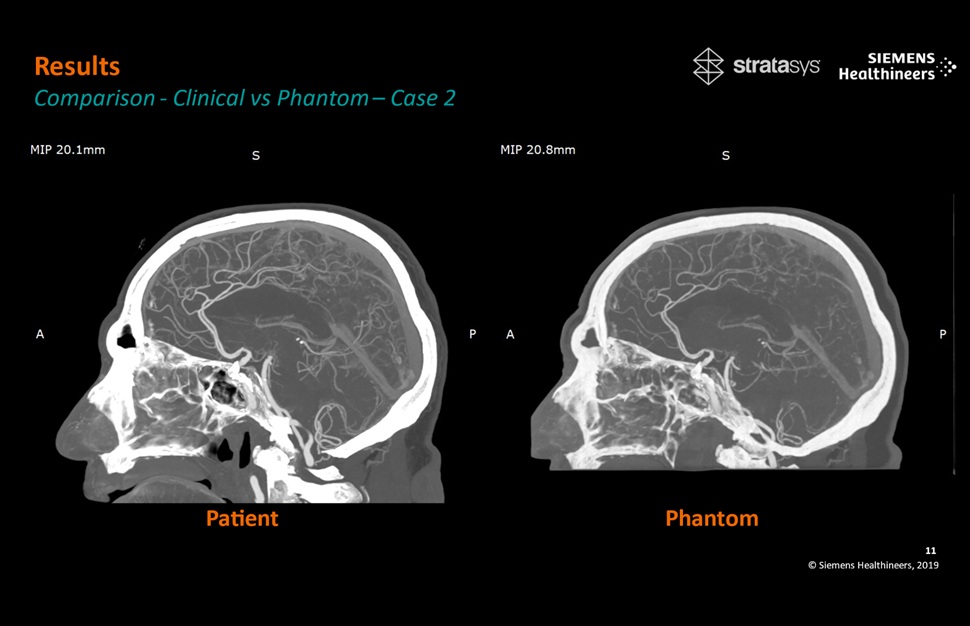
Patient-Specific 3D-Printed Phantoms Transform CT Imaging
New research has highlighted how anatomically precise, patient-specific 3D-printed phantoms are proving to be scalable, cost-effective, and efficient tools in the development of new CT scan algorithms... Read more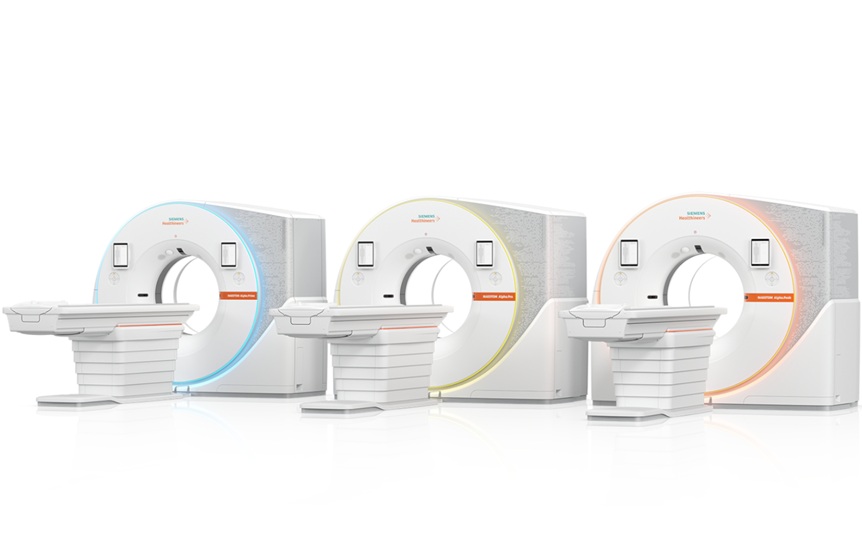
Siemens and Sectra Collaborate on Enhancing Radiology Workflows
Siemens Healthineers (Forchheim, Germany) and Sectra (Linköping, Sweden) have entered into a collaboration aimed at enhancing radiologists' diagnostic capabilities and, in turn, improving patient care... Read more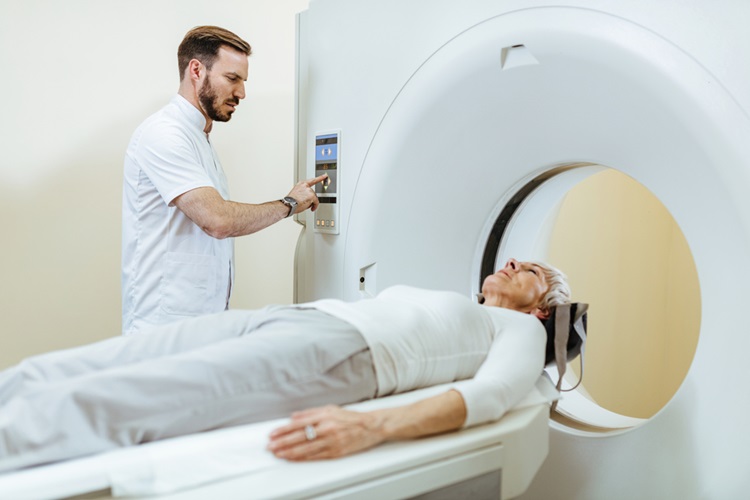