Radiomics Predicts Patient Benefits from Chemotherapy
By MedImaging International staff writers Posted on 04 Apr 2019 |
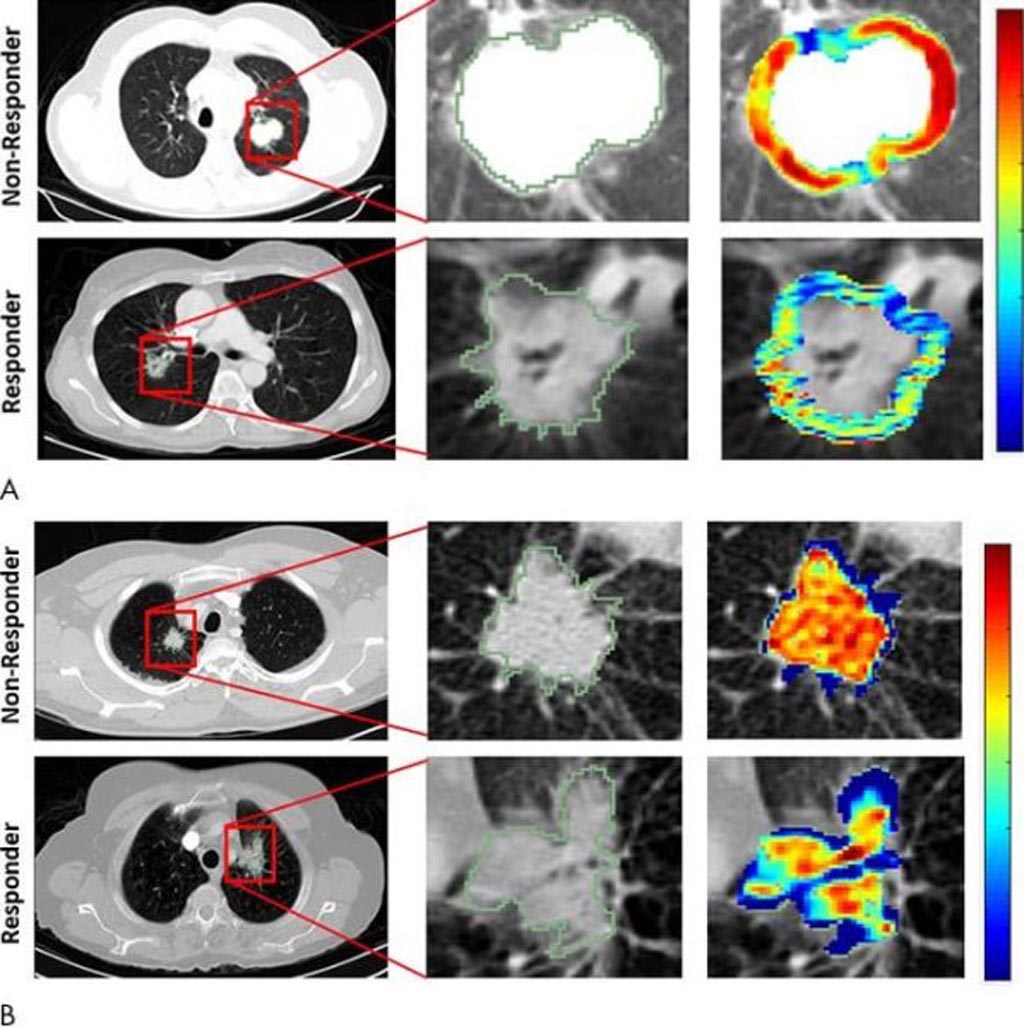
Image: Radiomic feature maps show differences between lesions in responders and nonresponders at pretreatment CT (Photo courtesy of Cleveland Clinic).
Data retrieved from computed tomography (CT) images may be able to forecast which lung cancer patients will respond to chemotherapy, claims a new study.
Researchers at Case Western Reserve University (CWRU; Cleveland, OH, USA), the Cleveland Clinic (CC; OH, USA), and other institutions retrospectively analyzed data from 125 patients with non–small cell lung cancer (NSCLC) who had been treated with pemetrexed-based platinum doublet chemotherapy, in order to identify the role of radiomics texture features--both within and outside the nodule--in predicting time to progression (TTP), overall survival (OS), and response to chemotherapy.
The patients were divided randomly into two sets, with the constraint that there were an equal number of responders and nonresponders in the training set. The training set comprised 53 patients with NSCLC, and the validation set comprised 72 patients. A machine learning classifier trained with radiomic texture features extracted from intra- and peritumoral regions of non–contrast-enhanced CT images was used to predict response to chemotherapy. The radiomic risk-score signature was generated by using least absolute shrinkage and selection operator, and association of the radiomic signature with TTP and OS was also evaluated.
The results showed that radiomic features derived from within the tumor and from the area around the tumor were able to distinguish between patients who responded to chemotherapy and those who did not with an accuracy of 0.77. In addition, the radiomic features predicted TTP and OS, and decision curve analysis demonstrated that in terms of clinical usefulness, the radiomics signature had a higher overall net benefit in prediction of high-risk patients to receive treatment than clinical-pathologic measurements. The study was published on March 20, 2019, in Radiology: Artificial Intelligence.
“This is the first study to demonstrate that computer-extracted patterns of heterogeneity, or diversity, from outside the tumor were predictive of response to chemotherapy,” said co-lead author Monica Khunger, MD, of the CC. “This is very critical because it could allow for predicting in advance of therapy which patients with lung cancer are likely to respond or not. This could help identify patients who are likely to not respond to chemotherapy for alternative therapies such as radiation or immunotherapy.”
Radiomics is an extension of computer-aided diagnosis, and refers to the comprehensive quantification of tumor phenotypes by extracting a large number of quantitative image features for data mining and precision medicine. In recent years radiomics has successfully extracted a variety of clinically relevant features, merging them into signatures, in order to estimate the probability of malignancy of identified breast cancer lesions.
Related Links:
Case Western Reserve University
Cleveland Clinic
Researchers at Case Western Reserve University (CWRU; Cleveland, OH, USA), the Cleveland Clinic (CC; OH, USA), and other institutions retrospectively analyzed data from 125 patients with non–small cell lung cancer (NSCLC) who had been treated with pemetrexed-based platinum doublet chemotherapy, in order to identify the role of radiomics texture features--both within and outside the nodule--in predicting time to progression (TTP), overall survival (OS), and response to chemotherapy.
The patients were divided randomly into two sets, with the constraint that there were an equal number of responders and nonresponders in the training set. The training set comprised 53 patients with NSCLC, and the validation set comprised 72 patients. A machine learning classifier trained with radiomic texture features extracted from intra- and peritumoral regions of non–contrast-enhanced CT images was used to predict response to chemotherapy. The radiomic risk-score signature was generated by using least absolute shrinkage and selection operator, and association of the radiomic signature with TTP and OS was also evaluated.
The results showed that radiomic features derived from within the tumor and from the area around the tumor were able to distinguish between patients who responded to chemotherapy and those who did not with an accuracy of 0.77. In addition, the radiomic features predicted TTP and OS, and decision curve analysis demonstrated that in terms of clinical usefulness, the radiomics signature had a higher overall net benefit in prediction of high-risk patients to receive treatment than clinical-pathologic measurements. The study was published on March 20, 2019, in Radiology: Artificial Intelligence.
“This is the first study to demonstrate that computer-extracted patterns of heterogeneity, or diversity, from outside the tumor were predictive of response to chemotherapy,” said co-lead author Monica Khunger, MD, of the CC. “This is very critical because it could allow for predicting in advance of therapy which patients with lung cancer are likely to respond or not. This could help identify patients who are likely to not respond to chemotherapy for alternative therapies such as radiation or immunotherapy.”
Radiomics is an extension of computer-aided diagnosis, and refers to the comprehensive quantification of tumor phenotypes by extracting a large number of quantitative image features for data mining and precision medicine. In recent years radiomics has successfully extracted a variety of clinically relevant features, merging them into signatures, in order to estimate the probability of malignancy of identified breast cancer lesions.
Related Links:
Case Western Reserve University
Cleveland Clinic
Latest General/Advanced Imaging News
- AI-Powered Imaging System Improves Lung Cancer Diagnosis
- AI Model Significantly Enhances Low-Dose CT Capabilities
- Ultra-Low Dose CT Aids Pneumonia Diagnosis in Immunocompromised Patients
- AI Reduces CT Lung Cancer Screening Workload by Almost 80%
- Cutting-Edge Technology Combines Light and Sound for Real-Time Stroke Monitoring
- AI System Detects Subtle Changes in Series of Medical Images Over Time
- New CT Scan Technique to Improve Prognosis and Treatments for Head and Neck Cancers
- World’s First Mobile Whole-Body CT Scanner to Provide Diagnostics at POC
- Comprehensive CT Scans Could Identify Atherosclerosis Among Lung Cancer Patients
- AI Improves Detection of Colorectal Cancer on Routine Abdominopelvic CT Scans
- Super-Resolution Technology Enhances Clinical Bone Imaging to Predict Osteoporotic Fracture Risk
- AI-Powered Abdomen Map Enables Early Cancer Detection
- Deep Learning Model Detects Lung Tumors on CT
- AI Predicts Cardiovascular Risk from CT Scans
- Deep Learning Based Algorithms Improve Tumor Detection in PET/CT Scans
- New Technology Provides Coronary Artery Calcification Scoring on Ungated Chest CT Scans
Channels
Radiography
view channel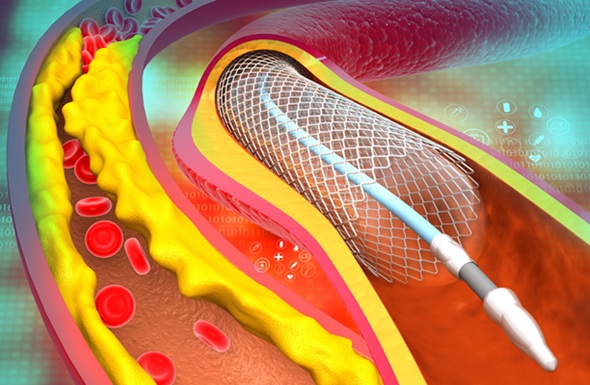
AI-Powered Imaging Technique Shows Promise in Evaluating Patients for PCI
Percutaneous coronary intervention (PCI), also known as coronary angioplasty, is a minimally invasive procedure where small metal tubes called stents are inserted into partially blocked coronary arteries... Read more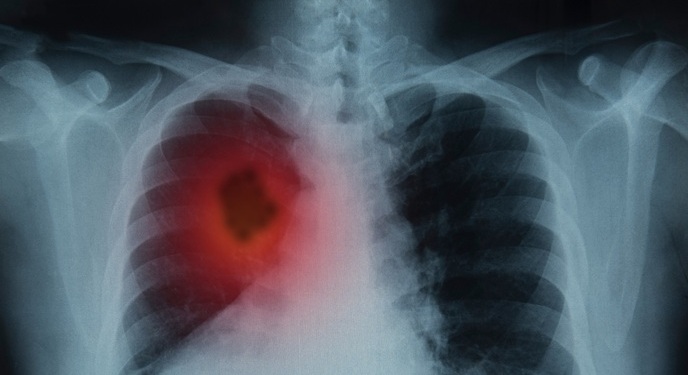
Higher Chest X-Ray Usage Catches Lung Cancer Earlier and Improves Survival
Lung cancer continues to be the leading cause of cancer-related deaths worldwide. While advanced technologies like CT scanners play a crucial role in detecting lung cancer, more accessible and affordable... Read moreMRI
view channel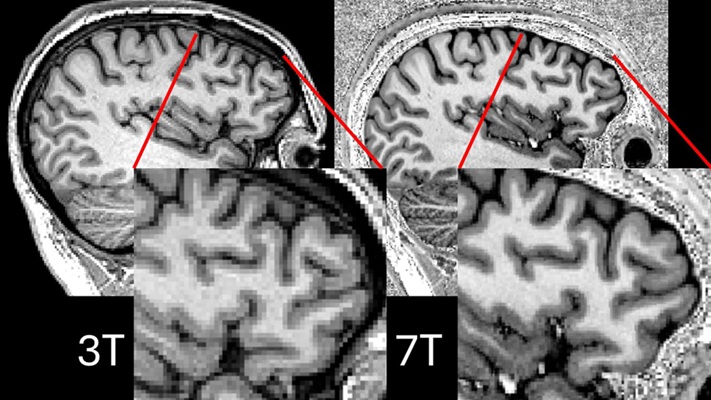
Ultra-Powerful MRI Scans Enable Life-Changing Surgery in Treatment-Resistant Epileptic Patients
Approximately 360,000 individuals in the UK suffer from focal epilepsy, a condition in which seizures spread from one part of the brain. Around a third of these patients experience persistent seizures... Read more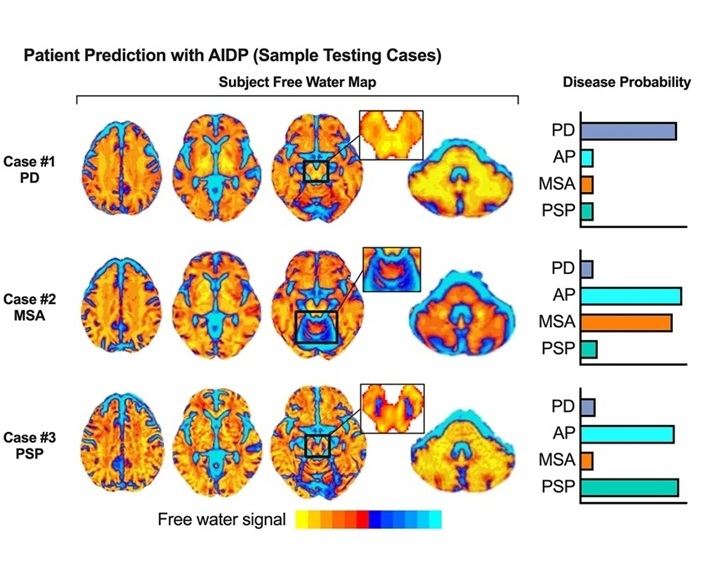
AI-Powered MRI Technology Improves Parkinson’s Diagnoses
Current research shows that the accuracy of diagnosing Parkinson’s disease typically ranges from 55% to 78% within the first five years of assessment. This is partly due to the similarities shared by Parkinson’s... Read more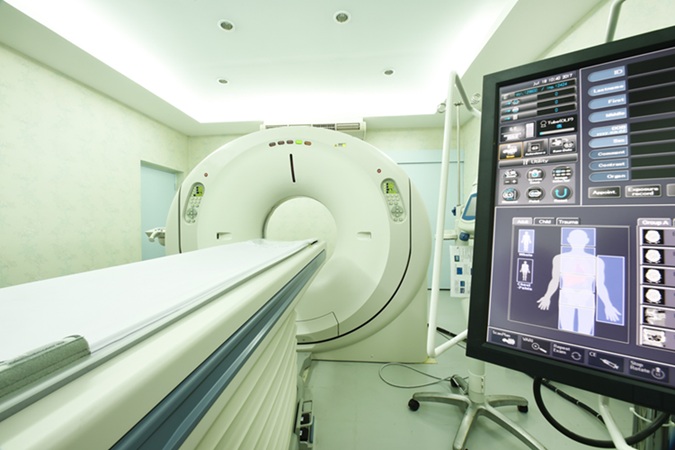
Biparametric MRI Combined with AI Enhances Detection of Clinically Significant Prostate Cancer
Artificial intelligence (AI) technologies are transforming the way medical images are analyzed, offering unprecedented capabilities in quantitatively extracting features that go beyond traditional visual... Read more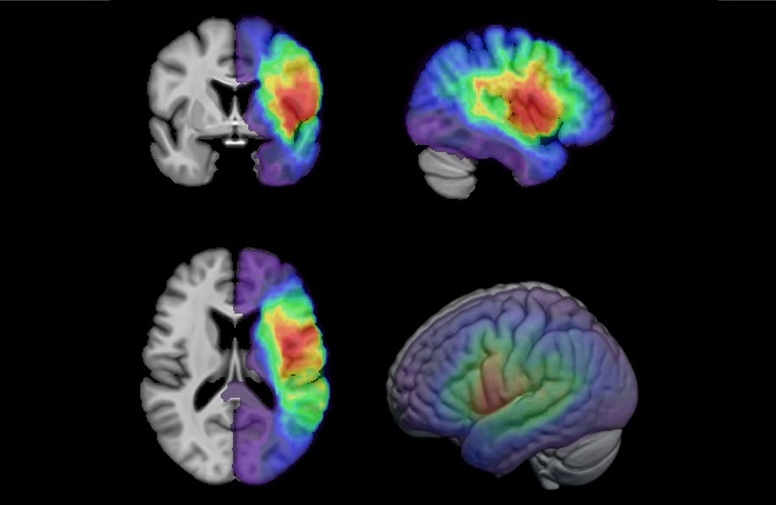
First-Of-Its-Kind AI-Driven Brain Imaging Platform to Better Guide Stroke Treatment Options
Each year, approximately 800,000 people in the U.S. experience strokes, with marginalized and minoritized groups being disproportionately affected. Strokes vary in terms of size and location within the... Read moreUltrasound
view channel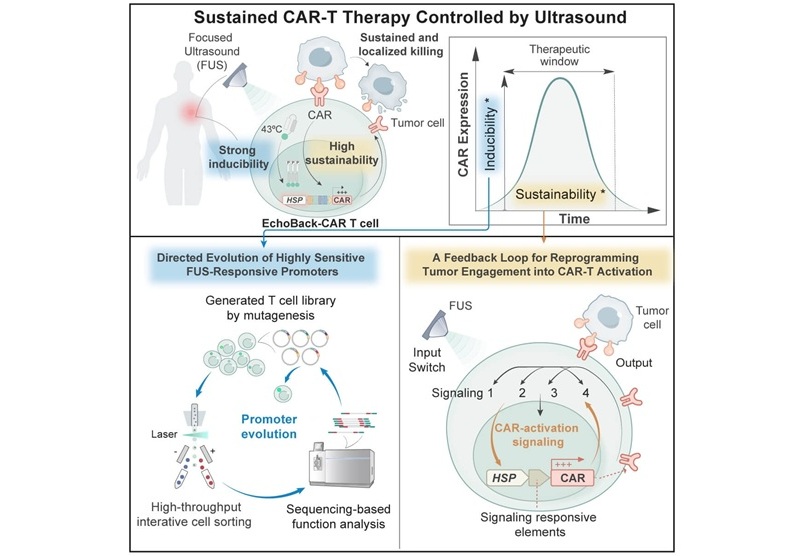
Smart Ultrasound-Activated Immune Cells Destroy Cancer Cells for Extended Periods
Chimeric antigen receptor (CAR) T-cell therapy has emerged as a highly promising cancer treatment, especially for bloodborne cancers like leukemia. This highly personalized therapy involves extracting... Read more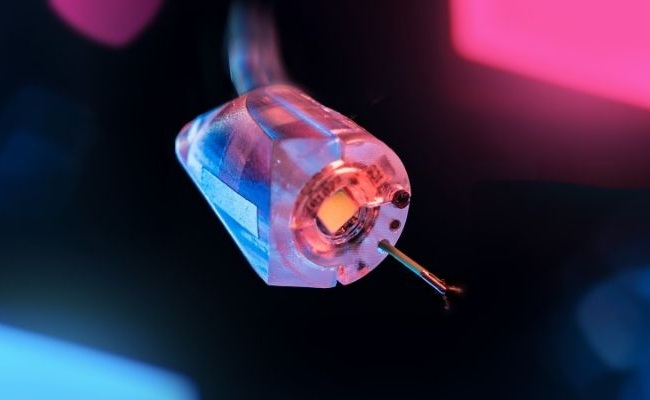
Tiny Magnetic Robot Takes 3D Scans from Deep Within Body
Colorectal cancer ranks as one of the leading causes of cancer-related mortality worldwide. However, when detected early, it is highly treatable. Now, a new minimally invasive technique could significantly... Read more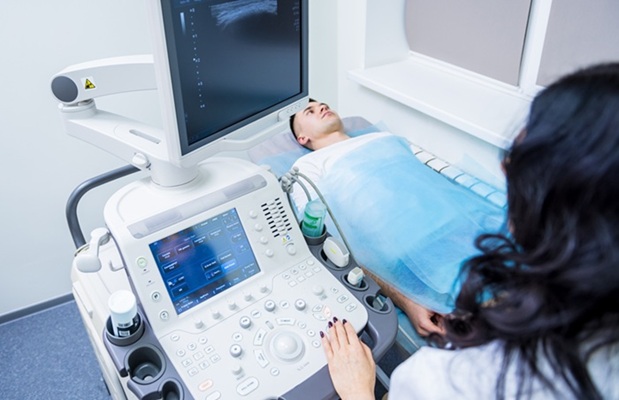
High Resolution Ultrasound Speeds Up Prostate Cancer Diagnosis
Each year, approximately one million prostate cancer biopsies are conducted across Europe, with similar numbers in the USA and around 100,000 in Canada. Most of these biopsies are performed using MRI images... Read more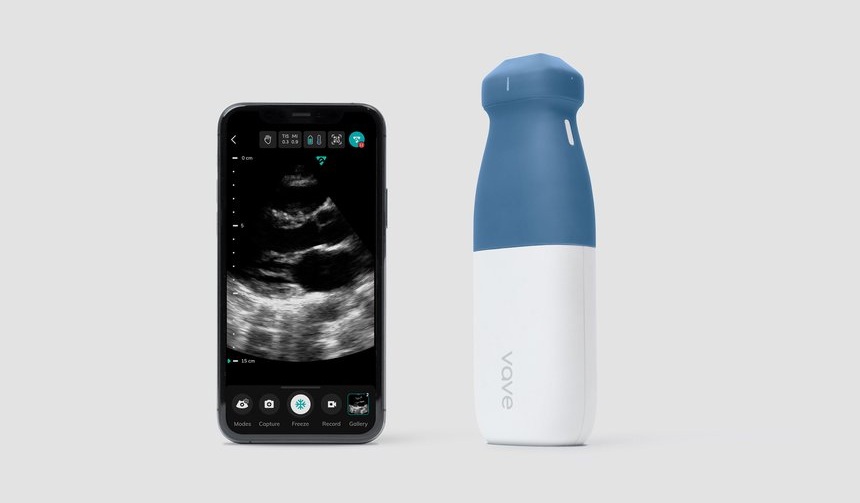
World's First Wireless, Handheld, Whole-Body Ultrasound with Single PZT Transducer Makes Imaging More Accessible
Ultrasound devices play a vital role in the medical field, routinely used to examine the body's internal tissues and structures. While advancements have steadily improved ultrasound image quality and processing... Read moreNuclear Medicine
view channel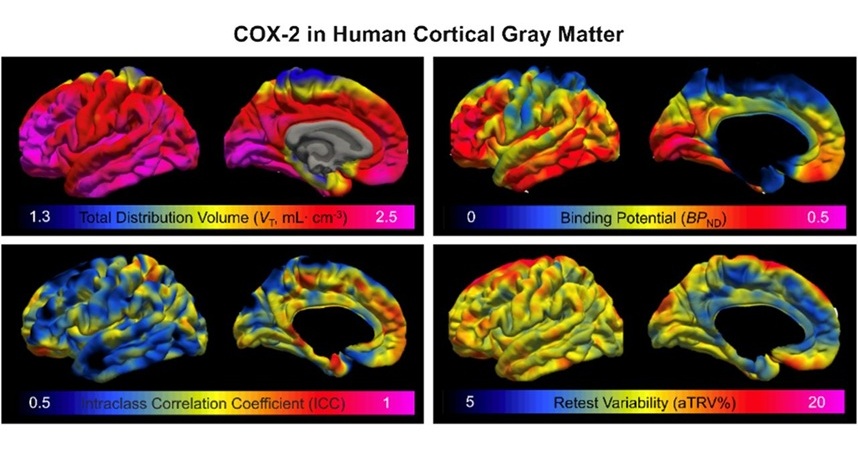
Novel PET Imaging Approach Offers Never-Before-Seen View of Neuroinflammation
COX-2, an enzyme that plays a key role in brain inflammation, can be significantly upregulated by inflammatory stimuli and neuroexcitation. Researchers suggest that COX-2 density in the brain could serve... Read more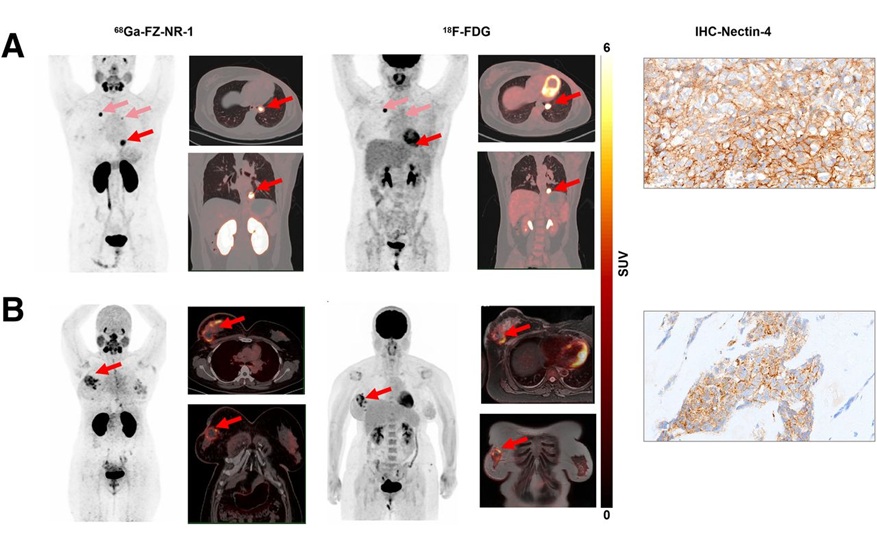
Novel Radiotracer Identifies Biomarker for Triple-Negative Breast Cancer
Triple-negative breast cancer (TNBC), which represents 15-20% of all breast cancer cases, is one of the most aggressive subtypes, with a five-year survival rate of about 40%. Due to its significant heterogeneity... Read moreImaging IT
view channel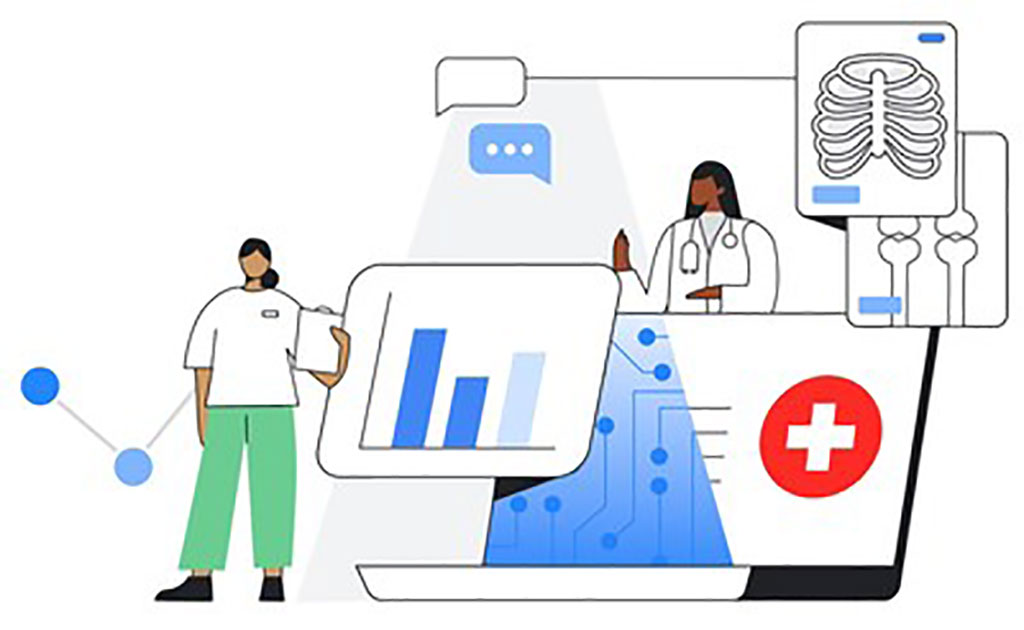
New Google Cloud Medical Imaging Suite Makes Imaging Healthcare Data More Accessible
Medical imaging is a critical tool used to diagnose patients, and there are billions of medical images scanned globally each year. Imaging data accounts for about 90% of all healthcare data1 and, until... Read more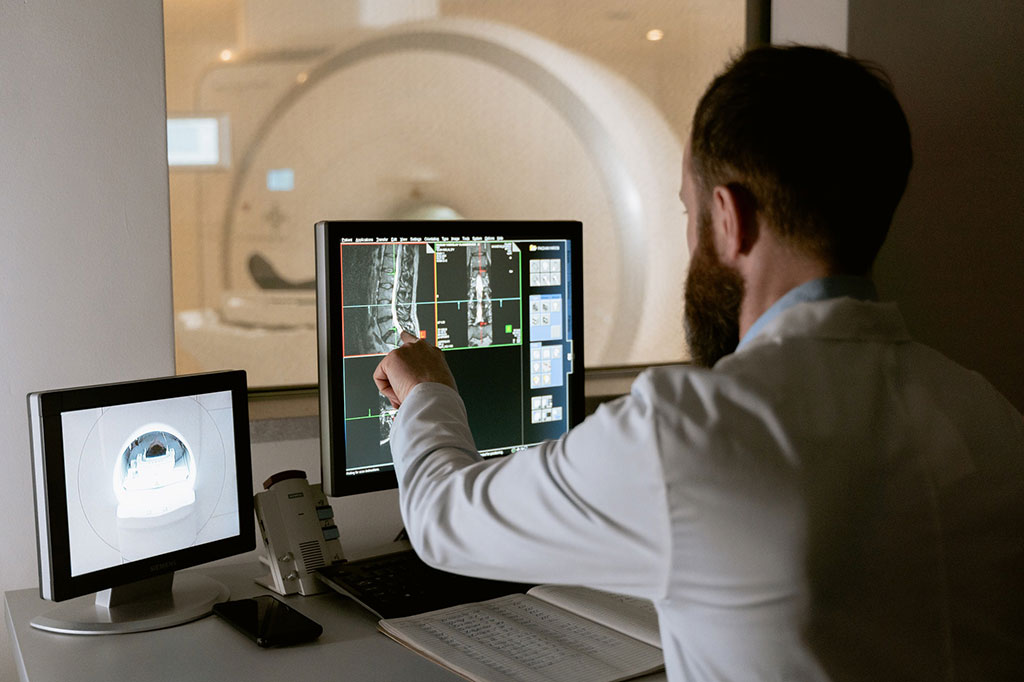
Global AI in Medical Diagnostics Market to Be Driven by Demand for Image Recognition in Radiology
The global artificial intelligence (AI) in medical diagnostics market is expanding with early disease detection being one of its key applications and image recognition becoming a compelling consumer proposition... Read moreIndustry News
view channel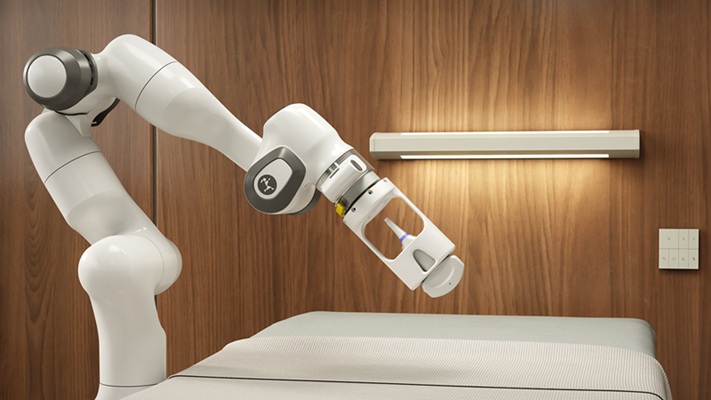
GE HealthCare and NVIDIA Collaboration to Reimagine Diagnostic Imaging
GE HealthCare (Chicago, IL, USA) has entered into a collaboration with NVIDIA (Santa Clara, CA, USA), expanding the existing relationship between the two companies to focus on pioneering innovation in... Read more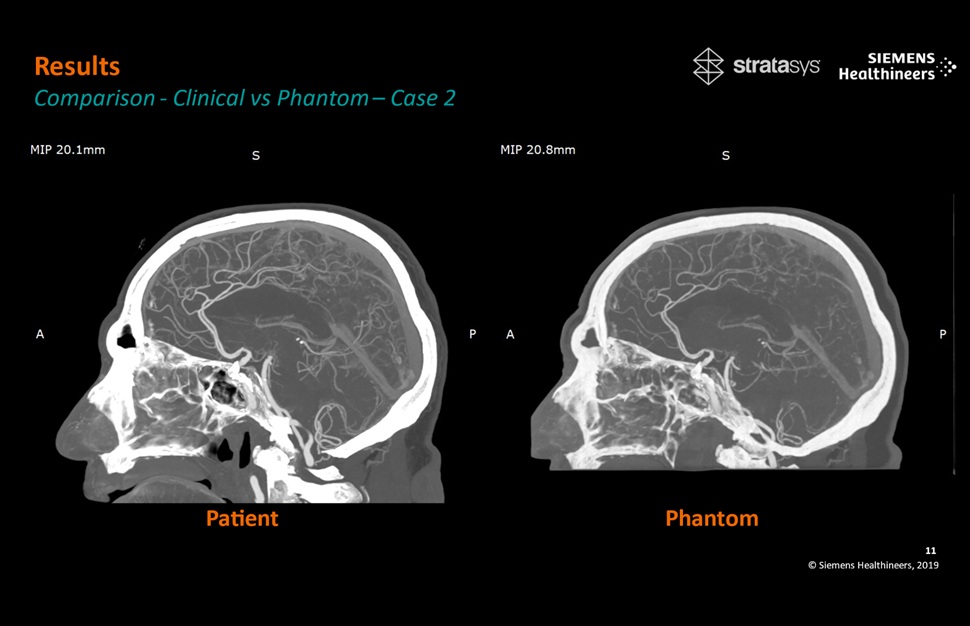
Patient-Specific 3D-Printed Phantoms Transform CT Imaging
New research has highlighted how anatomically precise, patient-specific 3D-printed phantoms are proving to be scalable, cost-effective, and efficient tools in the development of new CT scan algorithms... Read more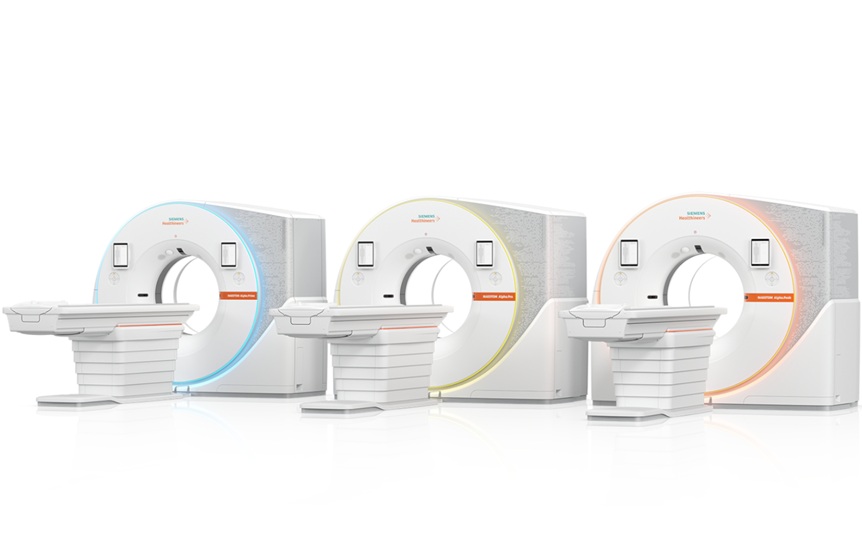
Siemens and Sectra Collaborate on Enhancing Radiology Workflows
Siemens Healthineers (Forchheim, Germany) and Sectra (Linköping, Sweden) have entered into a collaboration aimed at enhancing radiologists' diagnostic capabilities and, in turn, improving patient care... Read more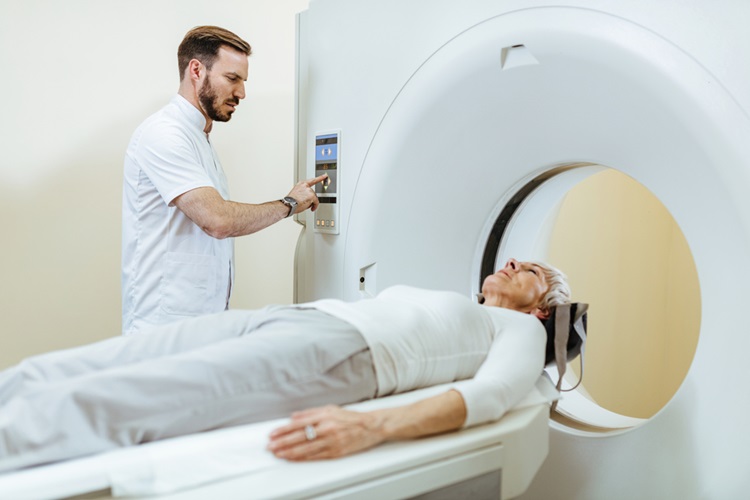