First-Ever Breast Cancer AI for Mammography Scans Shows How It Comes To Conclusions
By MedImaging International staff writers Posted on 27 Jan 2022 |
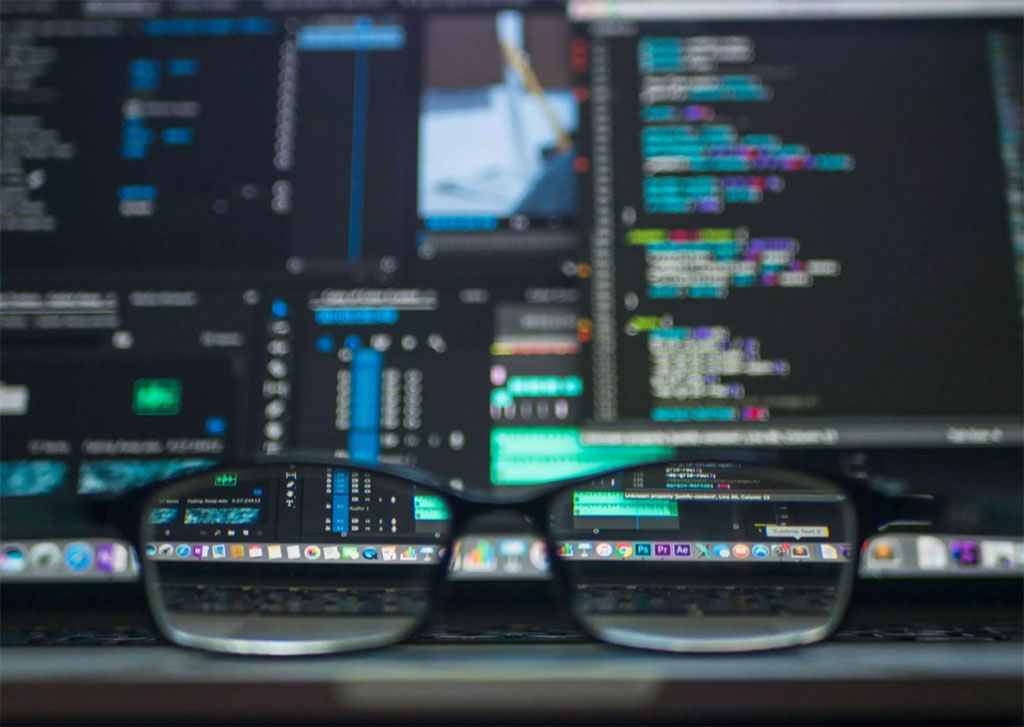
A new artificial intelligence (AI) tool for mammography scans aims to aid rather than replace human decision-making.
Computer engineers and radiologists at Duke University (Durham, NC, USA) have developed an AI platform to analyze potentially cancerous lesions in mammography scans to determine if a patient should receive an invasive biopsy. But unlike its many predecessors, this algorithm is interpretable, meaning it shows physicians exactly how it came to its conclusions.
The researchers trained the AI to locate and evaluate lesions just like an actual radiologist would be trained, rather than allowing it to freely develop its own procedures, giving it several advantages over its “black box” counterparts. It could make for a useful training platform to teach students how to read mammography images. It could also help physicians in sparsely populated regions around the world who do not regularly read mammography scans make better health care decisions.
The researchers trained the new AI with 1,136 images taken from 484 patients at Duke University Health System. They first taught the AI to find the suspicious lesions in question and ignore all of the healthy tissue and other irrelevant data. Then they hired radiologists to carefully label the images to teach the AI to focus on the edges of the lesions, where the potential tumors meet healthy surrounding tissue, and compare those edges to edges in images with known cancerous and benign outcomes. Radiating lines or fuzzy edges, known medically as mass margins, are the best predictor of cancerous breast tumors and the first thing that radiologists look for. This is because cancerous cells replicate and expand so fast that not all of a developing tumor’s edges are easy to see in mammograms.
After training was complete, the researches put the AI to the test. While it did not outperform human radiologists, it did just as well as other black box computer models. When the new AI is wrong, people working with it will be able to recognize that it is wrong and why it made the mistake. Moving forward, the team is working to add other physical characteristics for the AI to consider when making its decisions, such as a lesion’s shape, which is a second feature radiologists learn to look at.
“This is a unique way to train an AI how to look at medical imagery,” said Alina Barnett, a computer science PhD candidate at Duke and first author of the study. “Other AIs are not trying to imitate radiologists; they’re coming up with their own methods for answering the question that are often not helpful or, in some cases, depend on flawed reasoning processes.”
Related Links:
Duke University
Latest General/Advanced Imaging News
- Bone Density Test Uses Existing CT Images to Predict Fractures
- AI Predicts Cardiac Risk and Mortality from Routine Chest CT Scans
- Radiation Therapy Computed Tomography Solution Boosts Imaging Accuracy
- PET Scans Reveal Hidden Inflammation in Multiple Sclerosis Patients
- Artificial Intelligence Evaluates Cardiovascular Risk from CT Scans
- New AI Method Captures Uncertainty in Medical Images
- CT Coronary Angiography Reduces Need for Invasive Tests to Diagnose Coronary Artery Disease
- Novel Blood Test Could Reduce Need for PET Imaging of Patients with Alzheimer’s
- CT-Based Deep Learning Algorithm Accurately Differentiates Benign From Malignant Vertebral Fractures
- Minimally Invasive Procedure Could Help Patients Avoid Thyroid Surgery
- Self-Driving Mobile C-Arm Reduces Imaging Time during Surgery
- AR Application Turns Medical Scans Into Holograms for Assistance in Surgical Planning
- Imaging Technology Provides Ground-Breaking New Approach for Diagnosing and Treating Bowel Cancer
- CT Coronary Calcium Scoring Predicts Heart Attacks and Strokes
- AI Model Detects 90% of Lymphatic Cancer Cases from PET and CT Images
- Breakthrough Technology Revolutionizes Breast Imaging
Channels
Radiography
view channel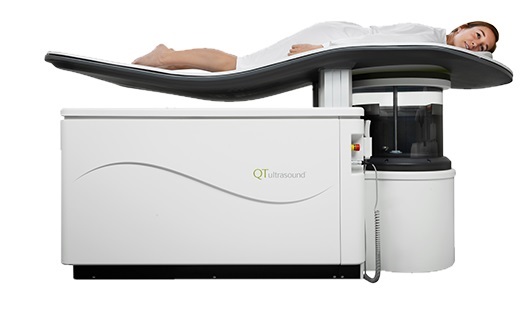
Novel Breast Imaging System Proves As Effective As Mammography
Breast cancer remains the most frequently diagnosed cancer among women. It is projected that one in eight women will be diagnosed with breast cancer during her lifetime, and one in 42 women who turn 50... Read more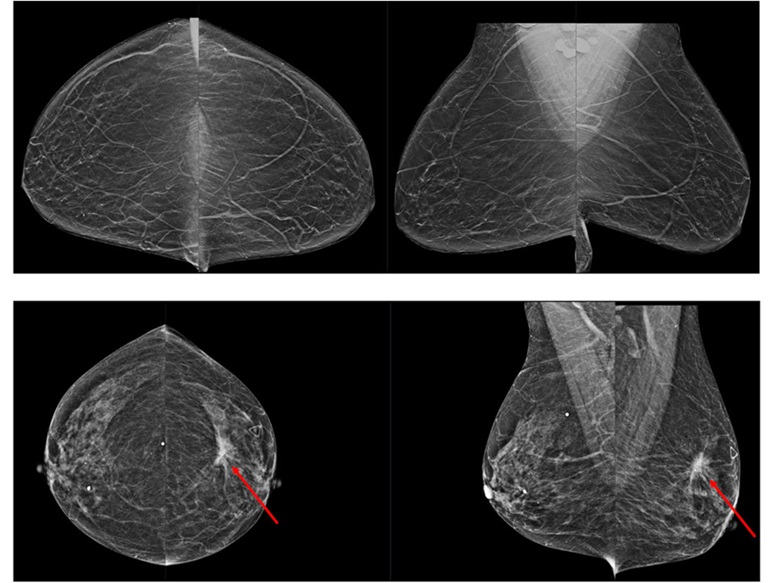
AI Assistance Improves Breast-Cancer Screening by Reducing False Positives
Radiologists typically detect one case of cancer for every 200 mammograms reviewed. However, these evaluations often result in false positives, leading to unnecessary patient recalls for additional testing,... Read moreMRI
view channel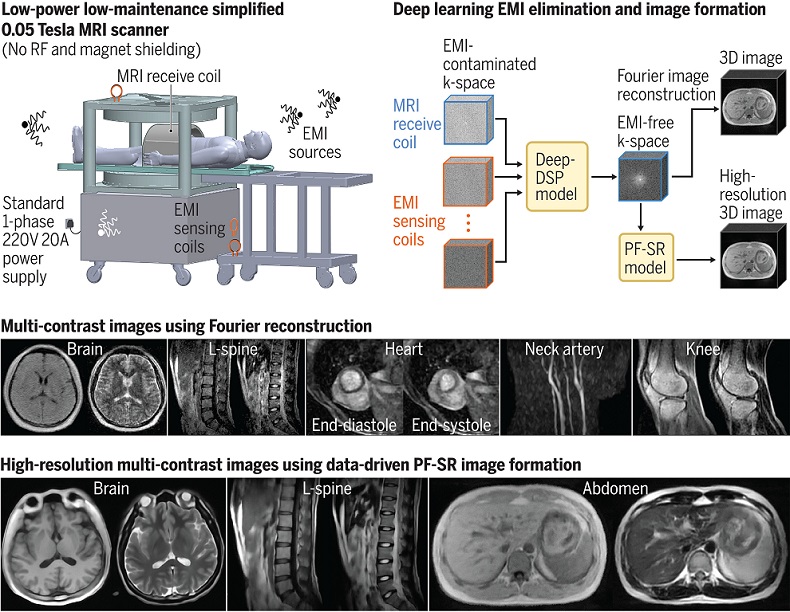
Low-Cost Whole-Body MRI Device Combined with AI Generates High-Quality Results
Magnetic Resonance Imaging (MRI) has significantly transformed healthcare, providing a noninvasive, radiation-free method for detailed imaging. It is especially promising for the future of medical diagnosis... Read more
World's First Whole-Body Ultra-High Field MRI Officially Comes To Market
The world's first whole-body ultra-high field (UHF) MRI has officially come to market, marking a remarkable advancement in diagnostic radiology. United Imaging (Shanghai, China) has secured clearance from the U.... Read moreUltrasound
view channel.jpg)
Diagnostic System Automatically Analyzes TTE Images to Identify Congenital Heart Disease
Congenital heart disease (CHD) is one of the most prevalent congenital anomalies worldwide, presenting substantial health and financial challenges for affected patients. Early detection and treatment of... Read more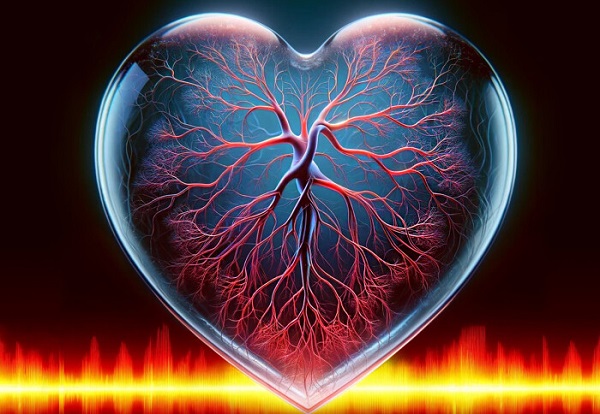
Super-Resolution Imaging Technique Could Improve Evaluation of Cardiac Conditions
The heart depends on efficient blood circulation to pump blood throughout the body, delivering oxygen to tissues and removing carbon dioxide and waste. Yet, when heart vessels are damaged, it can disrupt... Read more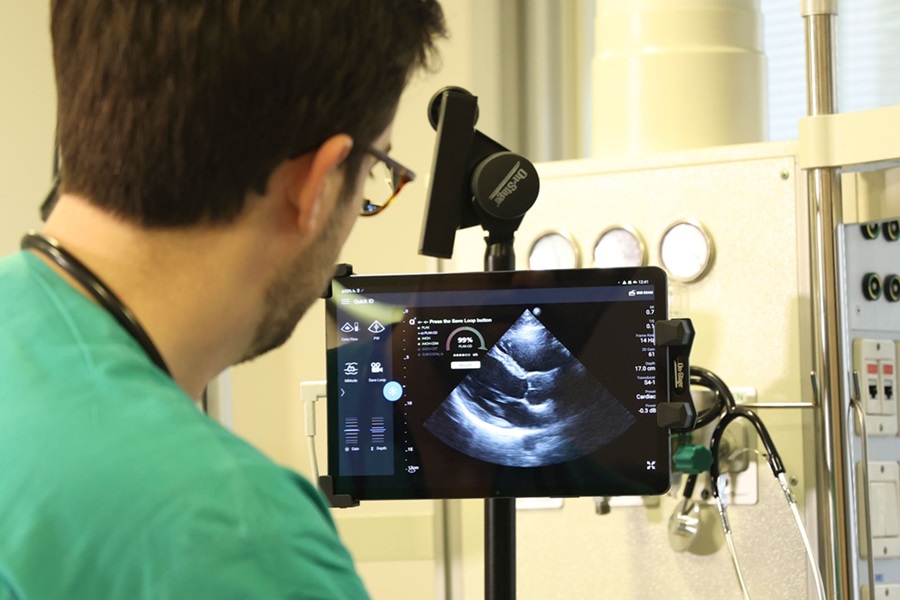
First AI-Powered POC Ultrasound Diagnostic Solution Helps Prioritize Cases Based On Severity
Ultrasound scans are essential for identifying and diagnosing various medical conditions, but often, patients must wait weeks or months for results due to a shortage of qualified medical professionals... Read moreNuclear Medicine
view channel![Image: Whole-body maximum-intensity projections over time after [68Ga]Ga-DPI-4452 administration (Photo courtesy of SNMMI) Image: Whole-body maximum-intensity projections over time after [68Ga]Ga-DPI-4452 administration (Photo courtesy of SNMMI)](https://globetechcdn.com/mobile_medicalimaging/images/stories/articles/article_images/2024-05-09/JNM May 2024 Hofman.jpg)
New PET Agent Rapidly and Accurately Visualizes Lesions in Clear Cell Renal Cell Carcinoma Patients
Clear cell renal cell cancer (ccRCC) represents 70-80% of renal cell carcinoma cases. While localized disease can be effectively treated with surgery and ablative therapies, one-third of patients either... Read more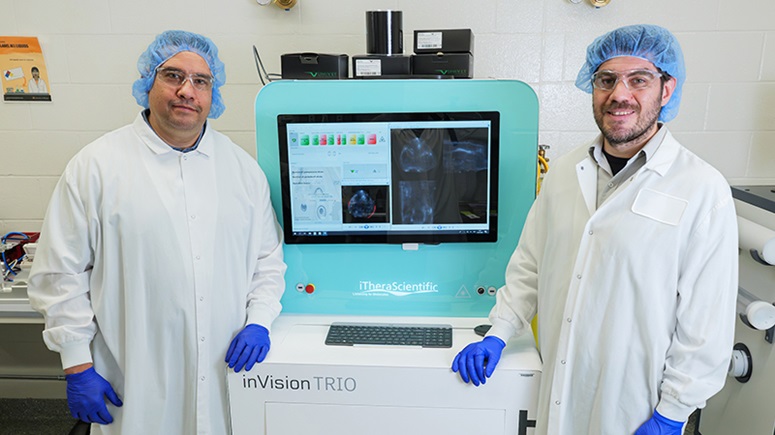
New Imaging Technique Monitors Inflammation Disorders without Radiation Exposure
Imaging inflammation using traditional radiological techniques presents significant challenges, including radiation exposure, poor image quality, high costs, and invasive procedures. Now, new contrast... Read more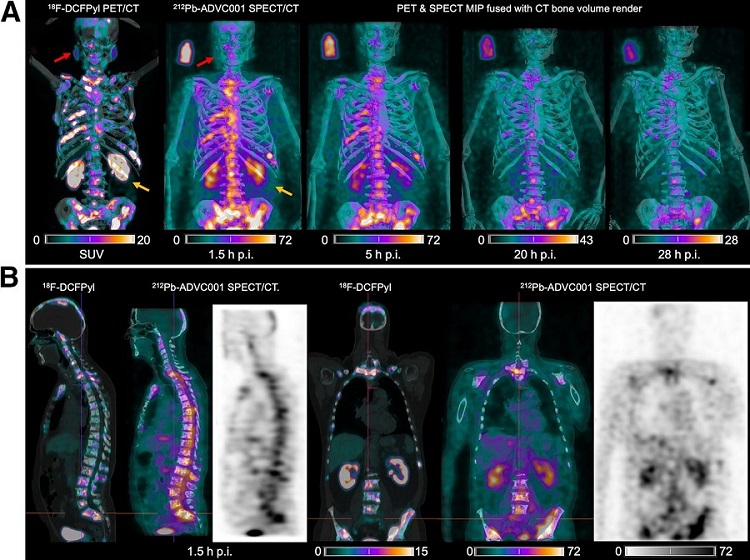
New SPECT/CT Technique Could Change Imaging Practices and Increase Patient Access
The development of lead-212 (212Pb)-PSMA–based targeted alpha therapy (TAT) is garnering significant interest in treating patients with metastatic castration-resistant prostate cancer. The imaging of 212Pb,... Read moreImaging IT
view channel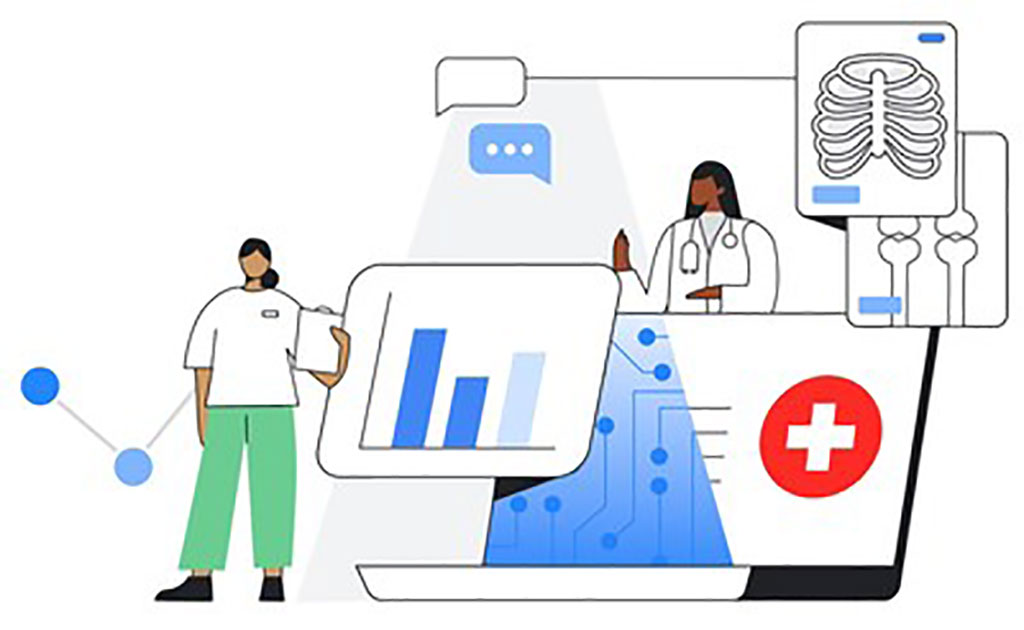
New Google Cloud Medical Imaging Suite Makes Imaging Healthcare Data More Accessible
Medical imaging is a critical tool used to diagnose patients, and there are billions of medical images scanned globally each year. Imaging data accounts for about 90% of all healthcare data1 and, until... Read more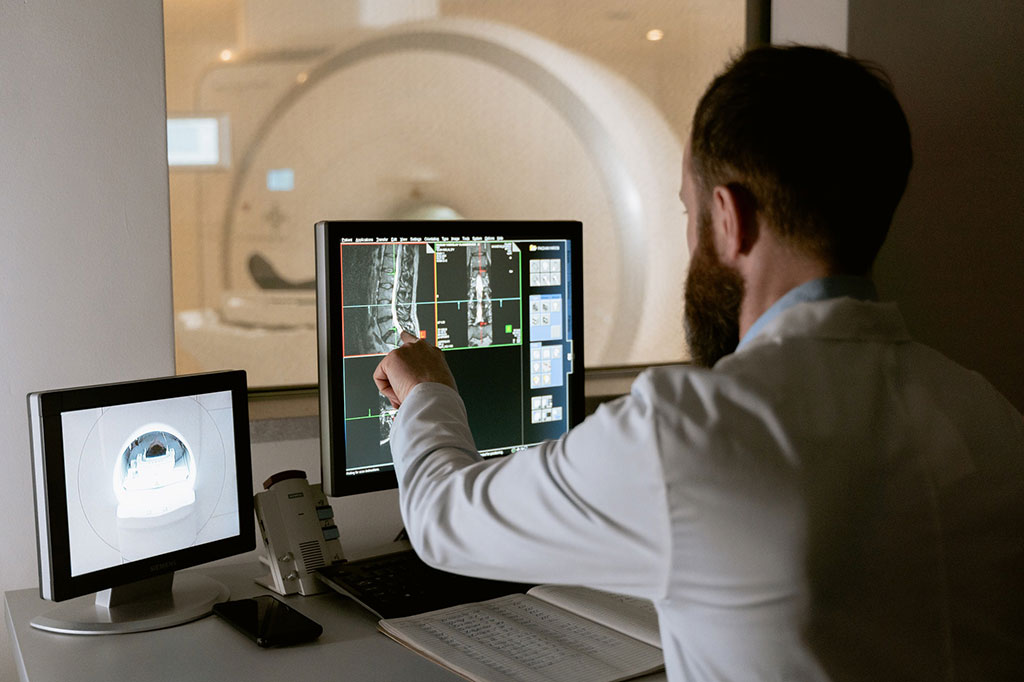
Global AI in Medical Diagnostics Market to Be Driven by Demand for Image Recognition in Radiology
The global artificial intelligence (AI) in medical diagnostics market is expanding with early disease detection being one of its key applications and image recognition becoming a compelling consumer proposition... Read moreIndustry News
view channel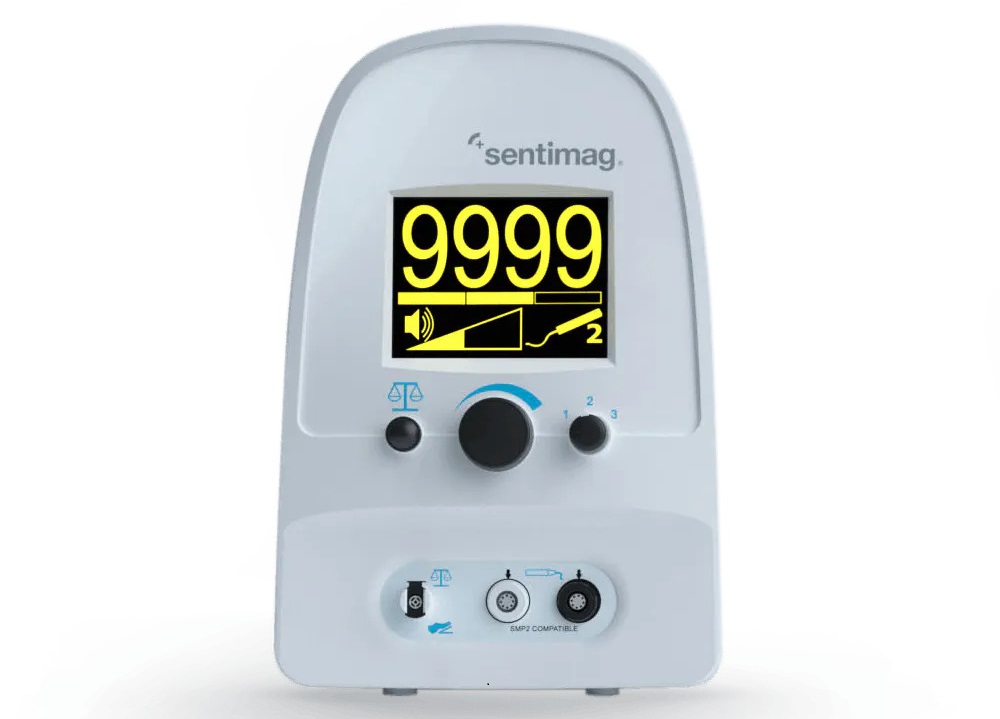
Hologic Acquires UK-Based Breast Surgical Guidance Company Endomagnetics Ltd.
Hologic, Inc. (Marlborough, MA, USA) has entered into a definitive agreement to acquire Endomagnetics Ltd. (Cambridge, UK), a privately held developer of breast cancer surgery technologies, for approximately... Read more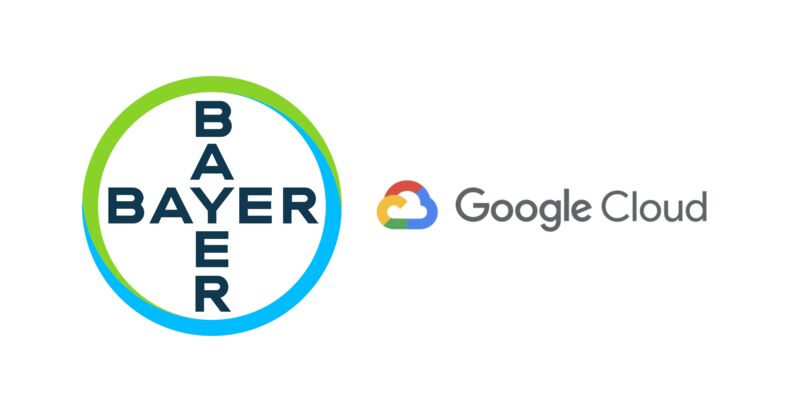
Bayer and Google Partner on New AI Product for Radiologists
Medical imaging data comprises around 90% of all healthcare data, and it is a highly complex and rich clinical data modality and serves as a vital tool for diagnosing patients. Each year, billions of medical... Read more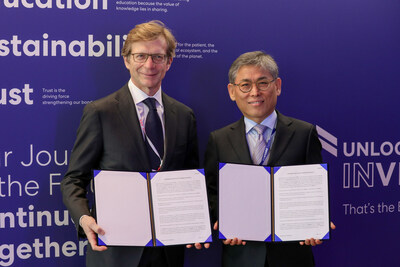