New AI Method Captures Uncertainty in Medical Images
Posted on 12 Apr 2024
In the field of biomedicine, segmentation is the process of annotating pixels from an important structure in medical images, such as organs or cells. Artificial Intelligence (AI) models are utilized to aid clinicians by highlighting pixels indicative of diseases or abnormalities. However, these AI models often provide a singular answer, despite the inherent ambiguity in medical image segmentation. For example, five different expert annotators might produce five varied segmentations of a lung nodule in a CT scan, each differing in the existence or extent of the borders of a nodule in a lung CT image. Recognizing these discrepancies and the inherent uncertainties can significantly influence clinical decision-making.
Now, researchers at MIT (Cambridge, MA, USA) have developed a new AI tool, named Tyche, which is designed to capture the uncertainty in a medical image. Tyche generates multiple plausible segmentations highlighting slightly different areas of a medical image. Users can set the number of output options Tyche provides and choose the most suitable one for their specific needs. One of the key advantages of Tyche is that it does not require retraining for each new segmentation task, making it more user-friendly for clinicians and biomedical researchers than some other AI methods. This tool can be used straight out of the box for various applications, ranging from detecting lesions in lung X-rays to identifying anomalies in brain MRIs, potentially enhancing diagnostic processes or supporting biomedical research by highlighting critical details that might be overlooked by other tools.
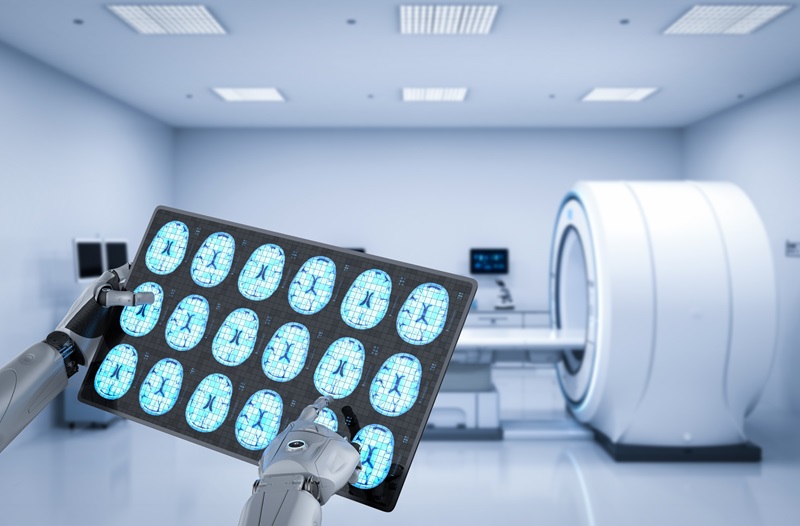
In tests using datasets of annotated medical images, Tyche demonstrated its ability to capture the diversity of human annotators effectively. Its best predictions were found to surpass those of baseline models, and it also operated faster. Tyche even outperformed more complex models trained with extensive, specialized datasets. Looking forward, the researchers intend to explore the use of more adaptable context sets, which could include textual descriptions or multiple image types. They are also interested in improving the accuracy of Tyche’s less accurate predictions and refining the system to better recommend the most reliable segmentation options.
“Ambiguity has been understudied. If your model completely misses a nodule that three experts say is there and two experts say is not, that is probably something you should pay attention to,” said senior author Adrian Dalca.
“If you want to take ambiguity into account, you often have to use an extremely complicated model. With the method we propose, our goal is to make it easy to use with a relatively small model so that it can make predictions quickly,” added Marianne Rakic, an MIT computer science Ph.D. candidate.
Related Links:
MIT