COVID-19 Findings Presented at RSNA 2020 Suggest AI Can Boost CT's Performance in Predicting Disease Severity
By MedImaging International staff writers Posted on 01 Dec 2020 |
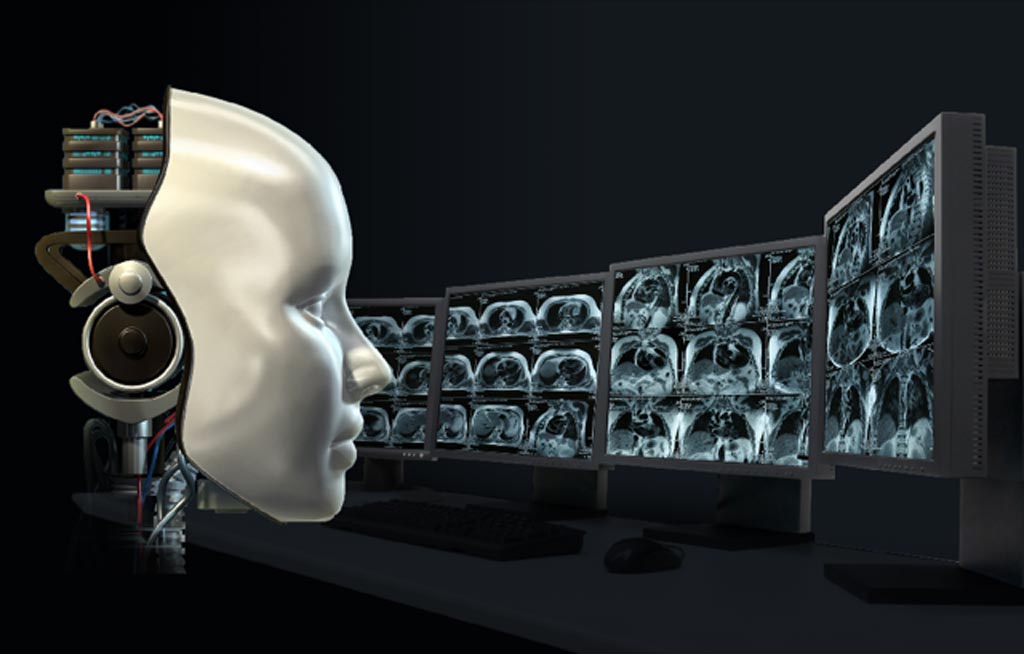
Illustration
Chest computed tomography (CT) when combined with artificial intelligence (AI) can become a valuable tool for diagnosing COVID-19, according to presentations on chest imaging made at a scientific session at the RSNA 2020.
In the first presentation, a team of scientists shared results from a study conducted by Nvidia (Santa Clara, CA, USA) that combined a deep-learning algorithm with chest CT to predict if COVID-19 patients needed to be admitted to the intensive care unit (ICU). The team analyzed 632 chest CT scans of COVID-19 patients confirmed by RT-PCR testing, out of which 69 patients were admitted to the ICU. The scientists developed a whole-lung segmentation algorithm and evaluated its effectiveness in terms of overall accuracy, sensitivity, and specificity when used along with CT. They found that the algorithm demonstrated high accuracy, specificity, and negative predictive value (NPV) in the identification of COVID-19 and predicting ICU admission by using chest CT. These findings indicate that AI can significantly improve the performance of CT in predicting COVID-19 severity.
"This deep-learning algorithm can alert the clinician to the enhanced potential of ICU admission, when combined with other clinical features," said Ziyue Xu, PhD, senior scientist at Nvidia. "Based upon chest CT alone, AI-based deep-learning algorithms can reasonably predict clinical outcomes such as ICU admission in patients with COVID-19 who underwent CT and PCR on the day of admission. The model is feasible with reasonable accuracy and specificity of prediction."
In another presentation, a team of researchers from the University of Pennsylvania (Philadelphia, PA, USA) highlighted their new approach for quantifying the percentage of lung volume involved in airspace disease on chest X-rays by using a convolutional neural network (CNN) algorithm based on 1,000 chest CT scans of COVID-19 patients. The study involved 86 patients with positive RT-PCR results who had chest CT and chest X-ray performed less than 48 hours apart. The algorithm used quantitative maps of lung tissue thickness and manifestations of airspace disease to project the CT exams' 3D lung and airspace disease segmentation on reconstructed X-rays. The researchers found that the CNN-reconstructed X-rays were as good as the human CT exam readers in quantifying airspace disease with CT recording a rate of 24.3% as against a rate of 24.4% by the CNN's digitally reconstructed X-rays.
"This approach may increase efficiency and consistency in chest x-ray interpretation of COVID-19 patients, especially when applied to longitudinal chest x-ray data to inform management," said Dr. Eduardo Jose Mortani Barbosa of the University of Pennsylvania.
Related Links:
Nvidia
University of Pennsylvania
In the first presentation, a team of scientists shared results from a study conducted by Nvidia (Santa Clara, CA, USA) that combined a deep-learning algorithm with chest CT to predict if COVID-19 patients needed to be admitted to the intensive care unit (ICU). The team analyzed 632 chest CT scans of COVID-19 patients confirmed by RT-PCR testing, out of which 69 patients were admitted to the ICU. The scientists developed a whole-lung segmentation algorithm and evaluated its effectiveness in terms of overall accuracy, sensitivity, and specificity when used along with CT. They found that the algorithm demonstrated high accuracy, specificity, and negative predictive value (NPV) in the identification of COVID-19 and predicting ICU admission by using chest CT. These findings indicate that AI can significantly improve the performance of CT in predicting COVID-19 severity.
"This deep-learning algorithm can alert the clinician to the enhanced potential of ICU admission, when combined with other clinical features," said Ziyue Xu, PhD, senior scientist at Nvidia. "Based upon chest CT alone, AI-based deep-learning algorithms can reasonably predict clinical outcomes such as ICU admission in patients with COVID-19 who underwent CT and PCR on the day of admission. The model is feasible with reasonable accuracy and specificity of prediction."
In another presentation, a team of researchers from the University of Pennsylvania (Philadelphia, PA, USA) highlighted their new approach for quantifying the percentage of lung volume involved in airspace disease on chest X-rays by using a convolutional neural network (CNN) algorithm based on 1,000 chest CT scans of COVID-19 patients. The study involved 86 patients with positive RT-PCR results who had chest CT and chest X-ray performed less than 48 hours apart. The algorithm used quantitative maps of lung tissue thickness and manifestations of airspace disease to project the CT exams' 3D lung and airspace disease segmentation on reconstructed X-rays. The researchers found that the CNN-reconstructed X-rays were as good as the human CT exam readers in quantifying airspace disease with CT recording a rate of 24.3% as against a rate of 24.4% by the CNN's digitally reconstructed X-rays.
"This approach may increase efficiency and consistency in chest x-ray interpretation of COVID-19 patients, especially when applied to longitudinal chest x-ray data to inform management," said Dr. Eduardo Jose Mortani Barbosa of the University of Pennsylvania.
Related Links:
Nvidia
University of Pennsylvania
Latest General/Advanced Imaging News
- Bone Density Test Uses Existing CT Images to Predict Fractures
- AI Predicts Cardiac Risk and Mortality from Routine Chest CT Scans
- Radiation Therapy Computed Tomography Solution Boosts Imaging Accuracy
- PET Scans Reveal Hidden Inflammation in Multiple Sclerosis Patients
- Artificial Intelligence Evaluates Cardiovascular Risk from CT Scans
- New AI Method Captures Uncertainty in Medical Images
- CT Coronary Angiography Reduces Need for Invasive Tests to Diagnose Coronary Artery Disease
- Novel Blood Test Could Reduce Need for PET Imaging of Patients with Alzheimer’s
- CT-Based Deep Learning Algorithm Accurately Differentiates Benign From Malignant Vertebral Fractures
- Minimally Invasive Procedure Could Help Patients Avoid Thyroid Surgery
- Self-Driving Mobile C-Arm Reduces Imaging Time during Surgery
- AR Application Turns Medical Scans Into Holograms for Assistance in Surgical Planning
- Imaging Technology Provides Ground-Breaking New Approach for Diagnosing and Treating Bowel Cancer
- CT Coronary Calcium Scoring Predicts Heart Attacks and Strokes
- AI Model Detects 90% of Lymphatic Cancer Cases from PET and CT Images
- Breakthrough Technology Revolutionizes Breast Imaging
Channels
Radiography
view channel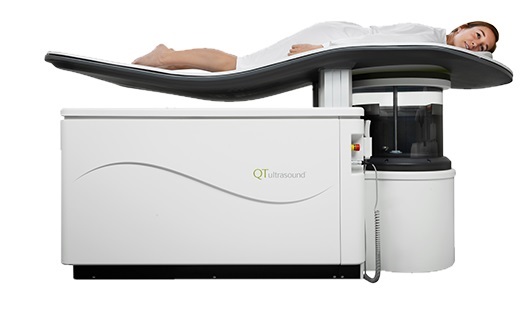
Novel Breast Imaging System Proves As Effective As Mammography
Breast cancer remains the most frequently diagnosed cancer among women. It is projected that one in eight women will be diagnosed with breast cancer during her lifetime, and one in 42 women who turn 50... Read more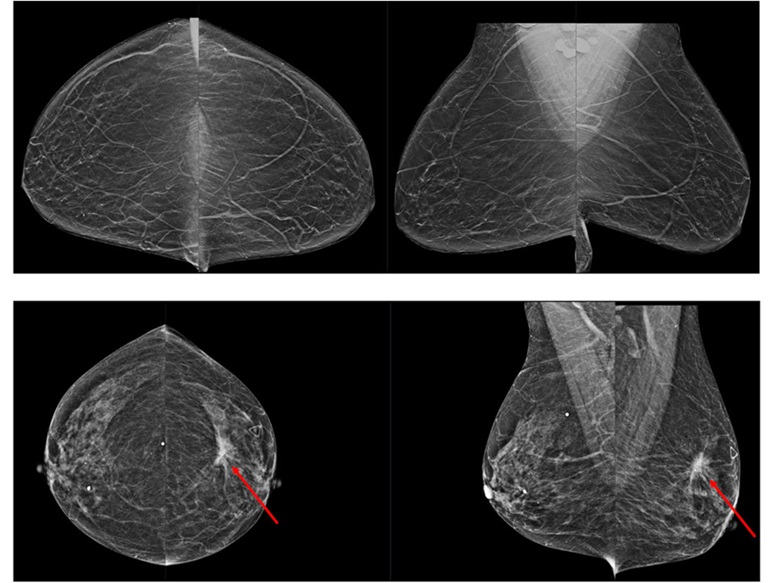
AI Assistance Improves Breast-Cancer Screening by Reducing False Positives
Radiologists typically detect one case of cancer for every 200 mammograms reviewed. However, these evaluations often result in false positives, leading to unnecessary patient recalls for additional testing,... Read moreMRI
view channel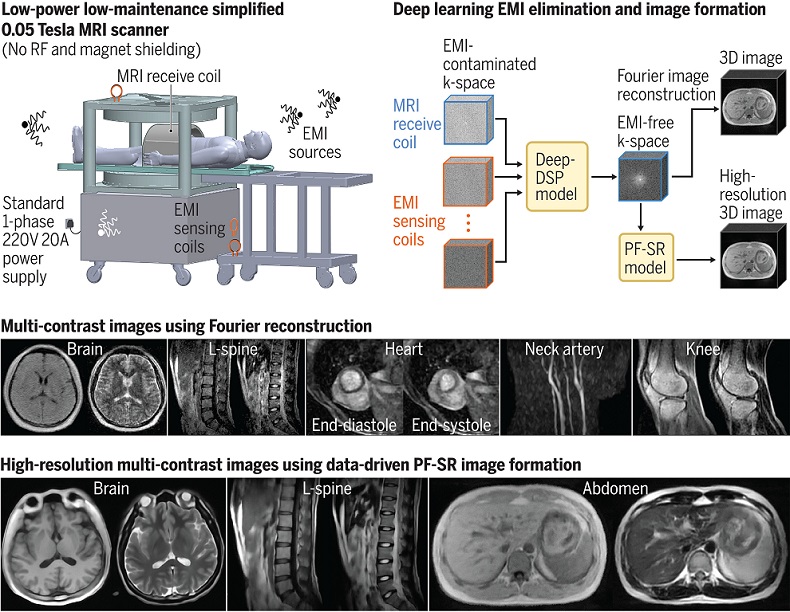
Low-Cost Whole-Body MRI Device Combined with AI Generates High-Quality Results
Magnetic Resonance Imaging (MRI) has significantly transformed healthcare, providing a noninvasive, radiation-free method for detailed imaging. It is especially promising for the future of medical diagnosis... Read more
World's First Whole-Body Ultra-High Field MRI Officially Comes To Market
The world's first whole-body ultra-high field (UHF) MRI has officially come to market, marking a remarkable advancement in diagnostic radiology. United Imaging (Shanghai, China) has secured clearance from the U.... Read moreUltrasound
view channel.jpg)
Diagnostic System Automatically Analyzes TTE Images to Identify Congenital Heart Disease
Congenital heart disease (CHD) is one of the most prevalent congenital anomalies worldwide, presenting substantial health and financial challenges for affected patients. Early detection and treatment of... Read more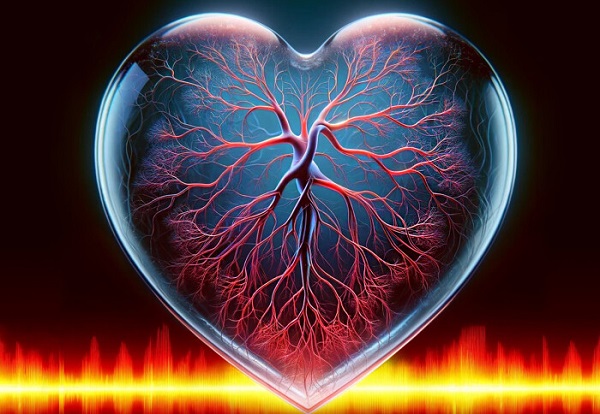
Super-Resolution Imaging Technique Could Improve Evaluation of Cardiac Conditions
The heart depends on efficient blood circulation to pump blood throughout the body, delivering oxygen to tissues and removing carbon dioxide and waste. Yet, when heart vessels are damaged, it can disrupt... Read more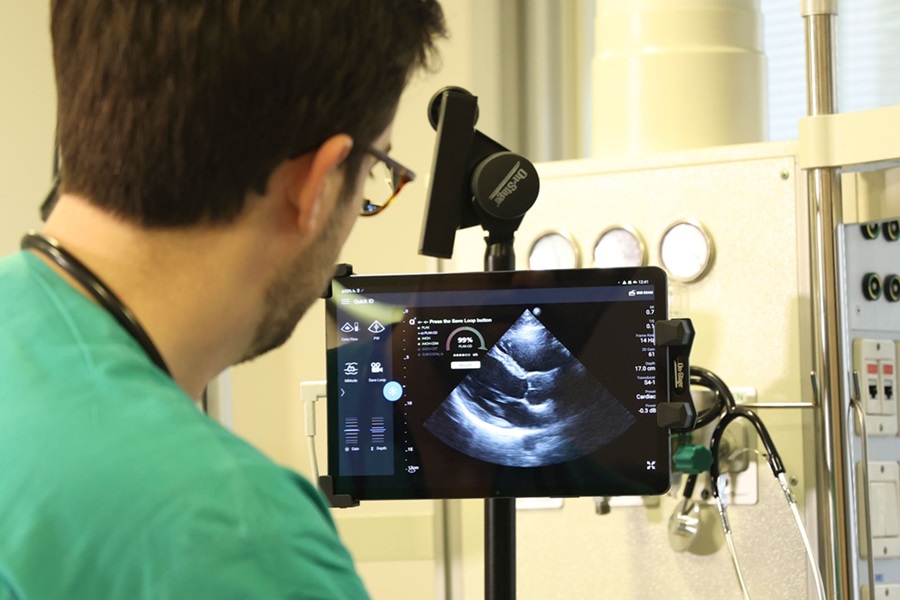
First AI-Powered POC Ultrasound Diagnostic Solution Helps Prioritize Cases Based On Severity
Ultrasound scans are essential for identifying and diagnosing various medical conditions, but often, patients must wait weeks or months for results due to a shortage of qualified medical professionals... Read moreNuclear Medicine
view channel![Image: Whole-body maximum-intensity projections over time after [68Ga]Ga-DPI-4452 administration (Photo courtesy of SNMMI) Image: Whole-body maximum-intensity projections over time after [68Ga]Ga-DPI-4452 administration (Photo courtesy of SNMMI)](https://globetechcdn.com/mobile_medicalimaging/images/stories/articles/article_images/2024-05-09/JNM May 2024 Hofman.jpg)
New PET Agent Rapidly and Accurately Visualizes Lesions in Clear Cell Renal Cell Carcinoma Patients
Clear cell renal cell cancer (ccRCC) represents 70-80% of renal cell carcinoma cases. While localized disease can be effectively treated with surgery and ablative therapies, one-third of patients either... Read more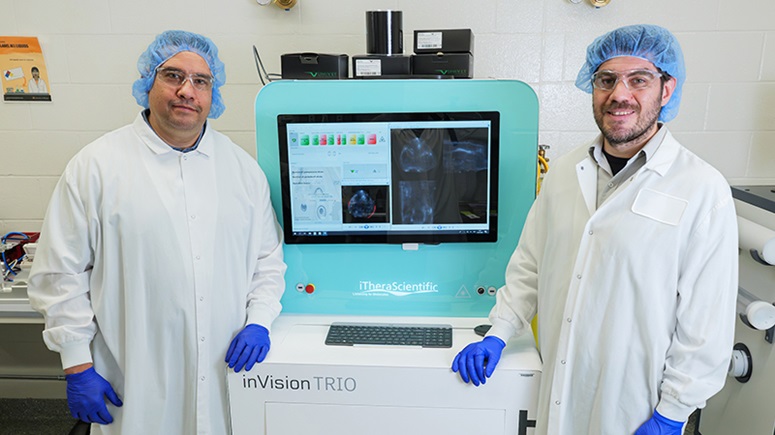
New Imaging Technique Monitors Inflammation Disorders without Radiation Exposure
Imaging inflammation using traditional radiological techniques presents significant challenges, including radiation exposure, poor image quality, high costs, and invasive procedures. Now, new contrast... Read more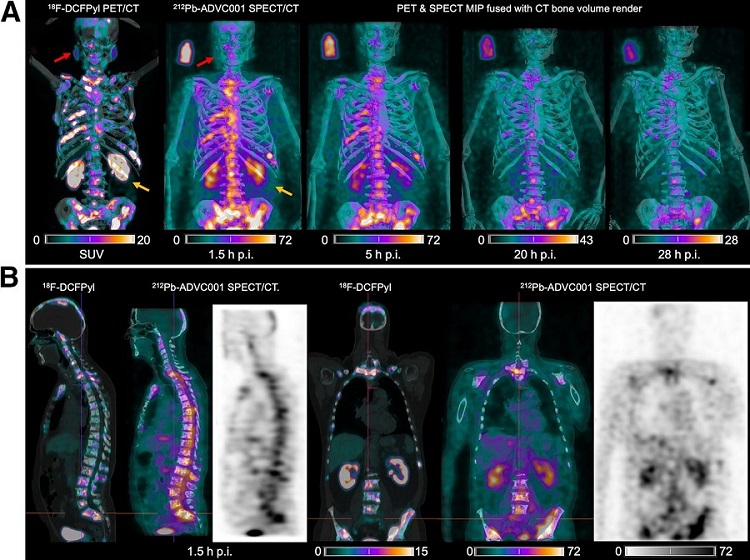
New SPECT/CT Technique Could Change Imaging Practices and Increase Patient Access
The development of lead-212 (212Pb)-PSMA–based targeted alpha therapy (TAT) is garnering significant interest in treating patients with metastatic castration-resistant prostate cancer. The imaging of 212Pb,... Read moreGeneral/Advanced Imaging
view channel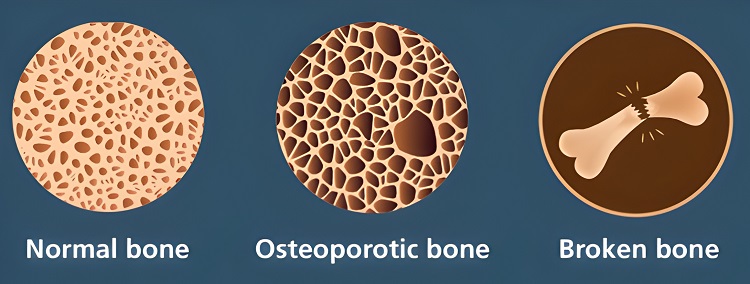
Bone Density Test Uses Existing CT Images to Predict Fractures
Osteoporotic fractures are not only devastating and deadly, especially hip fractures, but also impose significant costs. They rank among the top chronic diseases in terms of disability-adjusted life years... Read more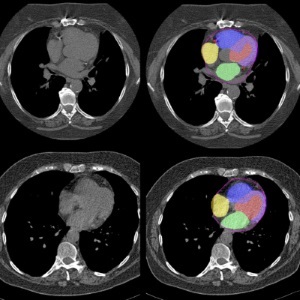
AI Predicts Cardiac Risk and Mortality from Routine Chest CT Scans
Heart disease remains the leading cause of death and is largely preventable, yet many individuals are unaware of their risk until it becomes severe. Early detection through screening can reveal heart issues,... Read moreImaging IT
view channel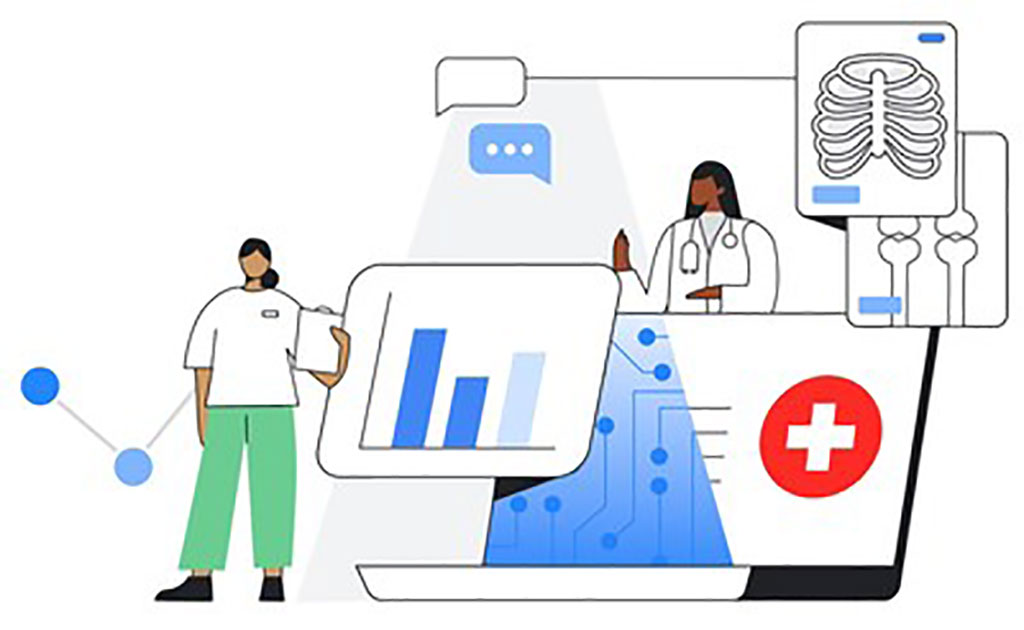
New Google Cloud Medical Imaging Suite Makes Imaging Healthcare Data More Accessible
Medical imaging is a critical tool used to diagnose patients, and there are billions of medical images scanned globally each year. Imaging data accounts for about 90% of all healthcare data1 and, until... Read more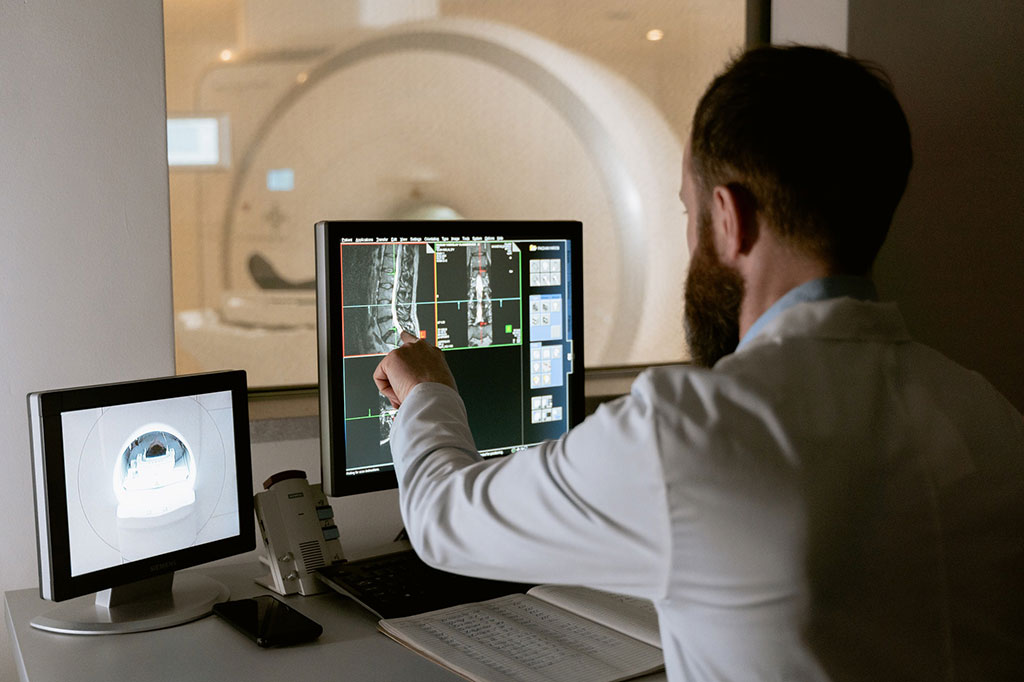
Global AI in Medical Diagnostics Market to Be Driven by Demand for Image Recognition in Radiology
The global artificial intelligence (AI) in medical diagnostics market is expanding with early disease detection being one of its key applications and image recognition becoming a compelling consumer proposition... Read moreIndustry News
view channel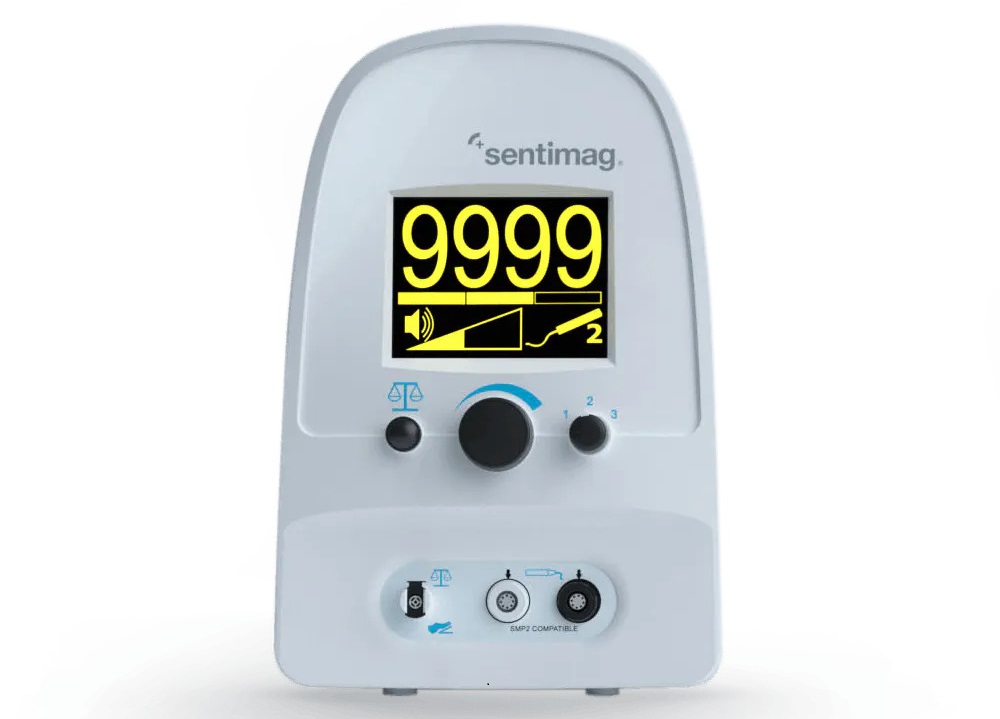
Hologic Acquires UK-Based Breast Surgical Guidance Company Endomagnetics Ltd.
Hologic, Inc. (Marlborough, MA, USA) has entered into a definitive agreement to acquire Endomagnetics Ltd. (Cambridge, UK), a privately held developer of breast cancer surgery technologies, for approximately... Read more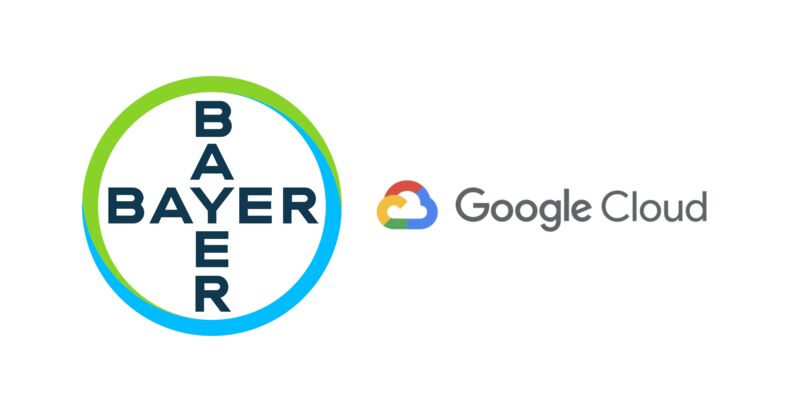
Bayer and Google Partner on New AI Product for Radiologists
Medical imaging data comprises around 90% of all healthcare data, and it is a highly complex and rich clinical data modality and serves as a vital tool for diagnosing patients. Each year, billions of medical... Read more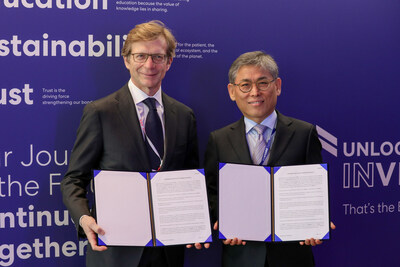