Cardiac CT Algorithm Quantifies Aortic Valve Calcium
By MedImaging International staff writers Posted on 22 Feb 2021 |
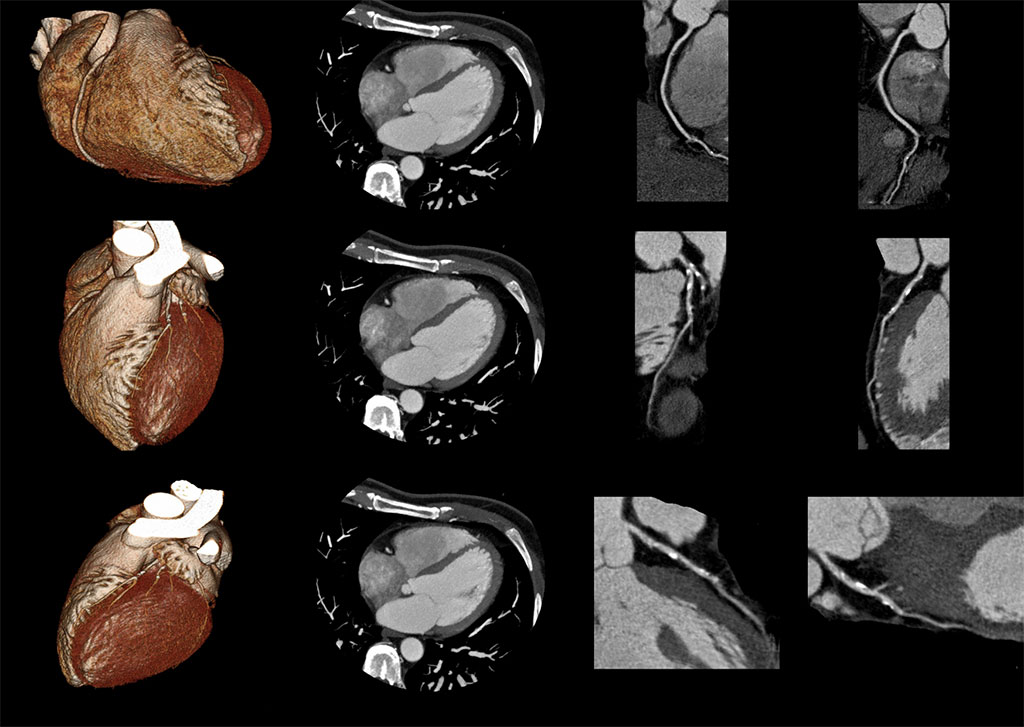
Image: Cardiac CT AI can detect calcium buildup on the aortic valve (Photo courtesy of Getty Images)
A new study shows that an artificial intelligence (AI) model can automatically detect aortic valve calcium (AVC) on cardiac CT, and is superior to visual grading by radiologists.
Developed by researchers at the Catholic University of Korea (Seoul, South Korea), Yonsei University College of Medicine (Seoul, South Korea), and other institutions, the deep learning (DL)-based algorithm was initially trained and validated on 452 non-enhanced electrocardiogram-gated cardiac CT scans. It was then tested on a separate set of 137 cases, with each CT exam manually annotated by a radiologist with seven years of experience in cardiothoracic imaging, and AVC volume and Agatston scores were compared.
The results revealed that when manually measured AVC Agatston score was used as a benchmark, the accuracy of DL-measured AVC Agatston score for AVC volume grading was 97%, which was better than that of the four radiologist readers (77.8–89.9 %). The accuracy of DL algorithm for Agatston score was 92.9%. Overall, the DL model was deemed to be superior to all four radiologists for predicting severe aortic valve calcium cases. The study was published on February 6, 2021, in European Journal of Radiology.
“For observer performance testing, four radiologists determined AVC grade in two reading rounds. The diagnostic performance of DL-measured AVC volume and Agaston score for classifying severe AVC was compared with that of each reader's assessment,” explained lead author Suyon Chang, MD, of the Catholic University of Korea, and colleagues. “To validate AVC segmentation performance, the Dice coefficient [a statistic used to gauge the similarity of two samples] was calculated; after applying the DL algorithm, the Dice coefficient score was 0.807.”
The Agatston score is a semi-automated tool to calculate the extent of coronary artery calcification detected by an unenhanced low-dose CT scan, which is routinely performed in patients undergoing cardiac CT. It allows for early risk stratification as patients with a high Agatston score (over 160) have an increased risk for a major adverse cardiac event (MACE). Although it does not allow for the assessment of soft non-calcified plaques, it has shown a good correlation with contrast-enhanced CT coronary angiography.
Related Links:
Catholic University of Korea
Yonsei University College of Medicine
Developed by researchers at the Catholic University of Korea (Seoul, South Korea), Yonsei University College of Medicine (Seoul, South Korea), and other institutions, the deep learning (DL)-based algorithm was initially trained and validated on 452 non-enhanced electrocardiogram-gated cardiac CT scans. It was then tested on a separate set of 137 cases, with each CT exam manually annotated by a radiologist with seven years of experience in cardiothoracic imaging, and AVC volume and Agatston scores were compared.
The results revealed that when manually measured AVC Agatston score was used as a benchmark, the accuracy of DL-measured AVC Agatston score for AVC volume grading was 97%, which was better than that of the four radiologist readers (77.8–89.9 %). The accuracy of DL algorithm for Agatston score was 92.9%. Overall, the DL model was deemed to be superior to all four radiologists for predicting severe aortic valve calcium cases. The study was published on February 6, 2021, in European Journal of Radiology.
“For observer performance testing, four radiologists determined AVC grade in two reading rounds. The diagnostic performance of DL-measured AVC volume and Agaston score for classifying severe AVC was compared with that of each reader's assessment,” explained lead author Suyon Chang, MD, of the Catholic University of Korea, and colleagues. “To validate AVC segmentation performance, the Dice coefficient [a statistic used to gauge the similarity of two samples] was calculated; after applying the DL algorithm, the Dice coefficient score was 0.807.”
The Agatston score is a semi-automated tool to calculate the extent of coronary artery calcification detected by an unenhanced low-dose CT scan, which is routinely performed in patients undergoing cardiac CT. It allows for early risk stratification as patients with a high Agatston score (over 160) have an increased risk for a major adverse cardiac event (MACE). Although it does not allow for the assessment of soft non-calcified plaques, it has shown a good correlation with contrast-enhanced CT coronary angiography.
Related Links:
Catholic University of Korea
Yonsei University College of Medicine
Latest Radiography News
- World's Largest Class Single Crystal Diamond Radiation Detector Opens New Possibilities for Diagnostic Imaging
- AI-Powered Imaging Technique Shows Promise in Evaluating Patients for PCI
- Higher Chest X-Ray Usage Catches Lung Cancer Earlier and Improves Survival
- AI-Powered Mammograms Predict Cardiovascular Risk
- Generative AI Model Significantly Reduces Chest X-Ray Reading Time
- AI-Powered Mammography Screening Boosts Cancer Detection in Single-Reader Settings
- Photon Counting Detectors Promise Fast Color X-Ray Images
- AI Can Flag Mammograms for Supplemental MRI
- 3D CT Imaging from Single X-Ray Projection Reduces Radiation Exposure
- AI Method Accurately Predicts Breast Cancer Risk by Analyzing Multiple Mammograms
- Printable Organic X-Ray Sensors Could Transform Treatment for Cancer Patients
- Highly Sensitive, Foldable Detector to Make X-Rays Safer
- Novel Breast Cancer Screening Technology Could Offer Superior Alternative to Mammogram
- Artificial Intelligence Accurately Predicts Breast Cancer Years Before Diagnosis
- AI-Powered Chest X-Ray Detects Pulmonary Nodules Three Years Before Lung Cancer Symptoms
- AI Model Identifies Vertebral Compression Fractures in Chest Radiographs
Channels
MRI
view channel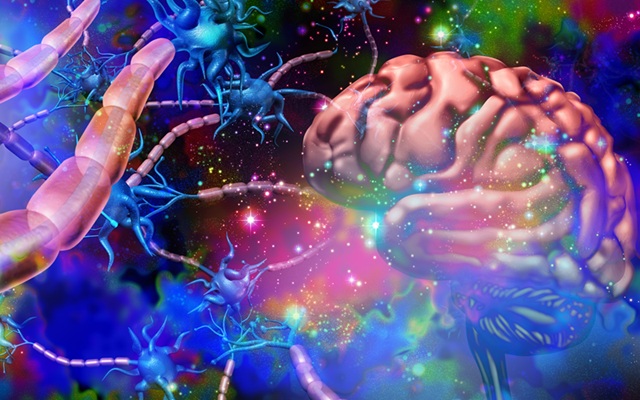
AI Tool Tracks Effectiveness of Multiple Sclerosis Treatments Using Brain MRI Scans
Multiple sclerosis (MS) is a condition in which the immune system attacks the brain and spinal cord, leading to impairments in movement, sensation, and cognition. Magnetic Resonance Imaging (MRI) markers... Read more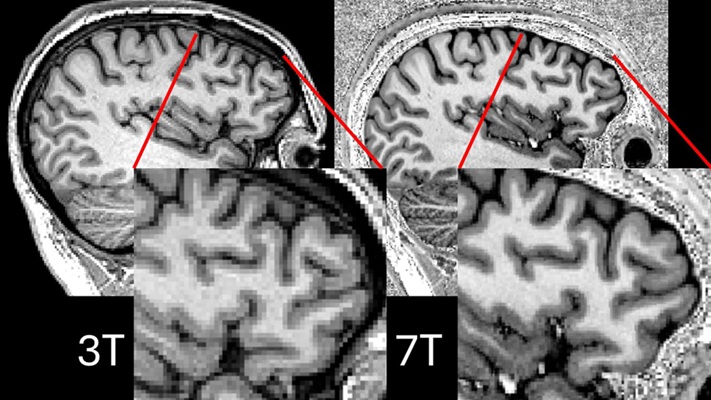
Ultra-Powerful MRI Scans Enable Life-Changing Surgery in Treatment-Resistant Epileptic Patients
Approximately 360,000 individuals in the UK suffer from focal epilepsy, a condition in which seizures spread from one part of the brain. Around a third of these patients experience persistent seizures... Read more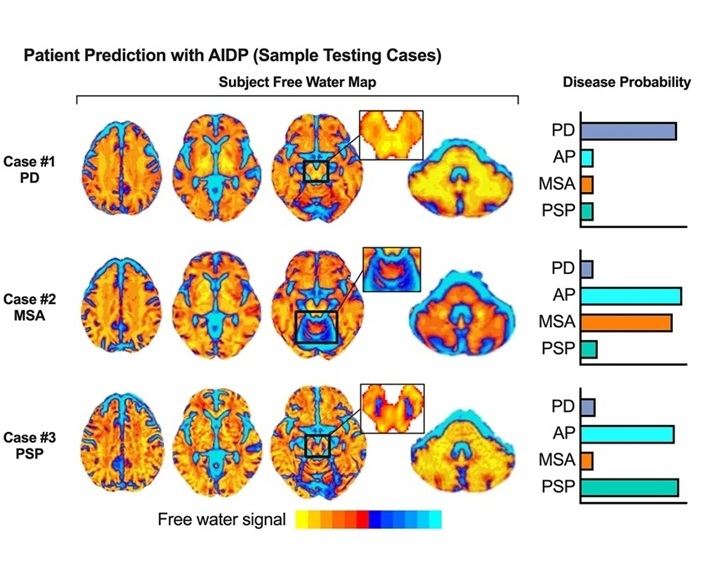
AI-Powered MRI Technology Improves Parkinson’s Diagnoses
Current research shows that the accuracy of diagnosing Parkinson’s disease typically ranges from 55% to 78% within the first five years of assessment. This is partly due to the similarities shared by Parkinson’s... Read more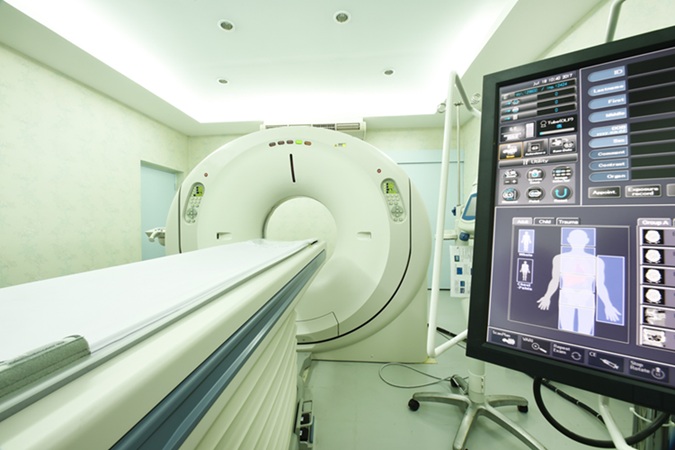
Biparametric MRI Combined with AI Enhances Detection of Clinically Significant Prostate Cancer
Artificial intelligence (AI) technologies are transforming the way medical images are analyzed, offering unprecedented capabilities in quantitatively extracting features that go beyond traditional visual... Read moreUltrasound
view channel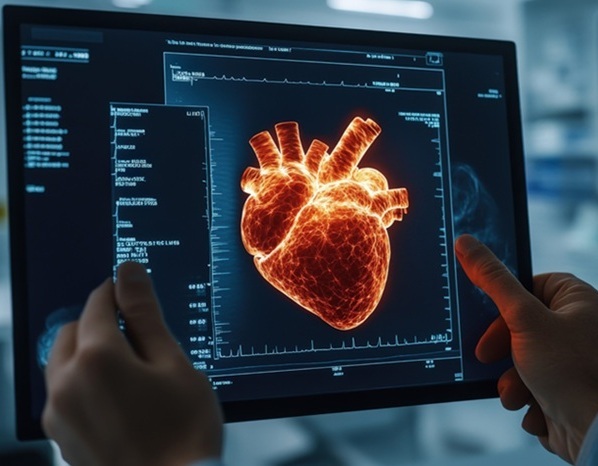
AI Identifies Heart Valve Disease from Common Imaging Test
Tricuspid regurgitation is a condition where the heart's tricuspid valve does not close completely during contraction, leading to backward blood flow, which can result in heart failure. A new artificial... Read more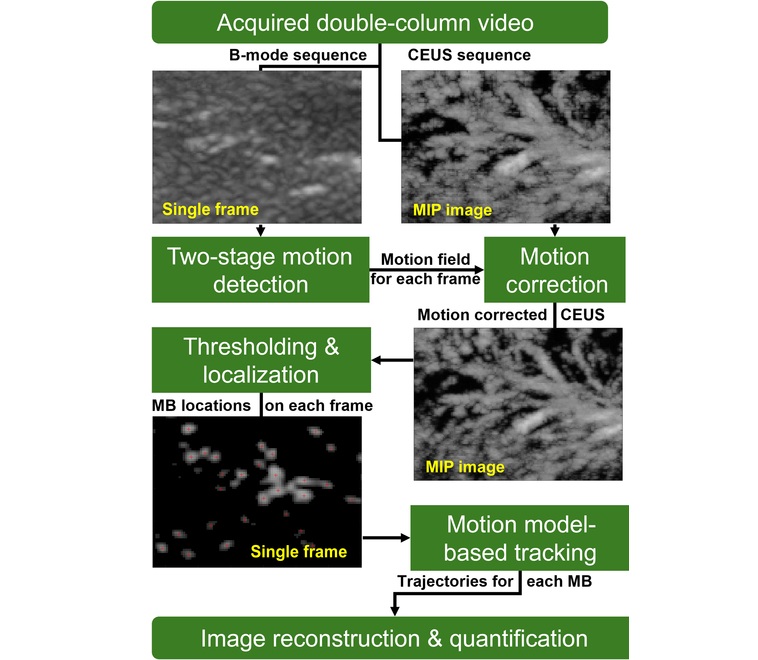
Novel Imaging Method Enables Early Diagnosis and Treatment Monitoring of Type 2 Diabetes
Type 2 diabetes is recognized as an autoimmune inflammatory disease, where chronic inflammation leads to alterations in pancreatic islet microvasculature, a key factor in β-cell dysfunction.... Read moreNuclear Medicine
view channel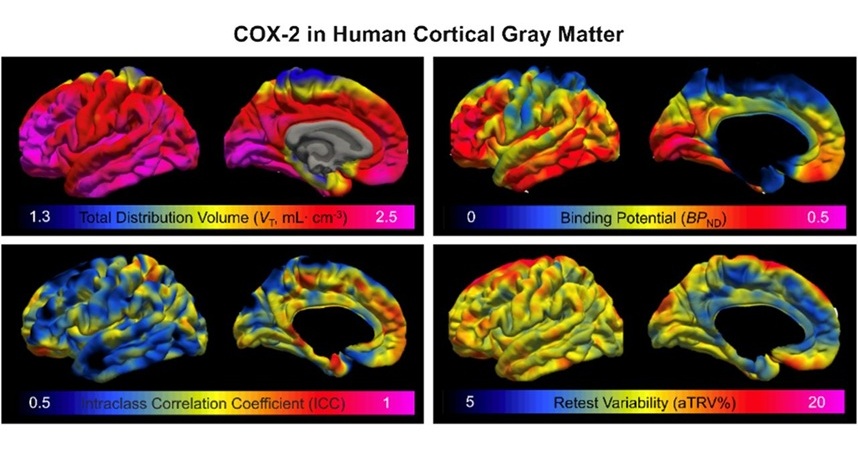
Novel PET Imaging Approach Offers Never-Before-Seen View of Neuroinflammation
COX-2, an enzyme that plays a key role in brain inflammation, can be significantly upregulated by inflammatory stimuli and neuroexcitation. Researchers suggest that COX-2 density in the brain could serve... Read more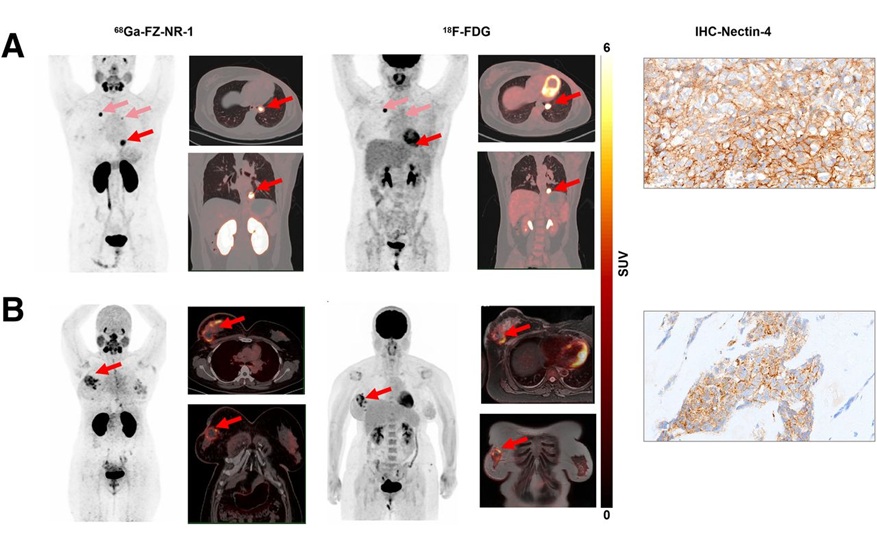
Novel Radiotracer Identifies Biomarker for Triple-Negative Breast Cancer
Triple-negative breast cancer (TNBC), which represents 15-20% of all breast cancer cases, is one of the most aggressive subtypes, with a five-year survival rate of about 40%. Due to its significant heterogeneity... Read moreGeneral/Advanced Imaging
view channel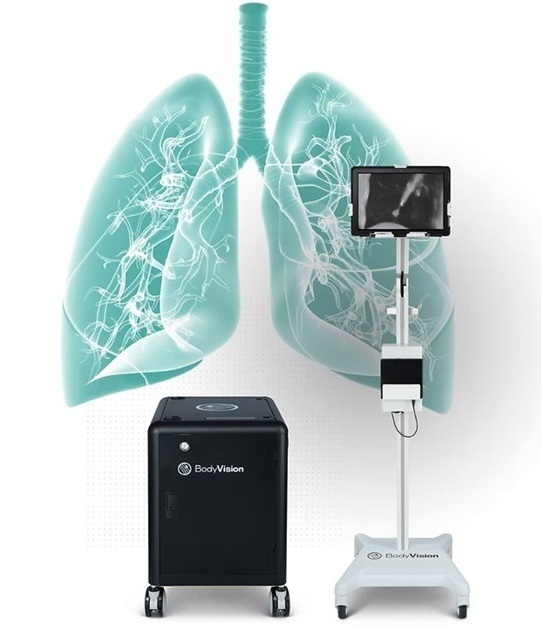
AI-Powered Imaging System Improves Lung Cancer Diagnosis
Given the need to detect lung cancer at earlier stages, there is an increasing need for a definitive diagnostic pathway for patients with suspicious pulmonary nodules. However, obtaining tissue samples... Read more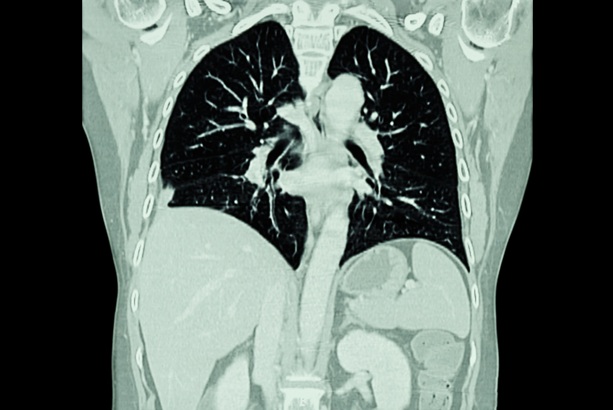
AI Model Significantly Enhances Low-Dose CT Capabilities
Lung cancer remains one of the most challenging diseases, making early diagnosis vital for effective treatment. Fortunately, advancements in artificial intelligence (AI) are revolutionizing lung cancer... Read moreImaging IT
view channel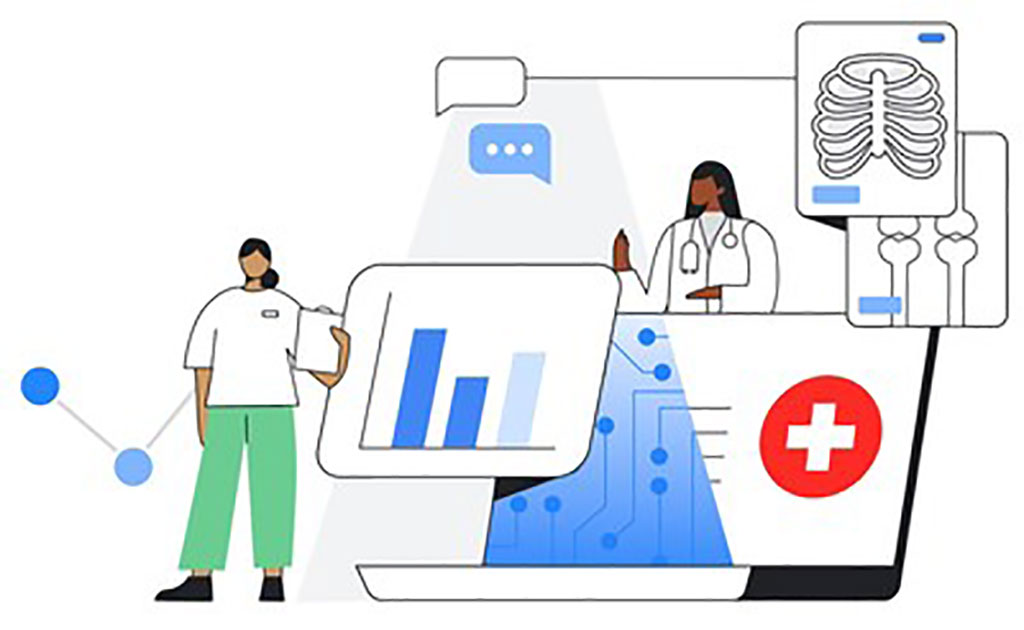
New Google Cloud Medical Imaging Suite Makes Imaging Healthcare Data More Accessible
Medical imaging is a critical tool used to diagnose patients, and there are billions of medical images scanned globally each year. Imaging data accounts for about 90% of all healthcare data1 and, until... Read more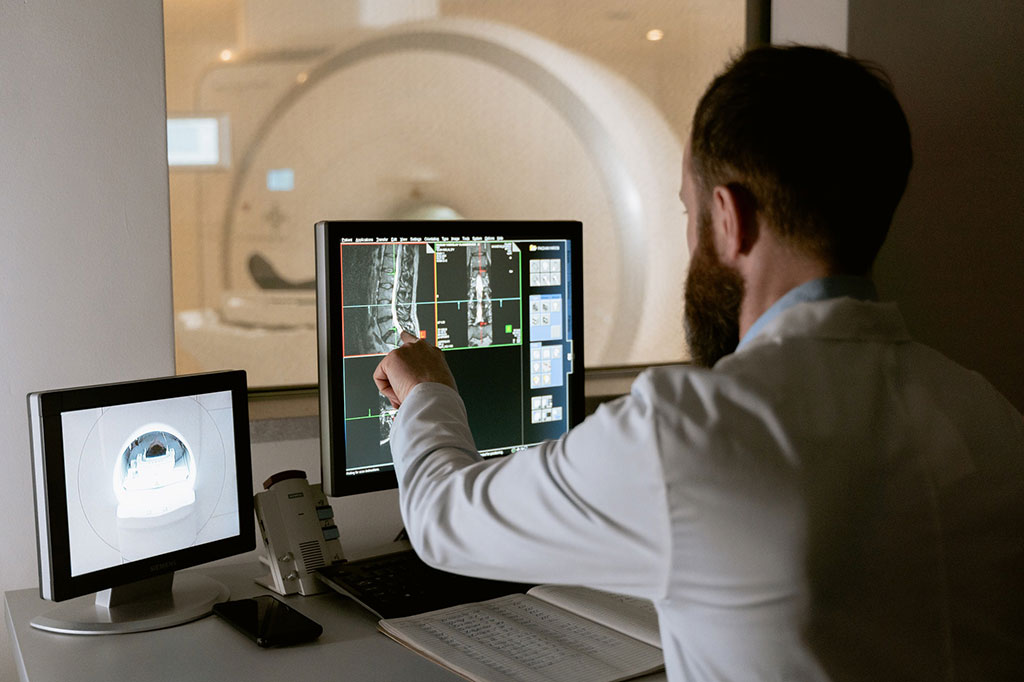
Global AI in Medical Diagnostics Market to Be Driven by Demand for Image Recognition in Radiology
The global artificial intelligence (AI) in medical diagnostics market is expanding with early disease detection being one of its key applications and image recognition becoming a compelling consumer proposition... Read moreIndustry News
view channel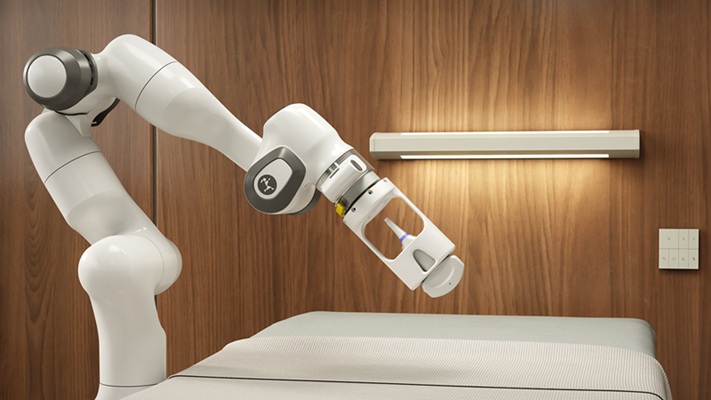
GE HealthCare and NVIDIA Collaboration to Reimagine Diagnostic Imaging
GE HealthCare (Chicago, IL, USA) has entered into a collaboration with NVIDIA (Santa Clara, CA, USA), expanding the existing relationship between the two companies to focus on pioneering innovation in... Read more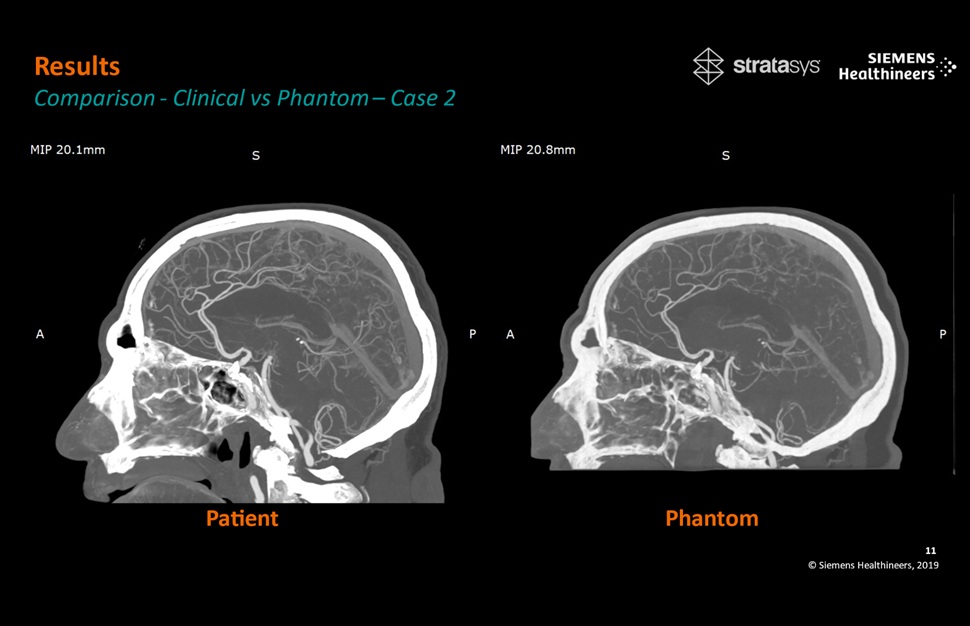
Patient-Specific 3D-Printed Phantoms Transform CT Imaging
New research has highlighted how anatomically precise, patient-specific 3D-printed phantoms are proving to be scalable, cost-effective, and efficient tools in the development of new CT scan algorithms... Read more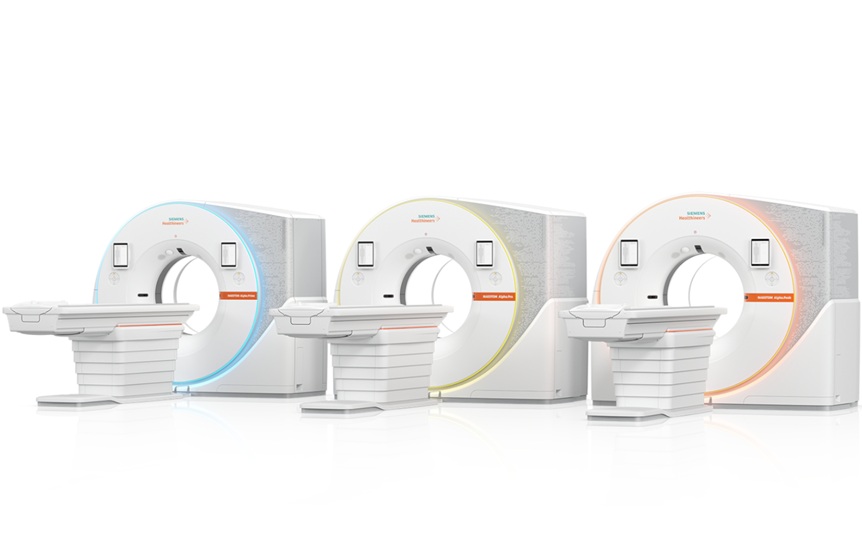
Siemens and Sectra Collaborate on Enhancing Radiology Workflows
Siemens Healthineers (Forchheim, Germany) and Sectra (Linköping, Sweden) have entered into a collaboration aimed at enhancing radiologists' diagnostic capabilities and, in turn, improving patient care... Read more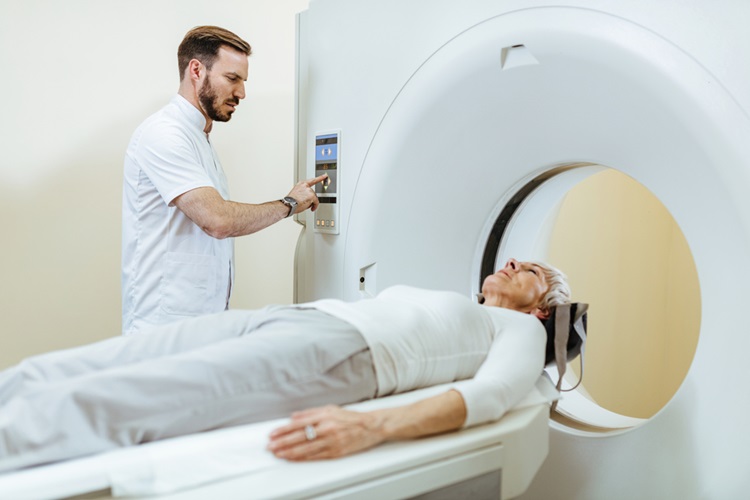