CT Radiomics Helps Classify Small Lung Nodules
By MedImaging International staff writers Posted on 01 Feb 2021 |
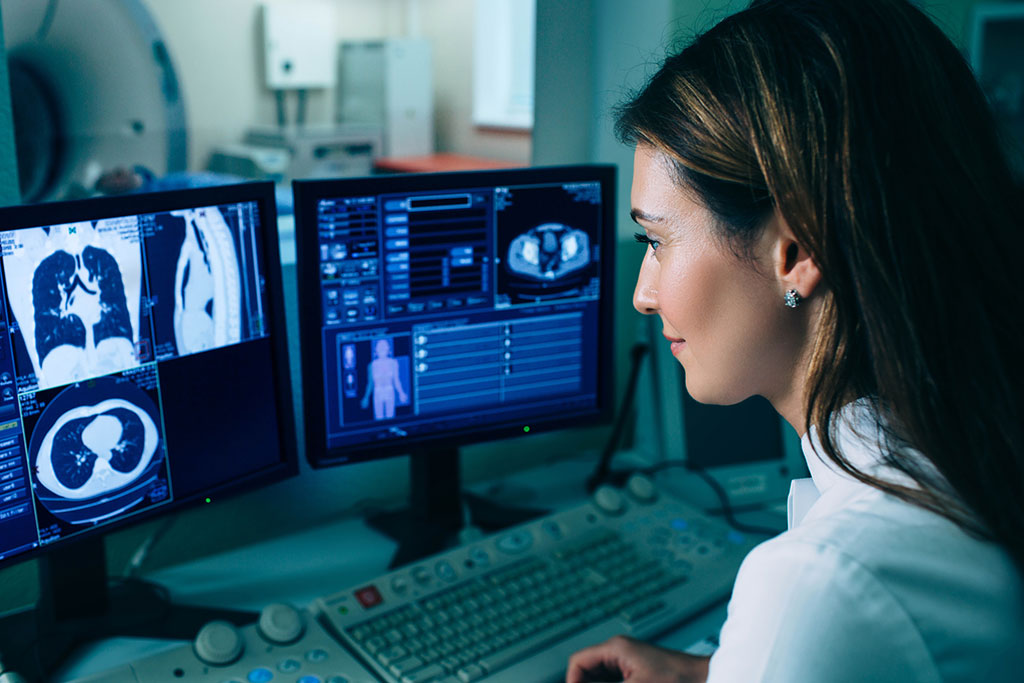
Image: CT radiomics can help classify lung nodule malignancy (Photo courtesy of Getty Images)
A machine-learning (ML) algorithm can be highly accurate for classifying very small lung nodules found in low-dose CT lung screening programs, according to a new study.
Researchers at the BC Cancer Research Center (BCCRC; Vancouver, Canada) trained a linear discriminant analysis (LDA) ML algorithm--using data from the Pan-Canadian Early Detection of Lung Cancer (PanCan) study--to characterize, analyze, and classify small lung nodules as malignant or benign by extracting approximately 170 texture and shape radiomic features, following semi-automated nodule segmentation on the images. They then compared the performance of the algorithm with that of the Prostate, Lung, Colorectal, and Ovarian (PLCO) m2012 malignancy risk score calculator on another dataset.
The study cohort consisted of 139 malignant nodules and 472 benign nodules that were approximately matched in size. The researchers applied size restrictions (based on Lung-RADS classification criteria) to remove any nodules from the dataset that would already be considered suspicious, which would include any nodule with solid components greater than 8 mm in diameter. The results showed the ML algorithm significantly outperformed the (PLCO) m2012 risk-prediction model, especially when demographic data were added to radiomics analysis. The study was presented at the AACR Virtual Special Conference on Artificial Intelligence, Diagnosis, and Imaging, held during January 2021.
“The best results were achieved in a subset of patients who were younger than 64, female, did not have emphysema, smoked fewer than 42 pack years, did not have a family history of lung cancer, and were not current smokers,” said senior author and study presenter Rohan Abraham, PhD. “Combined with clinician expertise and experience, this has the potential to enable earlier intervention and reduce the need for follow-up CT.”
Current lung nodule classification relies on nodule size, a factor that is of limited use for sub-centimeter nodules, or on volume doubling time, a variable that requires follow-up CT exams. As a result, very small lung nodules, with solid components of less than 8 mm in diameter (and therefore below the Lung-RADS 4A risk-stratification threshold), are very difficult to classify, and they are often given a "wait and see" management plan.
Related Links:
BC Cancer Research Center
Researchers at the BC Cancer Research Center (BCCRC; Vancouver, Canada) trained a linear discriminant analysis (LDA) ML algorithm--using data from the Pan-Canadian Early Detection of Lung Cancer (PanCan) study--to characterize, analyze, and classify small lung nodules as malignant or benign by extracting approximately 170 texture and shape radiomic features, following semi-automated nodule segmentation on the images. They then compared the performance of the algorithm with that of the Prostate, Lung, Colorectal, and Ovarian (PLCO) m2012 malignancy risk score calculator on another dataset.
The study cohort consisted of 139 malignant nodules and 472 benign nodules that were approximately matched in size. The researchers applied size restrictions (based on Lung-RADS classification criteria) to remove any nodules from the dataset that would already be considered suspicious, which would include any nodule with solid components greater than 8 mm in diameter. The results showed the ML algorithm significantly outperformed the (PLCO) m2012 risk-prediction model, especially when demographic data were added to radiomics analysis. The study was presented at the AACR Virtual Special Conference on Artificial Intelligence, Diagnosis, and Imaging, held during January 2021.
“The best results were achieved in a subset of patients who were younger than 64, female, did not have emphysema, smoked fewer than 42 pack years, did not have a family history of lung cancer, and were not current smokers,” said senior author and study presenter Rohan Abraham, PhD. “Combined with clinician expertise and experience, this has the potential to enable earlier intervention and reduce the need for follow-up CT.”
Current lung nodule classification relies on nodule size, a factor that is of limited use for sub-centimeter nodules, or on volume doubling time, a variable that requires follow-up CT exams. As a result, very small lung nodules, with solid components of less than 8 mm in diameter (and therefore below the Lung-RADS 4A risk-stratification threshold), are very difficult to classify, and they are often given a "wait and see" management plan.
Related Links:
BC Cancer Research Center
Latest Radiography News
- World's Largest Class Single Crystal Diamond Radiation Detector Opens New Possibilities for Diagnostic Imaging
- AI-Powered Imaging Technique Shows Promise in Evaluating Patients for PCI
- Higher Chest X-Ray Usage Catches Lung Cancer Earlier and Improves Survival
- AI-Powered Mammograms Predict Cardiovascular Risk
- Generative AI Model Significantly Reduces Chest X-Ray Reading Time
- AI-Powered Mammography Screening Boosts Cancer Detection in Single-Reader Settings
- Photon Counting Detectors Promise Fast Color X-Ray Images
- AI Can Flag Mammograms for Supplemental MRI
- 3D CT Imaging from Single X-Ray Projection Reduces Radiation Exposure
- AI Method Accurately Predicts Breast Cancer Risk by Analyzing Multiple Mammograms
- Printable Organic X-Ray Sensors Could Transform Treatment for Cancer Patients
- Highly Sensitive, Foldable Detector to Make X-Rays Safer
- Novel Breast Cancer Screening Technology Could Offer Superior Alternative to Mammogram
- Artificial Intelligence Accurately Predicts Breast Cancer Years Before Diagnosis
- AI-Powered Chest X-Ray Detects Pulmonary Nodules Three Years Before Lung Cancer Symptoms
- AI Model Identifies Vertebral Compression Fractures in Chest Radiographs
Channels
MRI
view channel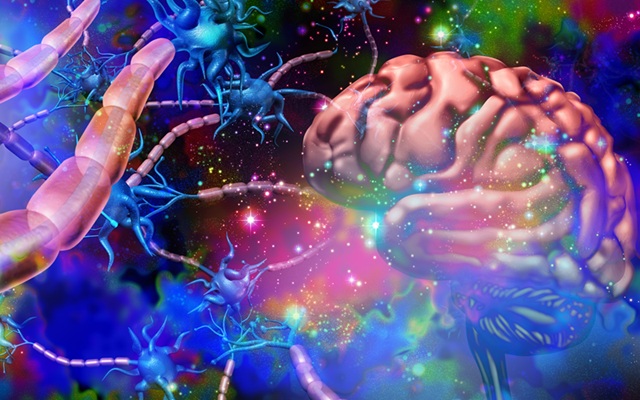
AI Tool Tracks Effectiveness of Multiple Sclerosis Treatments Using Brain MRI Scans
Multiple sclerosis (MS) is a condition in which the immune system attacks the brain and spinal cord, leading to impairments in movement, sensation, and cognition. Magnetic Resonance Imaging (MRI) markers... Read more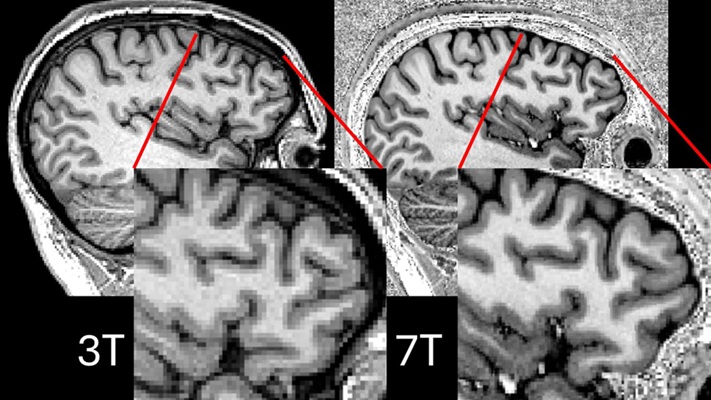
Ultra-Powerful MRI Scans Enable Life-Changing Surgery in Treatment-Resistant Epileptic Patients
Approximately 360,000 individuals in the UK suffer from focal epilepsy, a condition in which seizures spread from one part of the brain. Around a third of these patients experience persistent seizures... Read more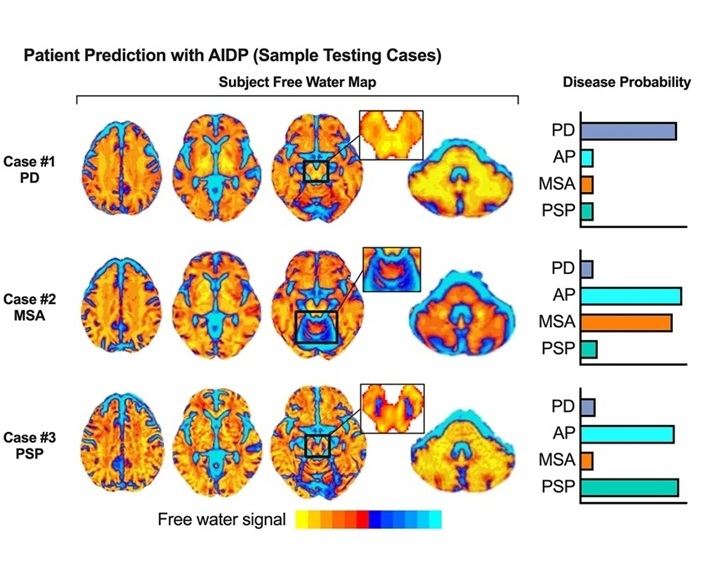
AI-Powered MRI Technology Improves Parkinson’s Diagnoses
Current research shows that the accuracy of diagnosing Parkinson’s disease typically ranges from 55% to 78% within the first five years of assessment. This is partly due to the similarities shared by Parkinson’s... Read more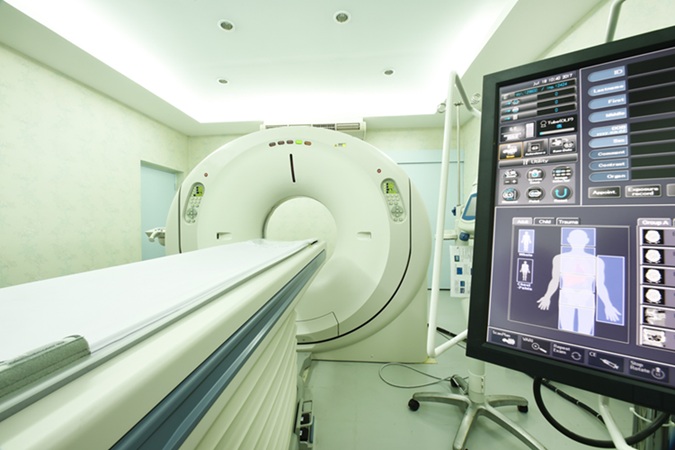
Biparametric MRI Combined with AI Enhances Detection of Clinically Significant Prostate Cancer
Artificial intelligence (AI) technologies are transforming the way medical images are analyzed, offering unprecedented capabilities in quantitatively extracting features that go beyond traditional visual... Read moreUltrasound
view channel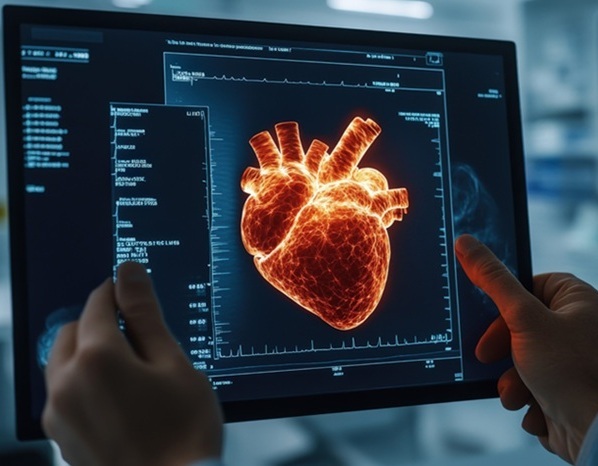
AI Identifies Heart Valve Disease from Common Imaging Test
Tricuspid regurgitation is a condition where the heart's tricuspid valve does not close completely during contraction, leading to backward blood flow, which can result in heart failure. A new artificial... Read more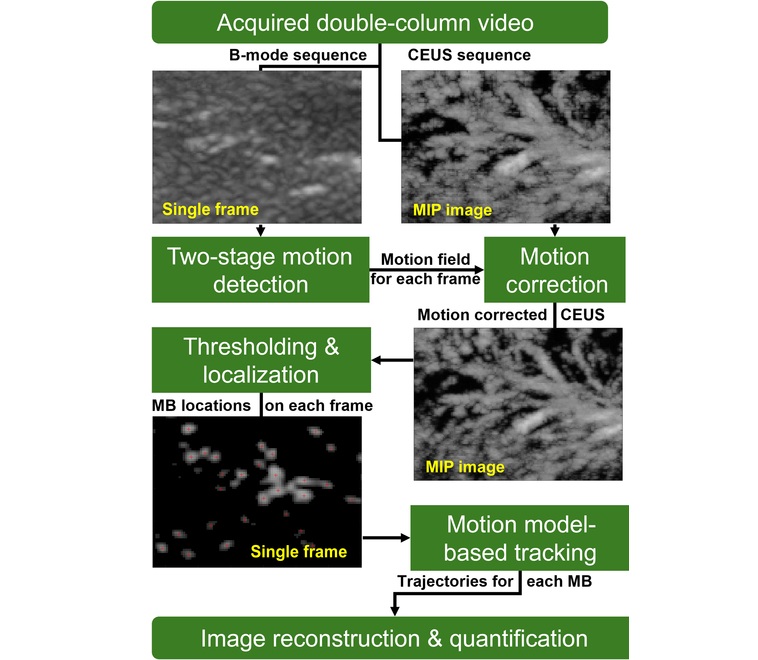
Novel Imaging Method Enables Early Diagnosis and Treatment Monitoring of Type 2 Diabetes
Type 2 diabetes is recognized as an autoimmune inflammatory disease, where chronic inflammation leads to alterations in pancreatic islet microvasculature, a key factor in β-cell dysfunction.... Read moreNuclear Medicine
view channel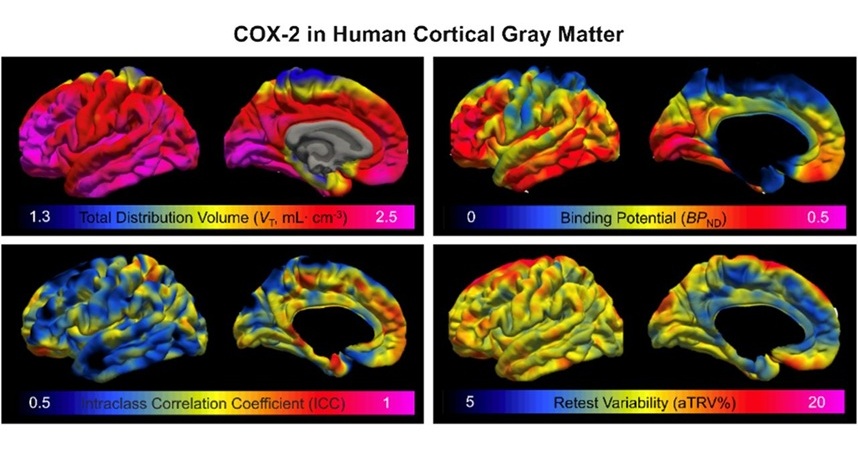
Novel PET Imaging Approach Offers Never-Before-Seen View of Neuroinflammation
COX-2, an enzyme that plays a key role in brain inflammation, can be significantly upregulated by inflammatory stimuli and neuroexcitation. Researchers suggest that COX-2 density in the brain could serve... Read more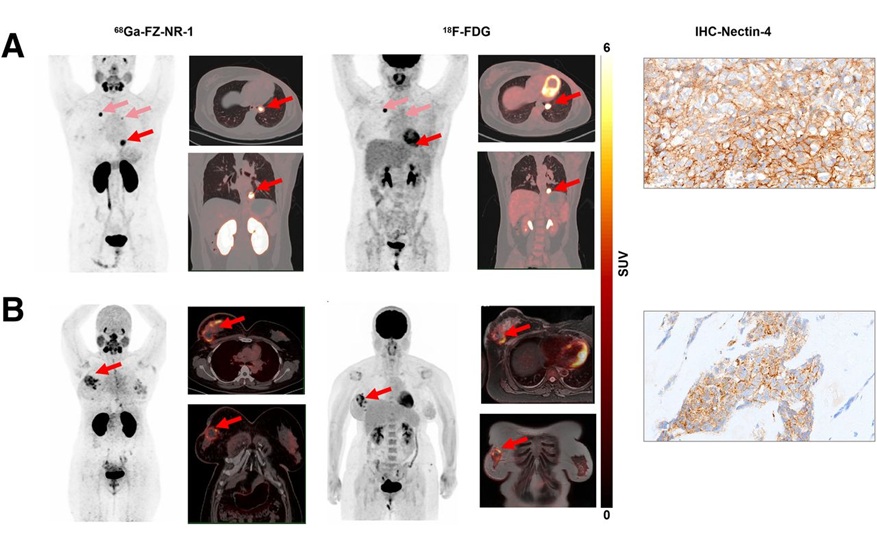
Novel Radiotracer Identifies Biomarker for Triple-Negative Breast Cancer
Triple-negative breast cancer (TNBC), which represents 15-20% of all breast cancer cases, is one of the most aggressive subtypes, with a five-year survival rate of about 40%. Due to its significant heterogeneity... Read moreGeneral/Advanced Imaging
view channel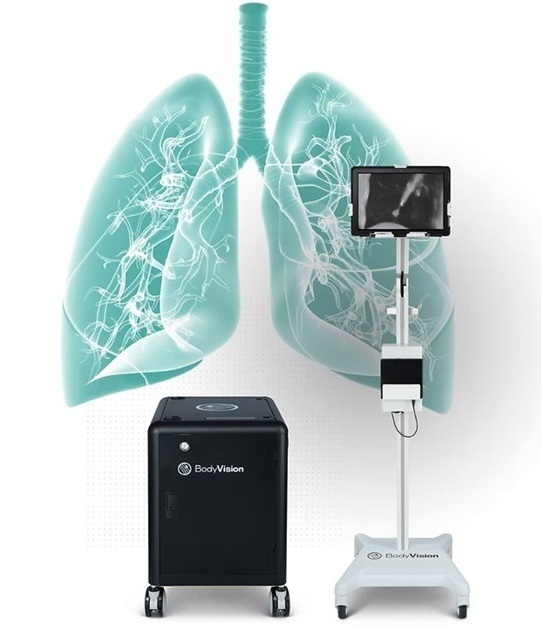
AI-Powered Imaging System Improves Lung Cancer Diagnosis
Given the need to detect lung cancer at earlier stages, there is an increasing need for a definitive diagnostic pathway for patients with suspicious pulmonary nodules. However, obtaining tissue samples... Read more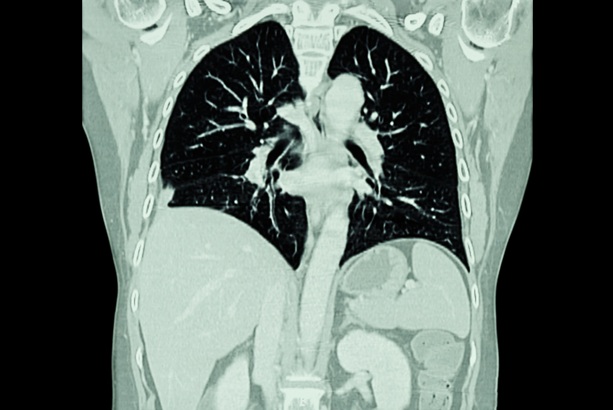
AI Model Significantly Enhances Low-Dose CT Capabilities
Lung cancer remains one of the most challenging diseases, making early diagnosis vital for effective treatment. Fortunately, advancements in artificial intelligence (AI) are revolutionizing lung cancer... Read moreImaging IT
view channel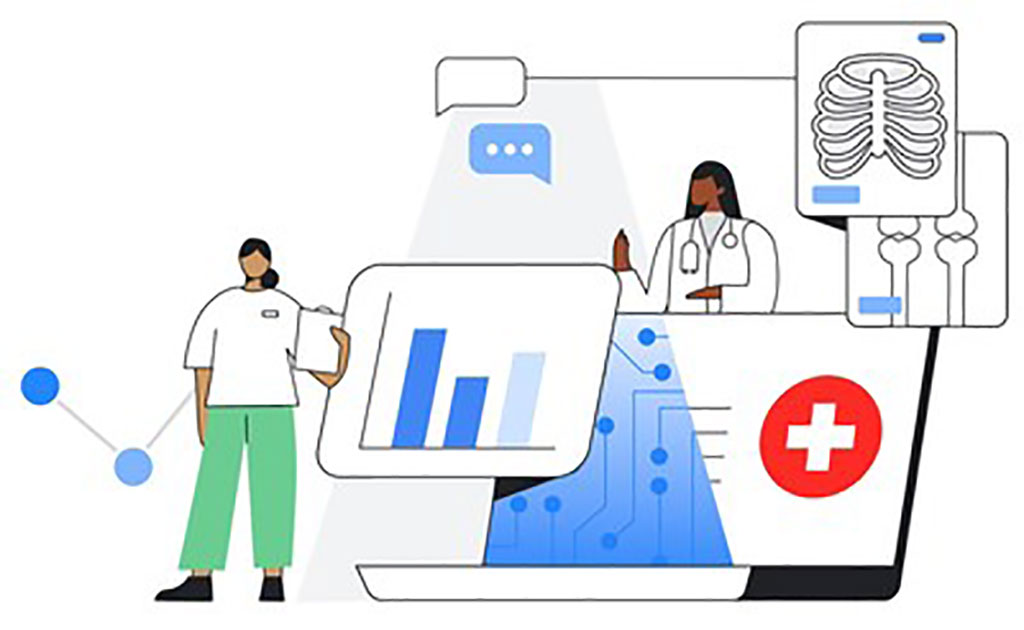
New Google Cloud Medical Imaging Suite Makes Imaging Healthcare Data More Accessible
Medical imaging is a critical tool used to diagnose patients, and there are billions of medical images scanned globally each year. Imaging data accounts for about 90% of all healthcare data1 and, until... Read more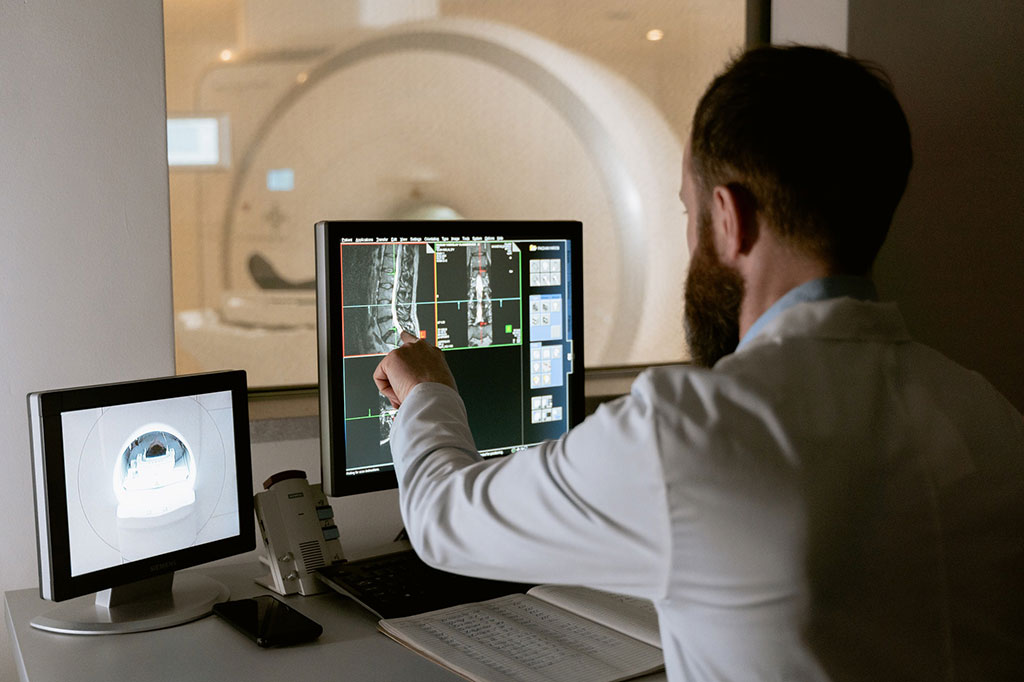
Global AI in Medical Diagnostics Market to Be Driven by Demand for Image Recognition in Radiology
The global artificial intelligence (AI) in medical diagnostics market is expanding with early disease detection being one of its key applications and image recognition becoming a compelling consumer proposition... Read moreIndustry News
view channel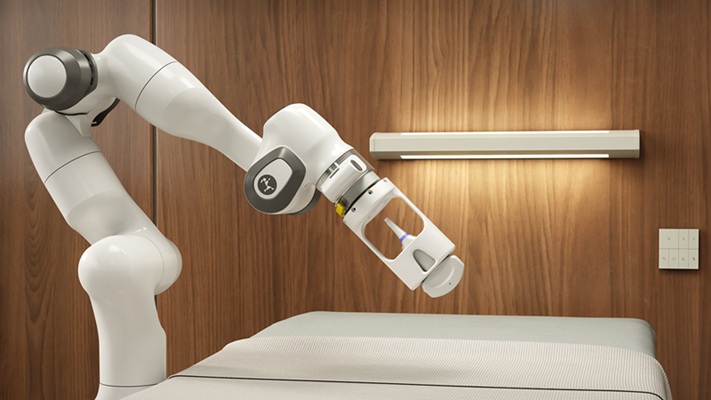
GE HealthCare and NVIDIA Collaboration to Reimagine Diagnostic Imaging
GE HealthCare (Chicago, IL, USA) has entered into a collaboration with NVIDIA (Santa Clara, CA, USA), expanding the existing relationship between the two companies to focus on pioneering innovation in... Read more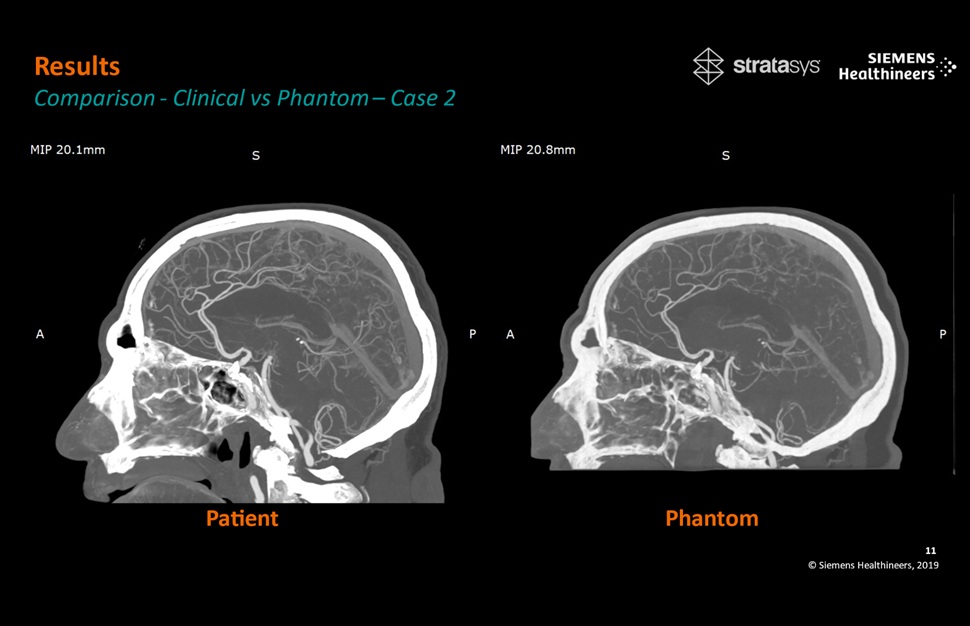
Patient-Specific 3D-Printed Phantoms Transform CT Imaging
New research has highlighted how anatomically precise, patient-specific 3D-printed phantoms are proving to be scalable, cost-effective, and efficient tools in the development of new CT scan algorithms... Read more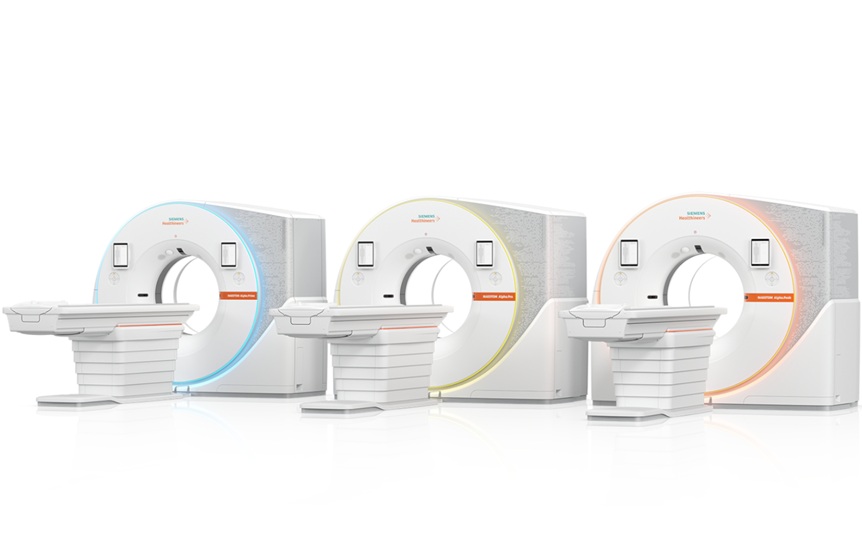
Siemens and Sectra Collaborate on Enhancing Radiology Workflows
Siemens Healthineers (Forchheim, Germany) and Sectra (Linköping, Sweden) have entered into a collaboration aimed at enhancing radiologists' diagnostic capabilities and, in turn, improving patient care... Read more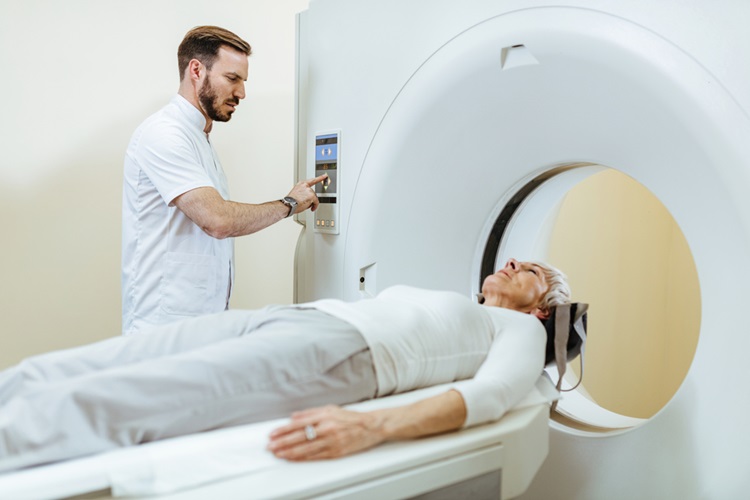