Combined MRI and AI Can Identify Focal Dystonia Instantly
By MedImaging International staff writers Posted on 13 Oct 2020 |
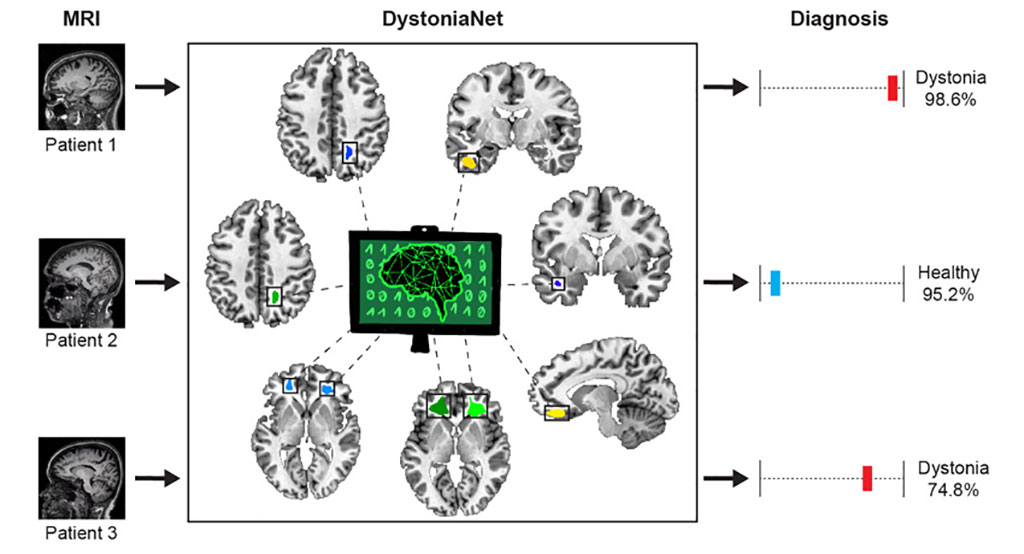
Image: Comparison of the MRI scans processed by DystoniaNet (Photo courtesy of MEEI)
The conjunction of magnetic resonance imaging (MRI) and artificial intelligence (AI) can identify patients with dystonia in under a second, according to a new study.
The new platform, called DystoniaNet, developed at the Massachusetts Eye and Ear Infirmary (MEEI; Boston, USA), can identify a microstructural neural network biomarker that allows objective and accurate diagnosis of isolated dystonia, based on the disorder pathophysiology and MRI structural brain images. The algorithm was developed using the MRI’s of 612 subjects, including 392 with three forms of isolated focal dystonia (laryngeal dystonia, cervical dystonia, and blepharospasm), and those of 220 healthy controls.
DystoniaNet successfully identified clusters in the corpus callosum, the anterior and posterior thalamic radiations, the inferior fronto-occipital fasciculus, and inferior temporal and superior orbital gyri as the biomarker components, regions that are known to contribute to abnormal interhemispheric information transfer, heteromodal sensorimotor processing, and executive control of motor commands. In all, the DystoniaNet AI biomarker showed an accuracy of 98.8% in diagnosing dystonia, with a referral of 3.5% of cases due to diagnostic uncertainty.
DystoniaNet also outperformed shallow machine-learning algorithms, showing nearly a 20% increase in benchmark diagnostic performance. Importantly, the microstructural neural network biomarker and its DystoniaNet platform showed substantial improvement over the current 34% agreement on dystonia diagnosis between clinicians. In addition, the diagnostic decision by DystoniaNet was computed in just 0.36 seconds. The study was published on October 1, 2020, in PNAS.
“We basically leveraged the advances made in deep learning and designed an architecture that was able to look at raw structural MRI and find a biomarker for dystonia that could help with the diagnosis of this disorder,” said study author Davide Valeriani, PhD. “We specifically took an approach that could be easily clinically translated, which is why we used raw structural MRI. Given the platform's performance and the average length of time to diagnosis, it has potential to beneficial impact the movement disorder field.”
Dystonia is a neurological disorder of heterogeneous pathophysiology, which causes involuntary muscle contractions leading to abnormal movements and postures. Its diagnosis is remarkably challenging due to the absence of a biomarker or gold standard diagnostic test. This leads to a low agreement between clinicians, with up to 50% of cases being misdiagnosed and diagnostic delays extending up to 10 years.
Related Links:
Massachusetts Eye and Ear Infirmary
The new platform, called DystoniaNet, developed at the Massachusetts Eye and Ear Infirmary (MEEI; Boston, USA), can identify a microstructural neural network biomarker that allows objective and accurate diagnosis of isolated dystonia, based on the disorder pathophysiology and MRI structural brain images. The algorithm was developed using the MRI’s of 612 subjects, including 392 with three forms of isolated focal dystonia (laryngeal dystonia, cervical dystonia, and blepharospasm), and those of 220 healthy controls.
DystoniaNet successfully identified clusters in the corpus callosum, the anterior and posterior thalamic radiations, the inferior fronto-occipital fasciculus, and inferior temporal and superior orbital gyri as the biomarker components, regions that are known to contribute to abnormal interhemispheric information transfer, heteromodal sensorimotor processing, and executive control of motor commands. In all, the DystoniaNet AI biomarker showed an accuracy of 98.8% in diagnosing dystonia, with a referral of 3.5% of cases due to diagnostic uncertainty.
DystoniaNet also outperformed shallow machine-learning algorithms, showing nearly a 20% increase in benchmark diagnostic performance. Importantly, the microstructural neural network biomarker and its DystoniaNet platform showed substantial improvement over the current 34% agreement on dystonia diagnosis between clinicians. In addition, the diagnostic decision by DystoniaNet was computed in just 0.36 seconds. The study was published on October 1, 2020, in PNAS.
“We basically leveraged the advances made in deep learning and designed an architecture that was able to look at raw structural MRI and find a biomarker for dystonia that could help with the diagnosis of this disorder,” said study author Davide Valeriani, PhD. “We specifically took an approach that could be easily clinically translated, which is why we used raw structural MRI. Given the platform's performance and the average length of time to diagnosis, it has potential to beneficial impact the movement disorder field.”
Dystonia is a neurological disorder of heterogeneous pathophysiology, which causes involuntary muscle contractions leading to abnormal movements and postures. Its diagnosis is remarkably challenging due to the absence of a biomarker or gold standard diagnostic test. This leads to a low agreement between clinicians, with up to 50% of cases being misdiagnosed and diagnostic delays extending up to 10 years.
Related Links:
Massachusetts Eye and Ear Infirmary
Latest MRI News
- Cutting-Edge MRI Technology to Revolutionize Diagnosis of Common Heart Problem
- New MRI Technique Reveals True Heart Age to Prevent Attacks and Strokes
- AI Tool Predicts Relapse of Pediatric Brain Cancer from Brain MRI Scans
- AI Tool Tracks Effectiveness of Multiple Sclerosis Treatments Using Brain MRI Scans
- Ultra-Powerful MRI Scans Enable Life-Changing Surgery in Treatment-Resistant Epileptic Patients
- AI-Powered MRI Technology Improves Parkinson’s Diagnoses
- Biparametric MRI Combined with AI Enhances Detection of Clinically Significant Prostate Cancer
- First-Of-Its-Kind AI-Driven Brain Imaging Platform to Better Guide Stroke Treatment Options
- New Model Improves Comparison of MRIs Taken at Different Institutions
- Groundbreaking New Scanner Sees 'Previously Undetectable' Cancer Spread
- First-Of-Its-Kind Tool Analyzes MRI Scans to Measure Brain Aging
- AI-Enhanced MRI Images Make Cancerous Breast Tissue Glow
- AI Model Automatically Segments MRI Images
- New Research Supports Routine Brain MRI Screening in Asymptomatic Late-Stage Breast Cancer Patients
- Revolutionary Portable Device Performs Rapid MRI-Based Stroke Imaging at Patient's Bedside
- AI Predicts After-Effects of Brain Tumor Surgery from MRI Scans
Channels
Radiography
view channel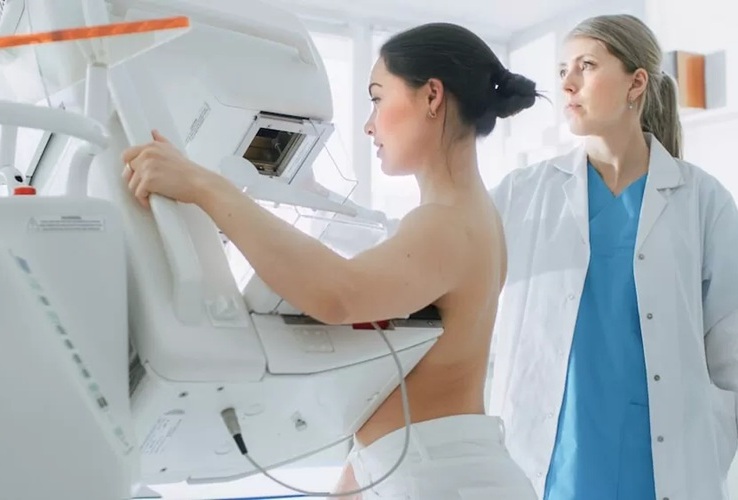
AI Improves Early Detection of Interval Breast Cancers
Interval breast cancers, which occur between routine screenings, are easier to treat when detected earlier. Early detection can reduce the need for aggressive treatments and improve the chances of better outcomes.... Read more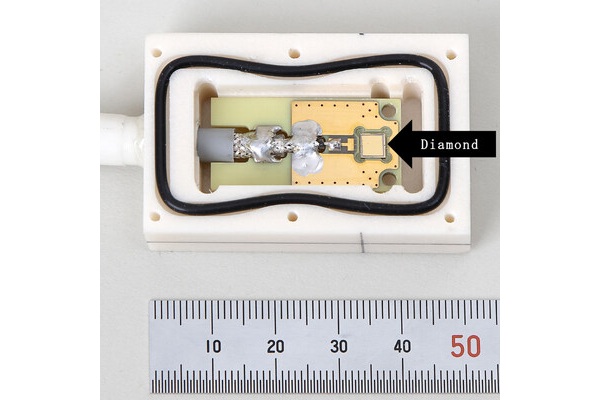
World's Largest Class Single Crystal Diamond Radiation Detector Opens New Possibilities for Diagnostic Imaging
Diamonds possess ideal physical properties for radiation detection, such as exceptional thermal and chemical stability along with a quick response time. Made of carbon with an atomic number of six, diamonds... Read moreUltrasound
view channel.jpeg)
AI-Powered Lung Ultrasound Outperforms Human Experts in Tuberculosis Diagnosis
Despite global declines in tuberculosis (TB) rates in previous years, the incidence of TB rose by 4.6% from 2020 to 2023. Early screening and rapid diagnosis are essential elements of the World Health... Read more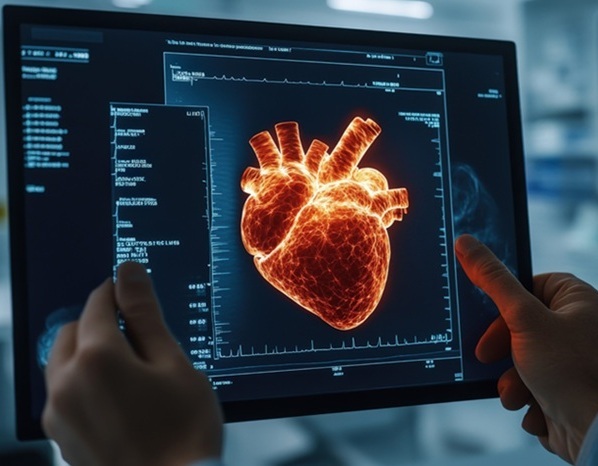
AI Identifies Heart Valve Disease from Common Imaging Test
Tricuspid regurgitation is a condition where the heart's tricuspid valve does not close completely during contraction, leading to backward blood flow, which can result in heart failure. A new artificial... Read moreNuclear Medicine
view channel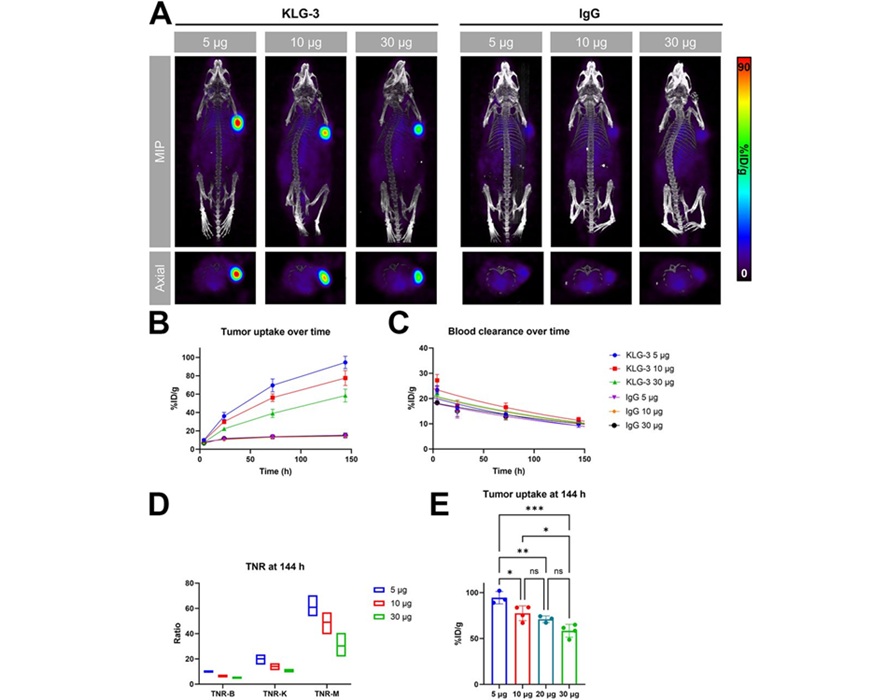
Novel Radiolabeled Antibody Improves Diagnosis and Treatment of Solid Tumors
Interleukin-13 receptor α-2 (IL13Rα2) is a cell surface receptor commonly found in solid tumors such as glioblastoma, melanoma, and breast cancer. It is minimally expressed in normal tissues, making it... Read more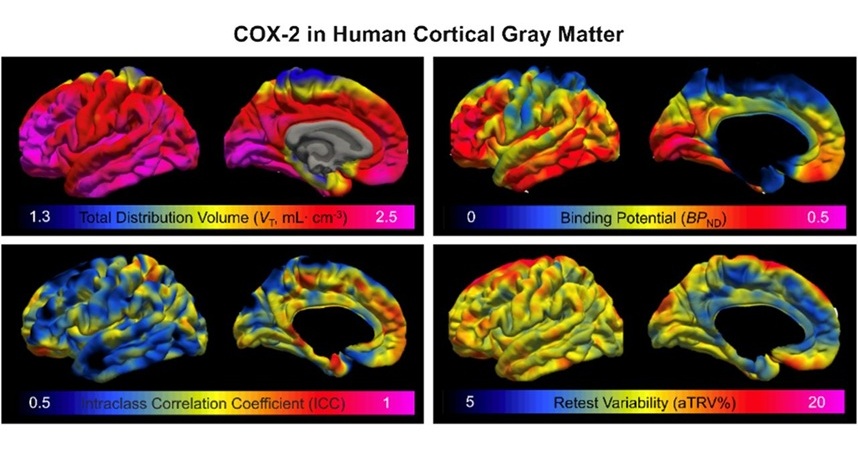
Novel PET Imaging Approach Offers Never-Before-Seen View of Neuroinflammation
COX-2, an enzyme that plays a key role in brain inflammation, can be significantly upregulated by inflammatory stimuli and neuroexcitation. Researchers suggest that COX-2 density in the brain could serve... Read moreGeneral/Advanced Imaging
view channel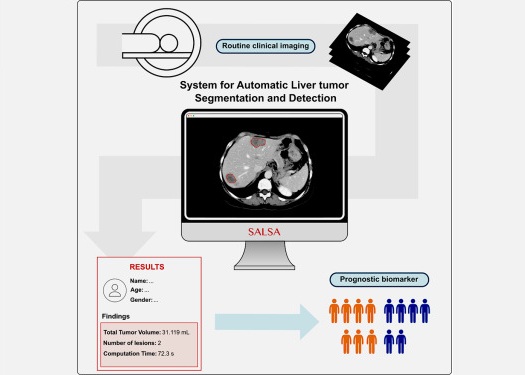
CT-Based Deep Learning-Driven Tool to Enhance Liver Cancer Diagnosis
Medical imaging, such as computed tomography (CT) scans, plays a crucial role in oncology, offering essential data for cancer detection, treatment planning, and monitoring of response to therapies.... Read more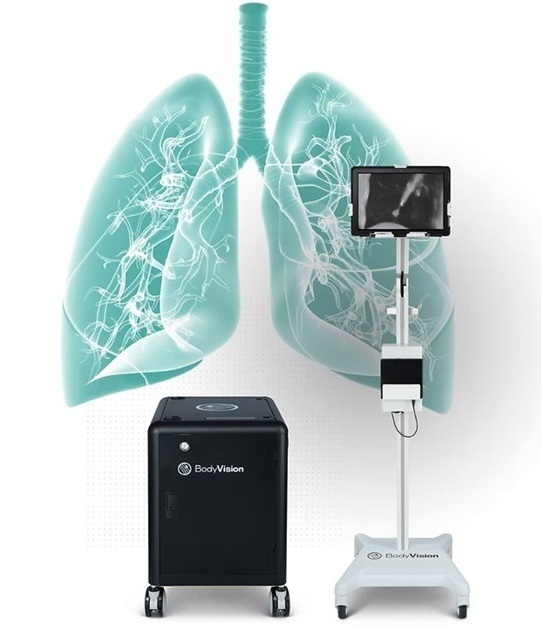
AI-Powered Imaging System Improves Lung Cancer Diagnosis
Given the need to detect lung cancer at earlier stages, there is an increasing need for a definitive diagnostic pathway for patients with suspicious pulmonary nodules. However, obtaining tissue samples... Read moreImaging IT
view channel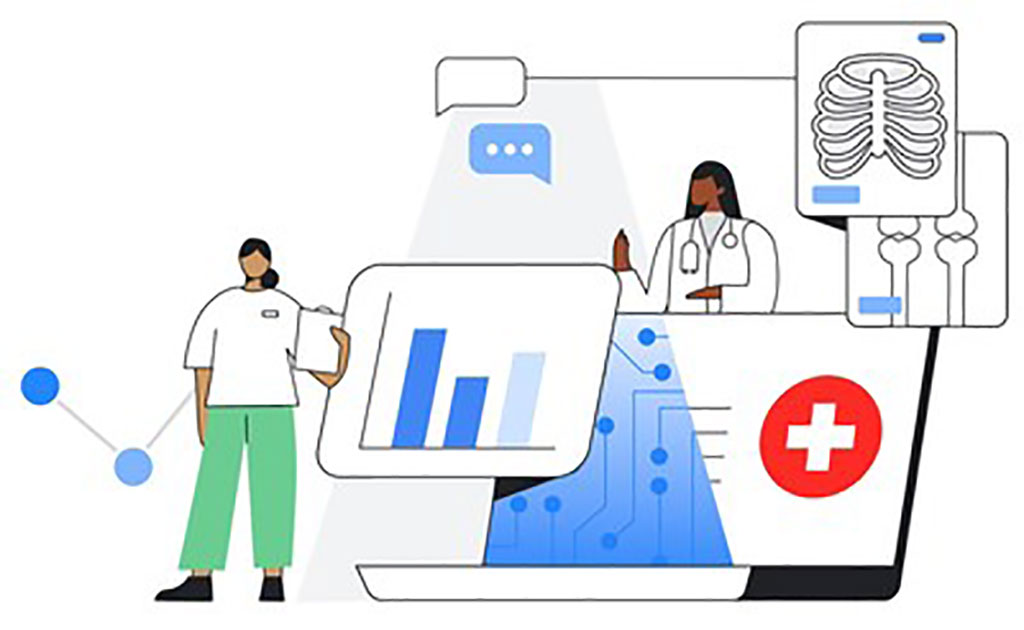
New Google Cloud Medical Imaging Suite Makes Imaging Healthcare Data More Accessible
Medical imaging is a critical tool used to diagnose patients, and there are billions of medical images scanned globally each year. Imaging data accounts for about 90% of all healthcare data1 and, until... Read more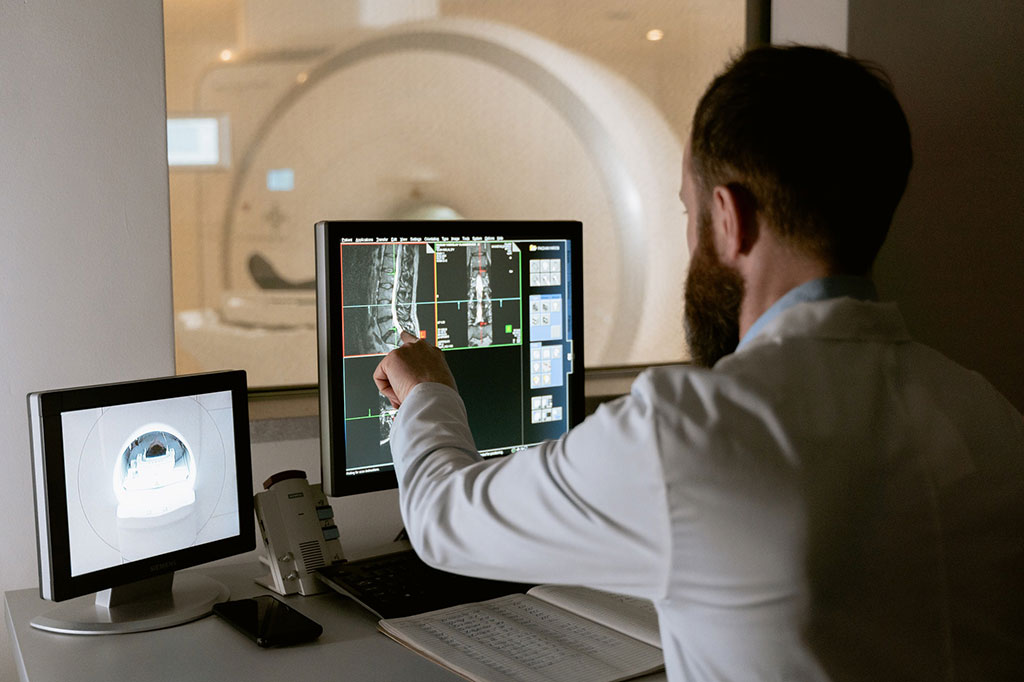
Global AI in Medical Diagnostics Market to Be Driven by Demand for Image Recognition in Radiology
The global artificial intelligence (AI) in medical diagnostics market is expanding with early disease detection being one of its key applications and image recognition becoming a compelling consumer proposition... Read moreIndustry News
view channel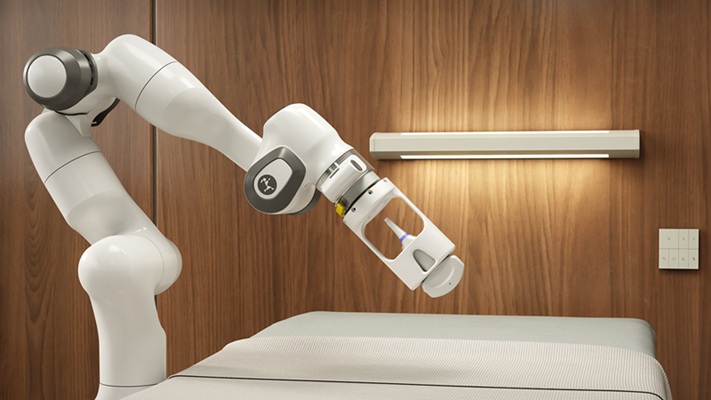
GE HealthCare and NVIDIA Collaboration to Reimagine Diagnostic Imaging
GE HealthCare (Chicago, IL, USA) has entered into a collaboration with NVIDIA (Santa Clara, CA, USA), expanding the existing relationship between the two companies to focus on pioneering innovation in... Read more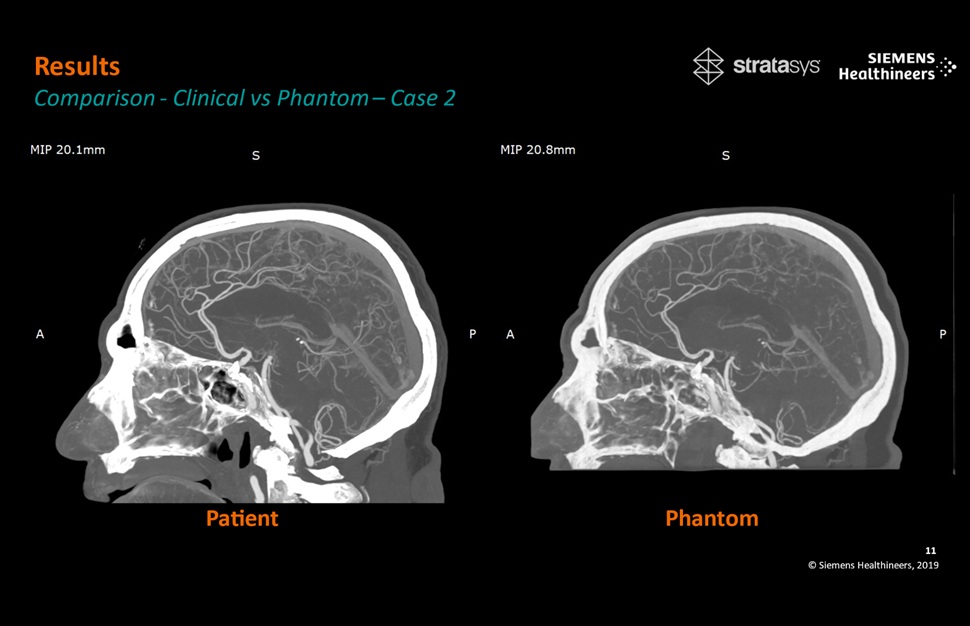
Patient-Specific 3D-Printed Phantoms Transform CT Imaging
New research has highlighted how anatomically precise, patient-specific 3D-printed phantoms are proving to be scalable, cost-effective, and efficient tools in the development of new CT scan algorithms... Read more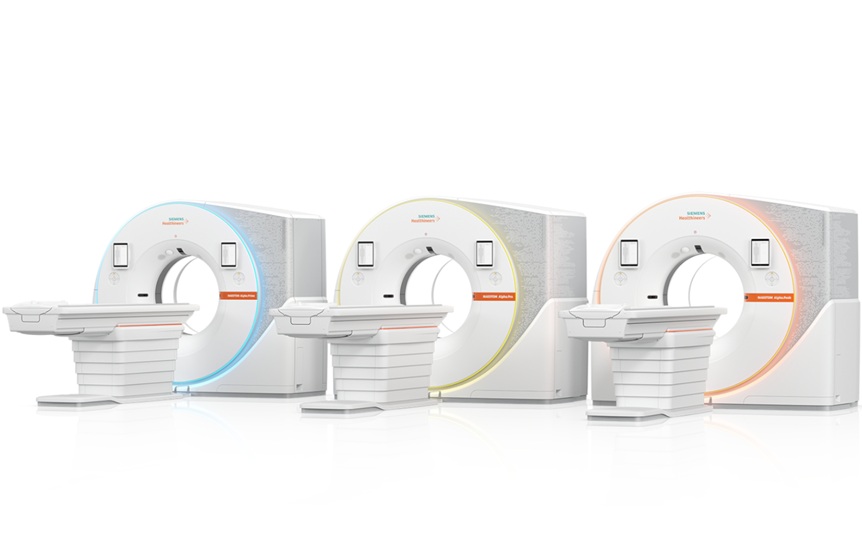
Siemens and Sectra Collaborate on Enhancing Radiology Workflows
Siemens Healthineers (Forchheim, Germany) and Sectra (Linköping, Sweden) have entered into a collaboration aimed at enhancing radiologists' diagnostic capabilities and, in turn, improving patient care... Read more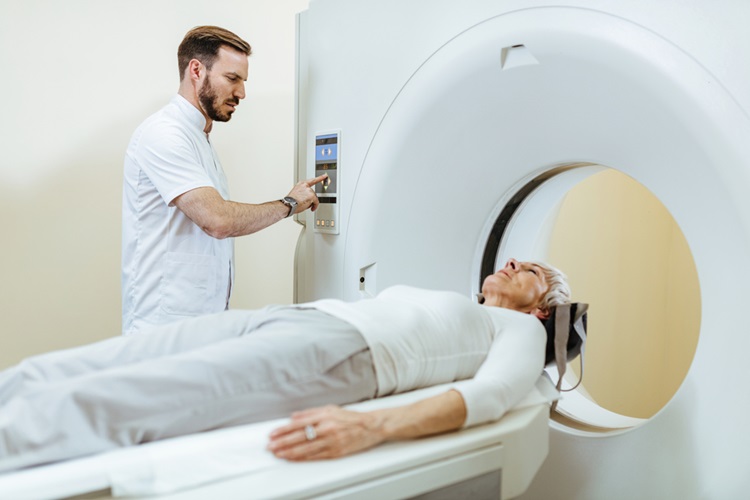