AI Model Automatically Segments MRI Images
Posted on 20 Feb 2025
Magnetic resonance imaging (MRI) plays a crucial role in providing detailed images of the human body, making it essential for diagnosing a wide range of medical conditions, from neurological disorders to musculoskeletal injuries. To interpret MRI images in depth, various anatomical structures such as organs, muscles, and bones are outlined or marked, a process known as segmentation. Traditionally, MRI images have been segmented manually, which is time-consuming, requires significant effort from radiologists, and is subject to variability between different readers. Automated systems offer the potential to reduce the radiologist's workload, minimize human errors, and deliver more consistent, reproducible results. Researchers have now developed and tested a robust artificial intelligence (AI) model capable of automatically segmenting major anatomical structures in MRI images, regardless of the sequence. In a study published in the journal Radiology, the AI model outperformed other publicly available tools.
Researchers at University Hospital Basel (Basel, Switzerland) developed an open-source automated segmentation tool called TotalSegmentator MRI, built on nnU-Net, a self-configuring framework that has set new benchmarks in medical image segmentation. This tool can adapt to new datasets with minimal user intervention, automatically adjusting its architecture, preprocessing, and training strategies to optimize performance. A similar model for CT (TotalSegmentator CT) is already in use by over 300,000 users worldwide, processing more than 100,000 CT images daily. In their retrospective study, the researchers trained TotalSegmentator MRI to provide sequence-independent segmentation of major anatomical structures using a randomly sampled dataset of 616 MRI and 527 CT exams.
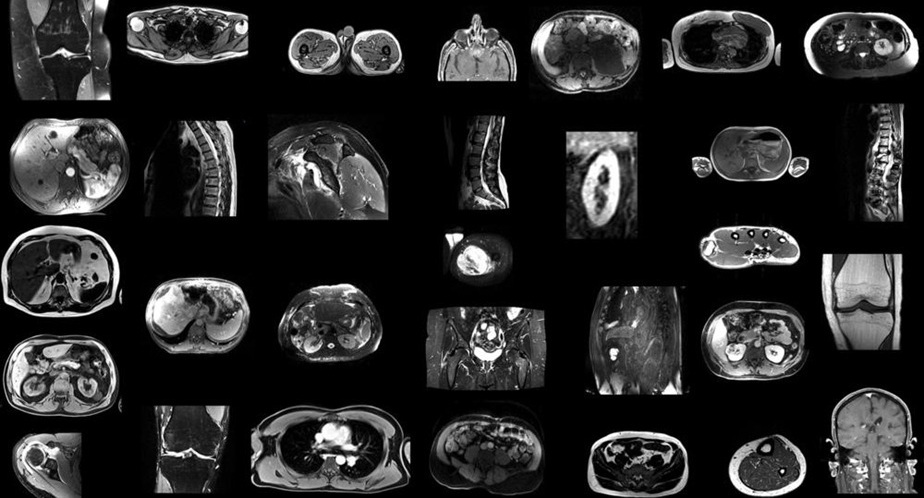
The training set included segmentation data for 80 anatomical structures, which are commonly used for tasks such as measuring volume, characterizing diseases, planning surgeries, and conducting opportunistic screenings. To assess the model’s performance, Dice scores—metrics that measure the similarity between two sets of data—were calculated by comparing the predicted segmentations to the reference standards set by radiologists. The model performed well across all 80 structures, achieving a Dice score of 0.839 on an internal MRI test set. It significantly outperformed two publicly available segmentation models, with scores of 0.862, 0.838, and 0.560, respectively, and matched the performance of TotalSegmentator CT. Beyond research and AI product development, the researchers believe the model has the potential to be used clinically for treatment planning, monitoring disease progression, and conducting opportunistic screenings.
“We used a lot more data and segmented many more organs, bones and muscles than has been previously done. Our model also works across different MRI scanners and image acquisition settings,” said Jakob Wasserthal, Ph.D., Radiology Department research scientist at University Hospital Basel. “To our knowledge, our model is the only one that can automatically segment the highest number of structures on MRIs of any sequence. It’s a tool that helps improve radiologists’ work, makes measurements more precise and enables other measurements to be done that would have taken too much time to do manually.”