New AI System Can Diagnose and Classify Intracranial Hemorrhage
By MedImaging International staff writers Posted on 23 Jan 2019 |
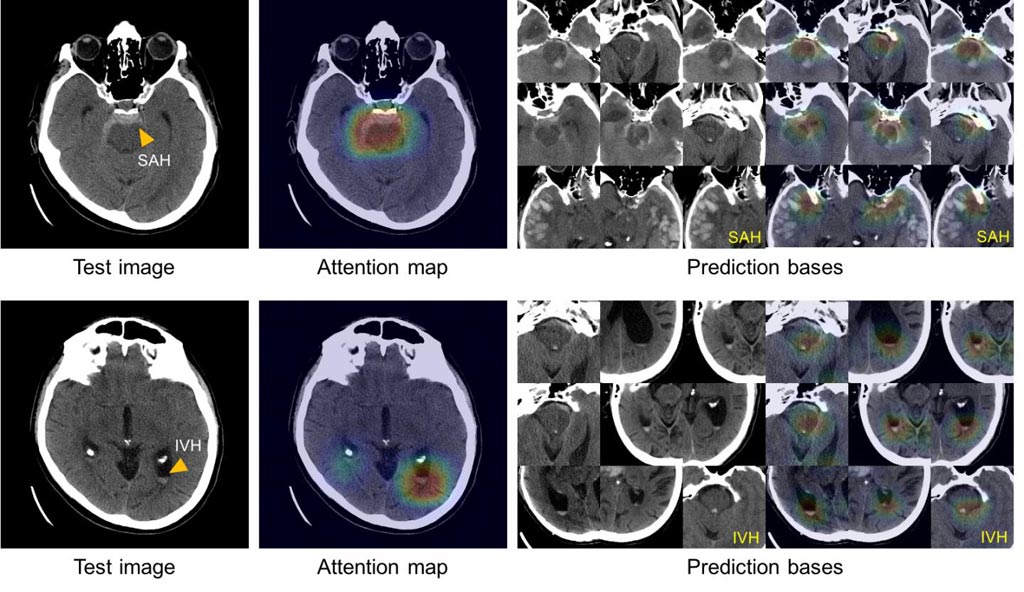
Image: These images show the system’s ability to explain its diagnosis of subarachnoid (left above) and intraventricular (left below) hemorrhage by displaying images with similar appearances (right) from an atlas of images used to train the system (Photo courtesy of Hyunkwang Lee, Harvard School of Engineering and Applied Sciences, and Sehyo Yune, MD, Massachusetts General Hospital Department of Radiology).
Researchers from the Massachusetts General Hospital (Boston, MA, USA) have developed an artificial intelligence (AI) system that can quickly diagnose and classify brain hemorrhages, as well as provide the basis of its decisions from relatively small image datasets. The system could help hospital emergency departments in evaluating patients with symptoms of a potentially life-threatening stroke, allowing rapid application of the correct treatment.
The researchers trained the system with 904 head CT scans, each consisting of around 40 individual images, that were labeled by neuroradiologists as to whether they depicted one of five hemorrhage subtypes, based on the location within the brain, or no hemorrhage. The team improved the accuracy of the deep-learning system by building in steps mimicking the way radiologists analyze images. These included adjusting factors such as contrast and brightness to reveal subtle differences not immediately apparent and scrolling through adjacent CT scan slices to determine whether or not something that appears on a single image reflects a real problem or is a meaningless artifact.
After the model system was created, the investigators tested it on two separate sets of CT scans – a retrospective set taken before the system was developed, consisting of 100 scans with and 100 without intracranial hemorrhage, and a prospective set of 79 scans with and 117 without hemorrhage, taken after the model was created. In its analysis of the retrospective set, the model system was as accurate in detecting and classifying intracranial hemorrhages as the radiologists that had reviewed the scans had been. In its analysis of the prospective set, the system proved to be even better than non-expert human readers.
To solve the “black box” problem, or the inability of systems to explain how they arrived at a decision, the team had the system review and save the images from the training dataset that most clearly represented the classic features of each of the five hemorrhage subtypes. Using this atlas of distinguishing features, the system is able to display a group of images similar to those of the CT scan being analyzed in order to explain the basis of its decisions.
“Rapid recognition of intracranial hemorrhage, leading to prompt appropriate treatment of patients with acute stroke symptoms, can prevent or mitigate major disability or death,” said co-author Michael Lev, MD, MGH Radiology. “Many facilities do not have access to specially trained neuroradiologists – especially at night or over weekends – which can require non-expert providers to determine whether or not a hemorrhage is the cause of a patient’s symptoms. The availability of a reliable, ‘virtual second opinion’ – trained by neuroradiologists – could make those providers more efficient and confident and help ensure that patients get the right treatment.”
“In addition to providing that much needed virtual second opinion, this system also could be deployed directly onto scanners, alerting the care team to the presence of a hemorrhage and triggering appropriate further testing before the patient is even off the scanner,” added co-author Shahein Tajmir, MD, MGH Radiology. “The next step will be to deploy the system into clinical areas and further validate its performance with many more cases. We are currently building a platform to allow for the widespread application of such tools throughout the department. Once we have this running in the clinical setting, we can evaluate its impact on turnaround time, clinical accuracy and the time to diagnosis.”
Related Links:
Massachusetts General Hospital
The researchers trained the system with 904 head CT scans, each consisting of around 40 individual images, that were labeled by neuroradiologists as to whether they depicted one of five hemorrhage subtypes, based on the location within the brain, or no hemorrhage. The team improved the accuracy of the deep-learning system by building in steps mimicking the way radiologists analyze images. These included adjusting factors such as contrast and brightness to reveal subtle differences not immediately apparent and scrolling through adjacent CT scan slices to determine whether or not something that appears on a single image reflects a real problem or is a meaningless artifact.
After the model system was created, the investigators tested it on two separate sets of CT scans – a retrospective set taken before the system was developed, consisting of 100 scans with and 100 without intracranial hemorrhage, and a prospective set of 79 scans with and 117 without hemorrhage, taken after the model was created. In its analysis of the retrospective set, the model system was as accurate in detecting and classifying intracranial hemorrhages as the radiologists that had reviewed the scans had been. In its analysis of the prospective set, the system proved to be even better than non-expert human readers.
To solve the “black box” problem, or the inability of systems to explain how they arrived at a decision, the team had the system review and save the images from the training dataset that most clearly represented the classic features of each of the five hemorrhage subtypes. Using this atlas of distinguishing features, the system is able to display a group of images similar to those of the CT scan being analyzed in order to explain the basis of its decisions.
“Rapid recognition of intracranial hemorrhage, leading to prompt appropriate treatment of patients with acute stroke symptoms, can prevent or mitigate major disability or death,” said co-author Michael Lev, MD, MGH Radiology. “Many facilities do not have access to specially trained neuroradiologists – especially at night or over weekends – which can require non-expert providers to determine whether or not a hemorrhage is the cause of a patient’s symptoms. The availability of a reliable, ‘virtual second opinion’ – trained by neuroradiologists – could make those providers more efficient and confident and help ensure that patients get the right treatment.”
“In addition to providing that much needed virtual second opinion, this system also could be deployed directly onto scanners, alerting the care team to the presence of a hemorrhage and triggering appropriate further testing before the patient is even off the scanner,” added co-author Shahein Tajmir, MD, MGH Radiology. “The next step will be to deploy the system into clinical areas and further validate its performance with many more cases. We are currently building a platform to allow for the widespread application of such tools throughout the department. Once we have this running in the clinical setting, we can evaluate its impact on turnaround time, clinical accuracy and the time to diagnosis.”
Related Links:
Massachusetts General Hospital
Latest Industry News News
- GE HealthCare and NVIDIA Collaboration to Reimagine Diagnostic Imaging
- Patient-Specific 3D-Printed Phantoms Transform CT Imaging
- Siemens and Sectra Collaborate on Enhancing Radiology Workflows
- Bracco Diagnostics and ColoWatch Partner to Expand Availability CRC Screening Tests Using Virtual Colonoscopy
- Mindray Partners with TeleRay to Streamline Ultrasound Delivery
- Philips and Medtronic Partner on Stroke Care
- Siemens and Medtronic Enter into Global Partnership for Advancing Spine Care Imaging Technologies
- RSNA 2024 Technical Exhibits to Showcase Latest Advances in Radiology
- Bracco Collaborates with Arrayus on Microbubble-Assisted Focused Ultrasound Therapy for Pancreatic Cancer
- Innovative Collaboration to Enhance Ischemic Stroke Detection and Elevate Standards in Diagnostic Imaging
- RSNA 2024 Registration Opens
- Microsoft collaborates with Leading Academic Medical Systems to Advance AI in Medical Imaging
- GE HealthCare Acquires Intelligent Ultrasound Group’s Clinical Artificial Intelligence Business
- Bayer and Rad AI Collaborate on Expanding Use of Cutting Edge AI Radiology Operational Solutions
- Polish Med-Tech Company BrainScan to Expand Extensively into Foreign Markets
- Hologic Acquires UK-Based Breast Surgical Guidance Company Endomagnetics Ltd.
Channels
Radiography
view channel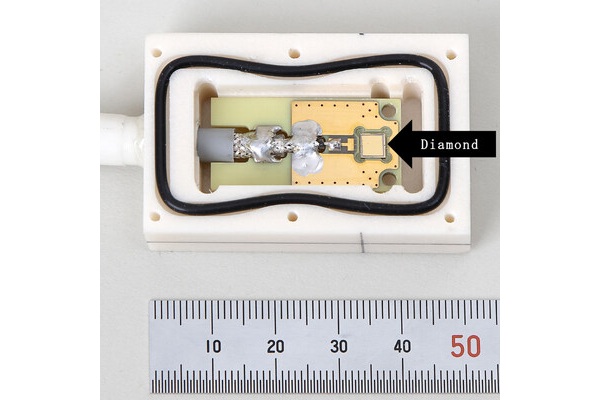
World's Largest Class Single Crystal Diamond Radiation Detector Opens New Possibilities for Diagnostic Imaging
Diamonds possess ideal physical properties for radiation detection, such as exceptional thermal and chemical stability along with a quick response time. Made of carbon with an atomic number of six, diamonds... Read more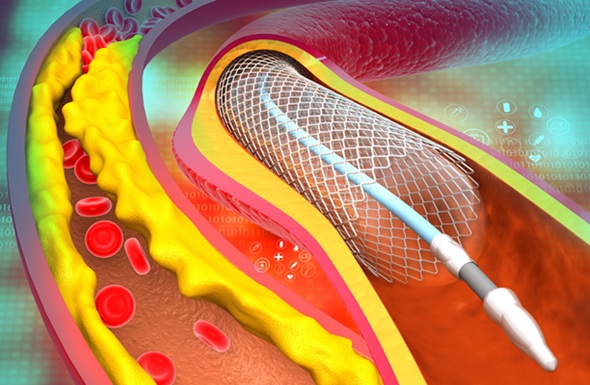
AI-Powered Imaging Technique Shows Promise in Evaluating Patients for PCI
Percutaneous coronary intervention (PCI), also known as coronary angioplasty, is a minimally invasive procedure where small metal tubes called stents are inserted into partially blocked coronary arteries... Read moreMRI
view channel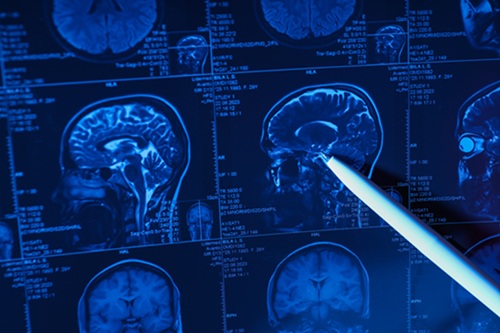
AI Tool Predicts Relapse of Pediatric Brain Cancer from Brain MRI Scans
Many pediatric gliomas are treatable with surgery alone, but relapses can be catastrophic. Predicting which patients are at risk for recurrence remains challenging, leading to frequent follow-ups with... Read more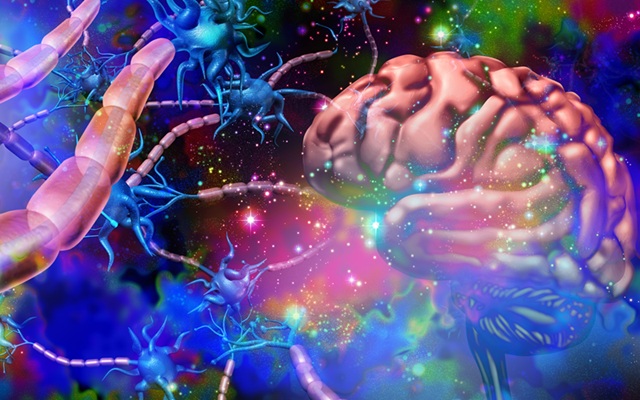
AI Tool Tracks Effectiveness of Multiple Sclerosis Treatments Using Brain MRI Scans
Multiple sclerosis (MS) is a condition in which the immune system attacks the brain and spinal cord, leading to impairments in movement, sensation, and cognition. Magnetic Resonance Imaging (MRI) markers... Read more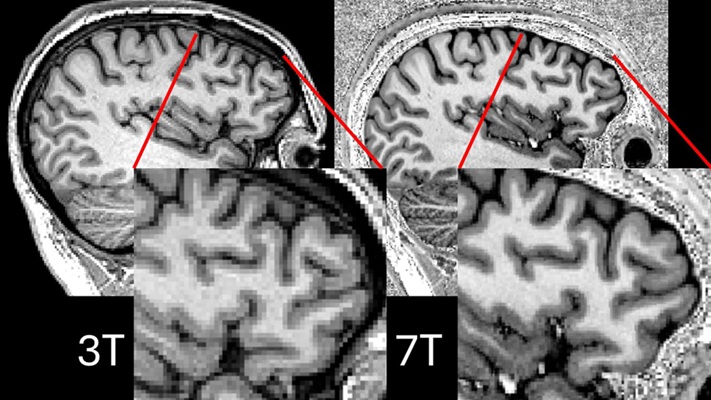
Ultra-Powerful MRI Scans Enable Life-Changing Surgery in Treatment-Resistant Epileptic Patients
Approximately 360,000 individuals in the UK suffer from focal epilepsy, a condition in which seizures spread from one part of the brain. Around a third of these patients experience persistent seizures... Read moreUltrasound
view channel.jpeg)
AI-Powered Lung Ultrasound Outperforms Human Experts in Tuberculosis Diagnosis
Despite global declines in tuberculosis (TB) rates in previous years, the incidence of TB rose by 4.6% from 2020 to 2023. Early screening and rapid diagnosis are essential elements of the World Health... Read more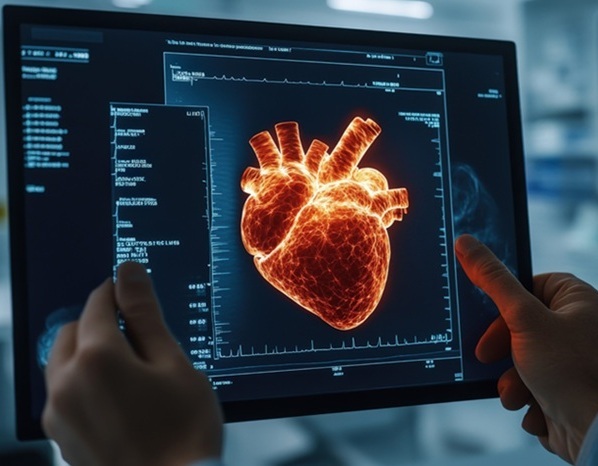
AI Identifies Heart Valve Disease from Common Imaging Test
Tricuspid regurgitation is a condition where the heart's tricuspid valve does not close completely during contraction, leading to backward blood flow, which can result in heart failure. A new artificial... Read moreNuclear Medicine
view channel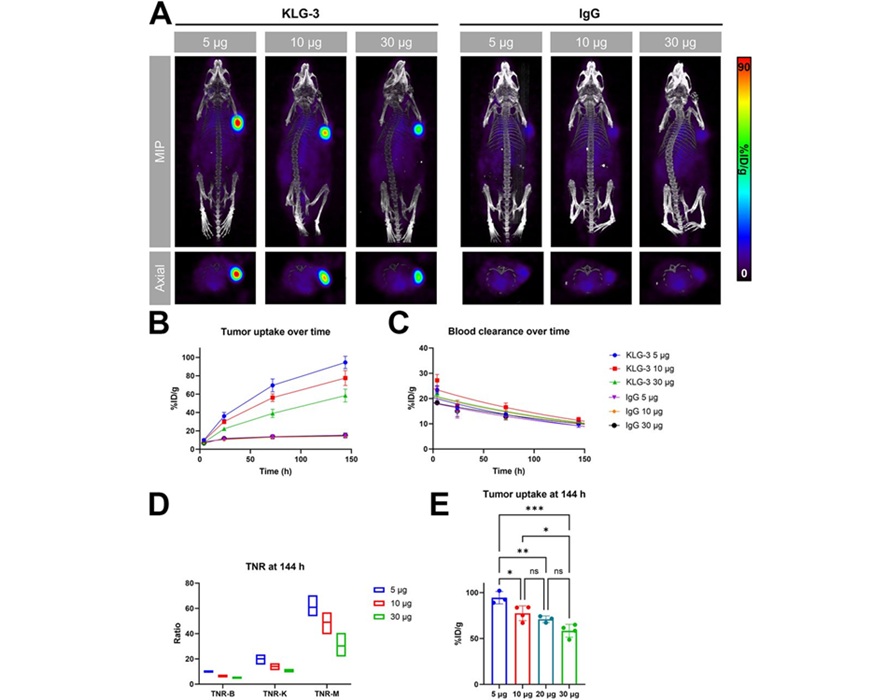
Novel Radiolabeled Antibody Improves Diagnosis and Treatment of Solid Tumors
Interleukin-13 receptor α-2 (IL13Rα2) is a cell surface receptor commonly found in solid tumors such as glioblastoma, melanoma, and breast cancer. It is minimally expressed in normal tissues, making it... Read more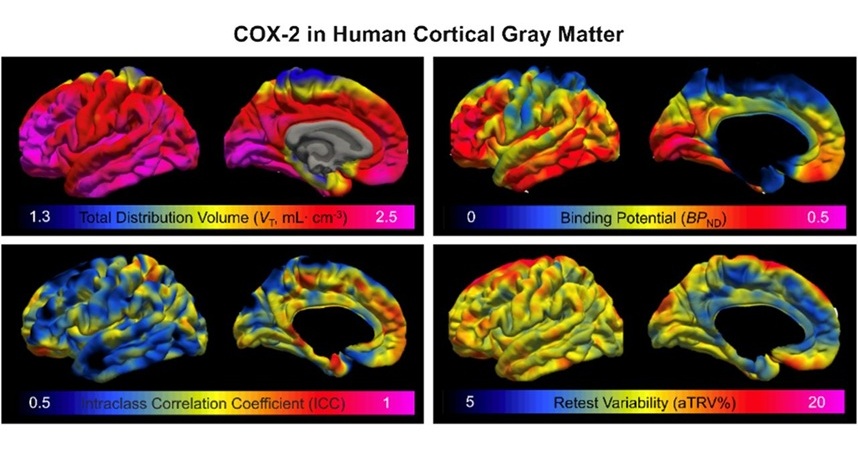
Novel PET Imaging Approach Offers Never-Before-Seen View of Neuroinflammation
COX-2, an enzyme that plays a key role in brain inflammation, can be significantly upregulated by inflammatory stimuli and neuroexcitation. Researchers suggest that COX-2 density in the brain could serve... Read moreGeneral/Advanced Imaging
view channel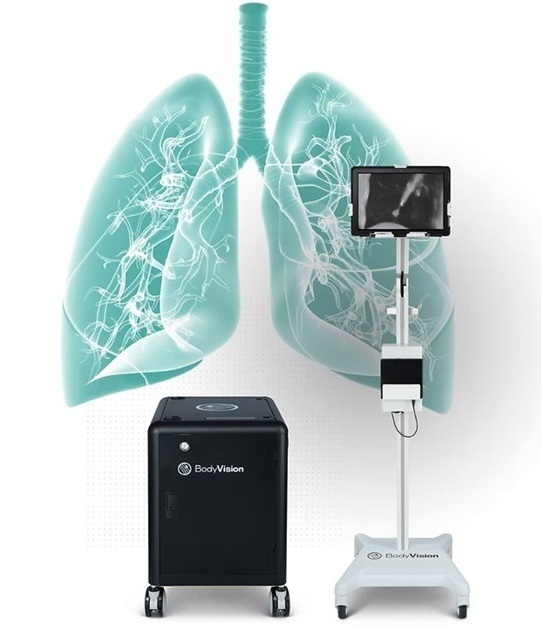
AI-Powered Imaging System Improves Lung Cancer Diagnosis
Given the need to detect lung cancer at earlier stages, there is an increasing need for a definitive diagnostic pathway for patients with suspicious pulmonary nodules. However, obtaining tissue samples... Read more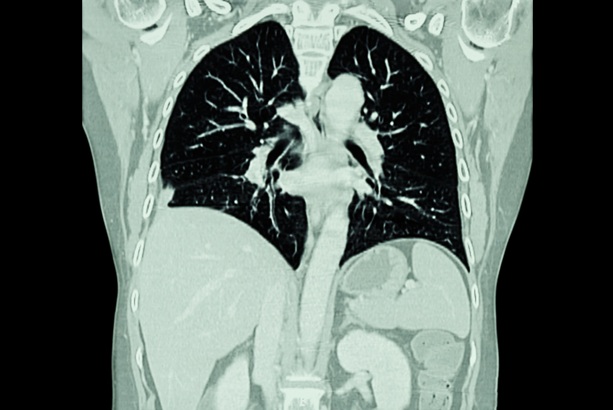
AI Model Significantly Enhances Low-Dose CT Capabilities
Lung cancer remains one of the most challenging diseases, making early diagnosis vital for effective treatment. Fortunately, advancements in artificial intelligence (AI) are revolutionizing lung cancer... Read moreImaging IT
view channel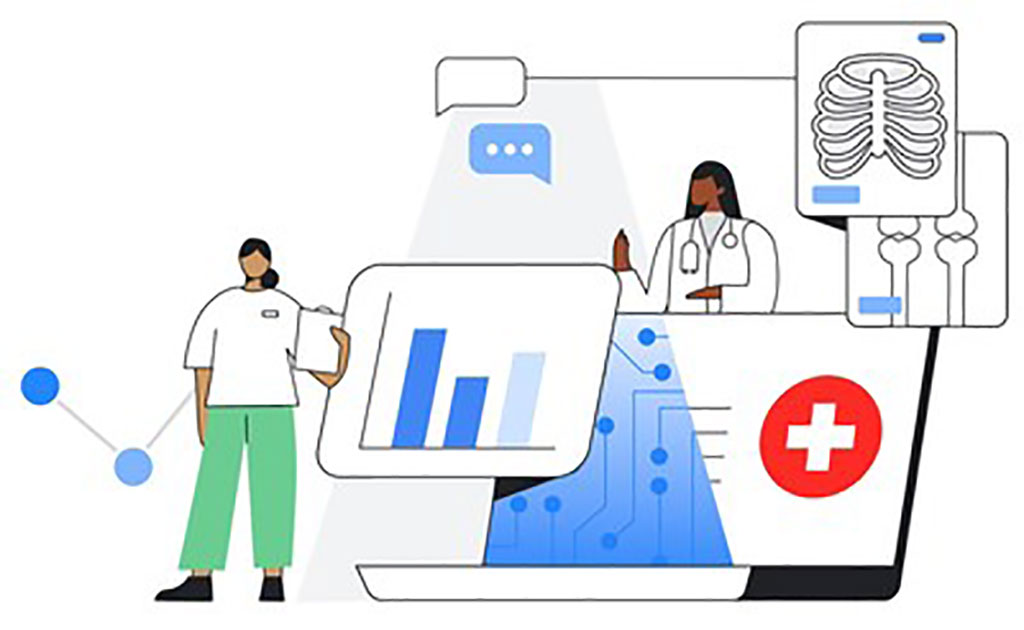
New Google Cloud Medical Imaging Suite Makes Imaging Healthcare Data More Accessible
Medical imaging is a critical tool used to diagnose patients, and there are billions of medical images scanned globally each year. Imaging data accounts for about 90% of all healthcare data1 and, until... Read more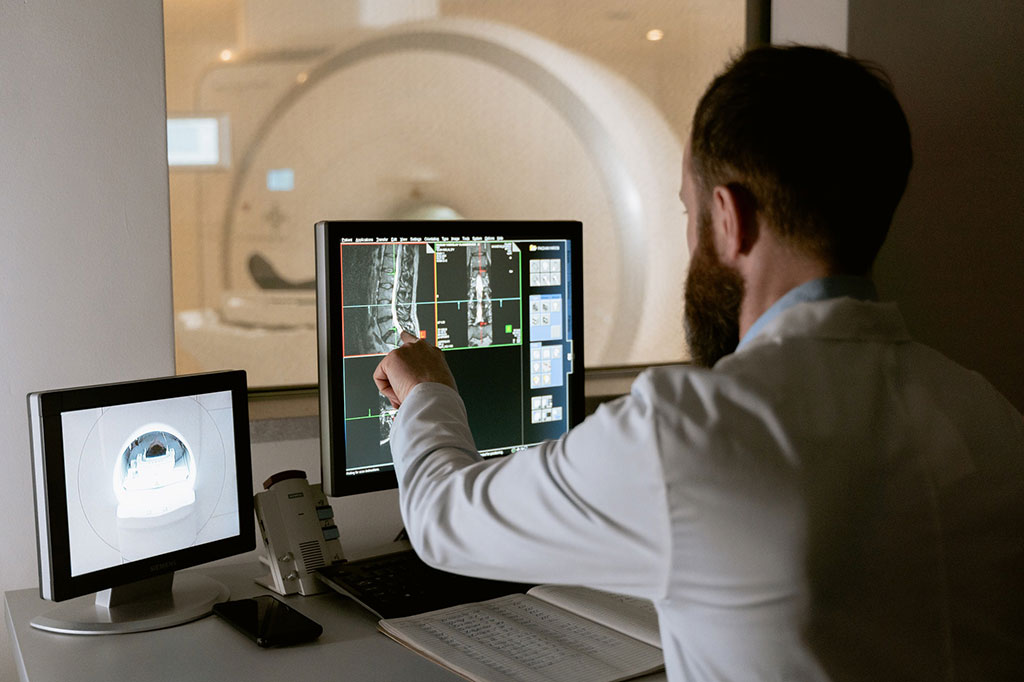