Largest-Ever Open-Source Dataset Released to Speed Up MRIs Using AI
By MedImaging International staff writers Posted on 18 Dec 2018 |
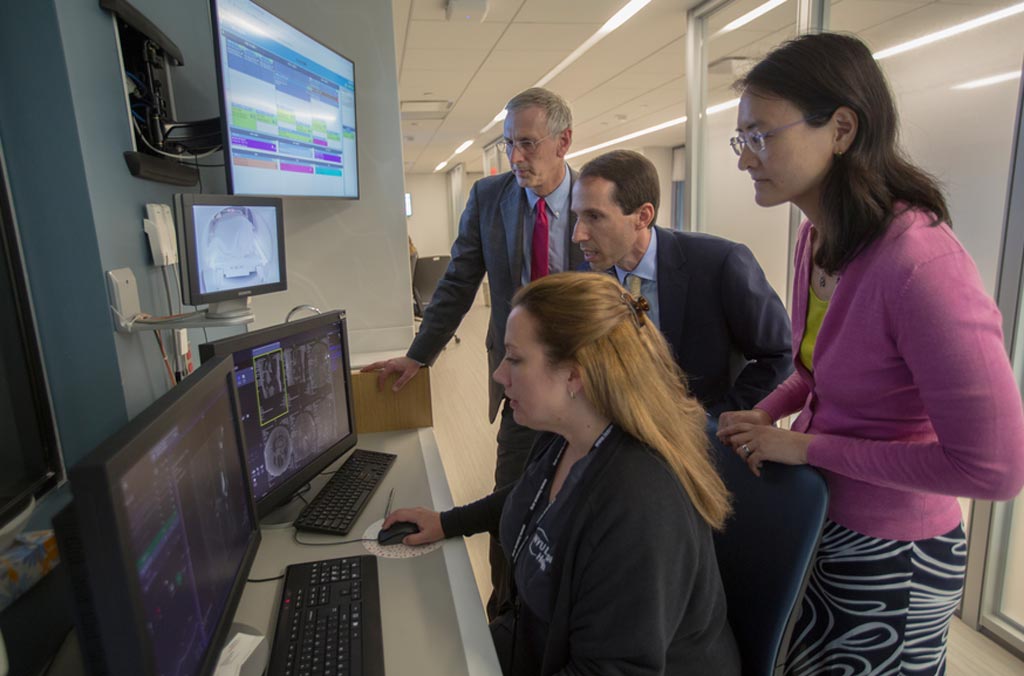
Image: NYU School of Medicine Department of Radiology chair, Michael Recht, MD; Daniel Sodickson, MD, PhD, vice chair for research and director of the Center for Advanced Imaging Innovation and Research; and Yvonne Lui, MD, director of artificial intelligence, watch an MRI exam take place with at NYU Langone Health in New York in August 2018.
The NYU School of Medicine’s (New York, NY, USA) Department of Radiology is releasing the first large-scale MRI dataset of its kind as part of fastMRI, a collaborative effort with Facebook AI Research (New York, NY, USA) to speed up MRI scans with artificial intelligence (AI).
The collaboration is aimed at sharing open source tools and spurring the development of AI systems to make MRI scans 10 times faster. The collaboration will promote research reproducibility, provide consistent evaluation methods, and empower the larger community of AI and medical imaging scientists.
Using AI, researchers believe it will be possible to capture less data, and therefore image faster, while preserving or even enhancing the rich information contained in MR images. Leaders of the study say that, if successful, fastMRI could benefit a wide range of people who may have difficulty tolerating lengthy scans, including young children, elderly patients, and claustrophobic individual. It could also decrease the need for anesthesia or sedation. Additionally, the project could expand access to this key diagnostic tool, particularly in areas where there is a shortage of MRI scanners and patients face long wait-times for their scans.
The initial dataset release includes more than 1.5 million anonymous MR images of the knee, drawn from 10,000 scans, in addition to raw measurement data from nearly 1,600 scans. While other sets of radiological images have been released previously, this dataset represents the largest public release of raw MRI data to date. The first phase of the project will involve data from knee MRI scans, but future releases will include data from liver and brain scans. The joint team will also provide a suite of tools, including baseline metrics to compare results, and a leaderboard to keep track of progress as part of an organized challenge to be announced in the near future.
"fastMRI not only could have an important impact in the medical field, it's also an interesting research challenge that will help to advance the field of AI," said Larry Zitnick, Research Manager, Facebook AI Research. "To be medically useful, our AI-reconstructed images need to be more than just good-looking, they must also be accurate representations of the ground-truth, even though they're created from significantly less data. The dataset NYU Langone is releasing and the baseline models we've open-sourced will enable other researchers to join us in working on this challenging problem, and we believe this open approach will bring about positive results more quickly."
“This collaboration focuses on applying the strengths of machine learning to reconstruct high-value images in new ways. Rather than using existing images to train AI algorithms, we will radically change the way medical images are acquired in the first place,” said Daniel Sodickson, MD, PhD, professor of radiology and neuroscience and physiology and director of CAIR. “Our aim is not merely enhanced data mining with AI, but rather creating new capabilities for medical visualization, to benefit human health.”
Related Links:
NYU School of Medicine
Facebook AI Research
The collaboration is aimed at sharing open source tools and spurring the development of AI systems to make MRI scans 10 times faster. The collaboration will promote research reproducibility, provide consistent evaluation methods, and empower the larger community of AI and medical imaging scientists.
Using AI, researchers believe it will be possible to capture less data, and therefore image faster, while preserving or even enhancing the rich information contained in MR images. Leaders of the study say that, if successful, fastMRI could benefit a wide range of people who may have difficulty tolerating lengthy scans, including young children, elderly patients, and claustrophobic individual. It could also decrease the need for anesthesia or sedation. Additionally, the project could expand access to this key diagnostic tool, particularly in areas where there is a shortage of MRI scanners and patients face long wait-times for their scans.
The initial dataset release includes more than 1.5 million anonymous MR images of the knee, drawn from 10,000 scans, in addition to raw measurement data from nearly 1,600 scans. While other sets of radiological images have been released previously, this dataset represents the largest public release of raw MRI data to date. The first phase of the project will involve data from knee MRI scans, but future releases will include data from liver and brain scans. The joint team will also provide a suite of tools, including baseline metrics to compare results, and a leaderboard to keep track of progress as part of an organized challenge to be announced in the near future.
"fastMRI not only could have an important impact in the medical field, it's also an interesting research challenge that will help to advance the field of AI," said Larry Zitnick, Research Manager, Facebook AI Research. "To be medically useful, our AI-reconstructed images need to be more than just good-looking, they must also be accurate representations of the ground-truth, even though they're created from significantly less data. The dataset NYU Langone is releasing and the baseline models we've open-sourced will enable other researchers to join us in working on this challenging problem, and we believe this open approach will bring about positive results more quickly."
“This collaboration focuses on applying the strengths of machine learning to reconstruct high-value images in new ways. Rather than using existing images to train AI algorithms, we will radically change the way medical images are acquired in the first place,” said Daniel Sodickson, MD, PhD, professor of radiology and neuroscience and physiology and director of CAIR. “Our aim is not merely enhanced data mining with AI, but rather creating new capabilities for medical visualization, to benefit human health.”
Related Links:
NYU School of Medicine
Facebook AI Research
Latest Industry News News
- GE HealthCare and NVIDIA Collaboration to Reimagine Diagnostic Imaging
- Patient-Specific 3D-Printed Phantoms Transform CT Imaging
- Siemens and Sectra Collaborate on Enhancing Radiology Workflows
- Bracco Diagnostics and ColoWatch Partner to Expand Availability CRC Screening Tests Using Virtual Colonoscopy
- Mindray Partners with TeleRay to Streamline Ultrasound Delivery
- Philips and Medtronic Partner on Stroke Care
- Siemens and Medtronic Enter into Global Partnership for Advancing Spine Care Imaging Technologies
- RSNA 2024 Technical Exhibits to Showcase Latest Advances in Radiology
- Bracco Collaborates with Arrayus on Microbubble-Assisted Focused Ultrasound Therapy for Pancreatic Cancer
- Innovative Collaboration to Enhance Ischemic Stroke Detection and Elevate Standards in Diagnostic Imaging
- RSNA 2024 Registration Opens
- Microsoft collaborates with Leading Academic Medical Systems to Advance AI in Medical Imaging
- GE HealthCare Acquires Intelligent Ultrasound Group’s Clinical Artificial Intelligence Business
- Bayer and Rad AI Collaborate on Expanding Use of Cutting Edge AI Radiology Operational Solutions
- Polish Med-Tech Company BrainScan to Expand Extensively into Foreign Markets
- Hologic Acquires UK-Based Breast Surgical Guidance Company Endomagnetics Ltd.
Channels
Radiography
view channel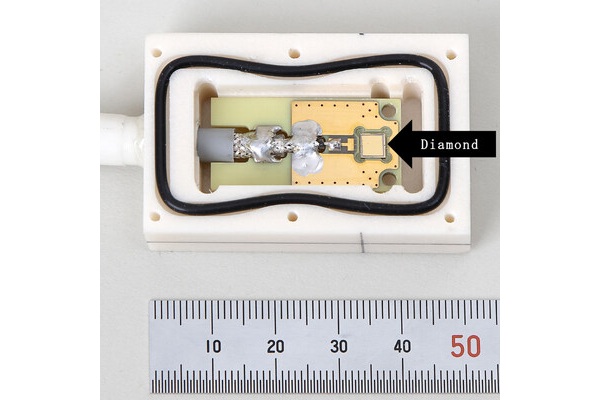
World's Largest Class Single Crystal Diamond Radiation Detector Opens New Possibilities for Diagnostic Imaging
Diamonds possess ideal physical properties for radiation detection, such as exceptional thermal and chemical stability along with a quick response time. Made of carbon with an atomic number of six, diamonds... Read more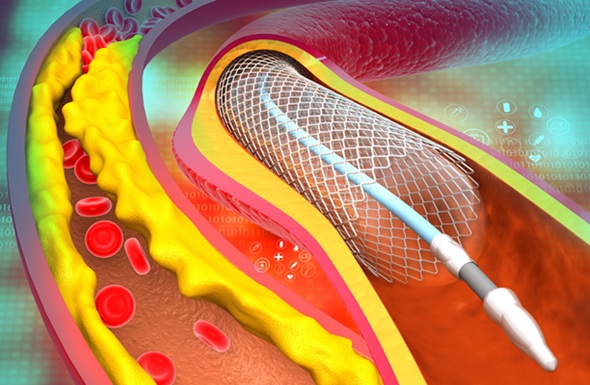
AI-Powered Imaging Technique Shows Promise in Evaluating Patients for PCI
Percutaneous coronary intervention (PCI), also known as coronary angioplasty, is a minimally invasive procedure where small metal tubes called stents are inserted into partially blocked coronary arteries... Read moreMRI
view channel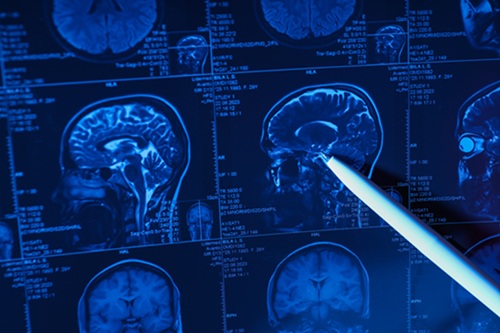
AI Tool Predicts Relapse of Pediatric Brain Cancer from Brain MRI Scans
Many pediatric gliomas are treatable with surgery alone, but relapses can be catastrophic. Predicting which patients are at risk for recurrence remains challenging, leading to frequent follow-ups with... Read more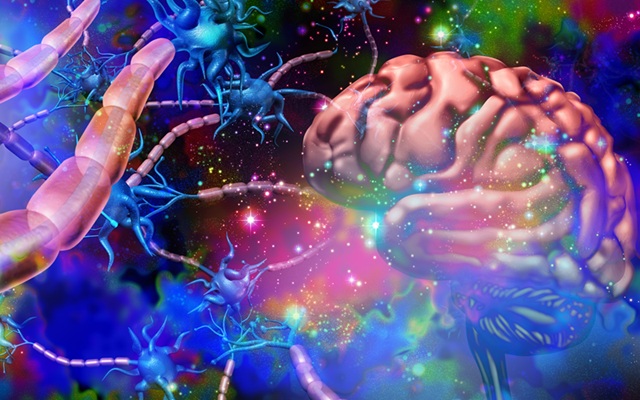
AI Tool Tracks Effectiveness of Multiple Sclerosis Treatments Using Brain MRI Scans
Multiple sclerosis (MS) is a condition in which the immune system attacks the brain and spinal cord, leading to impairments in movement, sensation, and cognition. Magnetic Resonance Imaging (MRI) markers... Read more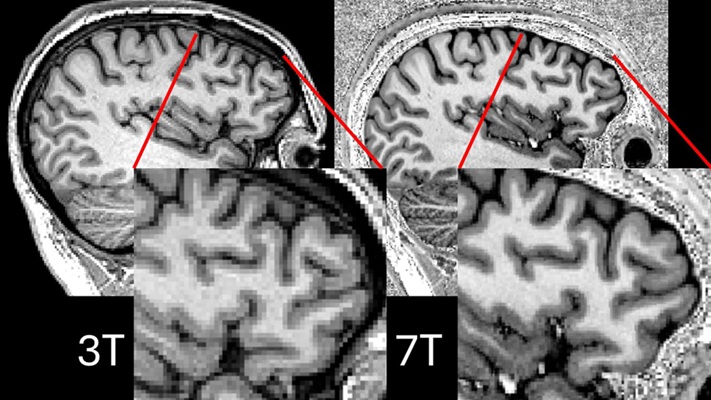
Ultra-Powerful MRI Scans Enable Life-Changing Surgery in Treatment-Resistant Epileptic Patients
Approximately 360,000 individuals in the UK suffer from focal epilepsy, a condition in which seizures spread from one part of the brain. Around a third of these patients experience persistent seizures... Read moreUltrasound
view channel.jpeg)
AI-Powered Lung Ultrasound Outperforms Human Experts in Tuberculosis Diagnosis
Despite global declines in tuberculosis (TB) rates in previous years, the incidence of TB rose by 4.6% from 2020 to 2023. Early screening and rapid diagnosis are essential elements of the World Health... Read more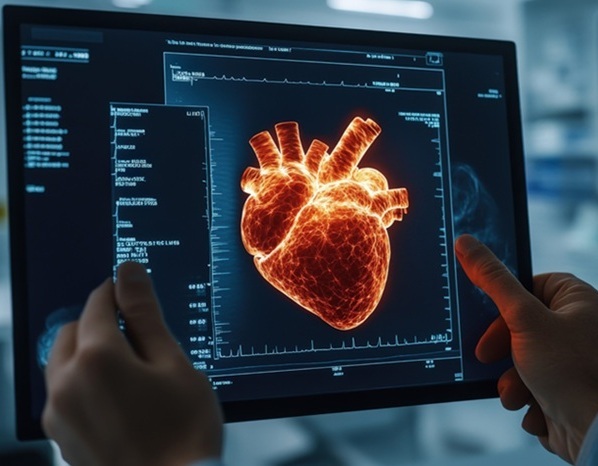
AI Identifies Heart Valve Disease from Common Imaging Test
Tricuspid regurgitation is a condition where the heart's tricuspid valve does not close completely during contraction, leading to backward blood flow, which can result in heart failure. A new artificial... Read moreNuclear Medicine
view channel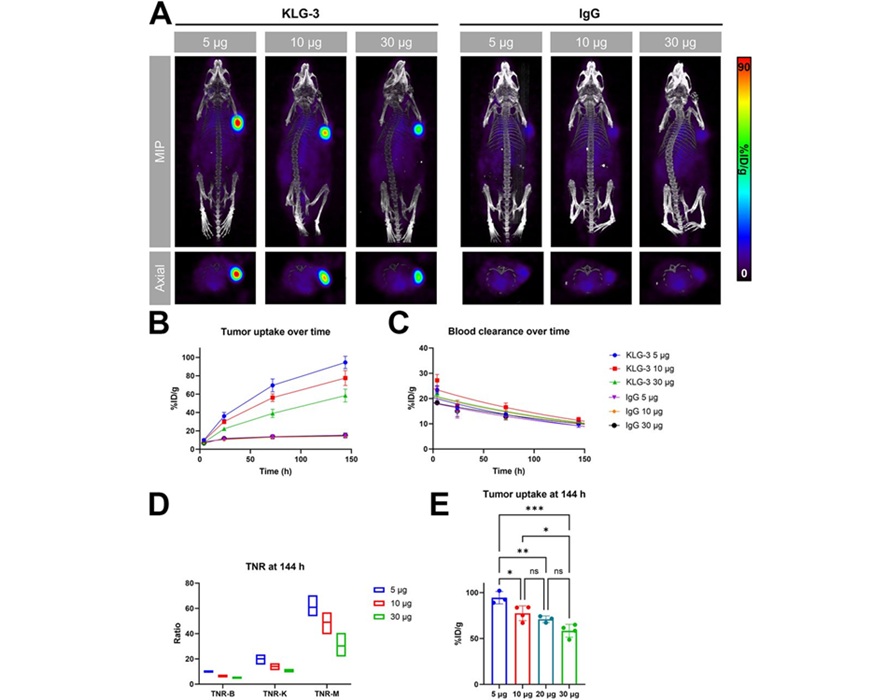
Novel Radiolabeled Antibody Improves Diagnosis and Treatment of Solid Tumors
Interleukin-13 receptor α-2 (IL13Rα2) is a cell surface receptor commonly found in solid tumors such as glioblastoma, melanoma, and breast cancer. It is minimally expressed in normal tissues, making it... Read more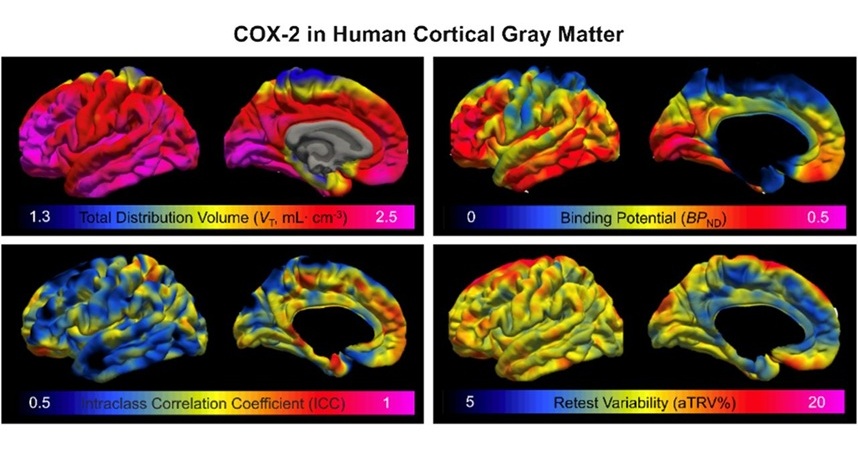
Novel PET Imaging Approach Offers Never-Before-Seen View of Neuroinflammation
COX-2, an enzyme that plays a key role in brain inflammation, can be significantly upregulated by inflammatory stimuli and neuroexcitation. Researchers suggest that COX-2 density in the brain could serve... Read moreGeneral/Advanced Imaging
view channel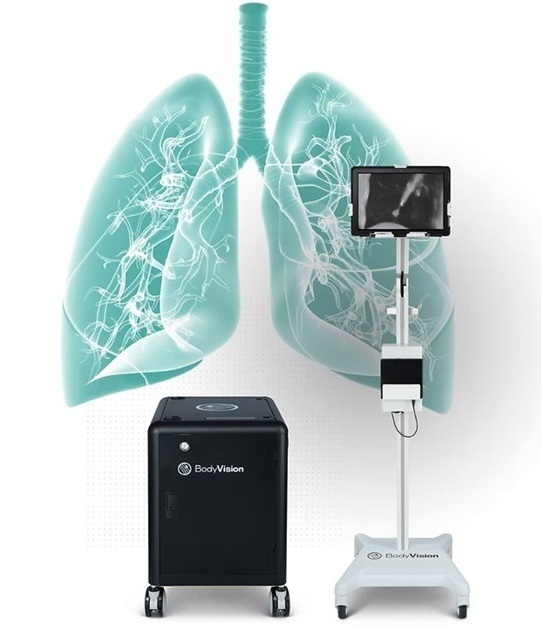
AI-Powered Imaging System Improves Lung Cancer Diagnosis
Given the need to detect lung cancer at earlier stages, there is an increasing need for a definitive diagnostic pathway for patients with suspicious pulmonary nodules. However, obtaining tissue samples... Read more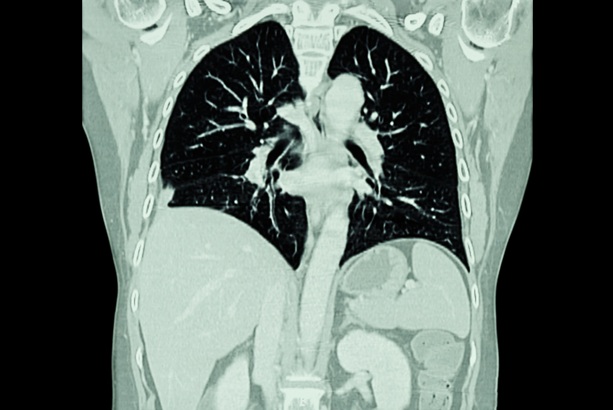
AI Model Significantly Enhances Low-Dose CT Capabilities
Lung cancer remains one of the most challenging diseases, making early diagnosis vital for effective treatment. Fortunately, advancements in artificial intelligence (AI) are revolutionizing lung cancer... Read moreImaging IT
view channel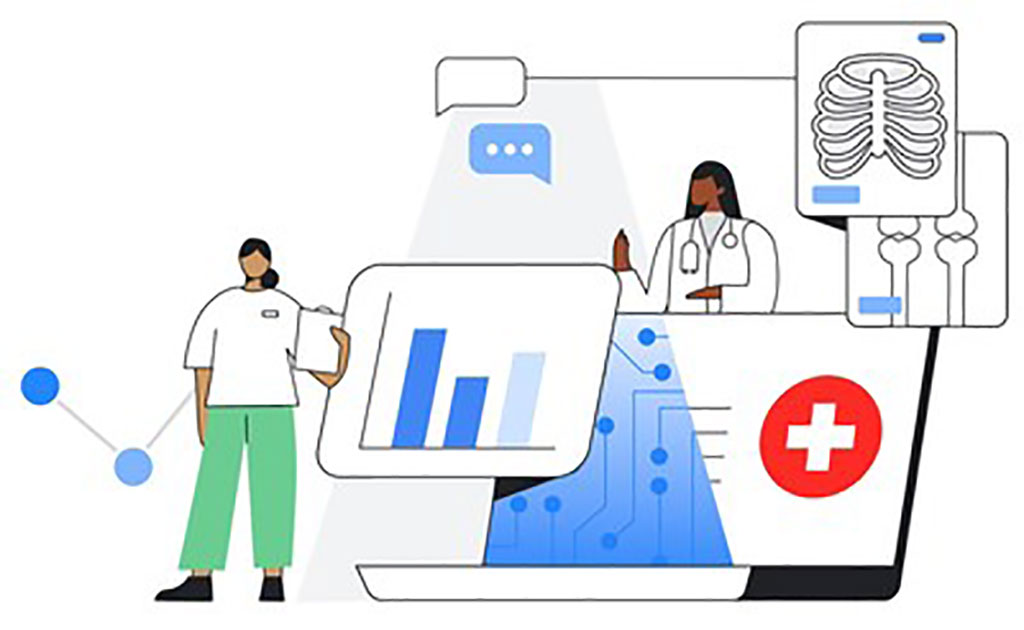
New Google Cloud Medical Imaging Suite Makes Imaging Healthcare Data More Accessible
Medical imaging is a critical tool used to diagnose patients, and there are billions of medical images scanned globally each year. Imaging data accounts for about 90% of all healthcare data1 and, until... Read more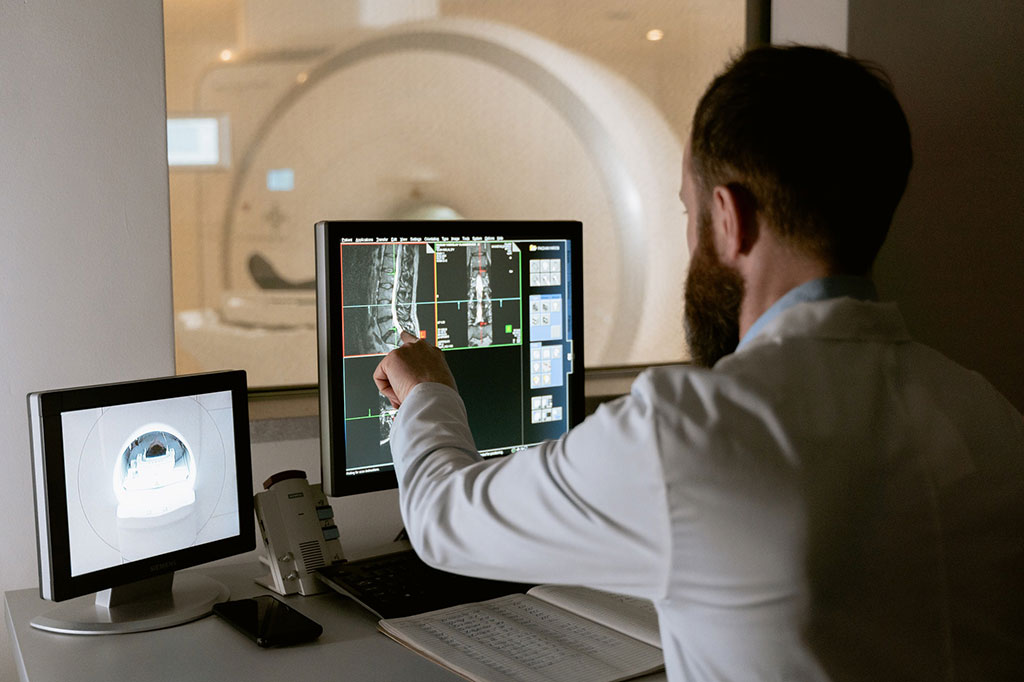