Researchers Use AI to Improve Mammogram Interpretation
By MedImaging International staff writers Posted on 04 Jul 2018 |
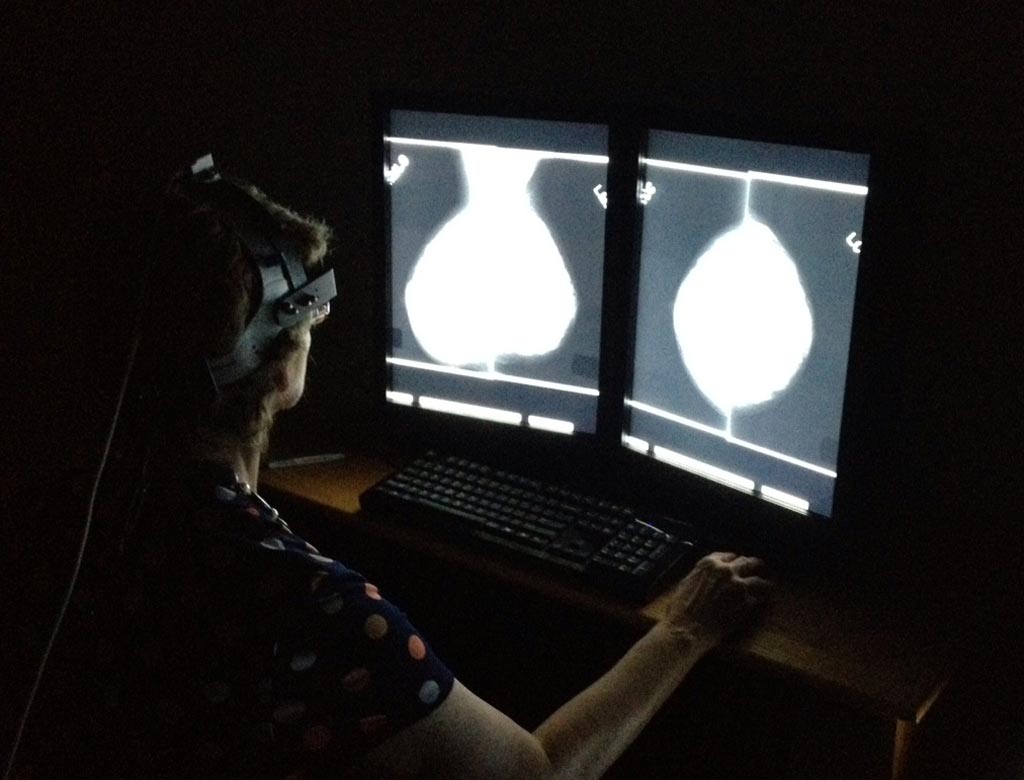
Image: Researchers used AI to improve mammogram image interpretation (Photo courtesy of the Department of Energy’s Oak Ridge National Laboratory).
A team of researchers at the Department of Energy’s Oak Ridge National Laboratory (Oak Ridge, TN, USA) successfully used artificial intelligence to improve understanding of the cognitive processes involved in image interpretation. Their work, which was published in the Journal of Medical Imaging, will help reduce errors in the analyses of diagnostic images by health professionals and has the potential to improve health outcomes for women affected by breast cancer.
Early detection of breast cancer is critical for effective treatment, which requires accurate interpretation of a patient’s mammogram. The ORNL-led team of researchers found that analyses of mammograms by radiologists were significantly influenced by context bias, or the radiologist’s previous diagnostic experiences. New radiology trainees were most susceptible to the phenomenon, although even more experienced radiologists fall victim to some degree, according to the researchers.
The researchers designed an experiment aimed at following the eye movements of radiologists at various skill levels to better understand the context bias involved in their individual interpretations of the images. The experiment followed the eye movements of three board certified radiologists and seven radiology residents as they analyzed 100 mammographic studies from the University of South Florida’s Digital Database for Screening Mammography. The 400 images, representing a mix of cancer, no cancer, and cases that mimicked cancer but were benign, were specifically selected to cover a range of cases similar to that found in a clinical setting.
The participants, who were grouped by levels of experience and had no prior knowledge of what was contained in the individual X-rays, were outfitted with a head-mounted eye-tracking device designed to record their “raw gaze data,” which characterized their overall visual behavior. The study also recorded the participants’ diagnostic decisions via the location of suspicious findings along with their characteristics according to the BI-RADS lexicon, the radiologists’ reporting scheme for mammograms. By computing a measure known as a fractal dimension on the individual participants’ scan path (map of eye movements) and performing a series of statistical calculations, the researchers were able to discern how the eye movements of the participants differed from mammogram to mammogram. They also calculated the deviation in the context of the different image categories, such as images that show cancer and those that may be easier or more difficult to decipher.
In order to effectively track the participants’ eye movements, the researchers had to employ real-time sensor data, which logs nearly every movement of the participants’ eyes. However, with 10 observers interpreting 100 cases, the data soon began adding up, making it impractical to manage such a data-intensive task manually. This made the researchers turn to artificial intelligence to help them efficiently and effectively make sense of the results. Using ORNL’s Titan supercomputer, the researchers were able to rapidly train the deep learning models required to make sense of the large datasets. While similar studies in the past have used aggregation methods to make sense of the enormous data sets, the team of researchers at ORNL processed the full data sequence, a critical task as over time this sequence revealed differentiations in the eye paths of the participants as they analyzed the various mammograms.
In a related paper published in the Journal of Human Performance in Extreme Environments, the researchers demonstrated how convolutional neural networks, a type of artificial intelligence commonly applied to the analysis of images, significantly outperformed other methods, such as deep neural networks and deep belief networks, in parsing the eye tracking data and, by extension, validating the experiment as a means to measure context bias. Furthermore, while the experiment focused on radiology, the resulting data drove home the need for “intelligent interfaces and decision support systems” to assist human performance across a range of complex tasks including air-traffic control and battlefield management.
While machines are unlikely to replace radiologists (or other humans involved in rapid, high-impact decision-making) any time soon, they do hold enormous potential to assist health professionals and other decision makers in reducing errors due to phenomena such as context bias, according to Gina Tourassi, team lead and director of ORNL’s Health Data Science Institute. “These findings will be critical in the future training of medical professionals to reduce errors in the interpretations of diagnostic imaging. These studies will inform human/computer interactions, going forward as we use artificial intelligence to augment and improve human performance,” said Tourassi.
Related Links:
Oak Ridge National Laboratory
Early detection of breast cancer is critical for effective treatment, which requires accurate interpretation of a patient’s mammogram. The ORNL-led team of researchers found that analyses of mammograms by radiologists were significantly influenced by context bias, or the radiologist’s previous diagnostic experiences. New radiology trainees were most susceptible to the phenomenon, although even more experienced radiologists fall victim to some degree, according to the researchers.
The researchers designed an experiment aimed at following the eye movements of radiologists at various skill levels to better understand the context bias involved in their individual interpretations of the images. The experiment followed the eye movements of three board certified radiologists and seven radiology residents as they analyzed 100 mammographic studies from the University of South Florida’s Digital Database for Screening Mammography. The 400 images, representing a mix of cancer, no cancer, and cases that mimicked cancer but were benign, were specifically selected to cover a range of cases similar to that found in a clinical setting.
The participants, who were grouped by levels of experience and had no prior knowledge of what was contained in the individual X-rays, were outfitted with a head-mounted eye-tracking device designed to record their “raw gaze data,” which characterized their overall visual behavior. The study also recorded the participants’ diagnostic decisions via the location of suspicious findings along with their characteristics according to the BI-RADS lexicon, the radiologists’ reporting scheme for mammograms. By computing a measure known as a fractal dimension on the individual participants’ scan path (map of eye movements) and performing a series of statistical calculations, the researchers were able to discern how the eye movements of the participants differed from mammogram to mammogram. They also calculated the deviation in the context of the different image categories, such as images that show cancer and those that may be easier or more difficult to decipher.
In order to effectively track the participants’ eye movements, the researchers had to employ real-time sensor data, which logs nearly every movement of the participants’ eyes. However, with 10 observers interpreting 100 cases, the data soon began adding up, making it impractical to manage such a data-intensive task manually. This made the researchers turn to artificial intelligence to help them efficiently and effectively make sense of the results. Using ORNL’s Titan supercomputer, the researchers were able to rapidly train the deep learning models required to make sense of the large datasets. While similar studies in the past have used aggregation methods to make sense of the enormous data sets, the team of researchers at ORNL processed the full data sequence, a critical task as over time this sequence revealed differentiations in the eye paths of the participants as they analyzed the various mammograms.
In a related paper published in the Journal of Human Performance in Extreme Environments, the researchers demonstrated how convolutional neural networks, a type of artificial intelligence commonly applied to the analysis of images, significantly outperformed other methods, such as deep neural networks and deep belief networks, in parsing the eye tracking data and, by extension, validating the experiment as a means to measure context bias. Furthermore, while the experiment focused on radiology, the resulting data drove home the need for “intelligent interfaces and decision support systems” to assist human performance across a range of complex tasks including air-traffic control and battlefield management.
While machines are unlikely to replace radiologists (or other humans involved in rapid, high-impact decision-making) any time soon, they do hold enormous potential to assist health professionals and other decision makers in reducing errors due to phenomena such as context bias, according to Gina Tourassi, team lead and director of ORNL’s Health Data Science Institute. “These findings will be critical in the future training of medical professionals to reduce errors in the interpretations of diagnostic imaging. These studies will inform human/computer interactions, going forward as we use artificial intelligence to augment and improve human performance,” said Tourassi.
Related Links:
Oak Ridge National Laboratory
Latest Industry News News
- GE HealthCare and NVIDIA Collaboration to Reimagine Diagnostic Imaging
- Patient-Specific 3D-Printed Phantoms Transform CT Imaging
- Siemens and Sectra Collaborate on Enhancing Radiology Workflows
- Bracco Diagnostics and ColoWatch Partner to Expand Availability CRC Screening Tests Using Virtual Colonoscopy
- Mindray Partners with TeleRay to Streamline Ultrasound Delivery
- Philips and Medtronic Partner on Stroke Care
- Siemens and Medtronic Enter into Global Partnership for Advancing Spine Care Imaging Technologies
- RSNA 2024 Technical Exhibits to Showcase Latest Advances in Radiology
- Bracco Collaborates with Arrayus on Microbubble-Assisted Focused Ultrasound Therapy for Pancreatic Cancer
- Innovative Collaboration to Enhance Ischemic Stroke Detection and Elevate Standards in Diagnostic Imaging
- RSNA 2024 Registration Opens
- Microsoft collaborates with Leading Academic Medical Systems to Advance AI in Medical Imaging
- GE HealthCare Acquires Intelligent Ultrasound Group’s Clinical Artificial Intelligence Business
- Bayer and Rad AI Collaborate on Expanding Use of Cutting Edge AI Radiology Operational Solutions
- Polish Med-Tech Company BrainScan to Expand Extensively into Foreign Markets
- Hologic Acquires UK-Based Breast Surgical Guidance Company Endomagnetics Ltd.
Channels
Radiography
view channel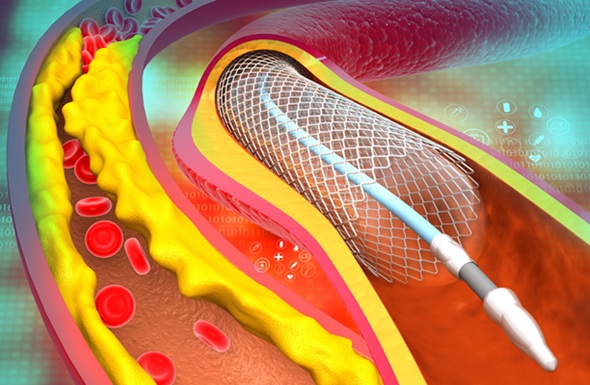
AI-Powered Imaging Technique Shows Promise in Evaluating Patients for PCI
Percutaneous coronary intervention (PCI), also known as coronary angioplasty, is a minimally invasive procedure where small metal tubes called stents are inserted into partially blocked coronary arteries... Read more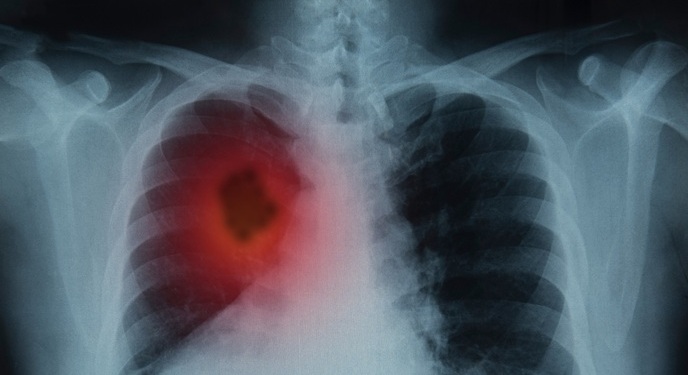
Higher Chest X-Ray Usage Catches Lung Cancer Earlier and Improves Survival
Lung cancer continues to be the leading cause of cancer-related deaths worldwide. While advanced technologies like CT scanners play a crucial role in detecting lung cancer, more accessible and affordable... Read moreMRI
view channel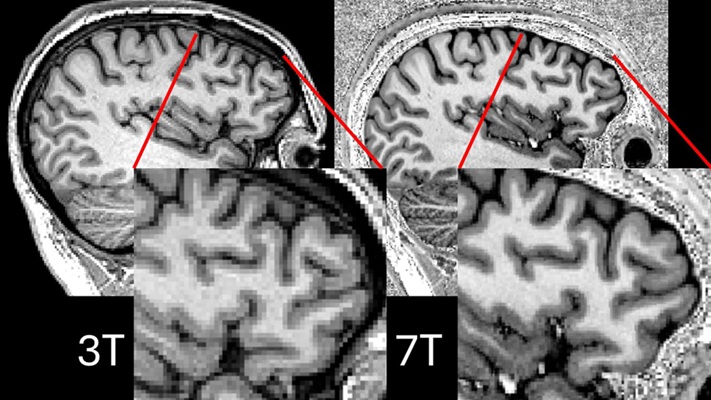
Ultra-Powerful MRI Scans Enable Life-Changing Surgery in Treatment-Resistant Epileptic Patients
Approximately 360,000 individuals in the UK suffer from focal epilepsy, a condition in which seizures spread from one part of the brain. Around a third of these patients experience persistent seizures... Read more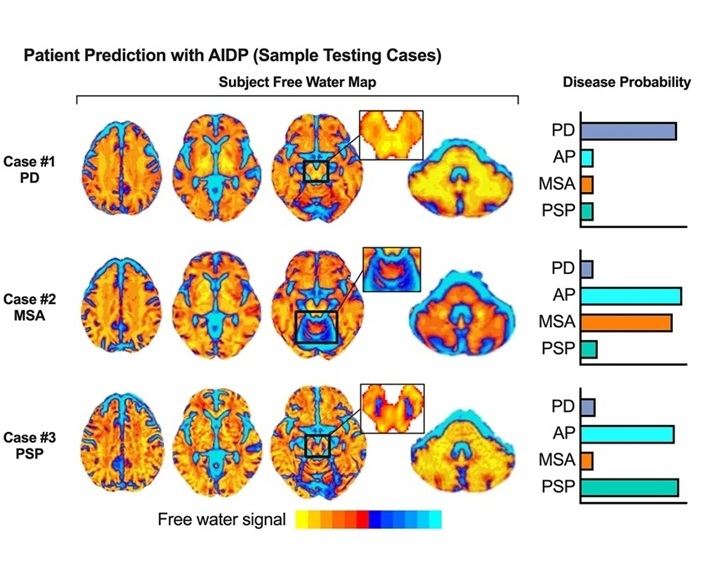
AI-Powered MRI Technology Improves Parkinson’s Diagnoses
Current research shows that the accuracy of diagnosing Parkinson’s disease typically ranges from 55% to 78% within the first five years of assessment. This is partly due to the similarities shared by Parkinson’s... Read more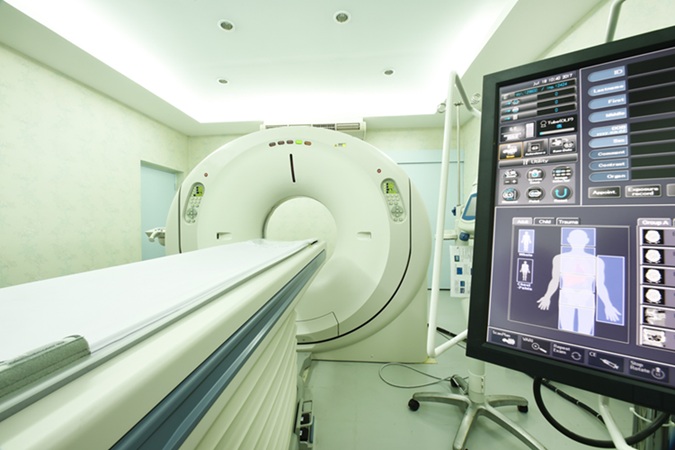
Biparametric MRI Combined with AI Enhances Detection of Clinically Significant Prostate Cancer
Artificial intelligence (AI) technologies are transforming the way medical images are analyzed, offering unprecedented capabilities in quantitatively extracting features that go beyond traditional visual... Read more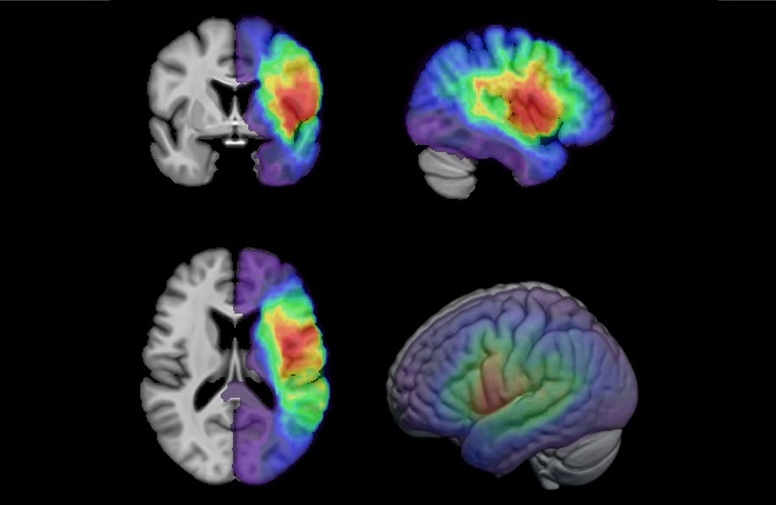
First-Of-Its-Kind AI-Driven Brain Imaging Platform to Better Guide Stroke Treatment Options
Each year, approximately 800,000 people in the U.S. experience strokes, with marginalized and minoritized groups being disproportionately affected. Strokes vary in terms of size and location within the... Read moreUltrasound
view channel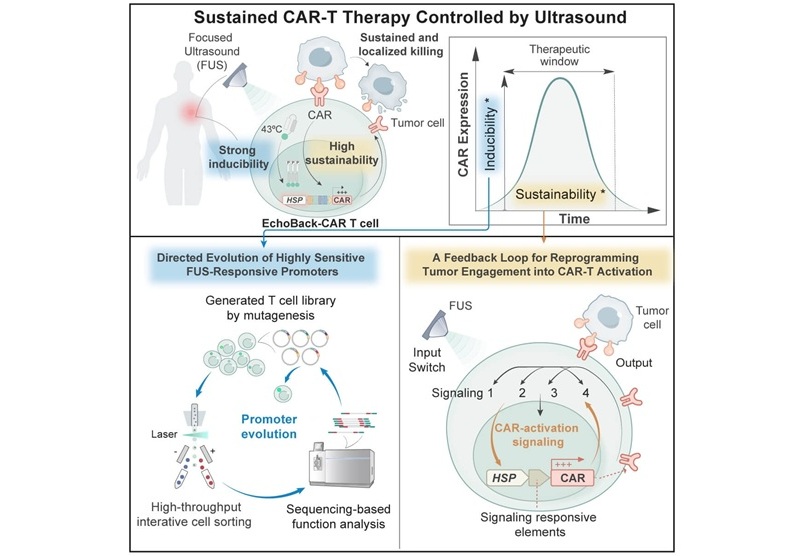
Smart Ultrasound-Activated Immune Cells Destroy Cancer Cells for Extended Periods
Chimeric antigen receptor (CAR) T-cell therapy has emerged as a highly promising cancer treatment, especially for bloodborne cancers like leukemia. This highly personalized therapy involves extracting... Read more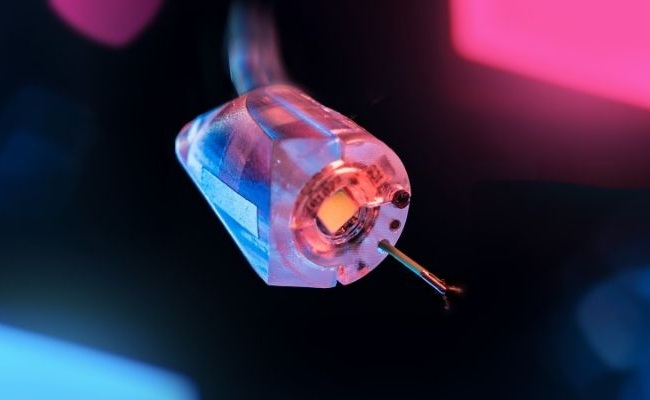
Tiny Magnetic Robot Takes 3D Scans from Deep Within Body
Colorectal cancer ranks as one of the leading causes of cancer-related mortality worldwide. However, when detected early, it is highly treatable. Now, a new minimally invasive technique could significantly... Read more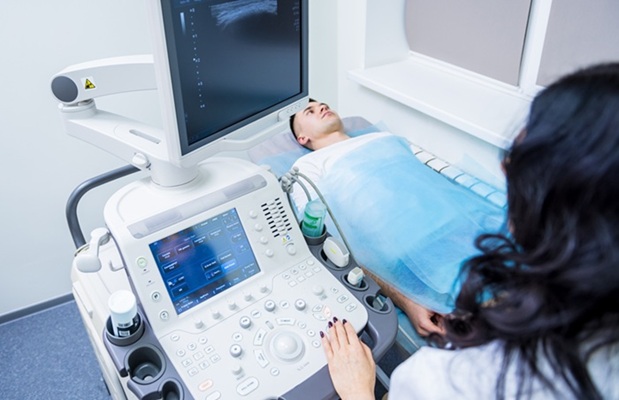
High Resolution Ultrasound Speeds Up Prostate Cancer Diagnosis
Each year, approximately one million prostate cancer biopsies are conducted across Europe, with similar numbers in the USA and around 100,000 in Canada. Most of these biopsies are performed using MRI images... Read more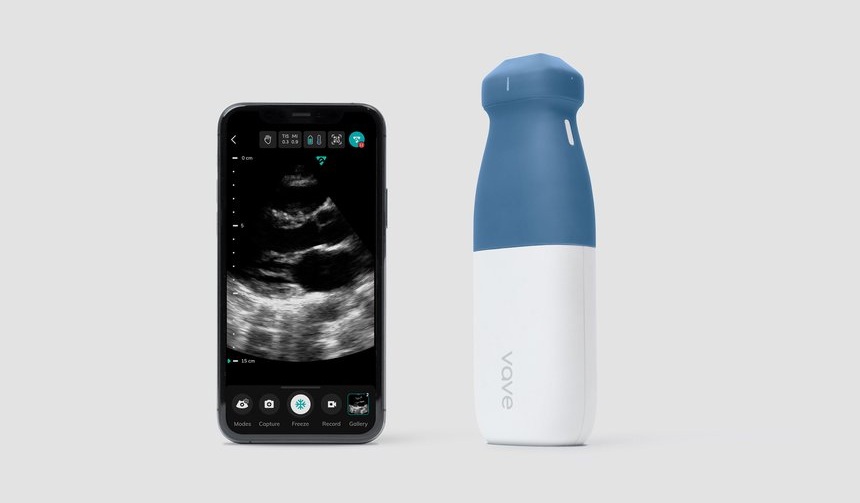
World's First Wireless, Handheld, Whole-Body Ultrasound with Single PZT Transducer Makes Imaging More Accessible
Ultrasound devices play a vital role in the medical field, routinely used to examine the body's internal tissues and structures. While advancements have steadily improved ultrasound image quality and processing... Read moreNuclear Medicine
view channel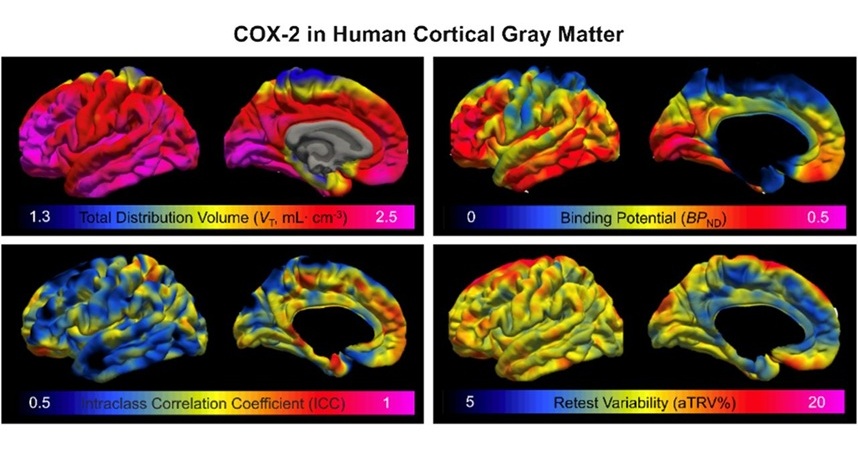
Novel PET Imaging Approach Offers Never-Before-Seen View of Neuroinflammation
COX-2, an enzyme that plays a key role in brain inflammation, can be significantly upregulated by inflammatory stimuli and neuroexcitation. Researchers suggest that COX-2 density in the brain could serve... Read more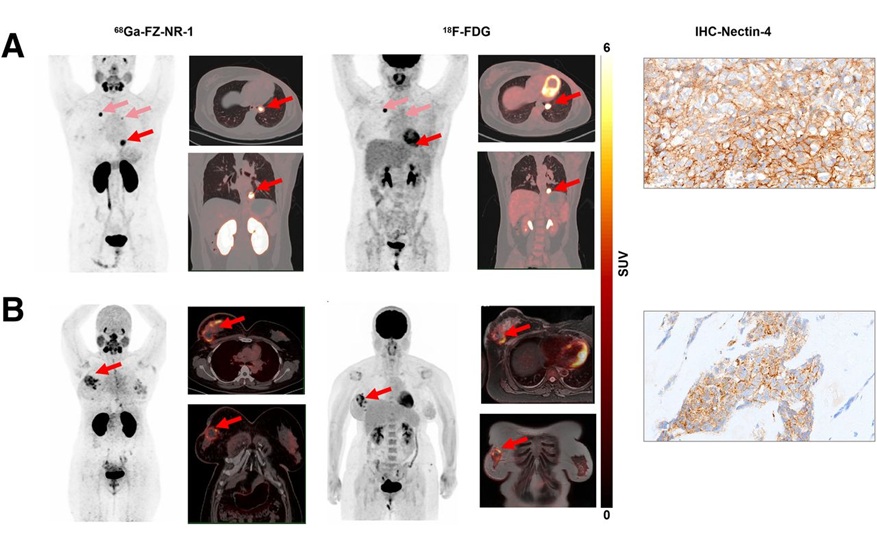
Novel Radiotracer Identifies Biomarker for Triple-Negative Breast Cancer
Triple-negative breast cancer (TNBC), which represents 15-20% of all breast cancer cases, is one of the most aggressive subtypes, with a five-year survival rate of about 40%. Due to its significant heterogeneity... Read moreGeneral/Advanced Imaging
view channel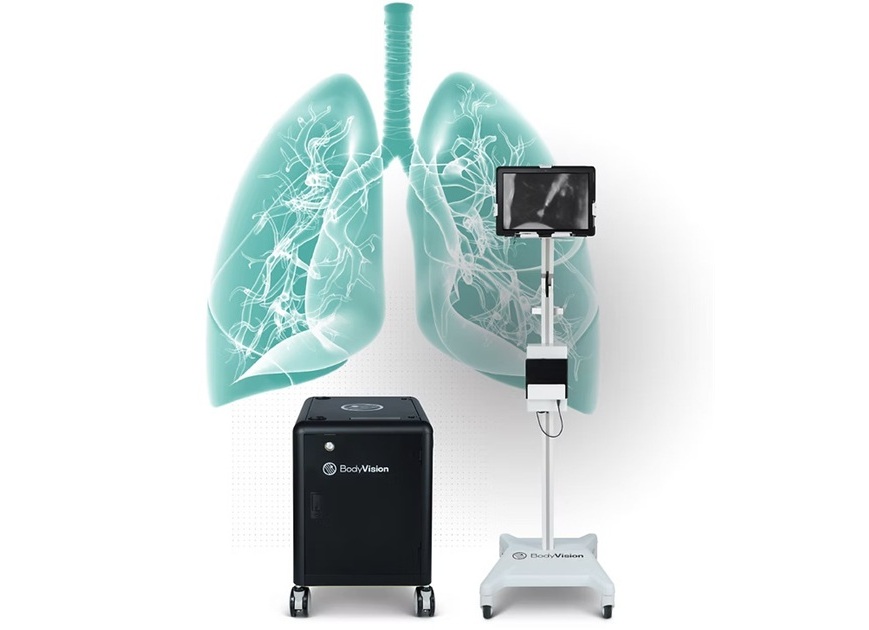
AI-Powered Imaging System Improves Lung Cancer Diagnosis
Given the need to detect lung cancer at earlier stages, there is an increasing need for a definitive diagnostic pathway for patients with suspicious pulmonary nodules. However, obtaining tissue samples... Read more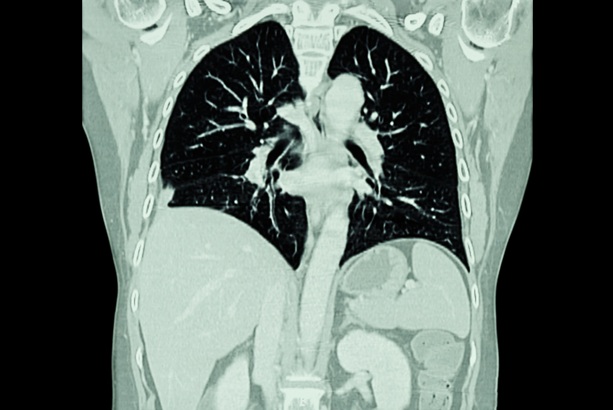
AI Model Significantly Enhances Low-Dose CT Capabilities
Lung cancer remains one of the most challenging diseases, making early diagnosis vital for effective treatment. Fortunately, advancements in artificial intelligence (AI) are revolutionizing lung cancer... Read moreImaging IT
view channel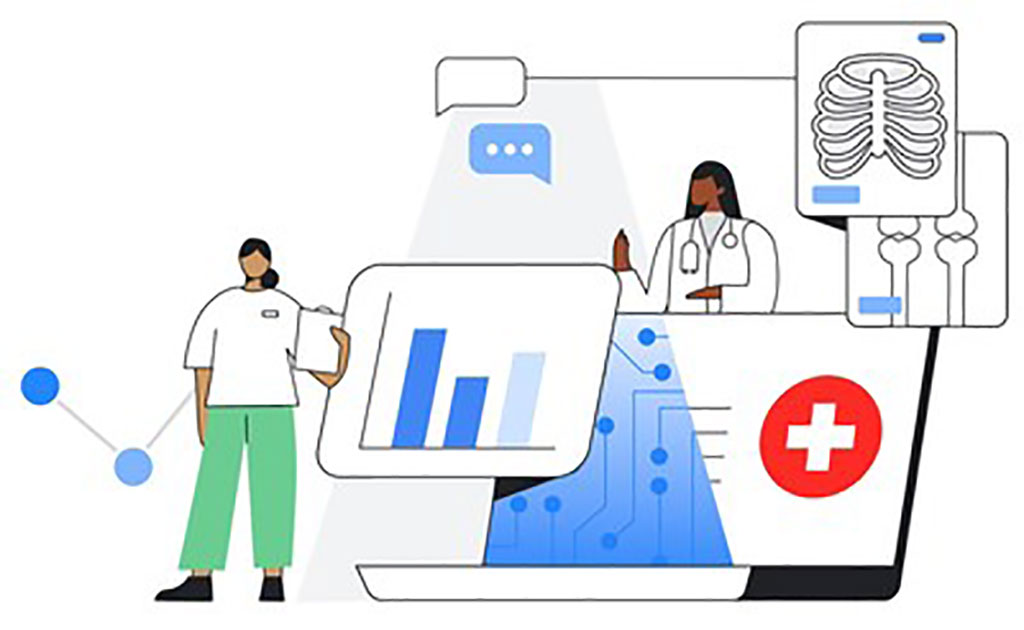
New Google Cloud Medical Imaging Suite Makes Imaging Healthcare Data More Accessible
Medical imaging is a critical tool used to diagnose patients, and there are billions of medical images scanned globally each year. Imaging data accounts for about 90% of all healthcare data1 and, until... Read more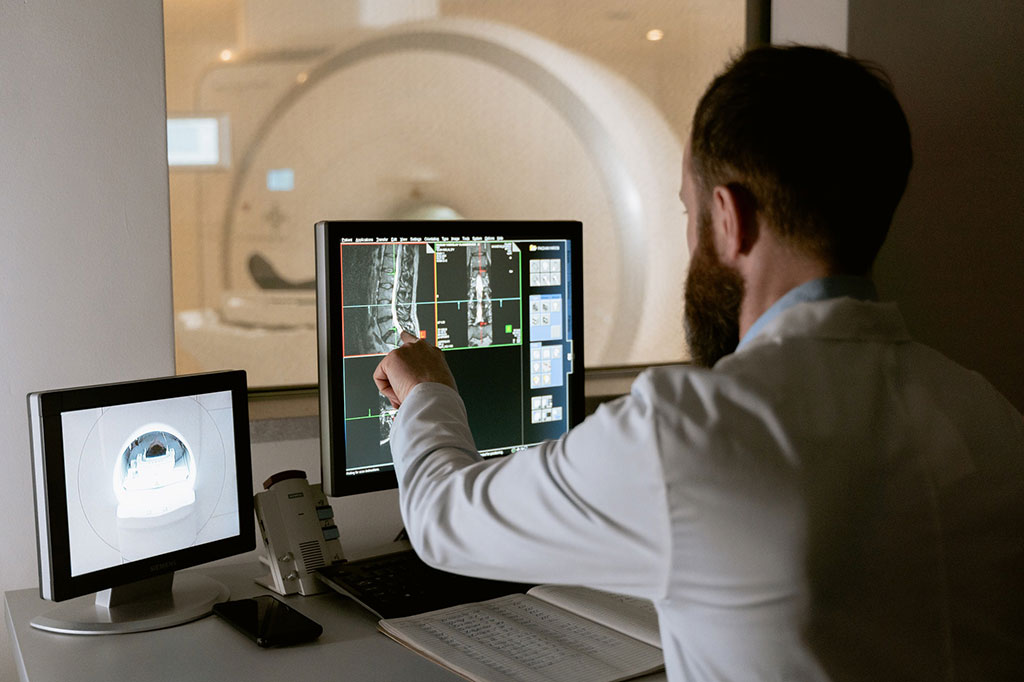