Cross-Sectional CT Imaging Could Predict Patient Longevity
By MedImaging International staff writers Posted on 14 Jun 2017 |
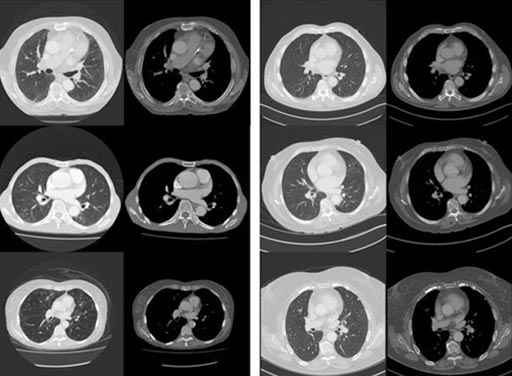
Image: Images of the proximal left anterior descending coronary artery most strongly predict mortality (L) and survival (R) cases (Photo courtesy of the University of Adelaide).
A new study suggests that analysis of computerized tomography (CT) images of internal organs could predict 5-year mortality with almost 70% accuracy.
Researchers at the University of Adelaide (UA; Australia) and other institutions conducted proof-of-concept experiments to demonstrate how routinely acquired cross-sectional CT imaging may be used to predict patient longevity as a proxy for overall individual health and disease status, using computer image analysis techniques. To do so, they first gathered 15,957 CT images of seven different tissues from patients aged 60 and older; using logistic regression, they identified a number of image features that were linked to 5-year mortality.
Based on the human-defined image features, they then used machine learning and a range of radiomic classifier models that included convolutional neural network random forests, support vector machines, and boosted tree algorithms in order to teach a computer to make 5-year mortality predictions. They found that as expected, the random forest model performed the best on the human-defined feature classifiers. An analysis showed the results were comparable to clinical methods for longevity prediction. The study was published on May 10, 2017, in Nature Scientific Reports.
“Recent advances in the field of medical image analysis have shown that machine-detectable image features can approximate the descriptive power of biopsy, microscopy, and even DNA analysis for a number of pathologies,” concluded lead author Luke Oakden-Rayner, PhD, of the UA School of Public Health, and colleagues. “Instead of focusing on diagnosing diseases, the automated systems can predict medical outcomes in a way that doctors are not trained to do, by incorporating large volumes of data and detecting subtle patterns.”
Deep learning, a computer learning method which automatically discovers visual features that are suited to a specific task through a process of optimization, has rapidly overtaken more traditional methods in many computer vision tasks, such as image recognition and segmentation, and have approached or even surpassed human level capabilities for complex “real-world” tasks such as image recognition, speech recognition, natural language processing, complex game playing, and more.
Related Links:
University of Adelaide
Researchers at the University of Adelaide (UA; Australia) and other institutions conducted proof-of-concept experiments to demonstrate how routinely acquired cross-sectional CT imaging may be used to predict patient longevity as a proxy for overall individual health and disease status, using computer image analysis techniques. To do so, they first gathered 15,957 CT images of seven different tissues from patients aged 60 and older; using logistic regression, they identified a number of image features that were linked to 5-year mortality.
Based on the human-defined image features, they then used machine learning and a range of radiomic classifier models that included convolutional neural network random forests, support vector machines, and boosted tree algorithms in order to teach a computer to make 5-year mortality predictions. They found that as expected, the random forest model performed the best on the human-defined feature classifiers. An analysis showed the results were comparable to clinical methods for longevity prediction. The study was published on May 10, 2017, in Nature Scientific Reports.
“Recent advances in the field of medical image analysis have shown that machine-detectable image features can approximate the descriptive power of biopsy, microscopy, and even DNA analysis for a number of pathologies,” concluded lead author Luke Oakden-Rayner, PhD, of the UA School of Public Health, and colleagues. “Instead of focusing on diagnosing diseases, the automated systems can predict medical outcomes in a way that doctors are not trained to do, by incorporating large volumes of data and detecting subtle patterns.”
Deep learning, a computer learning method which automatically discovers visual features that are suited to a specific task through a process of optimization, has rapidly overtaken more traditional methods in many computer vision tasks, such as image recognition and segmentation, and have approached or even surpassed human level capabilities for complex “real-world” tasks such as image recognition, speech recognition, natural language processing, complex game playing, and more.
Related Links:
University of Adelaide
Latest Radiography News
- AI-Powered Imaging Technique Shows Promise in Evaluating Patients for PCI
- Higher Chest X-Ray Usage Catches Lung Cancer Earlier and Improves Survival
- AI-Powered Mammograms Predict Cardiovascular Risk
- Generative AI Model Significantly Reduces Chest X-Ray Reading Time
- AI-Powered Mammography Screening Boosts Cancer Detection in Single-Reader Settings
- Photon Counting Detectors Promise Fast Color X-Ray Images
- AI Can Flag Mammograms for Supplemental MRI
- 3D CT Imaging from Single X-Ray Projection Reduces Radiation Exposure
- AI Method Accurately Predicts Breast Cancer Risk by Analyzing Multiple Mammograms
- Printable Organic X-Ray Sensors Could Transform Treatment for Cancer Patients
- Highly Sensitive, Foldable Detector to Make X-Rays Safer
- Novel Breast Cancer Screening Technology Could Offer Superior Alternative to Mammogram
- Artificial Intelligence Accurately Predicts Breast Cancer Years Before Diagnosis
- AI-Powered Chest X-Ray Detects Pulmonary Nodules Three Years Before Lung Cancer Symptoms
- AI Model Identifies Vertebral Compression Fractures in Chest Radiographs
- Advanced 3D Mammography Detects More Breast Cancers
Channels
MRI
view channel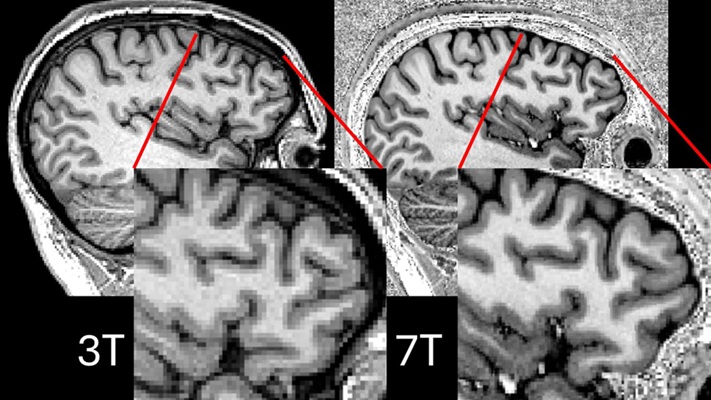
Ultra-Powerful MRI Scans Enable Life-Changing Surgery in Treatment-Resistant Epileptic Patients
Approximately 360,000 individuals in the UK suffer from focal epilepsy, a condition in which seizures spread from one part of the brain. Around a third of these patients experience persistent seizures... Read more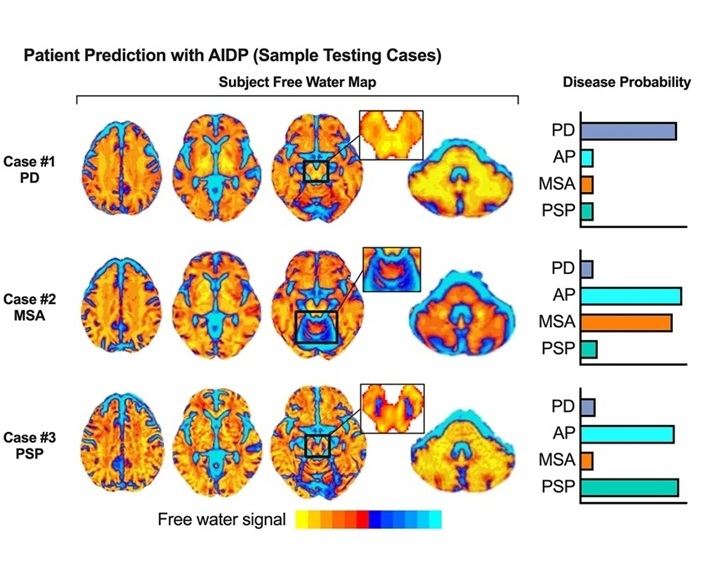
AI-Powered MRI Technology Improves Parkinson’s Diagnoses
Current research shows that the accuracy of diagnosing Parkinson’s disease typically ranges from 55% to 78% within the first five years of assessment. This is partly due to the similarities shared by Parkinson’s... Read more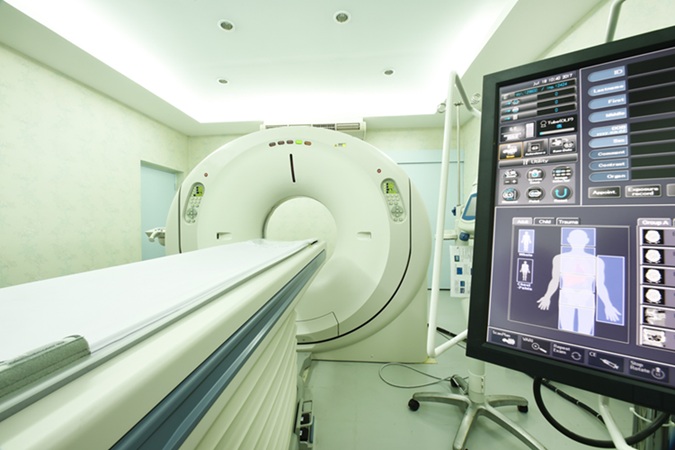
Biparametric MRI Combined with AI Enhances Detection of Clinically Significant Prostate Cancer
Artificial intelligence (AI) technologies are transforming the way medical images are analyzed, offering unprecedented capabilities in quantitatively extracting features that go beyond traditional visual... Read more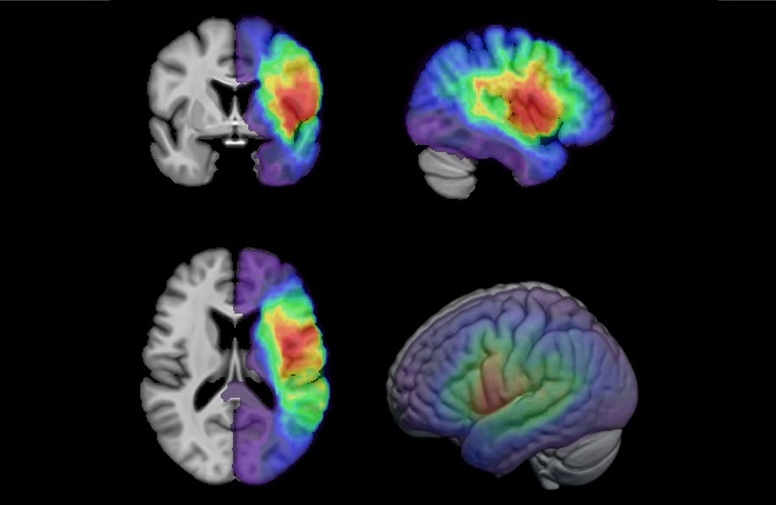
First-Of-Its-Kind AI-Driven Brain Imaging Platform to Better Guide Stroke Treatment Options
Each year, approximately 800,000 people in the U.S. experience strokes, with marginalized and minoritized groups being disproportionately affected. Strokes vary in terms of size and location within the... Read moreUltrasound
view channel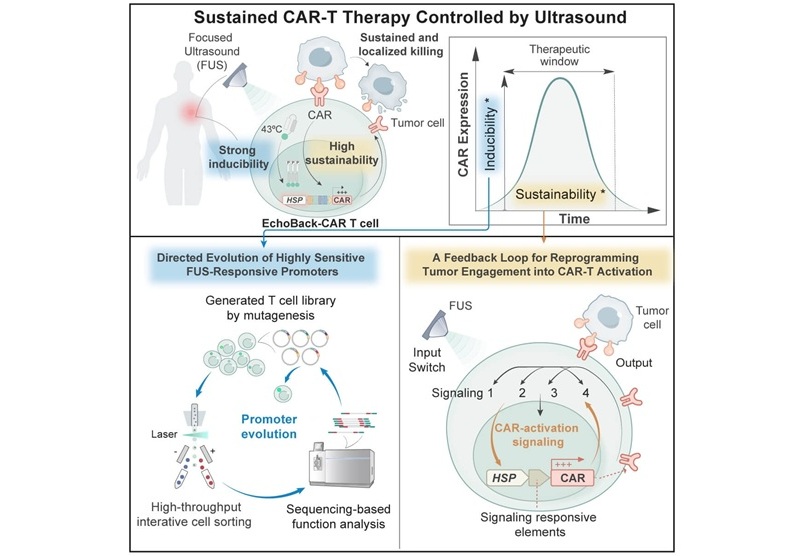
Smart Ultrasound-Activated Immune Cells Destroy Cancer Cells for Extended Periods
Chimeric antigen receptor (CAR) T-cell therapy has emerged as a highly promising cancer treatment, especially for bloodborne cancers like leukemia. This highly personalized therapy involves extracting... Read more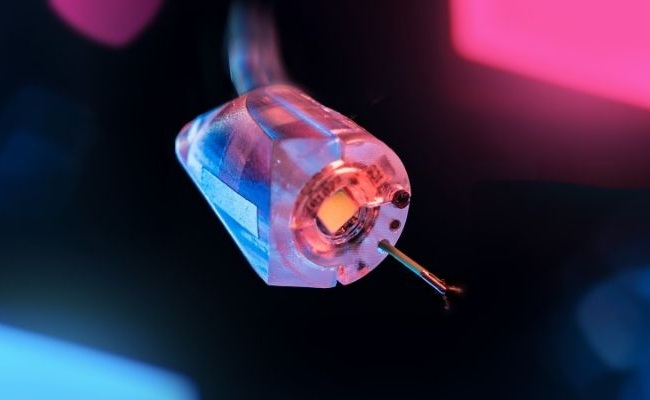
Tiny Magnetic Robot Takes 3D Scans from Deep Within Body
Colorectal cancer ranks as one of the leading causes of cancer-related mortality worldwide. However, when detected early, it is highly treatable. Now, a new minimally invasive technique could significantly... Read more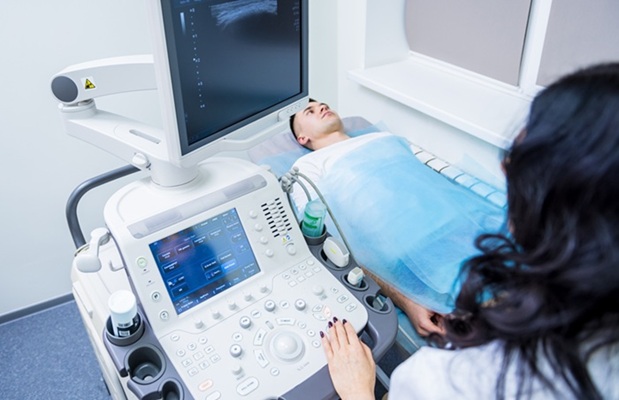
High Resolution Ultrasound Speeds Up Prostate Cancer Diagnosis
Each year, approximately one million prostate cancer biopsies are conducted across Europe, with similar numbers in the USA and around 100,000 in Canada. Most of these biopsies are performed using MRI images... Read more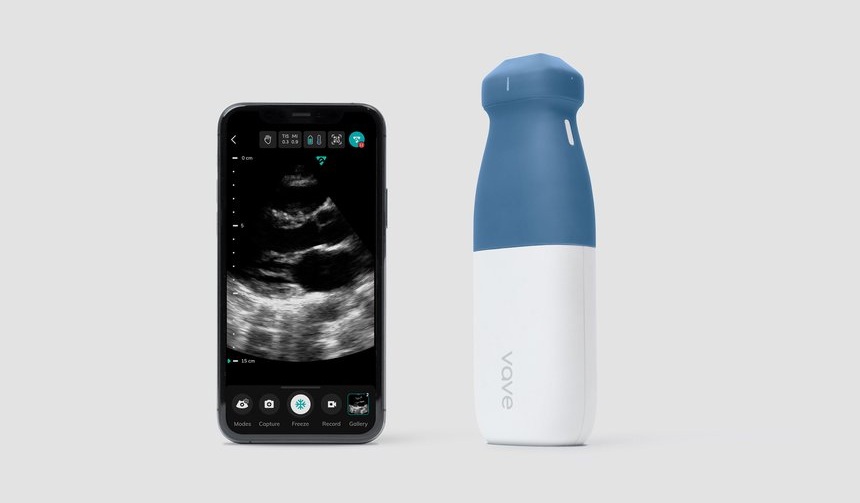
World's First Wireless, Handheld, Whole-Body Ultrasound with Single PZT Transducer Makes Imaging More Accessible
Ultrasound devices play a vital role in the medical field, routinely used to examine the body's internal tissues and structures. While advancements have steadily improved ultrasound image quality and processing... Read moreNuclear Medicine
view channel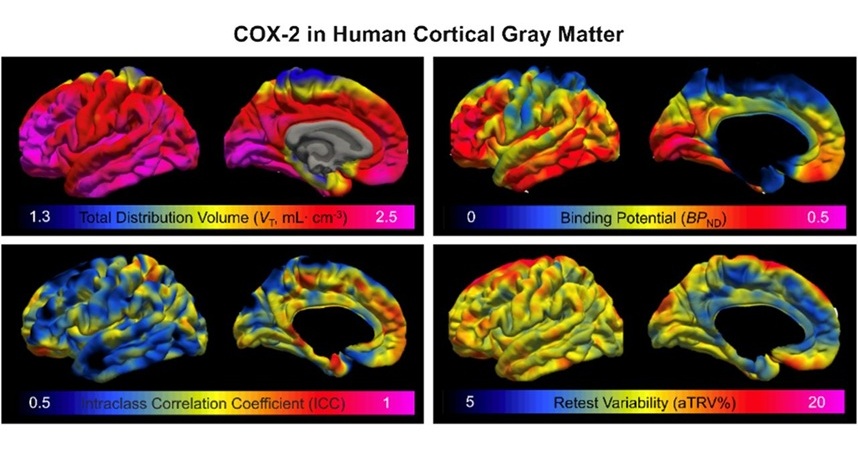
Novel PET Imaging Approach Offers Never-Before-Seen View of Neuroinflammation
COX-2, an enzyme that plays a key role in brain inflammation, can be significantly upregulated by inflammatory stimuli and neuroexcitation. Researchers suggest that COX-2 density in the brain could serve... Read more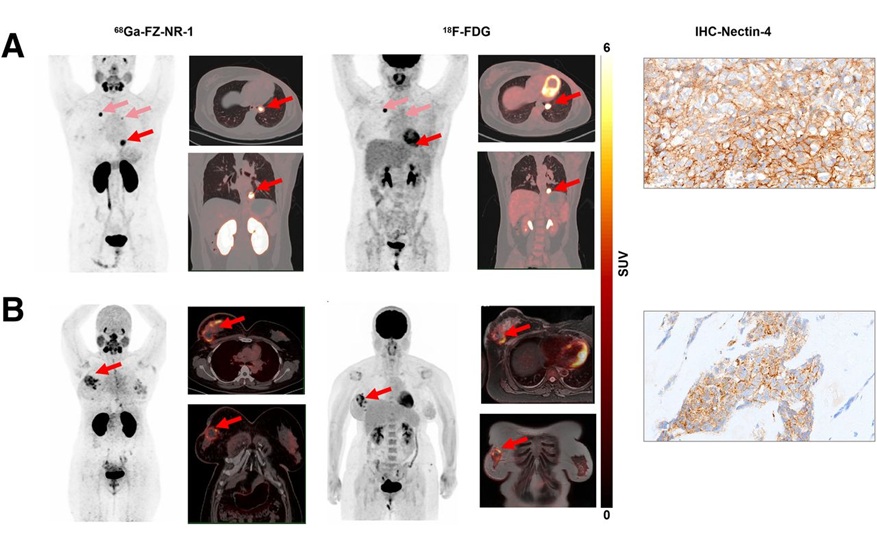
Novel Radiotracer Identifies Biomarker for Triple-Negative Breast Cancer
Triple-negative breast cancer (TNBC), which represents 15-20% of all breast cancer cases, is one of the most aggressive subtypes, with a five-year survival rate of about 40%. Due to its significant heterogeneity... Read moreGeneral/Advanced Imaging
view channel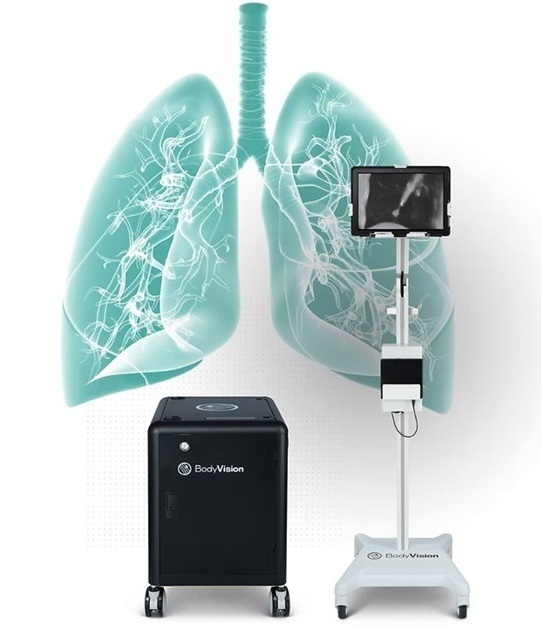
AI-Powered Imaging System Improves Lung Cancer Diagnosis
Given the need to detect lung cancer at earlier stages, there is an increasing need for a definitive diagnostic pathway for patients with suspicious pulmonary nodules. However, obtaining tissue samples... Read more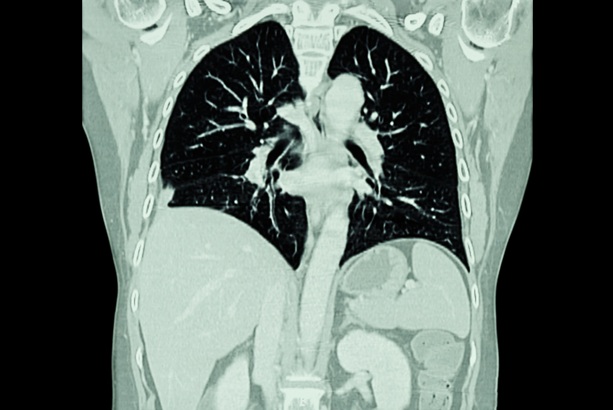
AI Model Significantly Enhances Low-Dose CT Capabilities
Lung cancer remains one of the most challenging diseases, making early diagnosis vital for effective treatment. Fortunately, advancements in artificial intelligence (AI) are revolutionizing lung cancer... Read moreImaging IT
view channel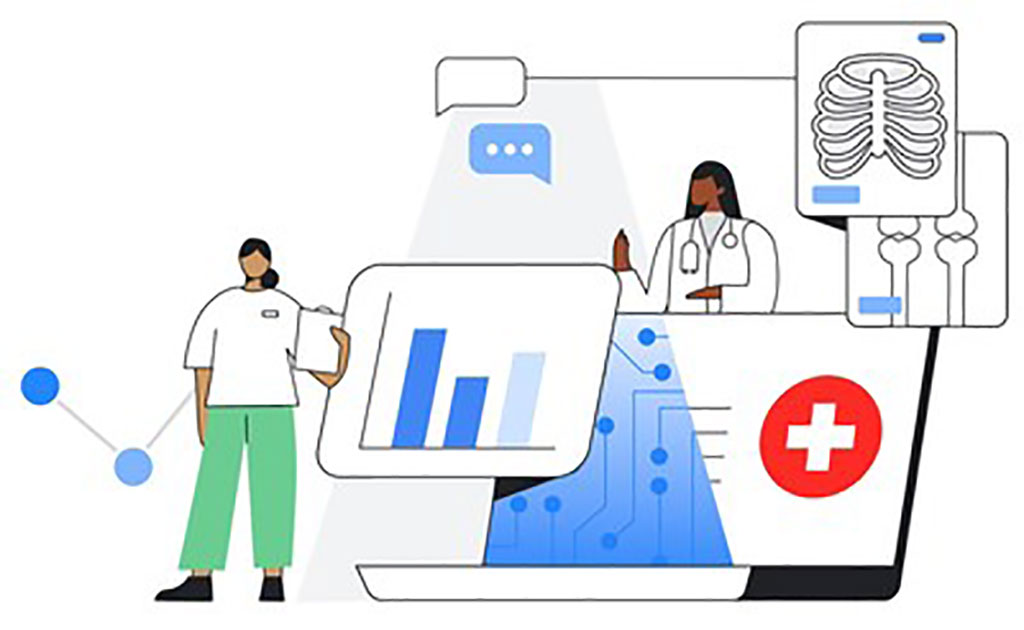
New Google Cloud Medical Imaging Suite Makes Imaging Healthcare Data More Accessible
Medical imaging is a critical tool used to diagnose patients, and there are billions of medical images scanned globally each year. Imaging data accounts for about 90% of all healthcare data1 and, until... Read more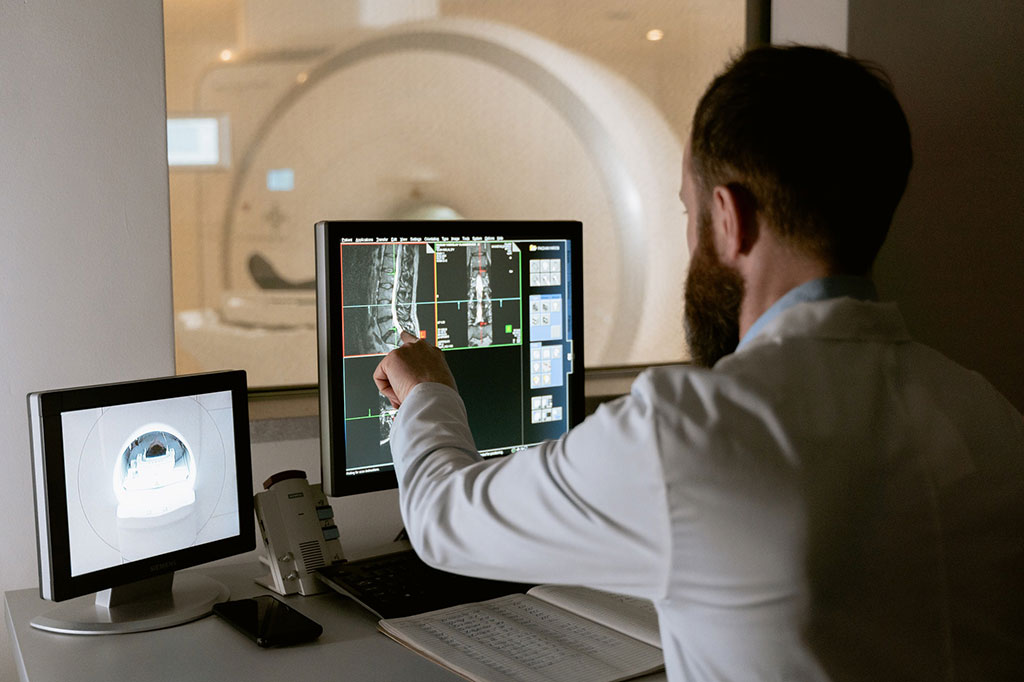
Global AI in Medical Diagnostics Market to Be Driven by Demand for Image Recognition in Radiology
The global artificial intelligence (AI) in medical diagnostics market is expanding with early disease detection being one of its key applications and image recognition becoming a compelling consumer proposition... Read moreIndustry News
view channel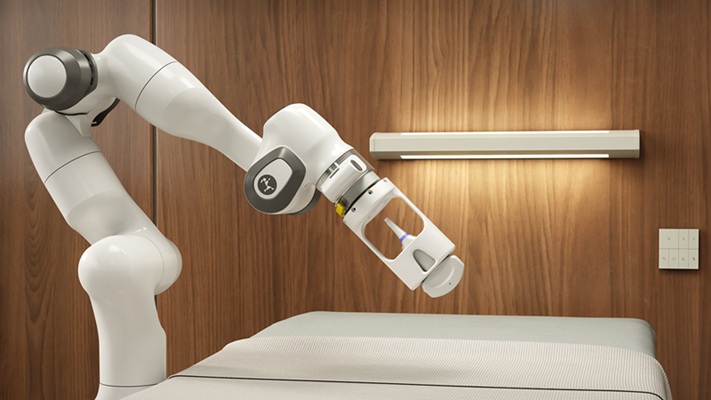
GE HealthCare and NVIDIA Collaboration to Reimagine Diagnostic Imaging
GE HealthCare (Chicago, IL, USA) has entered into a collaboration with NVIDIA (Santa Clara, CA, USA), expanding the existing relationship between the two companies to focus on pioneering innovation in... Read more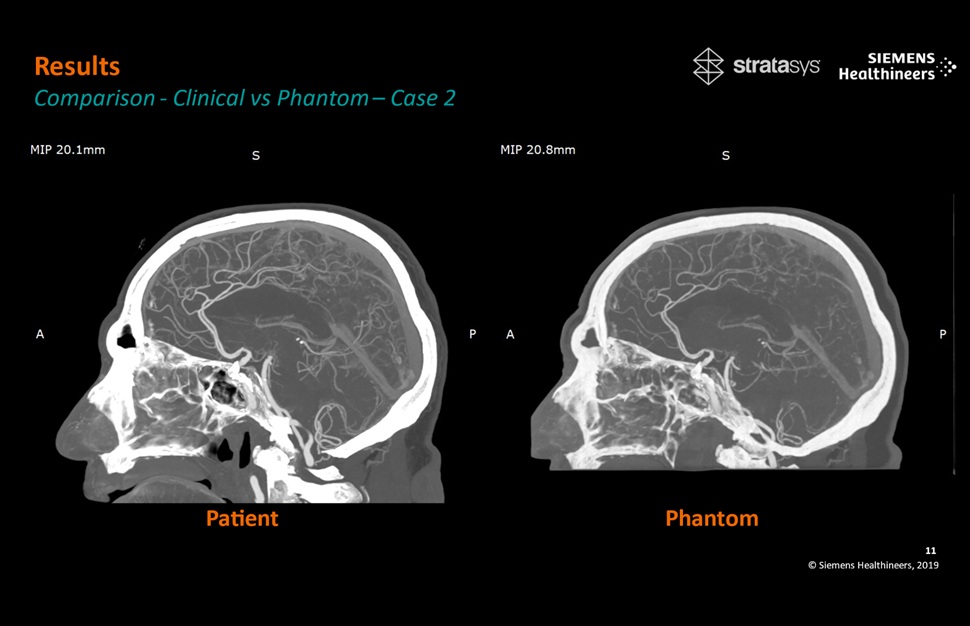
Patient-Specific 3D-Printed Phantoms Transform CT Imaging
New research has highlighted how anatomically precise, patient-specific 3D-printed phantoms are proving to be scalable, cost-effective, and efficient tools in the development of new CT scan algorithms... Read more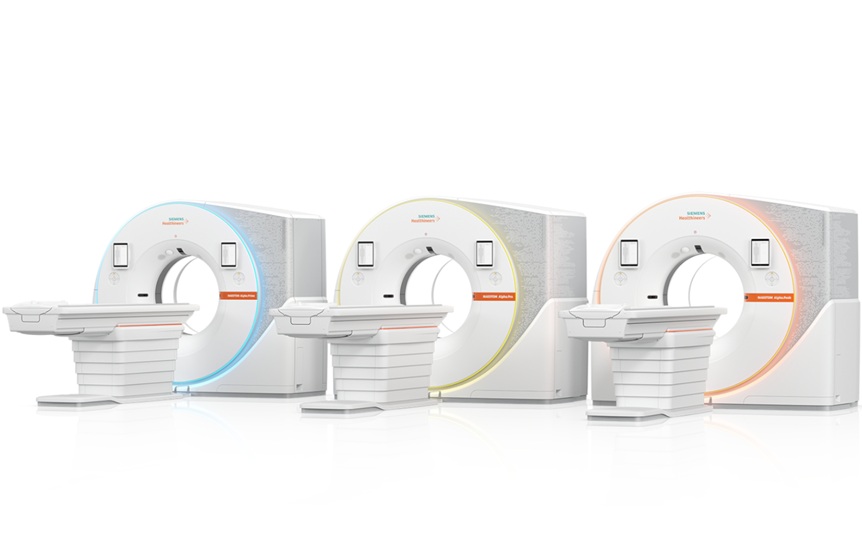
Siemens and Sectra Collaborate on Enhancing Radiology Workflows
Siemens Healthineers (Forchheim, Germany) and Sectra (Linköping, Sweden) have entered into a collaboration aimed at enhancing radiologists' diagnostic capabilities and, in turn, improving patient care... Read more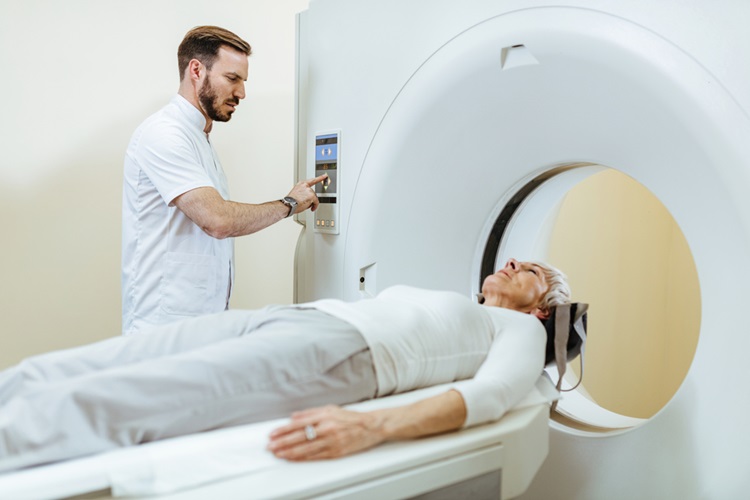