Segmentation Makes Lymph-Node Measurement More Accurate
By MedImaging International staff writers Posted on 15 Dec 2016 |
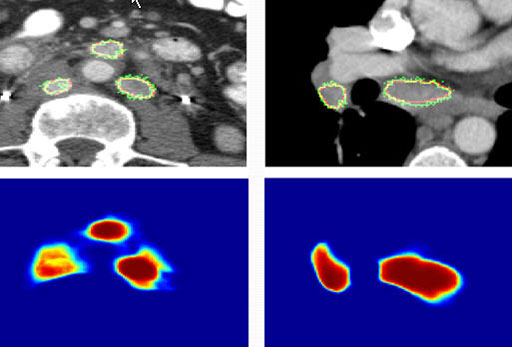
Image: Lymph nodes segmented by BNF (green), with corresponding BNF probability maps (Photo courtesy of Ronald Summers/NIH).
Neural networks could help a new automated lymph-node segmentation method improve cancer care, according to a new study.
Developed at the U.S. National Institutes of Health (NIH, Bethesda, MD, USA), the new analysis method combines two holistically nested neural networks (HNNs) and structured optimization techniques in order to solve the problem of separating lymph nodes from similar surrounding tissues on radiographic images. In addition, the technique can measure the volume of the lymph node clusters, which is a more accurate estimation of size than diameter.
The two HNNs, built on convolutional and deeply supervised neural networks, are trained to learn lymph-node cluster appearance (HNN-A) or contour (HNN-C) probabilistic output maps derived from thoracoabdominal computerized tomography (CT) images. The two components are prominent in the concept of boundary neural fields (BNF), which matches lesion measurements with more than 80% accuracy. To do so, HNN-A and HNN-C predictions are formulated into unary and pairwise terms of conditional random fields (CRFs).
The CRFs are subsequently solved using three standardized output methods: dense CRF (dCRF), graph cuts (GC), and BNFs. All of these segmentation predictions are used to compute lymph-node cluster volumes on the dataset. An expert radiologist then segments all enlarged lymph nodes with short-axis diameters of at least 10 mm. According to the researchers, the method could significantly improve cancer care by making lymph-node measurements more accurate. The new segmentation method was presented at the RSNA annual conference, held during November 2016 in Chicago (IL, USA).
“Thoracoabdominal lesions are among the most challenging to analyze on CT. The reason for this is that CT images of this region display very poor intensity and texture contrast. This is due to the fact that lymph nodes are very similar in intensity to many of the surrounding tissues,” said lead author and study presenter Isabella Nogues, MD. “Also, the high frequency of lymph-node clusters and the ambiguous boundaries of lymph nodes make accurate measurement very difficult.”
“The main conclusion was that boundary neural field was the most accurate segmentation method, which is very promising for the development of imaging biomarkers based on volume measurements,” concluded Dr. Nogues and colleagues of the NIH Imaging Biomarkers and Computer-Aided Diagnosis Laboratory. “These volume measurements, in turn, could potentially improve response evaluation criteria in solid tumors (RECIST) measurements for lymph nodes.”
Related Links:
U.S. National Institutes of Health
Developed at the U.S. National Institutes of Health (NIH, Bethesda, MD, USA), the new analysis method combines two holistically nested neural networks (HNNs) and structured optimization techniques in order to solve the problem of separating lymph nodes from similar surrounding tissues on radiographic images. In addition, the technique can measure the volume of the lymph node clusters, which is a more accurate estimation of size than diameter.
The two HNNs, built on convolutional and deeply supervised neural networks, are trained to learn lymph-node cluster appearance (HNN-A) or contour (HNN-C) probabilistic output maps derived from thoracoabdominal computerized tomography (CT) images. The two components are prominent in the concept of boundary neural fields (BNF), which matches lesion measurements with more than 80% accuracy. To do so, HNN-A and HNN-C predictions are formulated into unary and pairwise terms of conditional random fields (CRFs).
The CRFs are subsequently solved using three standardized output methods: dense CRF (dCRF), graph cuts (GC), and BNFs. All of these segmentation predictions are used to compute lymph-node cluster volumes on the dataset. An expert radiologist then segments all enlarged lymph nodes with short-axis diameters of at least 10 mm. According to the researchers, the method could significantly improve cancer care by making lymph-node measurements more accurate. The new segmentation method was presented at the RSNA annual conference, held during November 2016 in Chicago (IL, USA).
“Thoracoabdominal lesions are among the most challenging to analyze on CT. The reason for this is that CT images of this region display very poor intensity and texture contrast. This is due to the fact that lymph nodes are very similar in intensity to many of the surrounding tissues,” said lead author and study presenter Isabella Nogues, MD. “Also, the high frequency of lymph-node clusters and the ambiguous boundaries of lymph nodes make accurate measurement very difficult.”
“The main conclusion was that boundary neural field was the most accurate segmentation method, which is very promising for the development of imaging biomarkers based on volume measurements,” concluded Dr. Nogues and colleagues of the NIH Imaging Biomarkers and Computer-Aided Diagnosis Laboratory. “These volume measurements, in turn, could potentially improve response evaluation criteria in solid tumors (RECIST) measurements for lymph nodes.”
Related Links:
U.S. National Institutes of Health
Latest General/Advanced Imaging News
- AI-Powered Imaging System Improves Lung Cancer Diagnosis
- AI Model Significantly Enhances Low-Dose CT Capabilities
- Ultra-Low Dose CT Aids Pneumonia Diagnosis in Immunocompromised Patients
- AI Reduces CT Lung Cancer Screening Workload by Almost 80%
- Cutting-Edge Technology Combines Light and Sound for Real-Time Stroke Monitoring
- AI System Detects Subtle Changes in Series of Medical Images Over Time
- New CT Scan Technique to Improve Prognosis and Treatments for Head and Neck Cancers
- World’s First Mobile Whole-Body CT Scanner to Provide Diagnostics at POC
- Comprehensive CT Scans Could Identify Atherosclerosis Among Lung Cancer Patients
- AI Improves Detection of Colorectal Cancer on Routine Abdominopelvic CT Scans
- Super-Resolution Technology Enhances Clinical Bone Imaging to Predict Osteoporotic Fracture Risk
- AI-Powered Abdomen Map Enables Early Cancer Detection
- Deep Learning Model Detects Lung Tumors on CT
- AI Predicts Cardiovascular Risk from CT Scans
- Deep Learning Based Algorithms Improve Tumor Detection in PET/CT Scans
- New Technology Provides Coronary Artery Calcification Scoring on Ungated Chest CT Scans
Channels
Radiography
view channel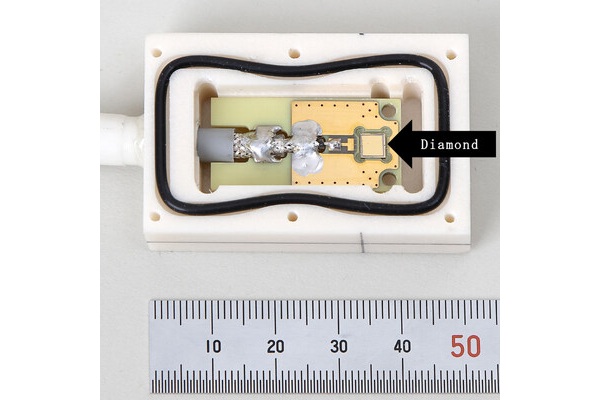
World's Largest Class Single Crystal Diamond Radiation Detector Opens New Possibilities for Diagnostic Imaging
Diamonds possess ideal physical properties for radiation detection, such as exceptional thermal and chemical stability along with a quick response time. Made of carbon with an atomic number of six, diamonds... Read more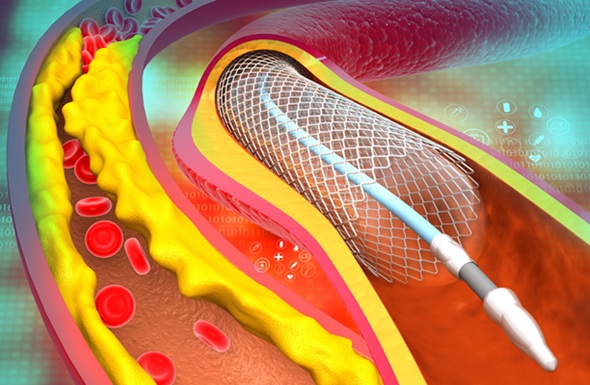
AI-Powered Imaging Technique Shows Promise in Evaluating Patients for PCI
Percutaneous coronary intervention (PCI), also known as coronary angioplasty, is a minimally invasive procedure where small metal tubes called stents are inserted into partially blocked coronary arteries... Read moreMRI
view channel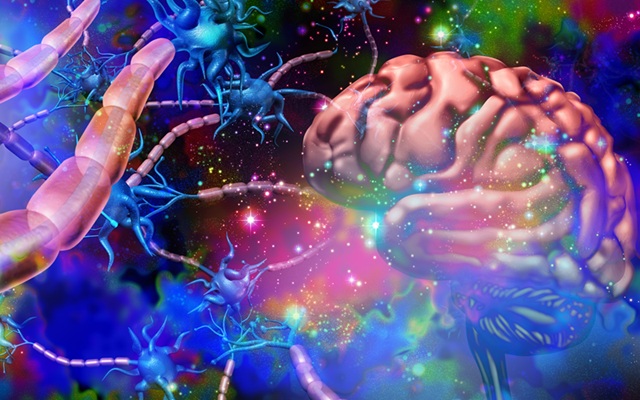
AI Tool Tracks Effectiveness of Multiple Sclerosis Treatments Using Brain MRI Scans
Multiple sclerosis (MS) is a condition in which the immune system attacks the brain and spinal cord, leading to impairments in movement, sensation, and cognition. Magnetic Resonance Imaging (MRI) markers... Read more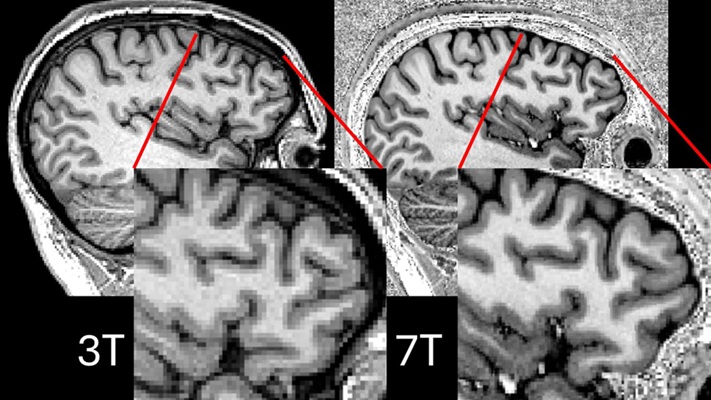
Ultra-Powerful MRI Scans Enable Life-Changing Surgery in Treatment-Resistant Epileptic Patients
Approximately 360,000 individuals in the UK suffer from focal epilepsy, a condition in which seizures spread from one part of the brain. Around a third of these patients experience persistent seizures... Read more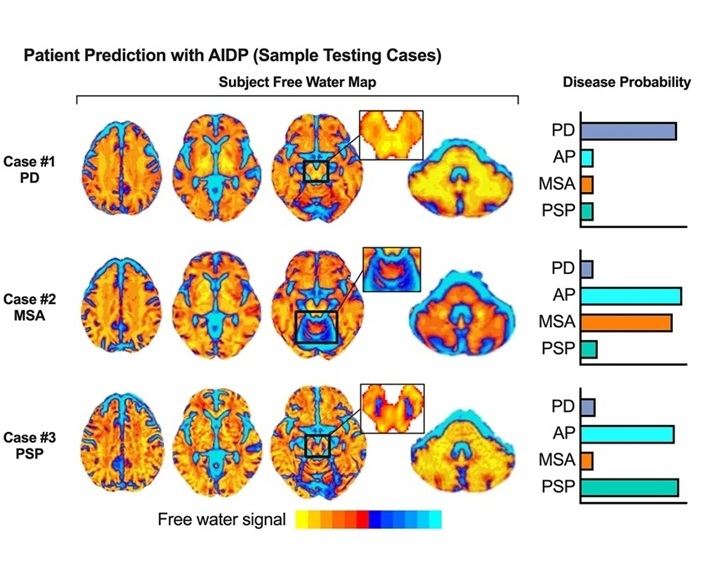
AI-Powered MRI Technology Improves Parkinson’s Diagnoses
Current research shows that the accuracy of diagnosing Parkinson’s disease typically ranges from 55% to 78% within the first five years of assessment. This is partly due to the similarities shared by Parkinson’s... Read more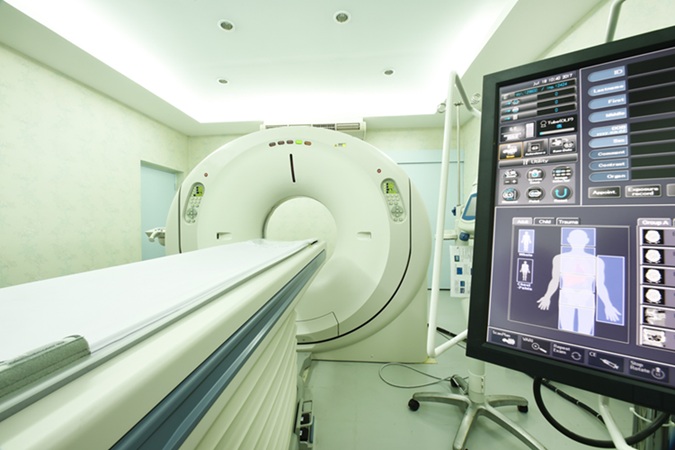
Biparametric MRI Combined with AI Enhances Detection of Clinically Significant Prostate Cancer
Artificial intelligence (AI) technologies are transforming the way medical images are analyzed, offering unprecedented capabilities in quantitatively extracting features that go beyond traditional visual... Read moreUltrasound
view channel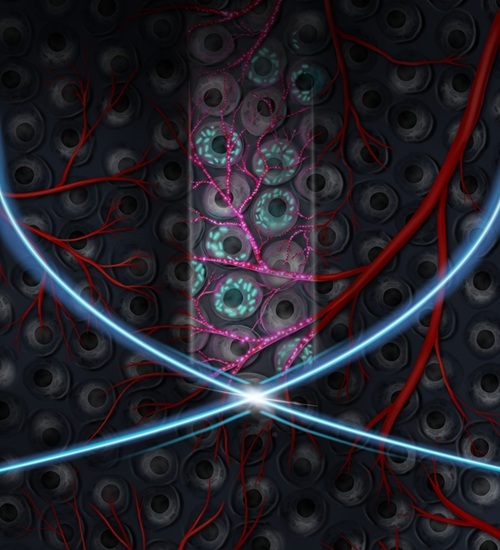
Ultrasound-Based Microscopy Technique to Help Diagnose Small Vessel Diseases
Clinical ultrasound, commonly used in pregnancy scans, provides real-time images of body structures. It is one of the most widely used imaging techniques in medicine, but until recently, it had little... Read more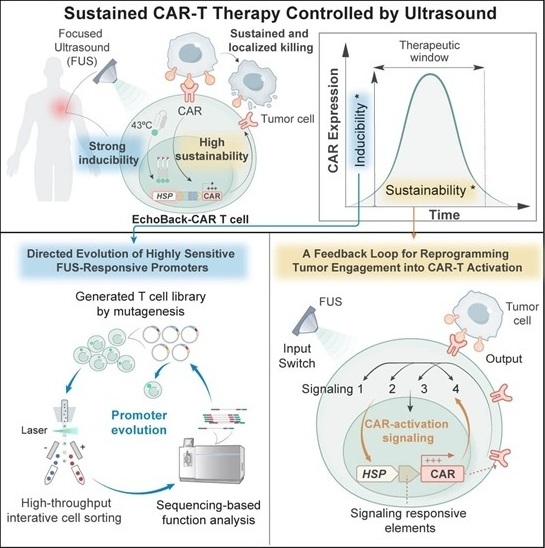
Smart Ultrasound-Activated Immune Cells Destroy Cancer Cells for Extended Periods
Chimeric antigen receptor (CAR) T-cell therapy has emerged as a highly promising cancer treatment, especially for bloodborne cancers like leukemia. This highly personalized therapy involves extracting... Read moreNuclear Medicine
view channel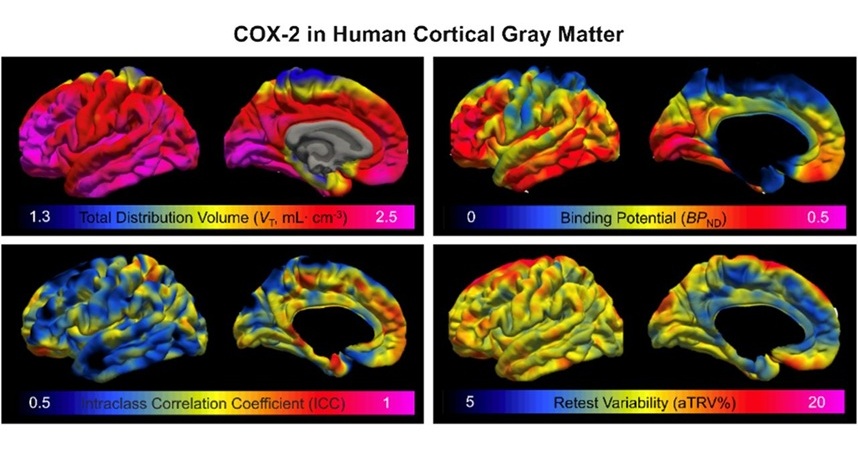
Novel PET Imaging Approach Offers Never-Before-Seen View of Neuroinflammation
COX-2, an enzyme that plays a key role in brain inflammation, can be significantly upregulated by inflammatory stimuli and neuroexcitation. Researchers suggest that COX-2 density in the brain could serve... Read more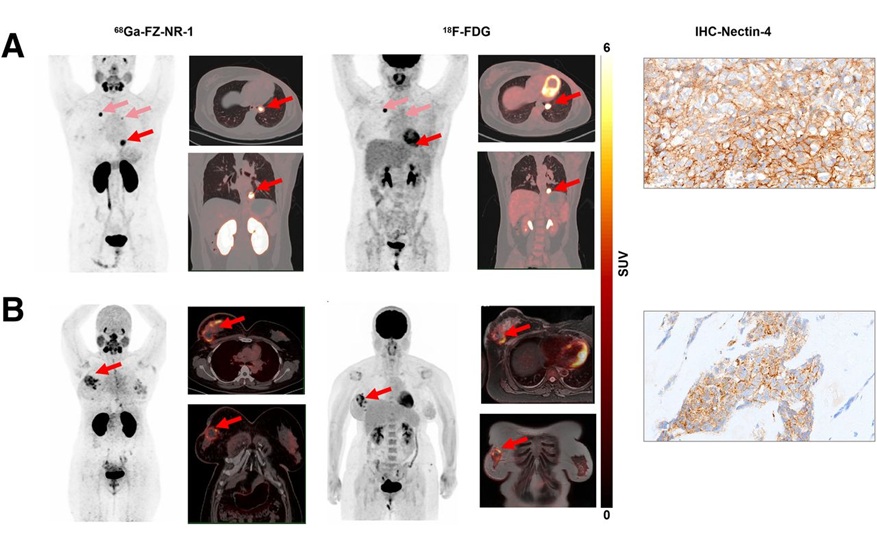
Novel Radiotracer Identifies Biomarker for Triple-Negative Breast Cancer
Triple-negative breast cancer (TNBC), which represents 15-20% of all breast cancer cases, is one of the most aggressive subtypes, with a five-year survival rate of about 40%. Due to its significant heterogeneity... Read moreImaging IT
view channel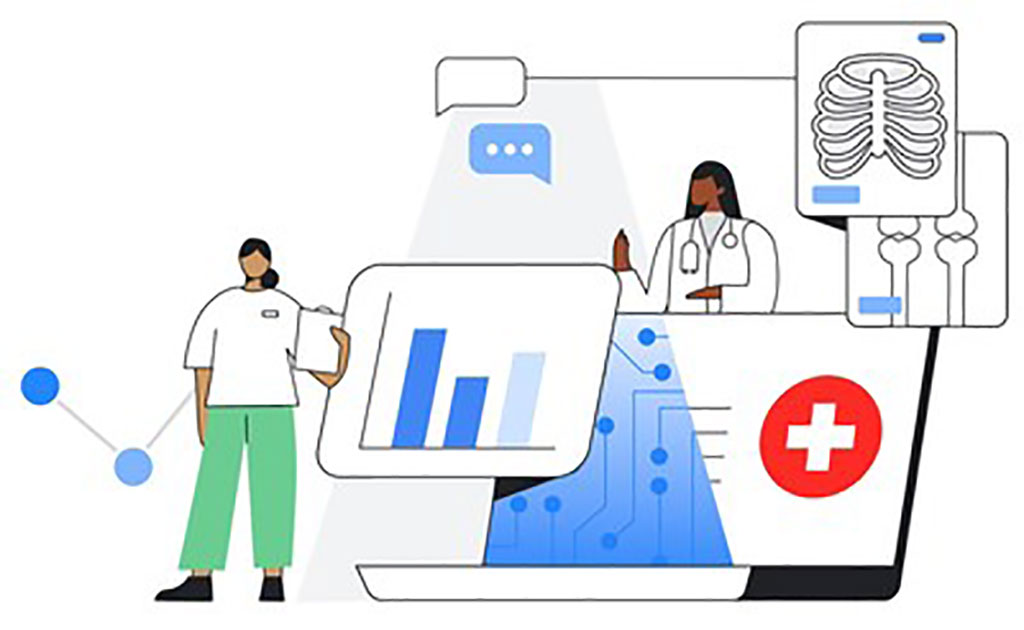
New Google Cloud Medical Imaging Suite Makes Imaging Healthcare Data More Accessible
Medical imaging is a critical tool used to diagnose patients, and there are billions of medical images scanned globally each year. Imaging data accounts for about 90% of all healthcare data1 and, until... Read more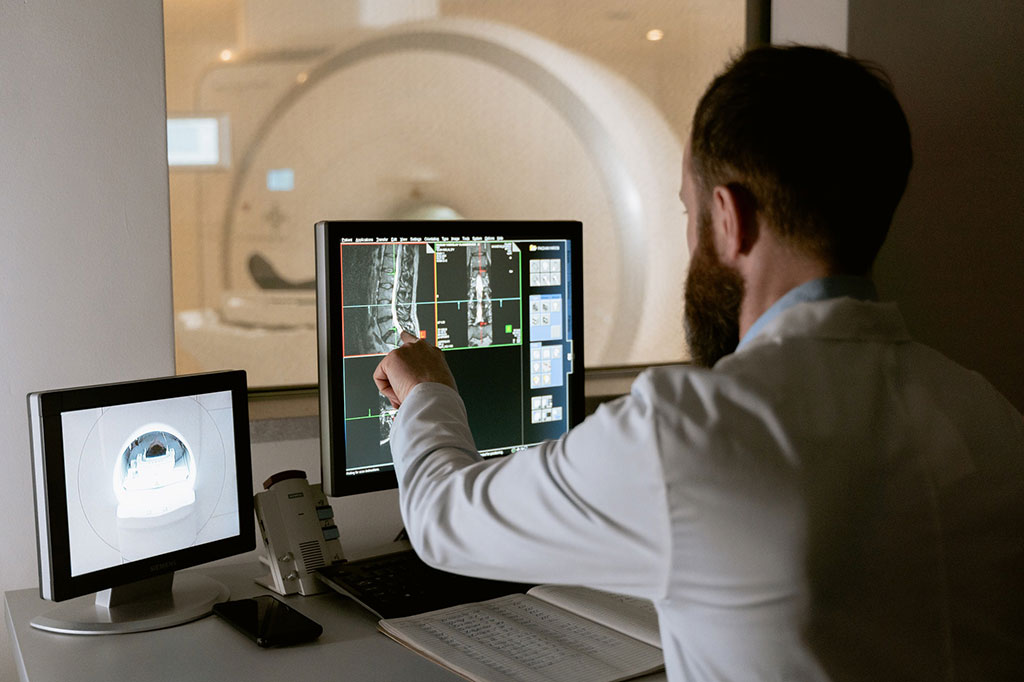
Global AI in Medical Diagnostics Market to Be Driven by Demand for Image Recognition in Radiology
The global artificial intelligence (AI) in medical diagnostics market is expanding with early disease detection being one of its key applications and image recognition becoming a compelling consumer proposition... Read moreIndustry News
view channel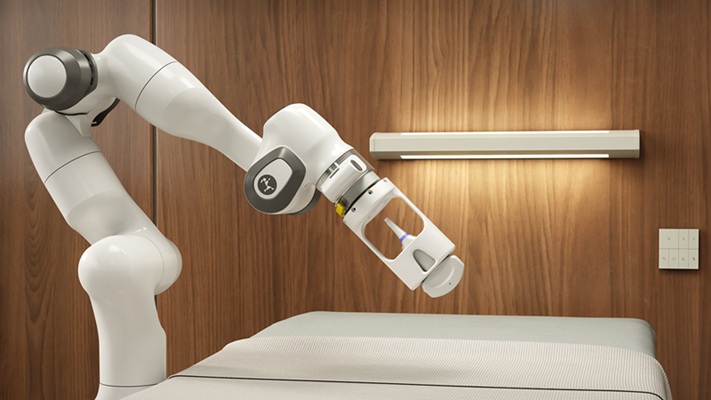
GE HealthCare and NVIDIA Collaboration to Reimagine Diagnostic Imaging
GE HealthCare (Chicago, IL, USA) has entered into a collaboration with NVIDIA (Santa Clara, CA, USA), expanding the existing relationship between the two companies to focus on pioneering innovation in... Read more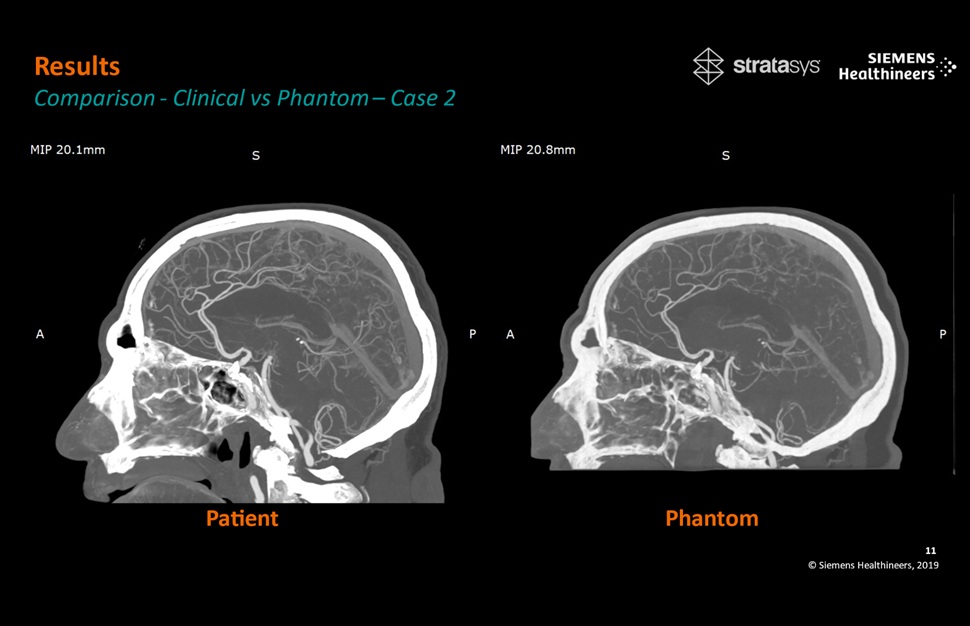
Patient-Specific 3D-Printed Phantoms Transform CT Imaging
New research has highlighted how anatomically precise, patient-specific 3D-printed phantoms are proving to be scalable, cost-effective, and efficient tools in the development of new CT scan algorithms... Read more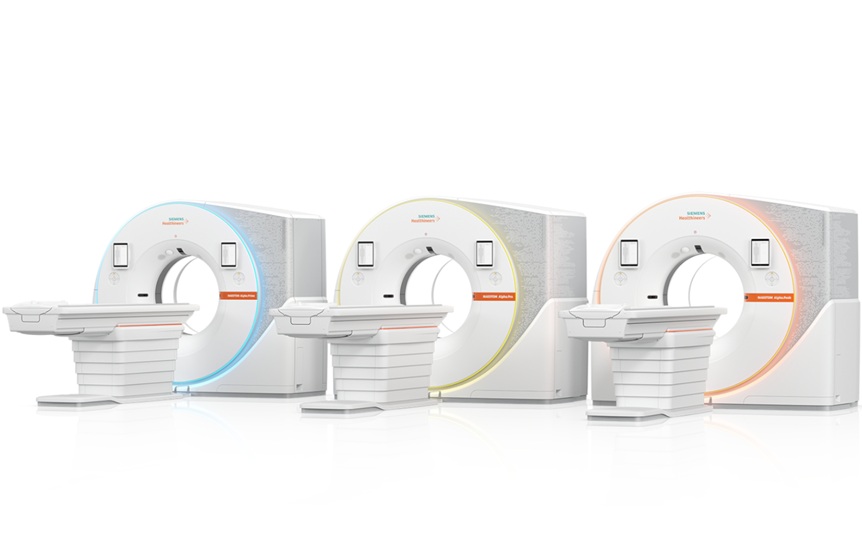
Siemens and Sectra Collaborate on Enhancing Radiology Workflows
Siemens Healthineers (Forchheim, Germany) and Sectra (Linköping, Sweden) have entered into a collaboration aimed at enhancing radiologists' diagnostic capabilities and, in turn, improving patient care... Read more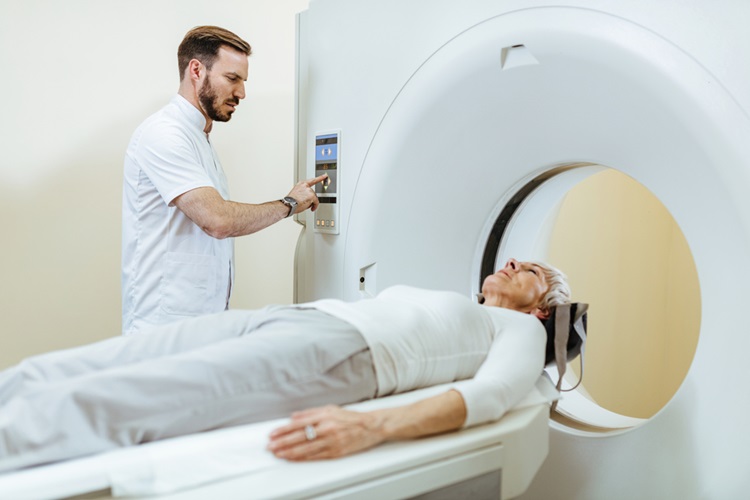