Deep Learning Outperforms Standard Machine Learning at Brain Imaging Analysis, Finds New Study
By MedImaging International staff writers Posted on 20 Jan 2021 |
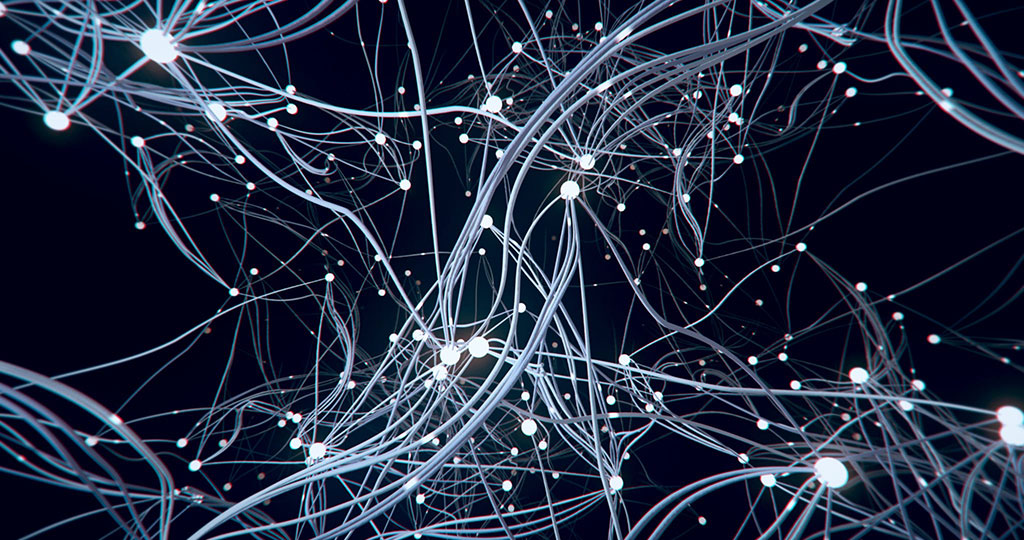
Illustration
Compared to standard machine learning models, deep learning models are largely superior at discerning patterns and discriminative features in brain imaging, despite being more complex in their architecture, according to a new study.
At the Center for Translational Research in Neuroimaging and Data Science (TReNDS; Atlanta, GA, USA), researchers are using deep learning to learn more about how mental illness and other disorders affect the brain. Advanced biomedical technologies such as structural and functional magnetic resonance imaging (MRI and fMRI) or genomic sequencing have produced an enormous volume of data about the human body. By extracting patterns from this information, scientists can glean new insights into health and disease. This is a challenging task, however, given the complexity of the data and the fact that the relationships among types of data are poorly understood. Deep learning, built on advanced neural networks, can characterize these relationships by combining and analyzing data from many sources.
Although deep learning models have been used to solve problems and answer questions in a number of different fields, some experts remain skeptical. Recent critical commentaries have unfavorably compared deep learning with standard machine learning approaches for analyzing brain imaging data. However, as demonstrated in the study, these conclusions are often based on pre-processed input that deprive deep learning of its main advantage—the ability to learn from the data with little to no preprocessing. In a comparative study of representative models from classical machine learning and deep learning, the researchers found that if trained properly, the deep-learning methods have the potential to offer substantially better results, generating superior representations for characterizing the human brain.
In some cases, the researchers found that standard machine learning can outperform deep learning. For example, diagnostic algorithms that plug in single-number measurements such as a patient’s body temperature or whether the patient smokes cigarettes would work better using classical machine learning approaches. The downside of deep learning models is they are “data hungry” at the outset and must be trained on lots of information. But once these models are trained, they are just as effective at analyzing reams of complex data as they are at answering simple questions.
Another advantage is that scientists can reverse analyze deep-learning models to understand how they are reaching conclusions about the data. As the published study shows, the trained deep learning models learn to identify meaningful brain biomarkers. The researchers envision that deep learning models are capable of extracting explanations and representations not already known to the field and act as an aid in growing our knowledge of how the human brain functions. They conclude that although more research is needed to find and address weaknesses of deep-learning models, from a mathematical point of view, it’s clear these models outperform standard machine learning models in many settings.
“We compared these models side-by-side, observing statistical protocols so everything is apples to apples. And we show that deep learning models perform better, as expected,” said co-author Sergey Plis, director of machine learning at TReNDS and associate professor of computer science. “Deep learning’s promise perhaps still outweighs its current usefulness to neuroimaging, but we are seeing a lot of real potential for these techniques.”
Related Links:
Center for Translational Research in Neuroimaging and Data Science
At the Center for Translational Research in Neuroimaging and Data Science (TReNDS; Atlanta, GA, USA), researchers are using deep learning to learn more about how mental illness and other disorders affect the brain. Advanced biomedical technologies such as structural and functional magnetic resonance imaging (MRI and fMRI) or genomic sequencing have produced an enormous volume of data about the human body. By extracting patterns from this information, scientists can glean new insights into health and disease. This is a challenging task, however, given the complexity of the data and the fact that the relationships among types of data are poorly understood. Deep learning, built on advanced neural networks, can characterize these relationships by combining and analyzing data from many sources.
Although deep learning models have been used to solve problems and answer questions in a number of different fields, some experts remain skeptical. Recent critical commentaries have unfavorably compared deep learning with standard machine learning approaches for analyzing brain imaging data. However, as demonstrated in the study, these conclusions are often based on pre-processed input that deprive deep learning of its main advantage—the ability to learn from the data with little to no preprocessing. In a comparative study of representative models from classical machine learning and deep learning, the researchers found that if trained properly, the deep-learning methods have the potential to offer substantially better results, generating superior representations for characterizing the human brain.
In some cases, the researchers found that standard machine learning can outperform deep learning. For example, diagnostic algorithms that plug in single-number measurements such as a patient’s body temperature or whether the patient smokes cigarettes would work better using classical machine learning approaches. The downside of deep learning models is they are “data hungry” at the outset and must be trained on lots of information. But once these models are trained, they are just as effective at analyzing reams of complex data as they are at answering simple questions.
Another advantage is that scientists can reverse analyze deep-learning models to understand how they are reaching conclusions about the data. As the published study shows, the trained deep learning models learn to identify meaningful brain biomarkers. The researchers envision that deep learning models are capable of extracting explanations and representations not already known to the field and act as an aid in growing our knowledge of how the human brain functions. They conclude that although more research is needed to find and address weaknesses of deep-learning models, from a mathematical point of view, it’s clear these models outperform standard machine learning models in many settings.
“We compared these models side-by-side, observing statistical protocols so everything is apples to apples. And we show that deep learning models perform better, as expected,” said co-author Sergey Plis, director of machine learning at TReNDS and associate professor of computer science. “Deep learning’s promise perhaps still outweighs its current usefulness to neuroimaging, but we are seeing a lot of real potential for these techniques.”
Related Links:
Center for Translational Research in Neuroimaging and Data Science
Latest Industry News News
- Bayer and Google Partner on New AI Product for Radiologists
- Samsung and Bracco Enter Into New Diagnostic Ultrasound Technology Agreement
- IBA Acquires Radcal to Expand Medical Imaging Quality Assurance Offering
- International Societies Suggest Key Considerations for AI Radiology Tools
- Samsung's X-Ray Devices to Be Powered by Lunit AI Solutions for Advanced Chest Screening
- Canon Medical and Olympus Collaborate on Endoscopic Ultrasound Systems
- GE HealthCare Acquires AI Imaging Analysis Company MIM Software
- First Ever International Criteria Lays Foundation for Improved Diagnostic Imaging of Brain Tumors
- RSNA Unveils 10 Most Cited Radiology Studies of 2023
- RSNA 2023 Technical Exhibits to Offer Innovations in AI, 3D Printing and More
- AI Medical Imaging Products to Increase Five-Fold by 2035, Finds Study
- RSNA 2023 Technical Exhibits to Highlight Latest Medical Imaging Innovations
- AI-Powered Technologies to Aid Interpretation of X-Ray and MRI Images for Improved Disease Diagnosis
- Hologic and Bayer Partner to Improve Mammography Imaging
- Global Fixed and Mobile C-Arms Market Driven by Increasing Surgical Procedures
- Global Contrast Enhanced Ultrasound Market Driven by Demand for Early Detection of Chronic Diseases
Channels
Radiography
view channel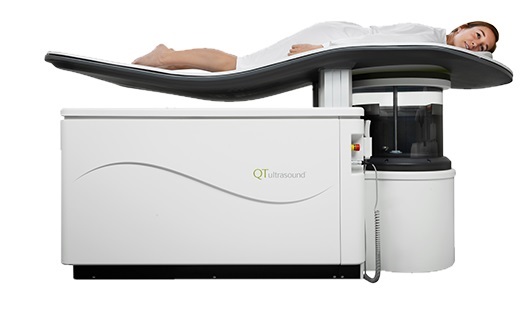
Novel Breast Imaging System Proves As Effective As Mammography
Breast cancer remains the most frequently diagnosed cancer among women. It is projected that one in eight women will be diagnosed with breast cancer during her lifetime, and one in 42 women who turn 50... Read more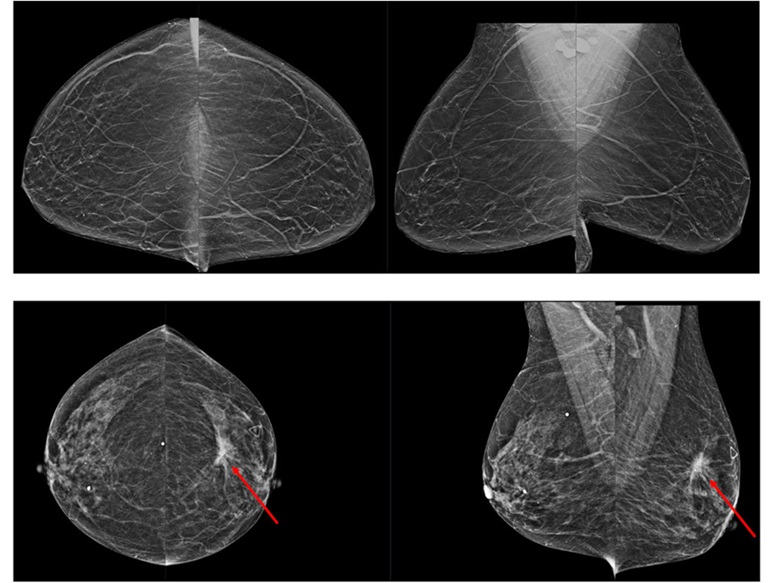
AI Assistance Improves Breast-Cancer Screening by Reducing False Positives
Radiologists typically detect one case of cancer for every 200 mammograms reviewed. However, these evaluations often result in false positives, leading to unnecessary patient recalls for additional testing,... Read moreMRI
view channel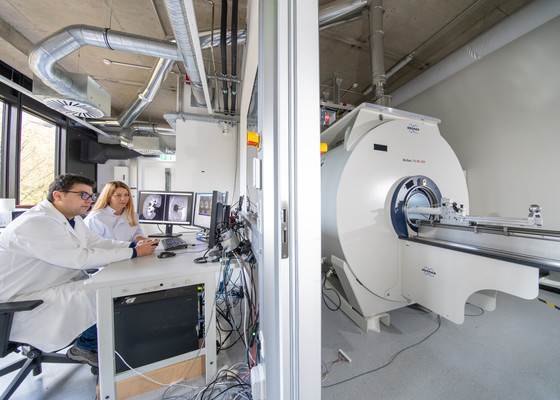
Diamond Dust Could Offer New Contrast Agent Option for Future MRI Scans
Gadolinium, a heavy metal used for over three decades as a contrast agent in medical imaging, enhances the clarity of MRI scans by highlighting affected areas. Despite its utility, gadolinium not only... Read more.jpg)
Combining MRI with PSA Testing Improves Clinical Outcomes for Prostate Cancer Patients
Prostate cancer is a leading health concern globally, consistently being one of the most common types of cancer among men and a major cause of cancer-related deaths. In the United States, it is the most... Read more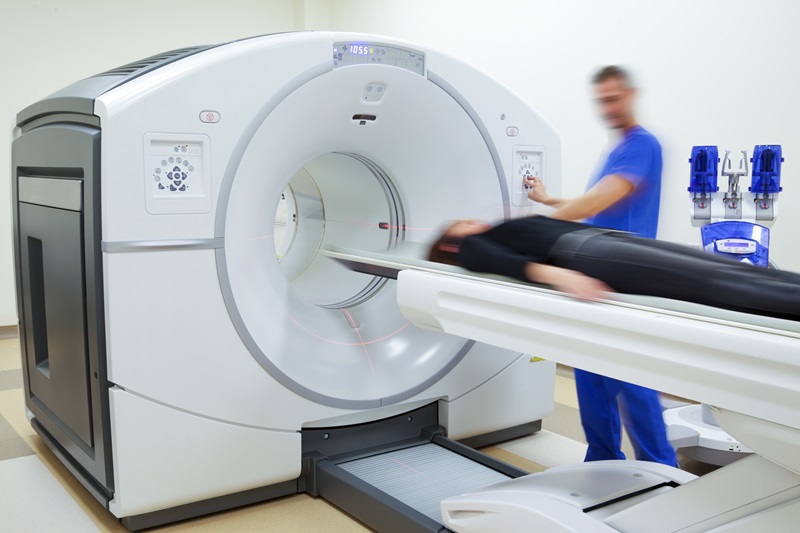
PET/MRI Improves Diagnostic Accuracy for Prostate Cancer Patients
The Prostate Imaging Reporting and Data System (PI-RADS) is a five-point scale to assess potential prostate cancer in MR images. PI-RADS category 3 which offers an unclear suggestion of clinically significant... Read more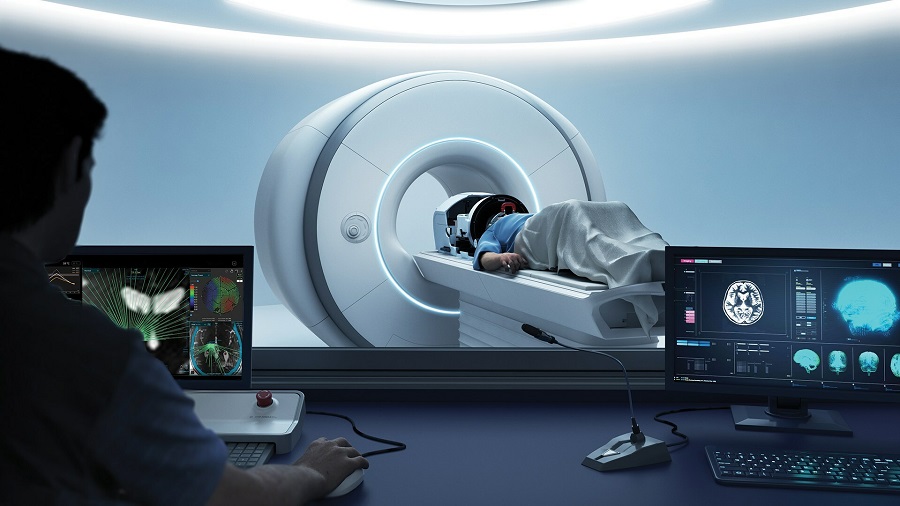
Next Generation MR-Guided Focused Ultrasound Ushers In Future of Incisionless Neurosurgery
Essential tremor, often called familial, idiopathic, or benign tremor, leads to uncontrollable shaking that significantly affects a person’s life. When traditional medications do not alleviate symptoms,... Read moreUltrasound
view channel.jpg)
Groundbreaking Technology Enables Precise, Automatic Measurement of Peripheral Blood Vessels
The current standard of care of using angiographic information is often inadequate for accurately assessing vessel size in the estimated 20 million people in the U.S. who suffer from peripheral vascular disease.... Read more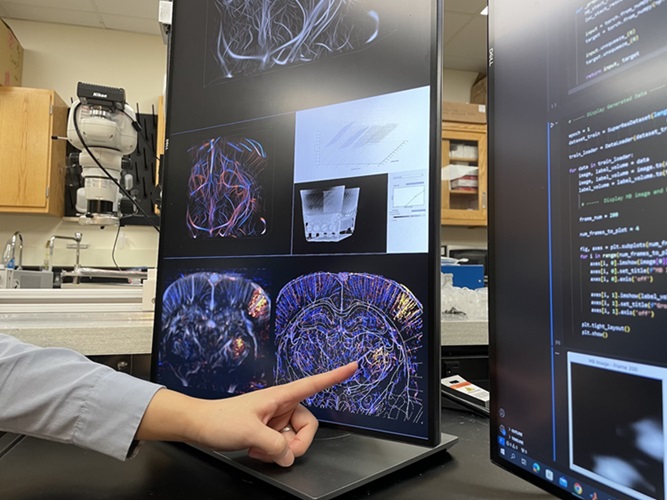
Deep Learning Advances Super-Resolution Ultrasound Imaging
Ultrasound localization microscopy (ULM) is an advanced imaging technique that offers high-resolution visualization of microvascular structures. It employs microbubbles, FDA-approved contrast agents, injected... Read more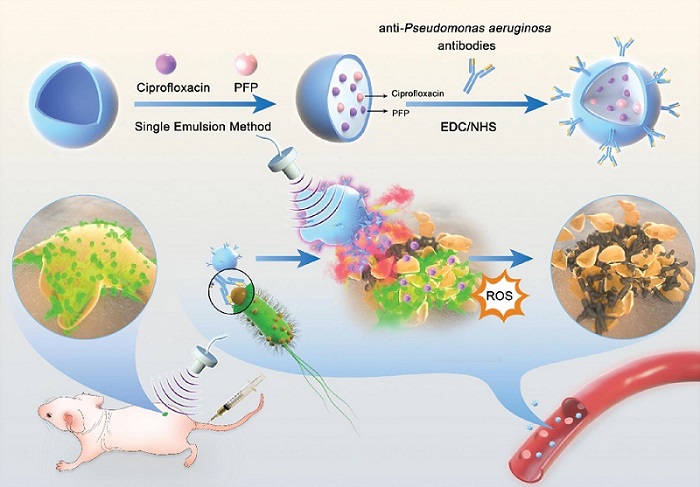
Novel Ultrasound-Launched Targeted Nanoparticle Eliminates Biofilm and Bacterial Infection
Biofilms, formed by bacteria aggregating into dense communities for protection against harsh environmental conditions, are a significant contributor to various infectious diseases. Biofilms frequently... Read moreNuclear Medicine
view channel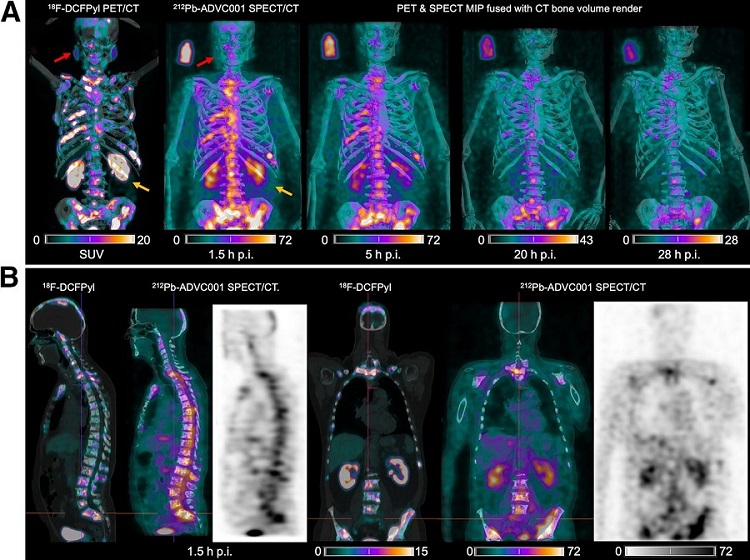
New SPECT/CT Technique Could Change Imaging Practices and Increase Patient Access
The development of lead-212 (212Pb)-PSMA–based targeted alpha therapy (TAT) is garnering significant interest in treating patients with metastatic castration-resistant prostate cancer. The imaging of 212Pb,... Read more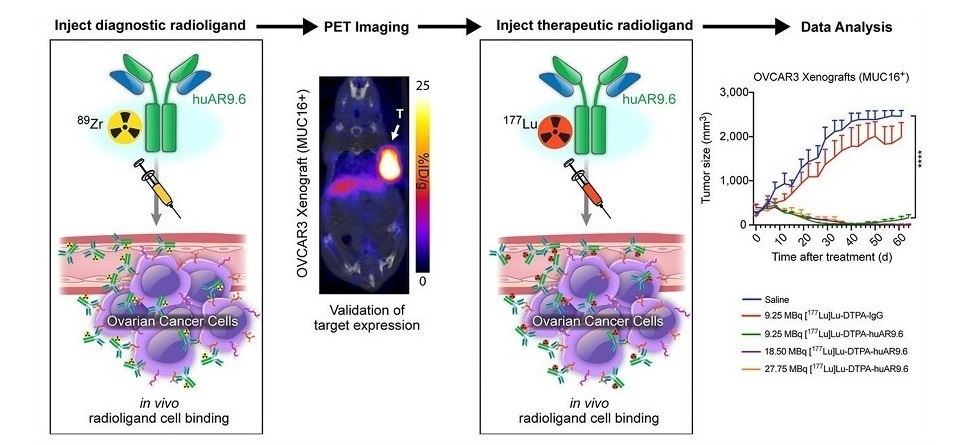
New Radiotheranostic System Detects and Treats Ovarian Cancer Noninvasively
Ovarian cancer is the most lethal gynecological cancer, with less than a 30% five-year survival rate for those diagnosed in late stages. Despite surgery and platinum-based chemotherapy being the standard... Read more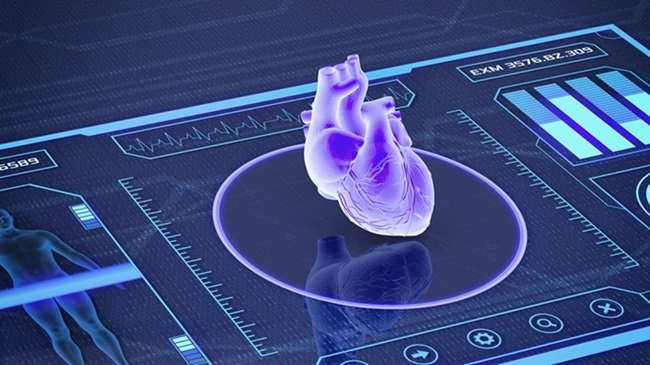
AI System Automatically and Reliably Detects Cardiac Amyloidosis Using Scintigraphy Imaging
Cardiac amyloidosis, a condition characterized by the buildup of abnormal protein deposits (amyloids) in the heart muscle, severely affects heart function and can lead to heart failure or death without... Read moreGeneral/Advanced Imaging
view channel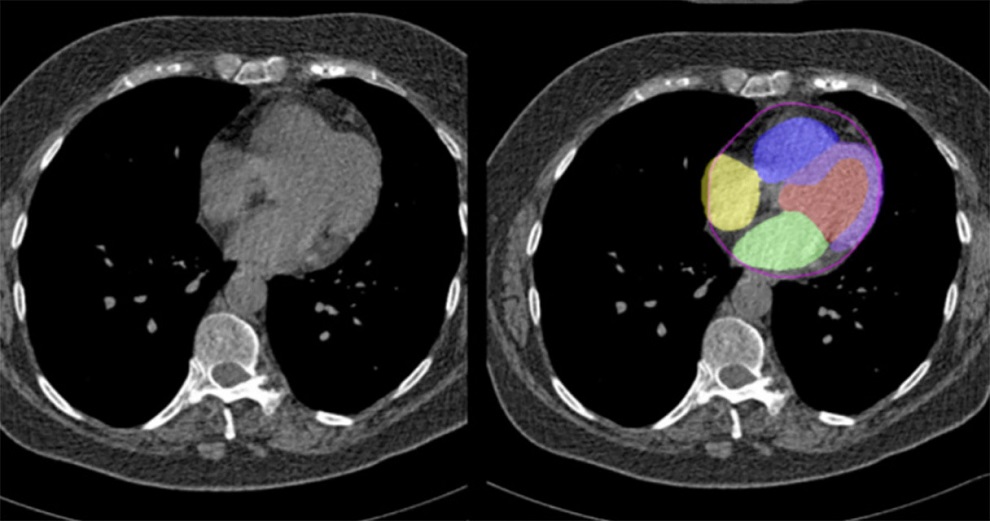
Artificial Intelligence Evaluates Cardiovascular Risk from CT Scans
Chest computed tomography (CT) is a common diagnostic tool, with approximately 15 million scans conducted each year in the United States, though many are underutilized or not fully explored.... Read more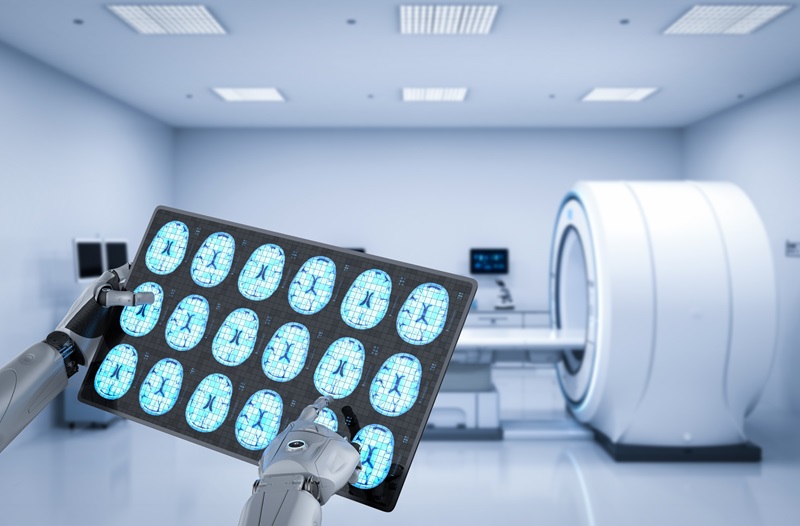
New AI Method Captures Uncertainty in Medical Images
In the field of biomedicine, segmentation is the process of annotating pixels from an important structure in medical images, such as organs or cells. Artificial Intelligence (AI) models are utilized to... Read more.jpg)
CT Coronary Angiography Reduces Need for Invasive Tests to Diagnose Coronary Artery Disease
Coronary artery disease (CAD), one of the leading causes of death worldwide, involves the narrowing of coronary arteries due to atherosclerosis, resulting in insufficient blood flow to the heart muscle.... Read more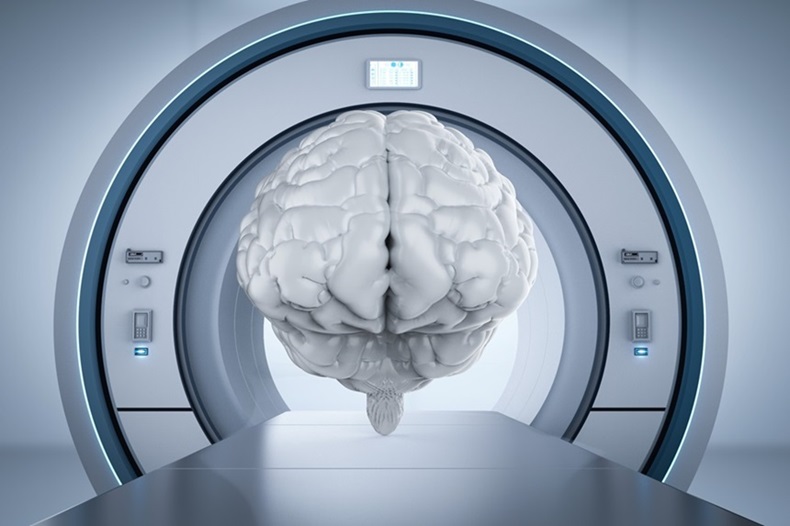
Novel Blood Test Could Reduce Need for PET Imaging of Patients with Alzheimer’s
Alzheimer's disease (AD), a condition marked by cognitive decline and the presence of beta-amyloid (Aβ) plaques and neurofibrillary tangles in the brain, poses diagnostic challenges. Amyloid positron emission... Read moreImaging IT
view channel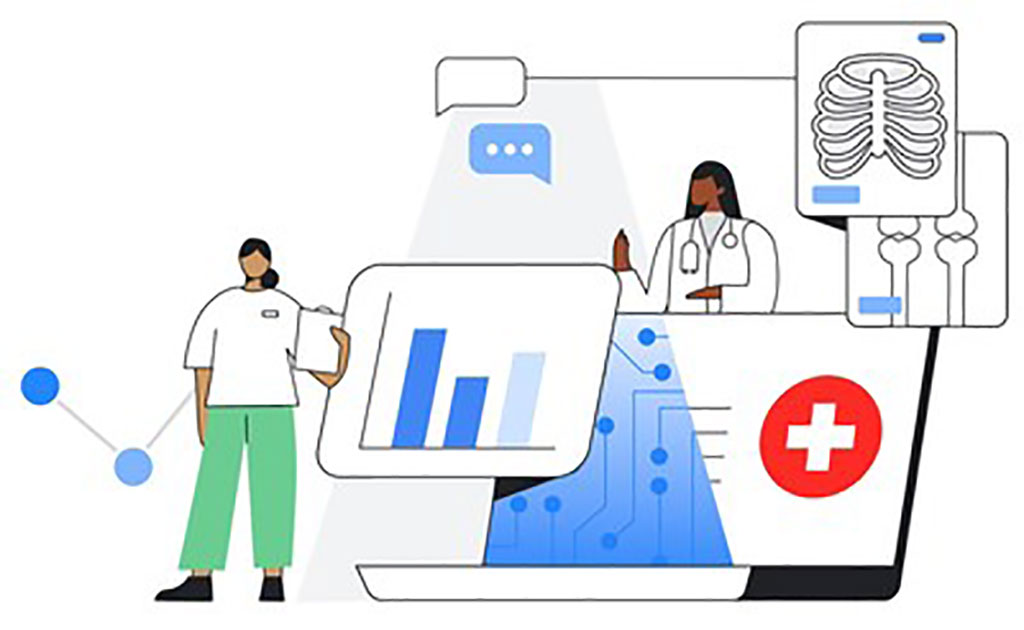
New Google Cloud Medical Imaging Suite Makes Imaging Healthcare Data More Accessible
Medical imaging is a critical tool used to diagnose patients, and there are billions of medical images scanned globally each year. Imaging data accounts for about 90% of all healthcare data1 and, until... Read more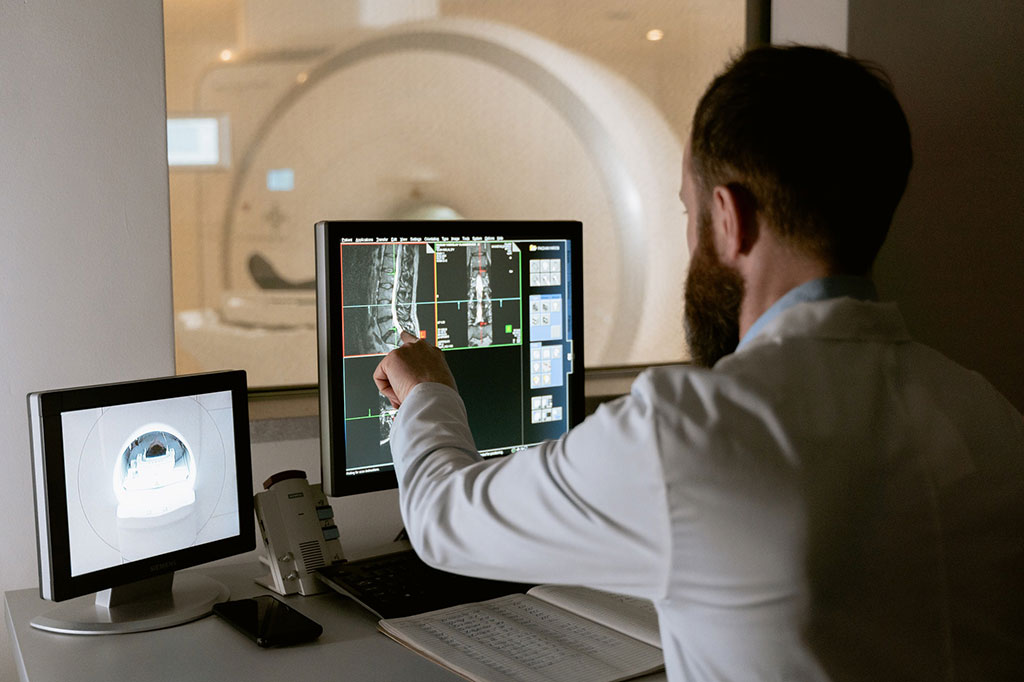