Machine Learning-Aided Tool Generates High-Quality Chest X-Ray Images to Diagnose COVID-19 More Accurately
By MedImaging International staff writers Posted on 15 Dec 2020 |
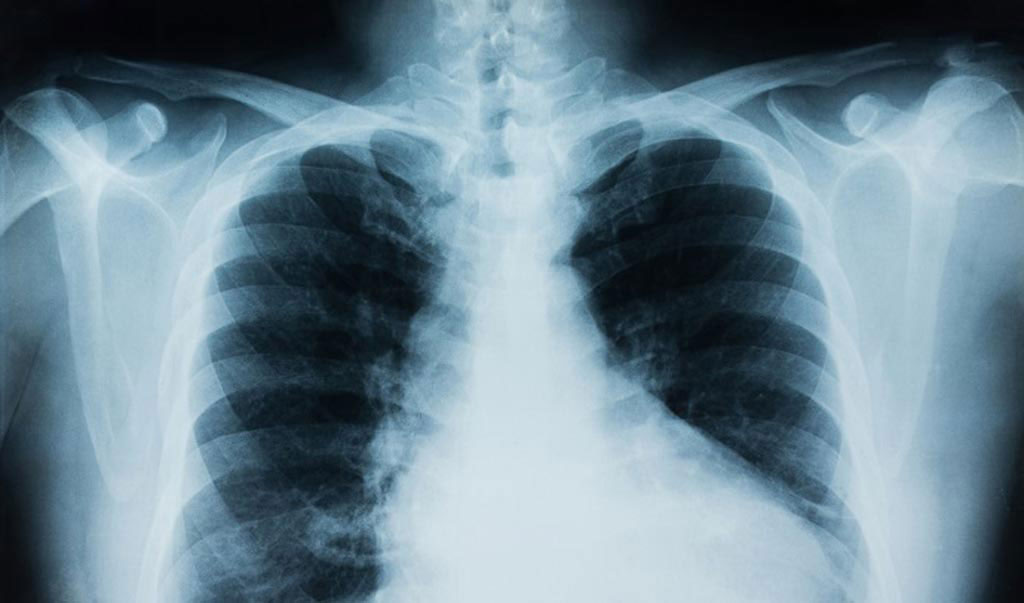
Illustration
A new method of generating high-quality chest X-ray images can be used to diagnose COVID-19 more accurately than current methods.
The team of researchers at the University of Maryland, Baltimore County (UMBC; Baltimore, MD, USA) has published its findings in the proceedings of the IEEE Big Data 2020 Conference. The need for rapid and accurate COVID-19 testing is high, including testing that can determine if COVID-19 is impacting a patient's respiratory system. Many clinicians use X-ray technology to classify images of possible cases of COVID-19, but the limited data available makes it more challenging to classify those images accurately.
The UMBC researchers developed their tool as an extension of generative adversarial networks (GANs) - machine learning frameworks that can quickly generate new data based on statistics from a training set. The team's more advanced method uses what they call Mean Teacher + Transfer Generative Adversarial Networks (MTT-GAN). The MTT-GANs are superior to GANs because the images they generate are much more similar to authentic images generated by x-ray machines. The MTT-GAN classification system has the potential to help improve the accuracy of COVID-19 classifiers, making it an important diagnostic tool for physicians who are still working to understand the range of ways this complex disease presents in patients.
"The availability of data is one of the most important aspects of machine learning and our research has taken an incremental theoretical step towards generating data using the MTT-GAN," said Sumeet Menon, a Ph.D. student in computer science at UMBC who led the research team. "This paper mainly focuses on generating more COVID-19 X-rays using the MTT-GAN, which could be widely used to train machine learning models and could have many applications, including classification of CT-scans and segmentation."
Related Links:
University of Maryland, Baltimore County
The team of researchers at the University of Maryland, Baltimore County (UMBC; Baltimore, MD, USA) has published its findings in the proceedings of the IEEE Big Data 2020 Conference. The need for rapid and accurate COVID-19 testing is high, including testing that can determine if COVID-19 is impacting a patient's respiratory system. Many clinicians use X-ray technology to classify images of possible cases of COVID-19, but the limited data available makes it more challenging to classify those images accurately.
The UMBC researchers developed their tool as an extension of generative adversarial networks (GANs) - machine learning frameworks that can quickly generate new data based on statistics from a training set. The team's more advanced method uses what they call Mean Teacher + Transfer Generative Adversarial Networks (MTT-GAN). The MTT-GANs are superior to GANs because the images they generate are much more similar to authentic images generated by x-ray machines. The MTT-GAN classification system has the potential to help improve the accuracy of COVID-19 classifiers, making it an important diagnostic tool for physicians who are still working to understand the range of ways this complex disease presents in patients.
"The availability of data is one of the most important aspects of machine learning and our research has taken an incremental theoretical step towards generating data using the MTT-GAN," said Sumeet Menon, a Ph.D. student in computer science at UMBC who led the research team. "This paper mainly focuses on generating more COVID-19 X-rays using the MTT-GAN, which could be widely used to train machine learning models and could have many applications, including classification of CT-scans and segmentation."
Related Links:
University of Maryland, Baltimore County
Latest Radiography News
- Novel Breast Imaging System Proves As Effective As Mammography
- AI Assistance Improves Breast-Cancer Screening by Reducing False Positives
- AI Could Boost Clinical Adoption of Chest DDR
- 3D Mammography Almost Halves Breast Cancer Incidence between Two Screening Tests
- AI Model Predicts 5-Year Breast Cancer Risk from Mammograms
- Deep Learning Framework Detects Fractures in X-Ray Images With 99% Accuracy
- Direct AI-Based Medical X-Ray Imaging System a Paradigm-Shift from Conventional DR and CT
- Chest X-Ray AI Solution Automatically Identifies, Categorizes and Highlights Suspicious Areas
- AI Diagnoses Wrist Fractures As Well As Radiologists
- Annual Mammography Beginning At 40 Cuts Breast Cancer Mortality By 42%
- 3D Human GPS Powered By Light Paves Way for Radiation-Free Minimally-Invasive Surgery
- Novel AI Technology to Revolutionize Cancer Detection in Dense Breasts
- AI Solution Provides Radiologists with 'Second Pair' Of Eyes to Detect Breast Cancers
- AI Helps General Radiologists Achieve Specialist-Level Performance in Interpreting Mammograms
- Novel Imaging Technique Could Transform Breast Cancer Detection
- Computer Program Combines AI and Heat-Imaging Technology for Early Breast Cancer Detection
Channels
Radiography
view channel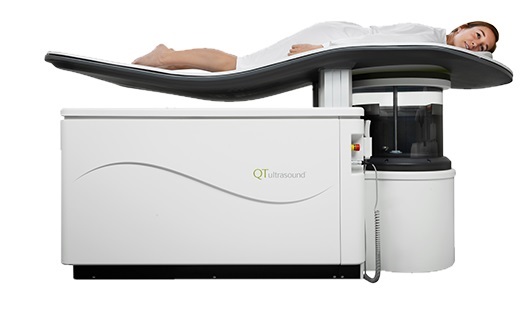
Novel Breast Imaging System Proves As Effective As Mammography
Breast cancer remains the most frequently diagnosed cancer among women. It is projected that one in eight women will be diagnosed with breast cancer during her lifetime, and one in 42 women who turn 50... Read more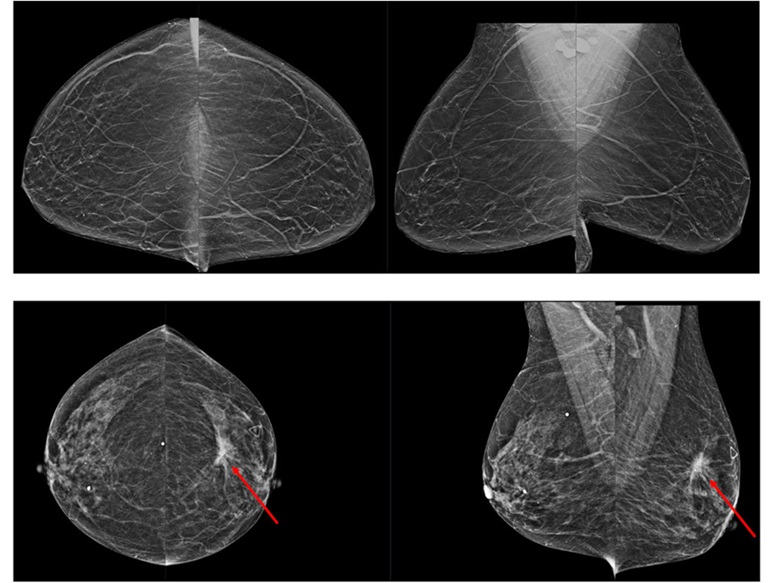
AI Assistance Improves Breast-Cancer Screening by Reducing False Positives
Radiologists typically detect one case of cancer for every 200 mammograms reviewed. However, these evaluations often result in false positives, leading to unnecessary patient recalls for additional testing,... Read moreMRI
view channel.jpg)
Combining MRI with PSA Testing Improves Clinical Outcomes for Prostate Cancer Patients
Prostate cancer is a leading health concern globally, consistently being one of the most common types of cancer among men and a major cause of cancer-related deaths. In the United States, it is the most... Read more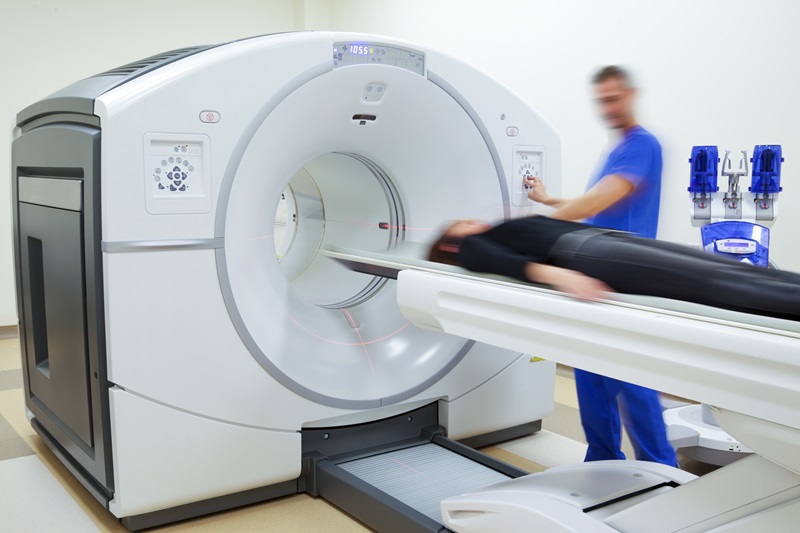
PET/MRI Improves Diagnostic Accuracy for Prostate Cancer Patients
The Prostate Imaging Reporting and Data System (PI-RADS) is a five-point scale to assess potential prostate cancer in MR images. PI-RADS category 3 which offers an unclear suggestion of clinically significant... Read more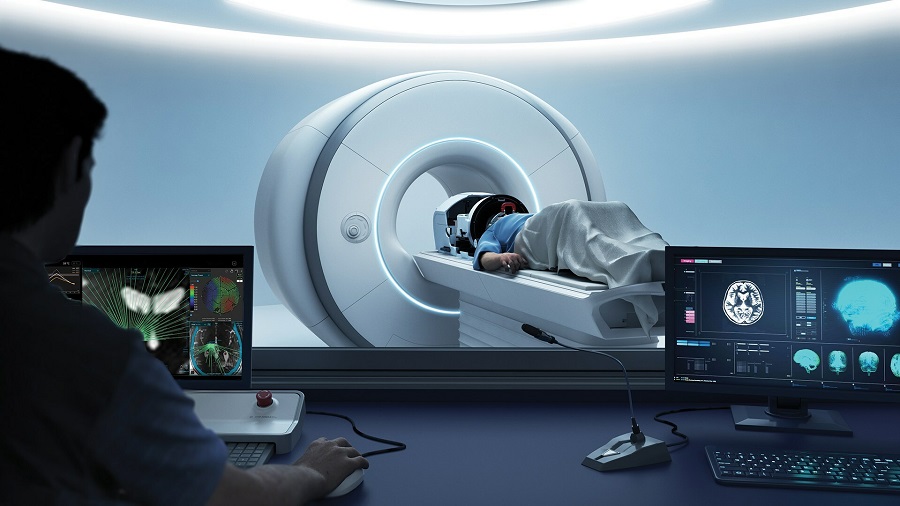
Next Generation MR-Guided Focused Ultrasound Ushers In Future of Incisionless Neurosurgery
Essential tremor, often called familial, idiopathic, or benign tremor, leads to uncontrollable shaking that significantly affects a person’s life. When traditional medications do not alleviate symptoms,... Read more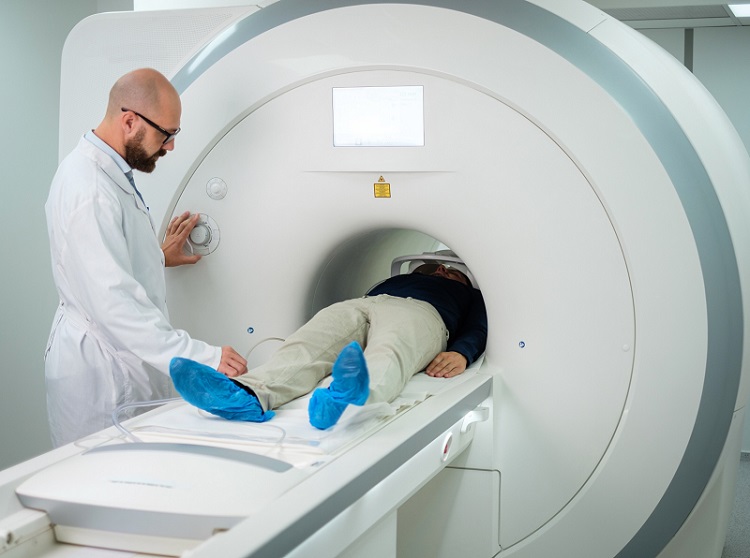
Two-Part MRI Scan Detects Prostate Cancer More Quickly without Compromising Diagnostic Quality
Prostate cancer ranks as the most prevalent cancer among men. Over the last decade, the introduction of MRI scans has significantly transformed the diagnosis process, marking the most substantial advancement... Read moreUltrasound
view channel.jpg)
Groundbreaking Technology Enables Precise, Automatic Measurement of Peripheral Blood Vessels
The current standard of care of using angiographic information is often inadequate for accurately assessing vessel size in the estimated 20 million people in the U.S. who suffer from peripheral vascular disease.... Read more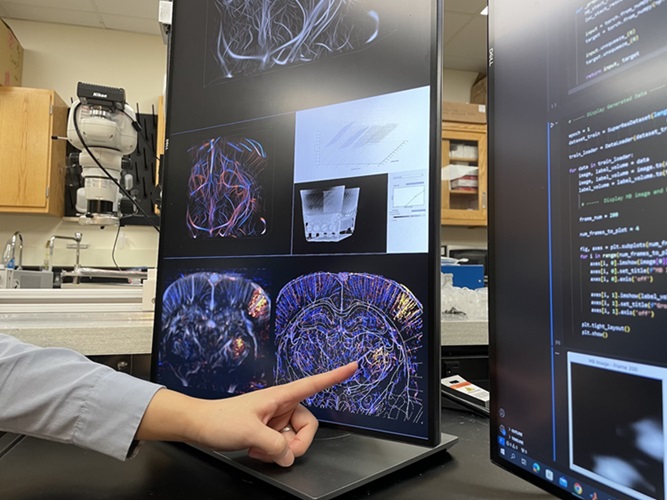
Deep Learning Advances Super-Resolution Ultrasound Imaging
Ultrasound localization microscopy (ULM) is an advanced imaging technique that offers high-resolution visualization of microvascular structures. It employs microbubbles, FDA-approved contrast agents, injected... Read more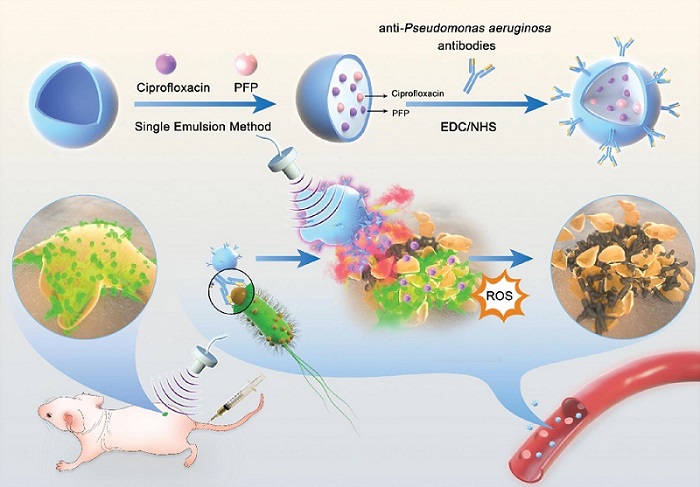
Novel Ultrasound-Launched Targeted Nanoparticle Eliminates Biofilm and Bacterial Infection
Biofilms, formed by bacteria aggregating into dense communities for protection against harsh environmental conditions, are a significant contributor to various infectious diseases. Biofilms frequently... Read moreNuclear Medicine
view channel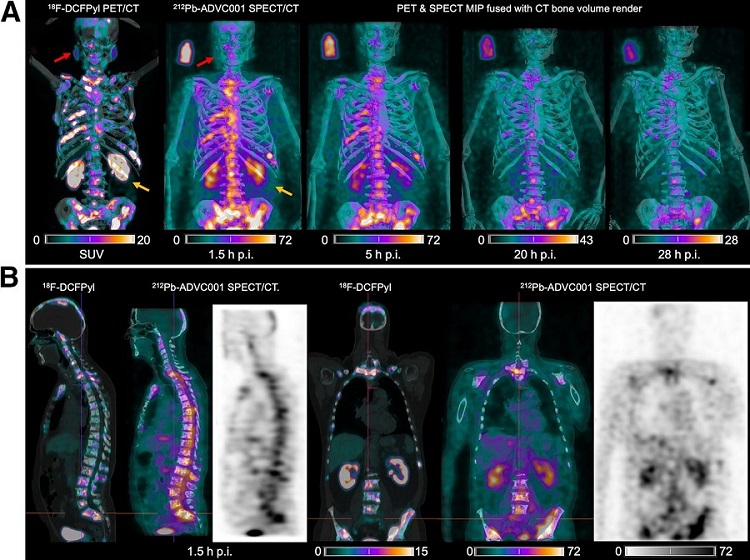
New SPECT/CT Technique Could Change Imaging Practices and Increase Patient Access
The development of lead-212 (212Pb)-PSMA–based targeted alpha therapy (TAT) is garnering significant interest in treating patients with metastatic castration-resistant prostate cancer. The imaging of 212Pb,... Read more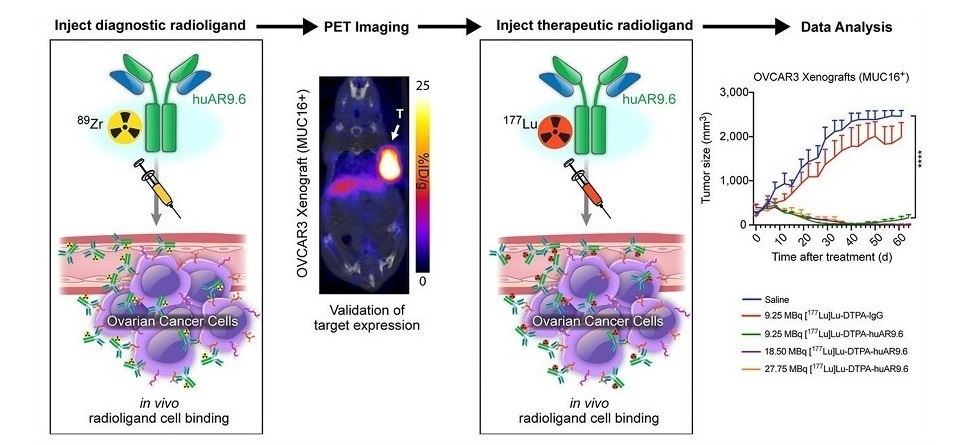
New Radiotheranostic System Detects and Treats Ovarian Cancer Noninvasively
Ovarian cancer is the most lethal gynecological cancer, with less than a 30% five-year survival rate for those diagnosed in late stages. Despite surgery and platinum-based chemotherapy being the standard... Read more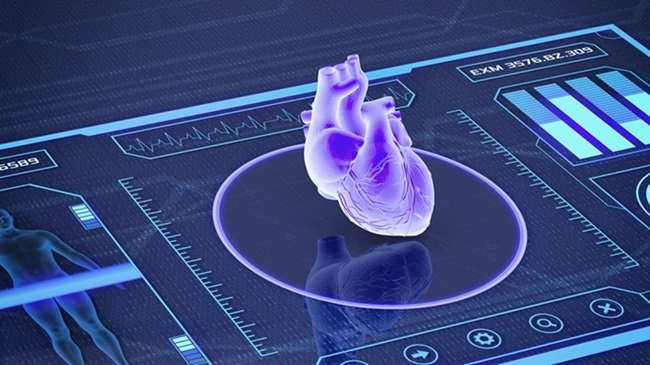
AI System Automatically and Reliably Detects Cardiac Amyloidosis Using Scintigraphy Imaging
Cardiac amyloidosis, a condition characterized by the buildup of abnormal protein deposits (amyloids) in the heart muscle, severely affects heart function and can lead to heart failure or death without... Read moreGeneral/Advanced Imaging
view channel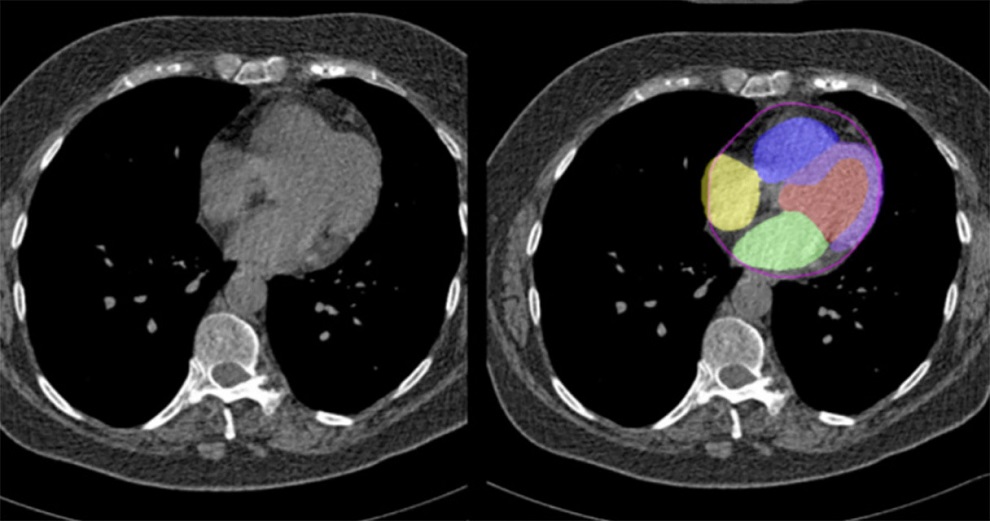
Artificial Intelligence Evaluates Cardiovascular Risk from CT Scans
Chest computed tomography (CT) is a common diagnostic tool, with approximately 15 million scans conducted each year in the United States, though many are underutilized or not fully explored.... Read more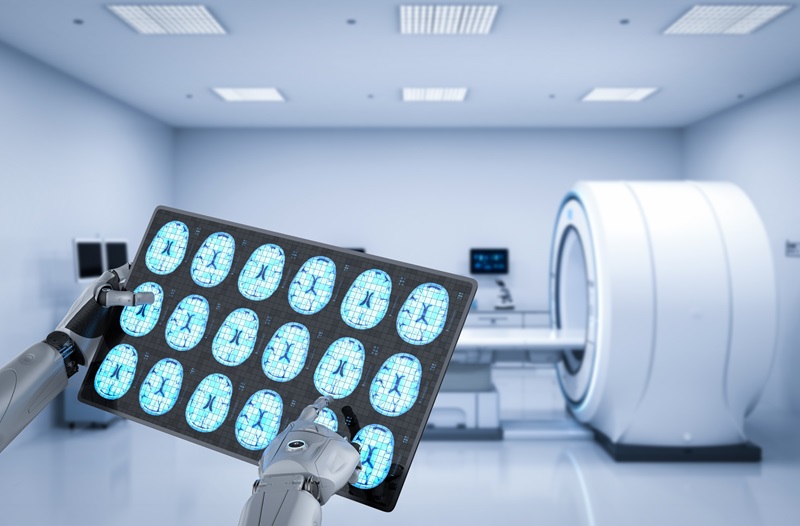
New AI Method Captures Uncertainty in Medical Images
In the field of biomedicine, segmentation is the process of annotating pixels from an important structure in medical images, such as organs or cells. Artificial Intelligence (AI) models are utilized to... Read more.jpg)
CT Coronary Angiography Reduces Need for Invasive Tests to Diagnose Coronary Artery Disease
Coronary artery disease (CAD), one of the leading causes of death worldwide, involves the narrowing of coronary arteries due to atherosclerosis, resulting in insufficient blood flow to the heart muscle.... Read more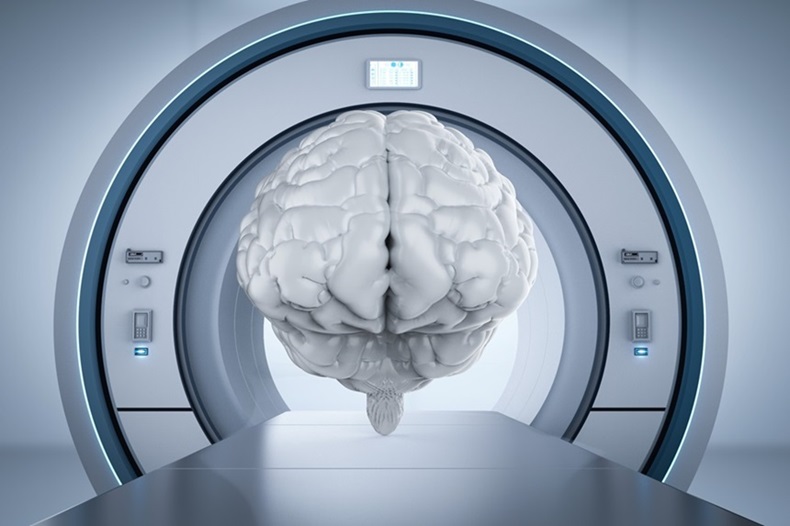
Novel Blood Test Could Reduce Need for PET Imaging of Patients with Alzheimer’s
Alzheimer's disease (AD), a condition marked by cognitive decline and the presence of beta-amyloid (Aβ) plaques and neurofibrillary tangles in the brain, poses diagnostic challenges. Amyloid positron emission... Read moreImaging IT
view channel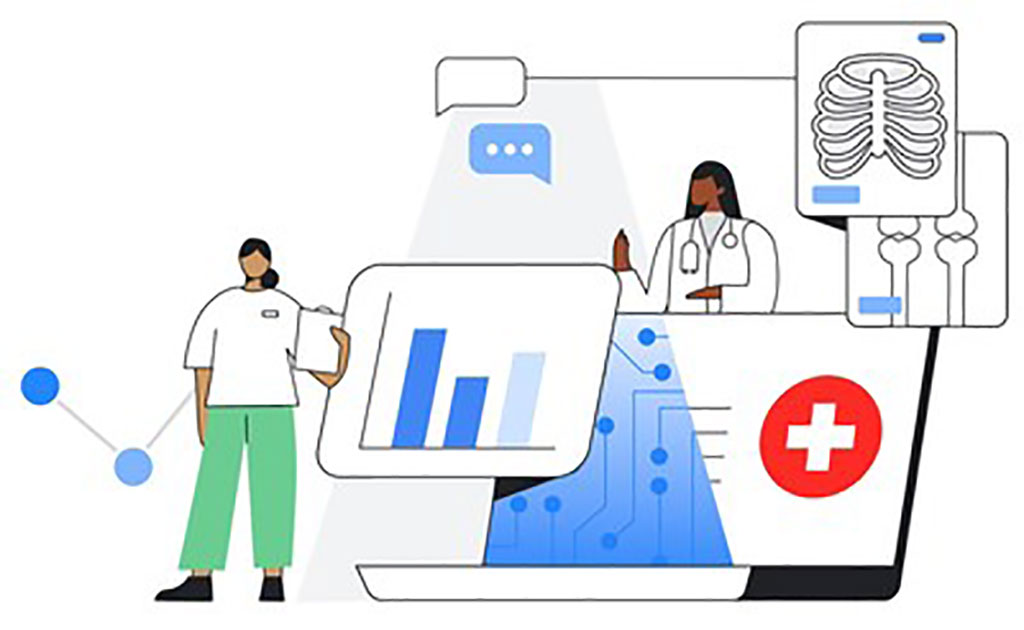
New Google Cloud Medical Imaging Suite Makes Imaging Healthcare Data More Accessible
Medical imaging is a critical tool used to diagnose patients, and there are billions of medical images scanned globally each year. Imaging data accounts for about 90% of all healthcare data1 and, until... Read more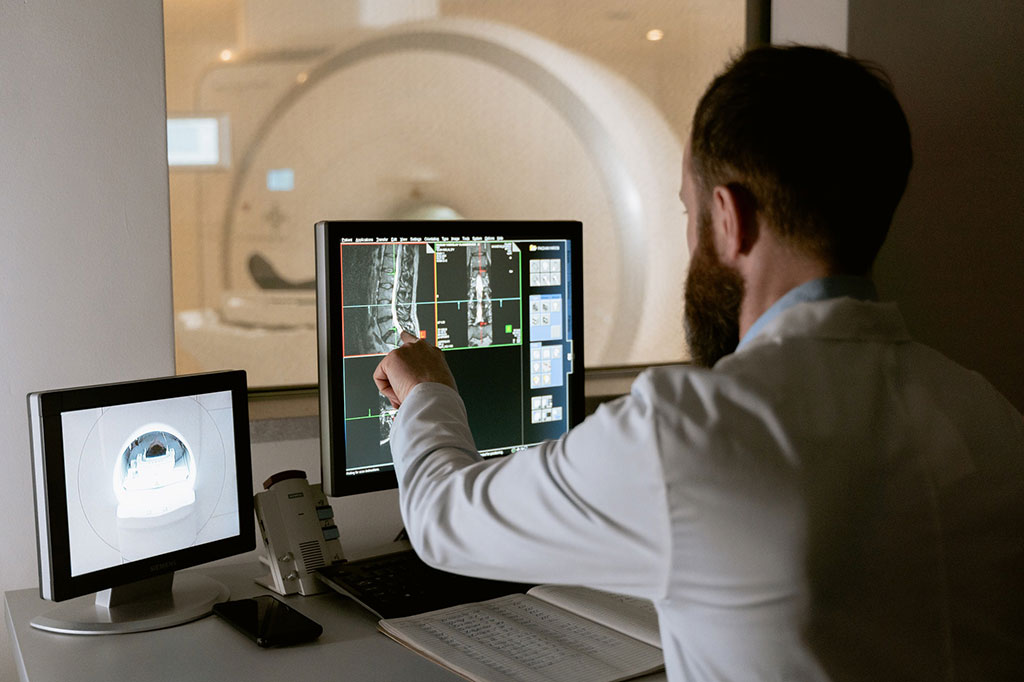
Global AI in Medical Diagnostics Market to Be Driven by Demand for Image Recognition in Radiology
The global artificial intelligence (AI) in medical diagnostics market is expanding with early disease detection being one of its key applications and image recognition becoming a compelling consumer proposition... Read moreIndustry News
view channel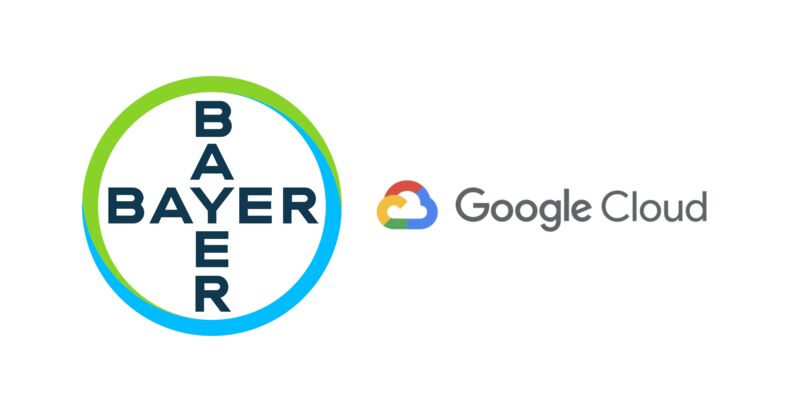
Bayer and Google Partner on New AI Product for Radiologists
Medical imaging data comprises around 90% of all healthcare data, and it is a highly complex and rich clinical data modality and serves as a vital tool for diagnosing patients. Each year, billions of medical... Read more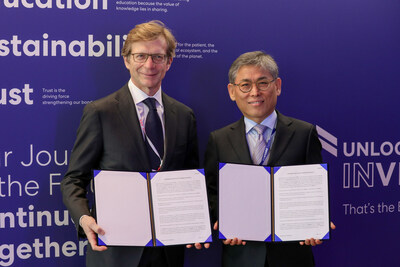