Deep Learning Framework Detects Fractures in X-Ray Images With 99% Accuracy
Posted on 26 Mar 2024
Globally, 1.7 billion people suffer from musculoskeletal conditions, which can cause significant pain and disability. These conditions often require quick and accurate diagnosis and treatment decisions, particularly in emergency scenarios. Although deep learning technologies have been explored for aiding medical decision-making, issues such as poor performance and opacity have hampered their effectiveness in identifying shoulder-related problems like fractures, arthritis, or deformities in X-ray images. Now, scientists have created a deep learning framework that can identify shoulder abnormalities such as fractures in X-ray images with a remarkable 99% accuracy, assisting clinicians in making rapid and accurate decisions during emergencies.
To build the deep learning framework, scientists at Queensland University of Technology (QUT, Brisbane, Australia) employed a feature fusion technique that combines features derived from seven deep neural models. The success of machine learning-based classification techniques largely depends on fully descriptive features to differentiate between various classes accurately. The feature fusion technique enhances the outcomes of individual models by providing a complete description of the internal data, resulting in a compact representation of fused features and thereby improving the diagnostic accuracy of the task.
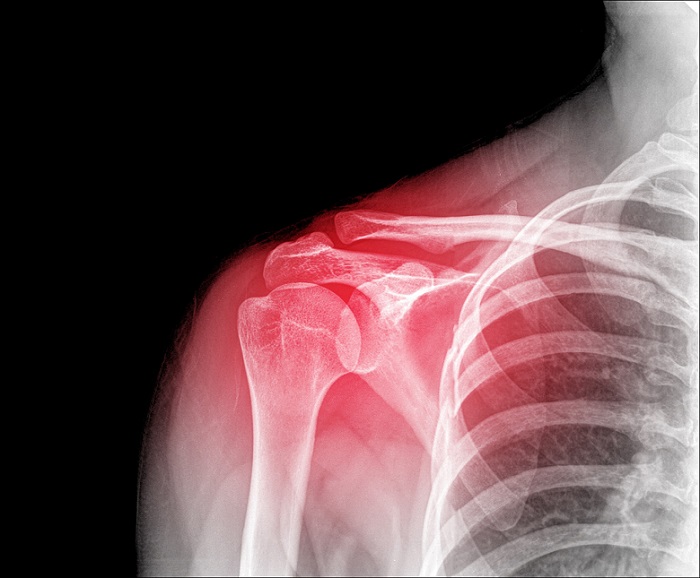
By individually training and evaluating seven deep convolutional neural networks for feature extraction, the researchers were able to merge these extracted features into a unified dataset for training machine learning classifiers. This proposed framework achieved an astounding accuracy rate of 99.2%, outperforming both previous computational methods and the diagnostic accuracy of human doctors, including orthopedic surgeons and radiologists, who achieved a 79% accuracy rate.
“The proposed framework has been validated against several potential biases to ensure trustworthy decision-making,” said co-researcher QUT Professor YuanTong Gu, Pro Vice-Chancellor and Head of the QUT School of Mechanical, Medical and Process Engineering. “This tool can provide real-time decisions, which is crucial for such a problem.”
Related Links:
Queensland University of Technology