Algorithm Outperforms Radiologists in Detecting Pneumonia on X-Rays
By MedImaging International staff writers Posted on 21 Nov 2017 |
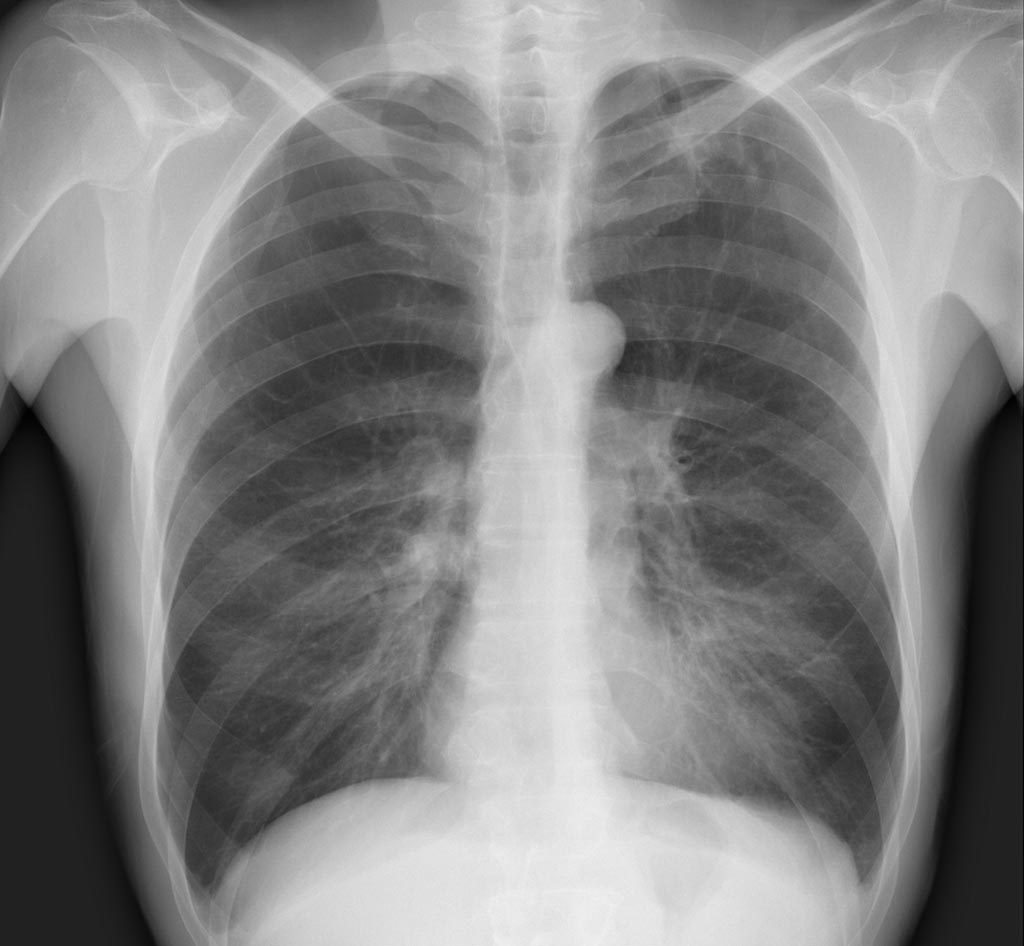
Image: The algorithm CheXNet can diagnose up to 14 types of medical conditions, including pneumonia (Photo courtesy of Stanford ML Group).
A deep learning algorithm developed by researchers from the Stanford University (Stanford, CA, USA) that evaluates chest X-rays for signs of disease has outperformed expert radiologists at diagnosing pneumonia in just over a month of its development. A paper about the algorithm named CheXNet, which can diagnose up to 14 types of medical conditions, was published November 14 on the open-access, scientific preprint website arXiv.
Soon after the National Institutes of Health Clinical Center recently released a public dataset containing 112,120 frontal-view chest X-ray images labeled with up to 14 possible pathologies, the Machine Learning Group at Stanford began developing an algorithm that could automatically diagnose the pathologies. Meanwhile, four Stanford radiologists independently annotated 420 of the images for possible indications of pneumonia. Within a week the researchers had developed an algorithm that diagnosed 10 of the pathologies labeled in the X-rays more accurately than the previous state-of-the-art results. In just over a month, CheXNet could beat these standards in all 14 identification tasks and also outperformed the four individual Stanford radiologists in pneumonia diagnoses.
The Stanford researchers have also developed a computer-based tool that produces what appears to be a heat map of chest X-rays, although instead of representing temperature, the colors of these maps represent the areas determined by the algorithm as the ones most likely to represent pneumonia. The tool could help reduce the amount of missed pneumonia cases and significantly accelerate the workflow of radiologists by indicating where to look first, resulting in faster diagnoses for the sickest patients.
“We plan to continue building and improving upon medical algorithms that can automatically detect abnormalities and we hope to make high-quality, anonymized medical datasets publicly available for others to work on similar problems,” said Jeremy Irvin, a graduate student in the Machine Learning group and co-lead author of the paper. “There is massive potential for machine learning to improve the current health care system, and we want to continue to be at the forefront of innovation in the field.”
Related Links:
Stanford University
Soon after the National Institutes of Health Clinical Center recently released a public dataset containing 112,120 frontal-view chest X-ray images labeled with up to 14 possible pathologies, the Machine Learning Group at Stanford began developing an algorithm that could automatically diagnose the pathologies. Meanwhile, four Stanford radiologists independently annotated 420 of the images for possible indications of pneumonia. Within a week the researchers had developed an algorithm that diagnosed 10 of the pathologies labeled in the X-rays more accurately than the previous state-of-the-art results. In just over a month, CheXNet could beat these standards in all 14 identification tasks and also outperformed the four individual Stanford radiologists in pneumonia diagnoses.
The Stanford researchers have also developed a computer-based tool that produces what appears to be a heat map of chest X-rays, although instead of representing temperature, the colors of these maps represent the areas determined by the algorithm as the ones most likely to represent pneumonia. The tool could help reduce the amount of missed pneumonia cases and significantly accelerate the workflow of radiologists by indicating where to look first, resulting in faster diagnoses for the sickest patients.
“We plan to continue building and improving upon medical algorithms that can automatically detect abnormalities and we hope to make high-quality, anonymized medical datasets publicly available for others to work on similar problems,” said Jeremy Irvin, a graduate student in the Machine Learning group and co-lead author of the paper. “There is massive potential for machine learning to improve the current health care system, and we want to continue to be at the forefront of innovation in the field.”
Related Links:
Stanford University
Latest Industry News News
- Hologic Acquires UK-Based Breast Surgical Guidance Company Endomagnetics Ltd.
- Bayer and Google Partner on New AI Product for Radiologists
- Samsung and Bracco Enter Into New Diagnostic Ultrasound Technology Agreement
- IBA Acquires Radcal to Expand Medical Imaging Quality Assurance Offering
- International Societies Suggest Key Considerations for AI Radiology Tools
- Samsung's X-Ray Devices to Be Powered by Lunit AI Solutions for Advanced Chest Screening
- Canon Medical and Olympus Collaborate on Endoscopic Ultrasound Systems
- GE HealthCare Acquires AI Imaging Analysis Company MIM Software
- First Ever International Criteria Lays Foundation for Improved Diagnostic Imaging of Brain Tumors
- RSNA Unveils 10 Most Cited Radiology Studies of 2023
- RSNA 2023 Technical Exhibits to Offer Innovations in AI, 3D Printing and More
- AI Medical Imaging Products to Increase Five-Fold by 2035, Finds Study
- RSNA 2023 Technical Exhibits to Highlight Latest Medical Imaging Innovations
- AI-Powered Technologies to Aid Interpretation of X-Ray and MRI Images for Improved Disease Diagnosis
- Hologic and Bayer Partner to Improve Mammography Imaging
- Global Fixed and Mobile C-Arms Market Driven by Increasing Surgical Procedures
Channels
Radiography
view channel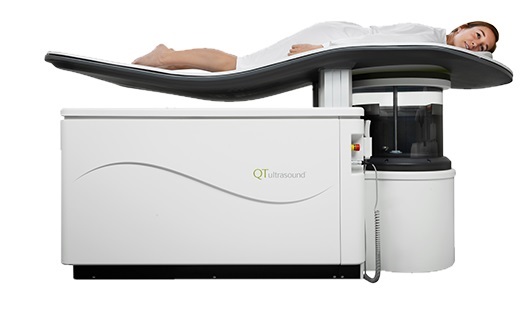
Novel Breast Imaging System Proves As Effective As Mammography
Breast cancer remains the most frequently diagnosed cancer among women. It is projected that one in eight women will be diagnosed with breast cancer during her lifetime, and one in 42 women who turn 50... Read more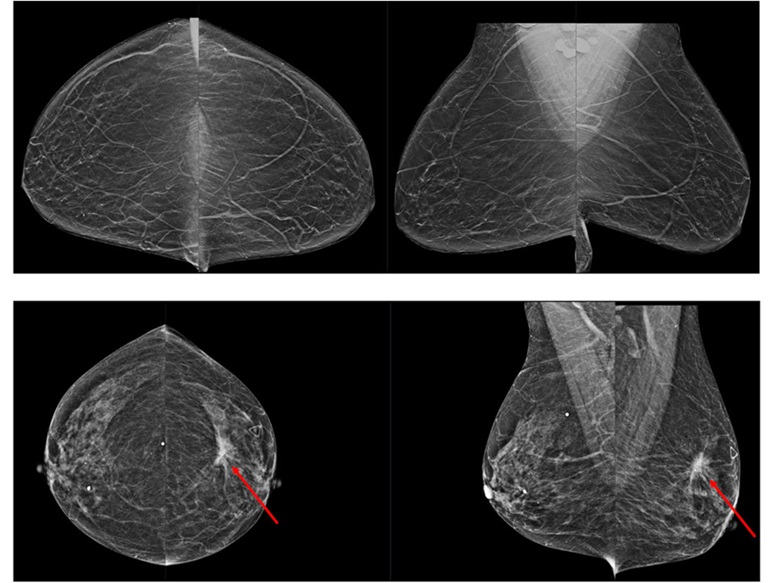
AI Assistance Improves Breast-Cancer Screening by Reducing False Positives
Radiologists typically detect one case of cancer for every 200 mammograms reviewed. However, these evaluations often result in false positives, leading to unnecessary patient recalls for additional testing,... Read moreMRI
view channel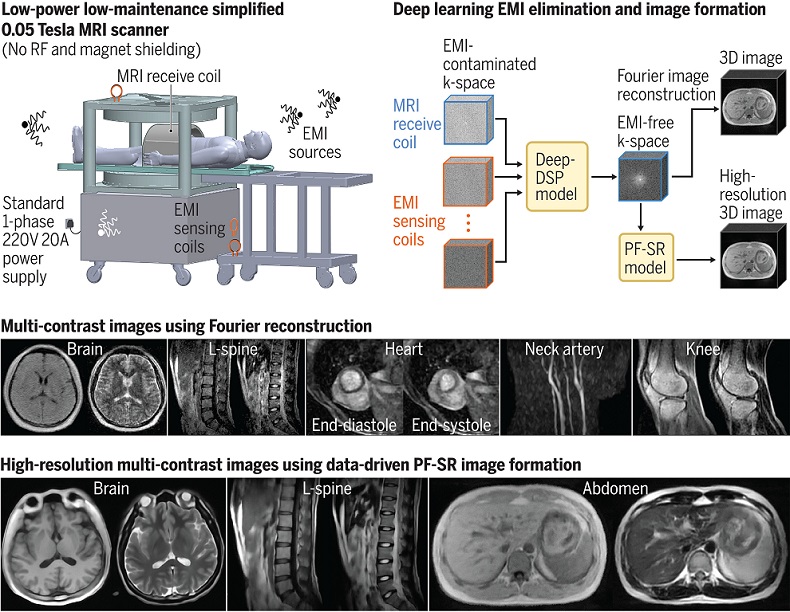
Low-Cost Whole-Body MRI Device Combined with AI Generates High-Quality Results
Magnetic Resonance Imaging (MRI) has significantly transformed healthcare, providing a noninvasive, radiation-free method for detailed imaging. It is especially promising for the future of medical diagnosis... Read more
World's First Whole-Body Ultra-High Field MRI Officially Comes To Market
The world's first whole-body ultra-high field (UHF) MRI has officially come to market, marking a remarkable advancement in diagnostic radiology. United Imaging (Shanghai, China) has secured clearance from the U.... Read moreUltrasound
view channel.jpg)
Diagnostic System Automatically Analyzes TTE Images to Identify Congenital Heart Disease
Congenital heart disease (CHD) is one of the most prevalent congenital anomalies worldwide, presenting substantial health and financial challenges for affected patients. Early detection and treatment of... Read more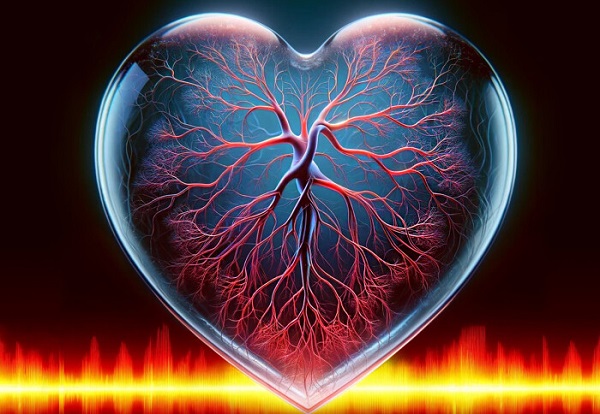
Super-Resolution Imaging Technique Could Improve Evaluation of Cardiac Conditions
The heart depends on efficient blood circulation to pump blood throughout the body, delivering oxygen to tissues and removing carbon dioxide and waste. Yet, when heart vessels are damaged, it can disrupt... Read more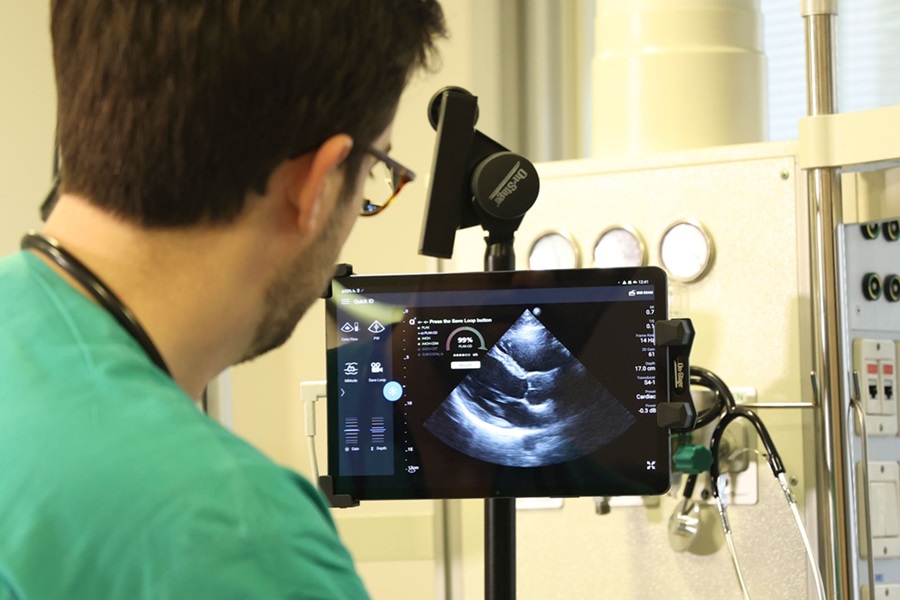
First AI-Powered POC Ultrasound Diagnostic Solution Helps Prioritize Cases Based On Severity
Ultrasound scans are essential for identifying and diagnosing various medical conditions, but often, patients must wait weeks or months for results due to a shortage of qualified medical professionals... Read moreNuclear Medicine
view channel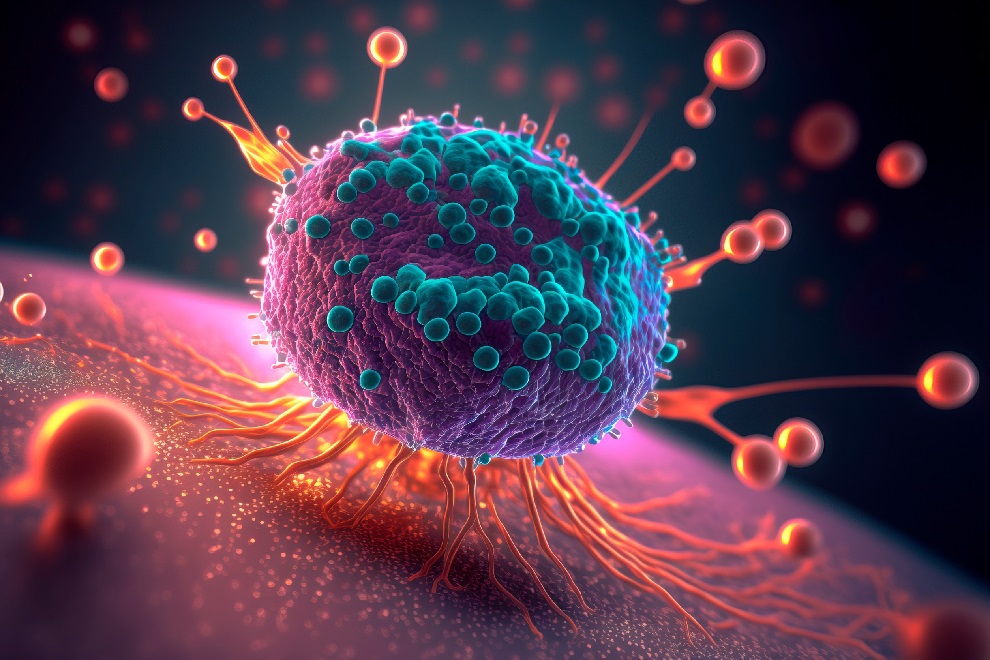
New PET Biomarker Predicts Success of Immune Checkpoint Blockade Therapy
Immunotherapies, such as immune checkpoint blockade (ICB), have shown promising clinical results in treating melanoma, non-small cell lung cancer, and other tumor types. However, the effectiveness of these... Read more![Image: Whole-body maximum-intensity projections over time after [68Ga]Ga-DPI-4452 administration (Photo courtesy of SNMMI) Image: Whole-body maximum-intensity projections over time after [68Ga]Ga-DPI-4452 administration (Photo courtesy of SNMMI)](https://globetechcdn.com/mobile_medicalimaging/images/stories/articles/article_images/2024-05-09/JNM May 2024 Hofman.jpg)
New PET Agent Rapidly and Accurately Visualizes Lesions in Clear Cell Renal Cell Carcinoma Patients
Clear cell renal cell cancer (ccRCC) represents 70-80% of renal cell carcinoma cases. While localized disease can be effectively treated with surgery and ablative therapies, one-third of patients either... Read more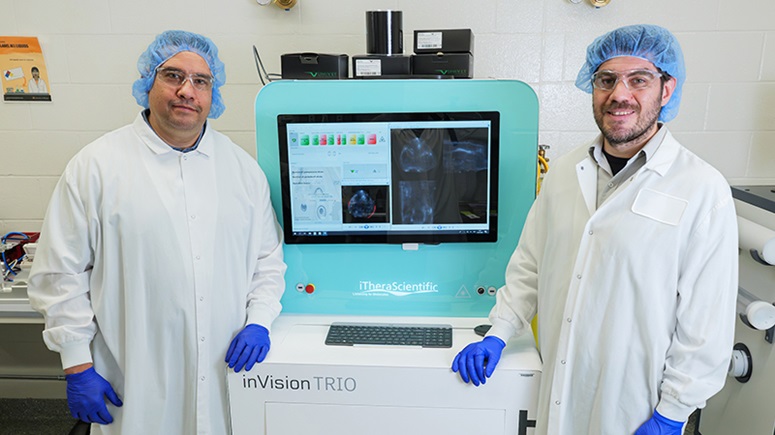
New Imaging Technique Monitors Inflammation Disorders without Radiation Exposure
Imaging inflammation using traditional radiological techniques presents significant challenges, including radiation exposure, poor image quality, high costs, and invasive procedures. Now, new contrast... Read more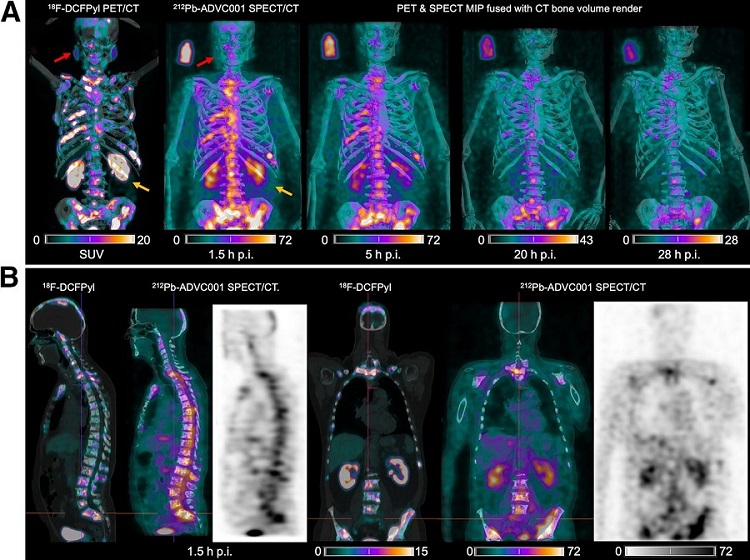
New SPECT/CT Technique Could Change Imaging Practices and Increase Patient Access
The development of lead-212 (212Pb)-PSMA–based targeted alpha therapy (TAT) is garnering significant interest in treating patients with metastatic castration-resistant prostate cancer. The imaging of 212Pb,... Read moreGeneral/Advanced Imaging
view channel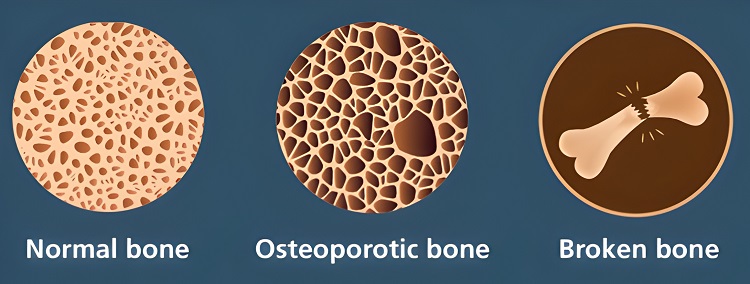
Bone Density Test Uses Existing CT Images to Predict Fractures
Osteoporotic fractures are not only devastating and deadly, especially hip fractures, but also impose significant costs. They rank among the top chronic diseases in terms of disability-adjusted life years... Read more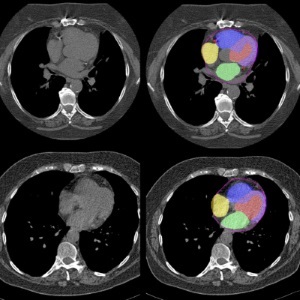
AI Predicts Cardiac Risk and Mortality from Routine Chest CT Scans
Heart disease remains the leading cause of death and is largely preventable, yet many individuals are unaware of their risk until it becomes severe. Early detection through screening can reveal heart issues,... Read moreImaging IT
view channel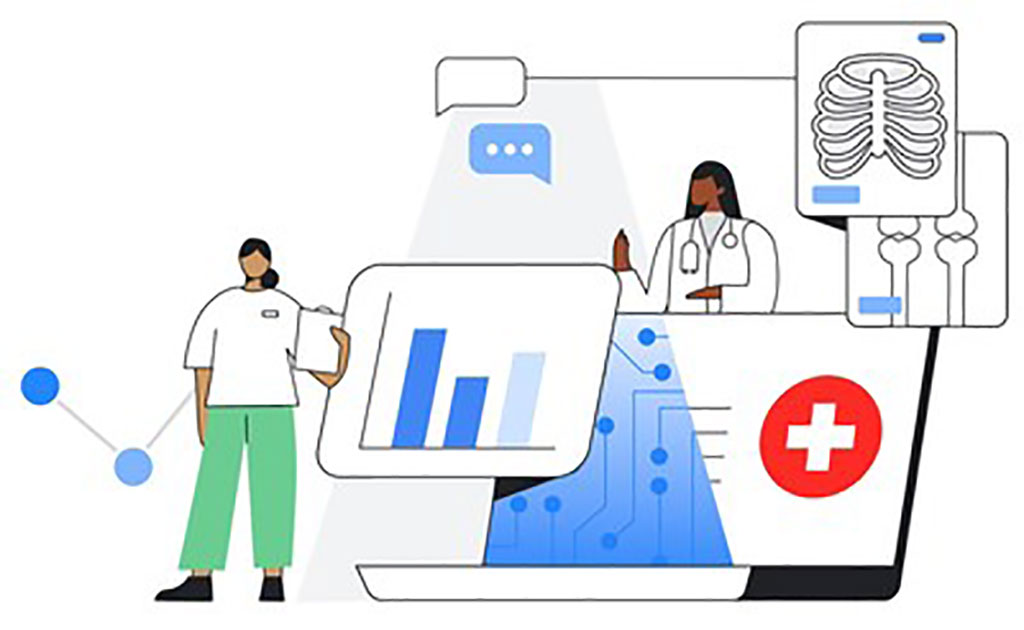
New Google Cloud Medical Imaging Suite Makes Imaging Healthcare Data More Accessible
Medical imaging is a critical tool used to diagnose patients, and there are billions of medical images scanned globally each year. Imaging data accounts for about 90% of all healthcare data1 and, until... Read more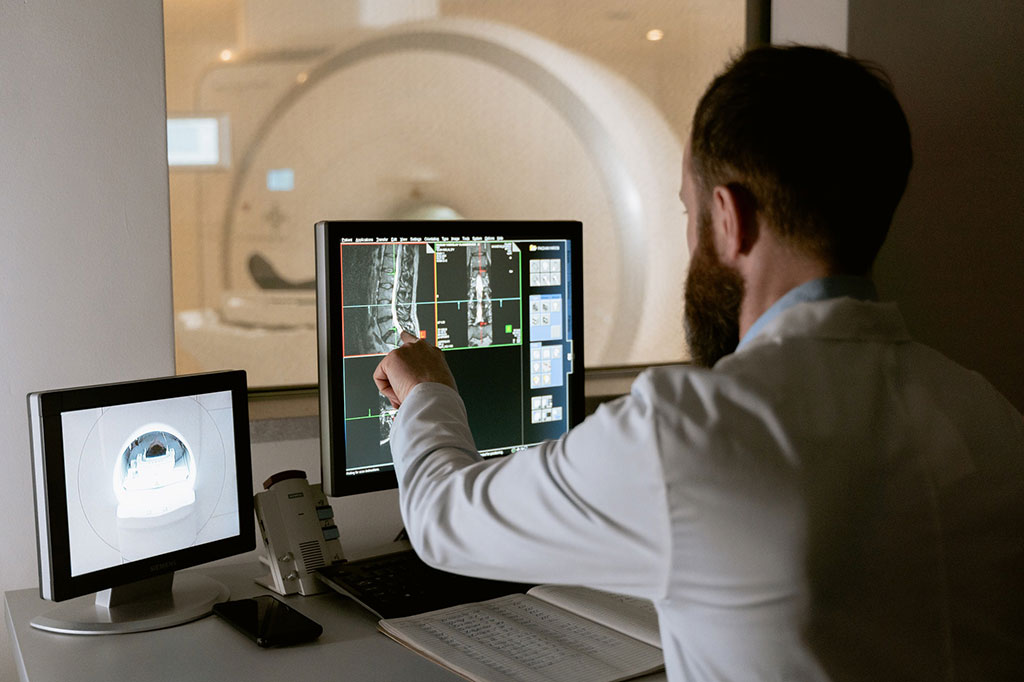