Segmentation Makes Lymph-Node Measurement More Accurate
By MedImaging International staff writers Posted on 15 Dec 2016 |
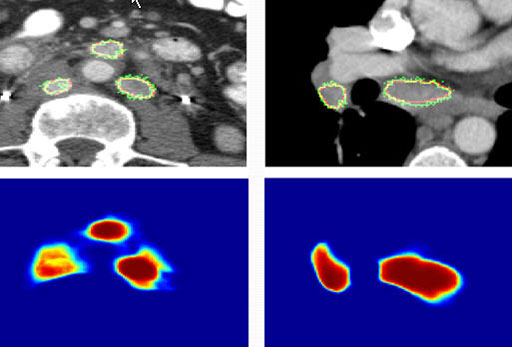
Image: Lymph nodes segmented by BNF (green), with corresponding BNF probability maps (Photo courtesy of Ronald Summers/NIH).
Neural networks could help a new automated lymph-node segmentation method improve cancer care, according to a new study.
Developed at the U.S. National Institutes of Health (NIH, Bethesda, MD, USA), the new analysis method combines two holistically nested neural networks (HNNs) and structured optimization techniques in order to solve the problem of separating lymph nodes from similar surrounding tissues on radiographic images. In addition, the technique can measure the volume of the lymph node clusters, which is a more accurate estimation of size than diameter.
The two HNNs, built on convolutional and deeply supervised neural networks, are trained to learn lymph-node cluster appearance (HNN-A) or contour (HNN-C) probabilistic output maps derived from thoracoabdominal computerized tomography (CT) images. The two components are prominent in the concept of boundary neural fields (BNF), which matches lesion measurements with more than 80% accuracy. To do so, HNN-A and HNN-C predictions are formulated into unary and pairwise terms of conditional random fields (CRFs).
The CRFs are subsequently solved using three standardized output methods: dense CRF (dCRF), graph cuts (GC), and BNFs. All of these segmentation predictions are used to compute lymph-node cluster volumes on the dataset. An expert radiologist then segments all enlarged lymph nodes with short-axis diameters of at least 10 mm. According to the researchers, the method could significantly improve cancer care by making lymph-node measurements more accurate. The new segmentation method was presented at the RSNA annual conference, held during November 2016 in Chicago (IL, USA).
“Thoracoabdominal lesions are among the most challenging to analyze on CT. The reason for this is that CT images of this region display very poor intensity and texture contrast. This is due to the fact that lymph nodes are very similar in intensity to many of the surrounding tissues,” said lead author and study presenter Isabella Nogues, MD. “Also, the high frequency of lymph-node clusters and the ambiguous boundaries of lymph nodes make accurate measurement very difficult.”
“The main conclusion was that boundary neural field was the most accurate segmentation method, which is very promising for the development of imaging biomarkers based on volume measurements,” concluded Dr. Nogues and colleagues of the NIH Imaging Biomarkers and Computer-Aided Diagnosis Laboratory. “These volume measurements, in turn, could potentially improve response evaluation criteria in solid tumors (RECIST) measurements for lymph nodes.”
Related Links:
U.S. National Institutes of Health
Developed at the U.S. National Institutes of Health (NIH, Bethesda, MD, USA), the new analysis method combines two holistically nested neural networks (HNNs) and structured optimization techniques in order to solve the problem of separating lymph nodes from similar surrounding tissues on radiographic images. In addition, the technique can measure the volume of the lymph node clusters, which is a more accurate estimation of size than diameter.
The two HNNs, built on convolutional and deeply supervised neural networks, are trained to learn lymph-node cluster appearance (HNN-A) or contour (HNN-C) probabilistic output maps derived from thoracoabdominal computerized tomography (CT) images. The two components are prominent in the concept of boundary neural fields (BNF), which matches lesion measurements with more than 80% accuracy. To do so, HNN-A and HNN-C predictions are formulated into unary and pairwise terms of conditional random fields (CRFs).
The CRFs are subsequently solved using three standardized output methods: dense CRF (dCRF), graph cuts (GC), and BNFs. All of these segmentation predictions are used to compute lymph-node cluster volumes on the dataset. An expert radiologist then segments all enlarged lymph nodes with short-axis diameters of at least 10 mm. According to the researchers, the method could significantly improve cancer care by making lymph-node measurements more accurate. The new segmentation method was presented at the RSNA annual conference, held during November 2016 in Chicago (IL, USA).
“Thoracoabdominal lesions are among the most challenging to analyze on CT. The reason for this is that CT images of this region display very poor intensity and texture contrast. This is due to the fact that lymph nodes are very similar in intensity to many of the surrounding tissues,” said lead author and study presenter Isabella Nogues, MD. “Also, the high frequency of lymph-node clusters and the ambiguous boundaries of lymph nodes make accurate measurement very difficult.”
“The main conclusion was that boundary neural field was the most accurate segmentation method, which is very promising for the development of imaging biomarkers based on volume measurements,” concluded Dr. Nogues and colleagues of the NIH Imaging Biomarkers and Computer-Aided Diagnosis Laboratory. “These volume measurements, in turn, could potentially improve response evaluation criteria in solid tumors (RECIST) measurements for lymph nodes.”
Related Links:
U.S. National Institutes of Health
Latest General/Advanced Imaging News
- Bone Density Test Uses Existing CT Images to Predict Fractures
- AI Predicts Cardiac Risk and Mortality from Routine Chest CT Scans
- Radiation Therapy Computed Tomography Solution Boosts Imaging Accuracy
- PET Scans Reveal Hidden Inflammation in Multiple Sclerosis Patients
- Artificial Intelligence Evaluates Cardiovascular Risk from CT Scans
- New AI Method Captures Uncertainty in Medical Images
- CT Coronary Angiography Reduces Need for Invasive Tests to Diagnose Coronary Artery Disease
- Novel Blood Test Could Reduce Need for PET Imaging of Patients with Alzheimer’s
- CT-Based Deep Learning Algorithm Accurately Differentiates Benign From Malignant Vertebral Fractures
- Minimally Invasive Procedure Could Help Patients Avoid Thyroid Surgery
- Self-Driving Mobile C-Arm Reduces Imaging Time during Surgery
- AR Application Turns Medical Scans Into Holograms for Assistance in Surgical Planning
- Imaging Technology Provides Ground-Breaking New Approach for Diagnosing and Treating Bowel Cancer
- CT Coronary Calcium Scoring Predicts Heart Attacks and Strokes
- AI Model Detects 90% of Lymphatic Cancer Cases from PET and CT Images
- Breakthrough Technology Revolutionizes Breast Imaging
Channels
Radiography
view channel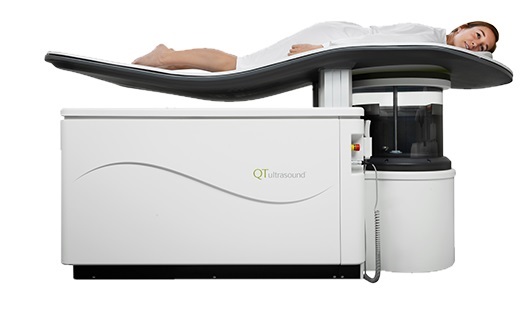
Novel Breast Imaging System Proves As Effective As Mammography
Breast cancer remains the most frequently diagnosed cancer among women. It is projected that one in eight women will be diagnosed with breast cancer during her lifetime, and one in 42 women who turn 50... Read more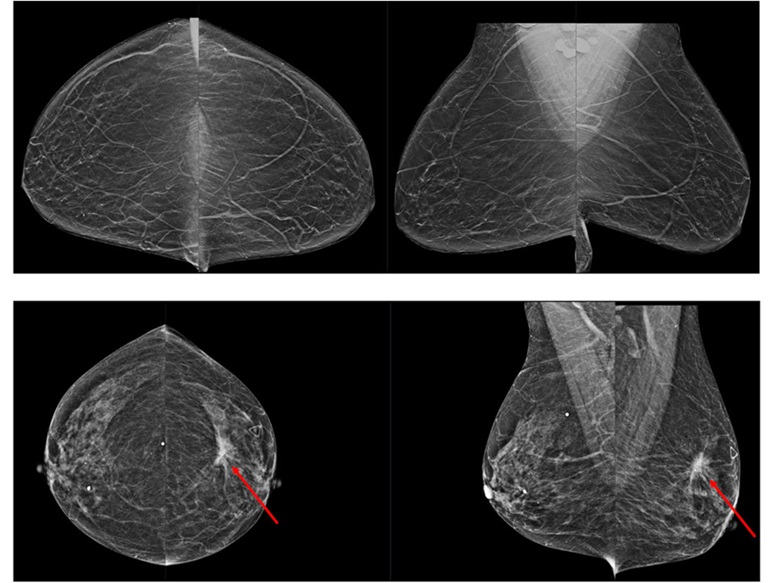
AI Assistance Improves Breast-Cancer Screening by Reducing False Positives
Radiologists typically detect one case of cancer for every 200 mammograms reviewed. However, these evaluations often result in false positives, leading to unnecessary patient recalls for additional testing,... Read moreMRI
view channel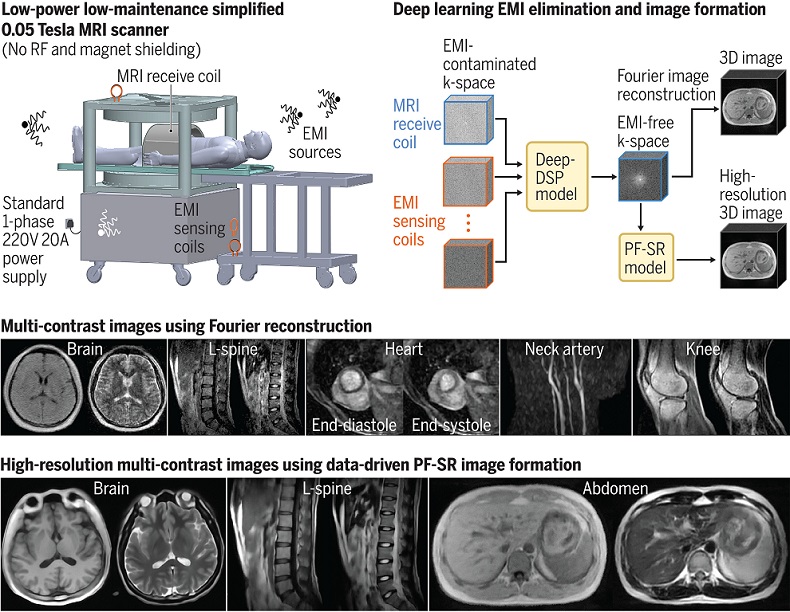
Low-Cost Whole-Body MRI Device Combined with AI Generates High-Quality Results
Magnetic Resonance Imaging (MRI) has significantly transformed healthcare, providing a noninvasive, radiation-free method for detailed imaging. It is especially promising for the future of medical diagnosis... Read more
World's First Whole-Body Ultra-High Field MRI Officially Comes To Market
The world's first whole-body ultra-high field (UHF) MRI has officially come to market, marking a remarkable advancement in diagnostic radiology. United Imaging (Shanghai, China) has secured clearance from the U.... Read moreUltrasound
view channel.jpg)
Diagnostic System Automatically Analyzes TTE Images to Identify Congenital Heart Disease
Congenital heart disease (CHD) is one of the most prevalent congenital anomalies worldwide, presenting substantial health and financial challenges for affected patients. Early detection and treatment of... Read more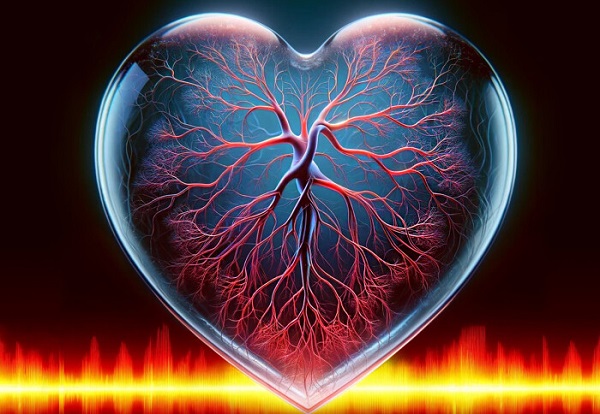
Super-Resolution Imaging Technique Could Improve Evaluation of Cardiac Conditions
The heart depends on efficient blood circulation to pump blood throughout the body, delivering oxygen to tissues and removing carbon dioxide and waste. Yet, when heart vessels are damaged, it can disrupt... Read more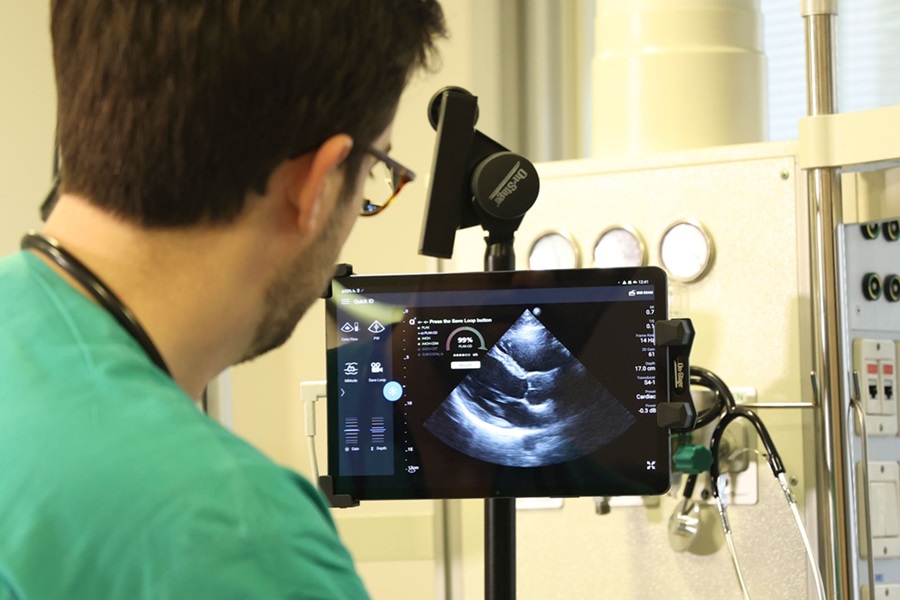
First AI-Powered POC Ultrasound Diagnostic Solution Helps Prioritize Cases Based On Severity
Ultrasound scans are essential for identifying and diagnosing various medical conditions, but often, patients must wait weeks or months for results due to a shortage of qualified medical professionals... Read moreNuclear Medicine
view channel![Image: Whole-body maximum-intensity projections over time after [68Ga]Ga-DPI-4452 administration (Photo courtesy of SNMMI) Image: Whole-body maximum-intensity projections over time after [68Ga]Ga-DPI-4452 administration (Photo courtesy of SNMMI)](https://globetechcdn.com/mobile_medicalimaging/images/stories/articles/article_images/2024-05-09/JNM May 2024 Hofman.jpg)
New PET Agent Rapidly and Accurately Visualizes Lesions in Clear Cell Renal Cell Carcinoma Patients
Clear cell renal cell cancer (ccRCC) represents 70-80% of renal cell carcinoma cases. While localized disease can be effectively treated with surgery and ablative therapies, one-third of patients either... Read more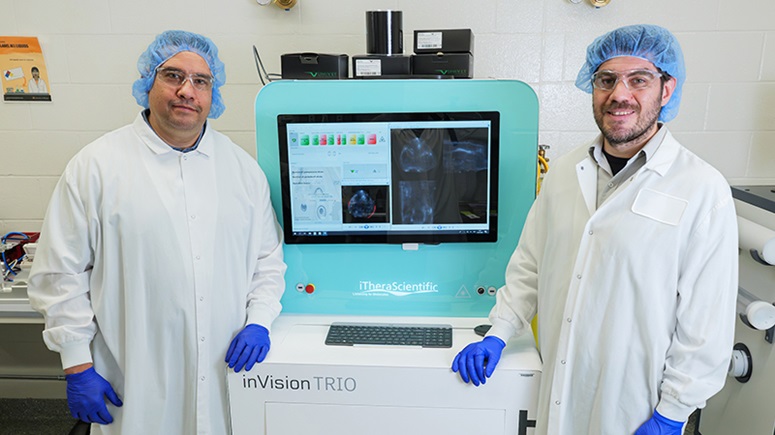
New Imaging Technique Monitors Inflammation Disorders without Radiation Exposure
Imaging inflammation using traditional radiological techniques presents significant challenges, including radiation exposure, poor image quality, high costs, and invasive procedures. Now, new contrast... Read more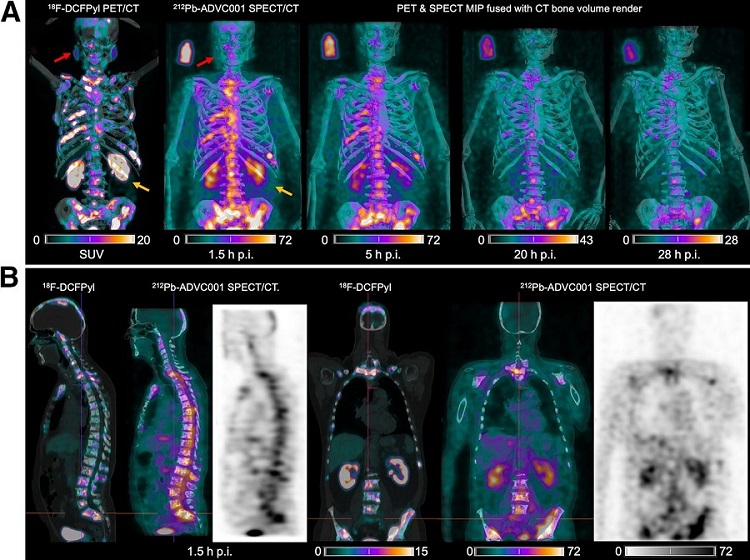
New SPECT/CT Technique Could Change Imaging Practices and Increase Patient Access
The development of lead-212 (212Pb)-PSMA–based targeted alpha therapy (TAT) is garnering significant interest in treating patients with metastatic castration-resistant prostate cancer. The imaging of 212Pb,... Read moreImaging IT
view channel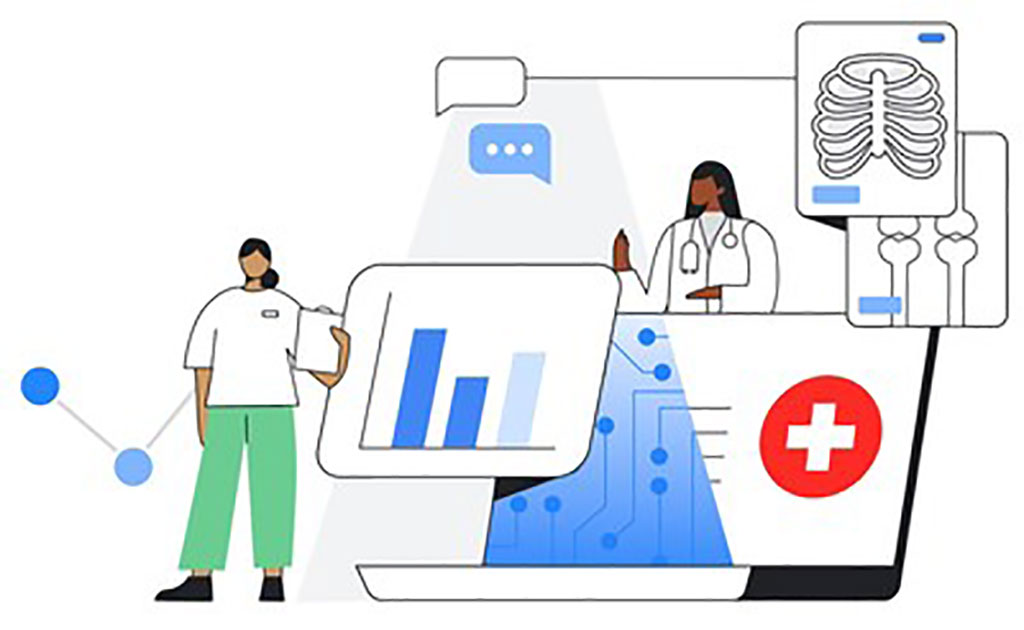
New Google Cloud Medical Imaging Suite Makes Imaging Healthcare Data More Accessible
Medical imaging is a critical tool used to diagnose patients, and there are billions of medical images scanned globally each year. Imaging data accounts for about 90% of all healthcare data1 and, until... Read more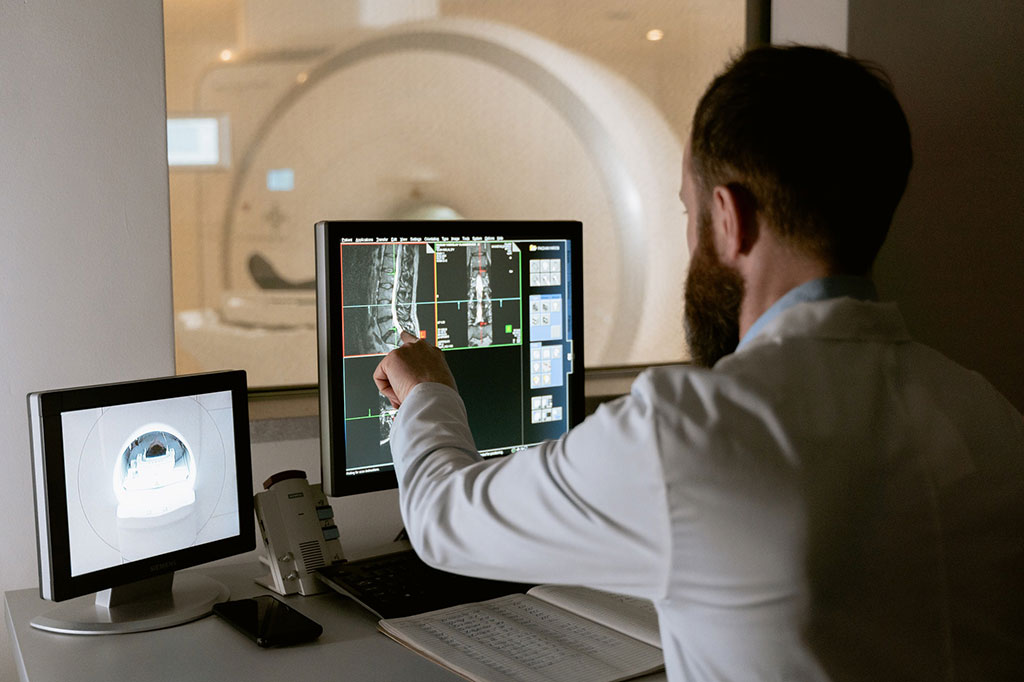
Global AI in Medical Diagnostics Market to Be Driven by Demand for Image Recognition in Radiology
The global artificial intelligence (AI) in medical diagnostics market is expanding with early disease detection being one of its key applications and image recognition becoming a compelling consumer proposition... Read moreIndustry News
view channel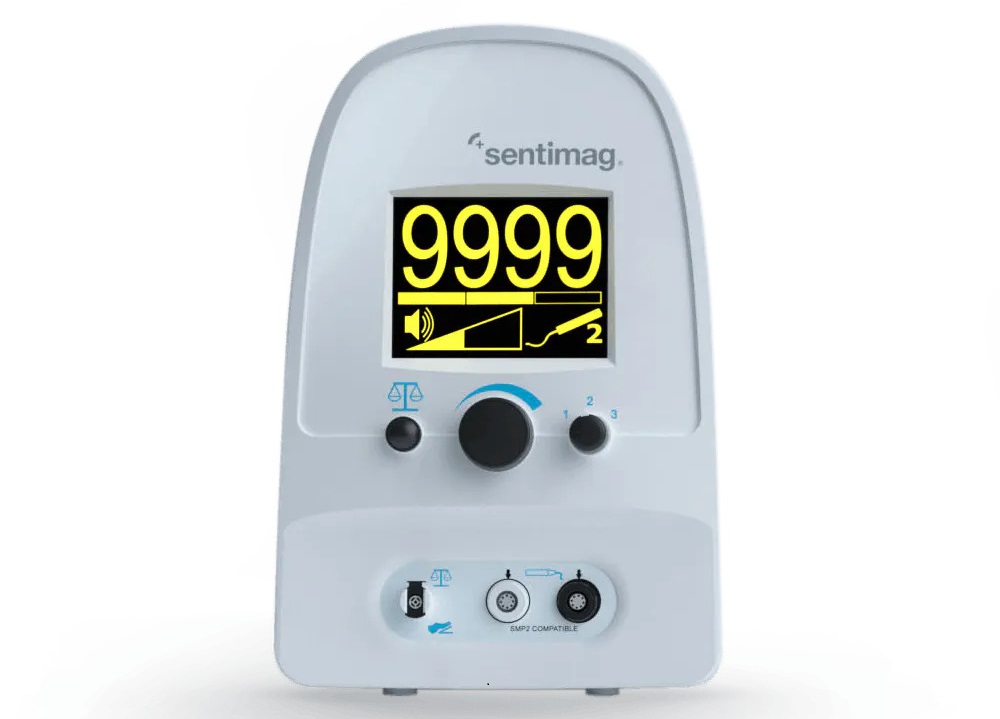
Hologic Acquires UK-Based Breast Surgical Guidance Company Endomagnetics Ltd.
Hologic, Inc. (Marlborough, MA, USA) has entered into a definitive agreement to acquire Endomagnetics Ltd. (Cambridge, UK), a privately held developer of breast cancer surgery technologies, for approximately... Read more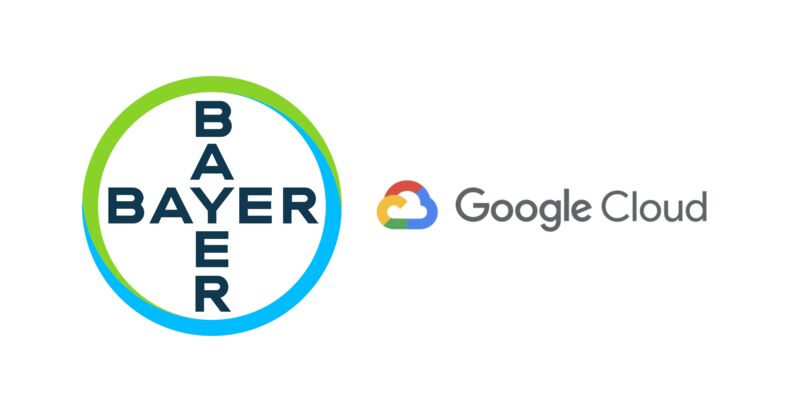
Bayer and Google Partner on New AI Product for Radiologists
Medical imaging data comprises around 90% of all healthcare data, and it is a highly complex and rich clinical data modality and serves as a vital tool for diagnosing patients. Each year, billions of medical... Read more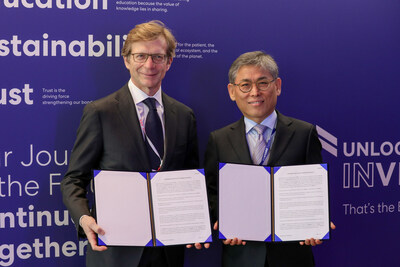