AI Can Distinguish Brain Tumors from Healthy Tissue
By MedImaging International staff writers Posted on 22 Nov 2024 |
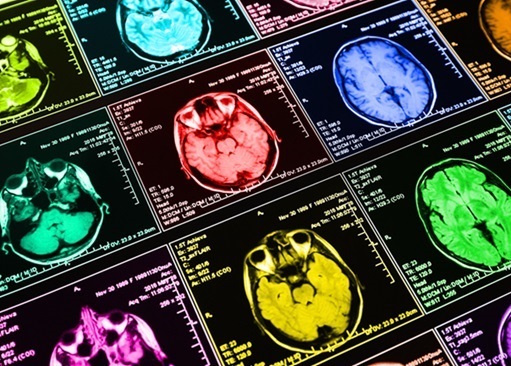
Researchers have made significant advancements in artificial intelligence (AI) for medical applications. AI holds particular promise in radiology, where delays in processing medical images can often postpone patient care. Convolutional neural networks (CNNs) are robust tools used to train AI models on large image datasets to identify and classify images. This enables the networks to “learn” to distinguish between different types of images. Furthermore, CNNs also have the ability for “transfer learning,” allowing models trained for one task to be applied to similar new tasks. AI models have already demonstrated the ability to identify brain tumors in MRI images with near-human accuracy. Now, in a new study, researchers have shown that AI models can be trained to differentiate between brain tumors and healthy tissue.
While detecting camouflaged animals and classifying brain tumors may seem unrelated, the researchers from Boston University (Boston, MA, USA) saw a connection between the natural camouflage of animals and the way cancerous cells blend with surrounding healthy tissue. The ability to generalize — the process of categorizing various items under a common identity — is crucial for the AI model to detect camouflaged objects. This capability could be particularly advantageous for detecting tumors. In their retrospective study using publicly available MRI data, the researchers explored how neural networks could be trained using brain cancer imaging data, incorporating a unique camouflage detection step to enhance the networks' tumor detection capabilities.
The researchers utilized MRIs from public repositories of both cancerous and healthy brain scans to train the networks to identify cancerous areas, distinguish them from healthy tissue, and classify the type of cancer. The results, published in Biology Methods and Protocols, showed that the networks performed nearly flawlessly at detecting healthy brain scans, with only 1-2 false negatives, and were also able to differentiate between cancerous and non-cancerous brains. One of the networks achieved an accuracy of 85.99% in detecting brain cancer, while the other reached 83.85%. An important feature of these networks is their ability to explain their decisions, which can increase the trust that both medical professionals and patients place in the AI models. This transparency is particularly valuable, as deep learning models are often criticized for their lack of interpretability. The network was capable of generating images that highlighted specific areas in its classification of tumor-positive or tumor-negative scans, which would allow radiologists to verify the AI's findings, serving almost as a second opinion in radiology.
Going forward, the researchers believe that developing deep network models with decisions that are easy to explain will be crucial for AI to play a transparent and supportive role in clinical settings. While the networks performed less effectively when distinguishing between different types of brain cancer, the study demonstrated that they exhibited distinct internal representations. The accuracy and clarity of the networks improved as they were trained using camouflage detection. Transfer learning increased the networks' accuracy, and while the best performing model was about 6% less accurate than standard human detection, the research successfully highlights the improvements in accuracy brought about by this training approach. The researchers argue that, when combined with methods to explain the network’s decisions, this approach will foster the transparency needed for future AI applications in clinical settings.
“Advances in AI permit more accurate detection and recognition of patterns,” said the paper’s lead author, Arash Yazdanbakhsh. “This consequently allows for better imaging-based diagnosis aid and screening, but also necessitate more explanation for how AI accomplishes the task. Aiming for AI explainability enhances communication between humans and AI in general. This is particularly important between medical professionals and AI designed for medical purposes. Clear and explainable models are better positioned to assist diagnosis, track disease progression, and monitor treatment.”
Latest MRI News
- AI Tool Tracks Effectiveness of Multiple Sclerosis Treatments Using Brain MRI Scans
- Ultra-Powerful MRI Scans Enable Life-Changing Surgery in Treatment-Resistant Epileptic Patients
- AI-Powered MRI Technology Improves Parkinson’s Diagnoses
- Biparametric MRI Combined with AI Enhances Detection of Clinically Significant Prostate Cancer
- First-Of-Its-Kind AI-Driven Brain Imaging Platform to Better Guide Stroke Treatment Options
- New Model Improves Comparison of MRIs Taken at Different Institutions
- Groundbreaking New Scanner Sees 'Previously Undetectable' Cancer Spread
- First-Of-Its-Kind Tool Analyzes MRI Scans to Measure Brain Aging
- AI-Enhanced MRI Images Make Cancerous Breast Tissue Glow
- AI Model Automatically Segments MRI Images
- New Research Supports Routine Brain MRI Screening in Asymptomatic Late-Stage Breast Cancer Patients
- Revolutionary Portable Device Performs Rapid MRI-Based Stroke Imaging at Patient's Bedside
- AI Predicts After-Effects of Brain Tumor Surgery from MRI Scans
- MRI-First Strategy for Prostate Cancer Detection Proven Safe
- First-Of-Its-Kind 10' x 48' Mobile MRI Scanner Transforms User and Patient Experience
- New Model Makes MRI More Accurate and Reliable
Channels
Radiography
view channel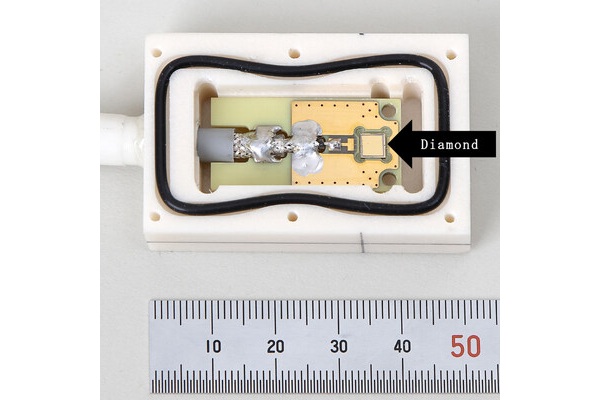
World's Largest Class Single Crystal Diamond Radiation Detector Opens New Possibilities for Diagnostic Imaging
Diamonds possess ideal physical properties for radiation detection, such as exceptional thermal and chemical stability along with a quick response time. Made of carbon with an atomic number of six, diamonds... Read more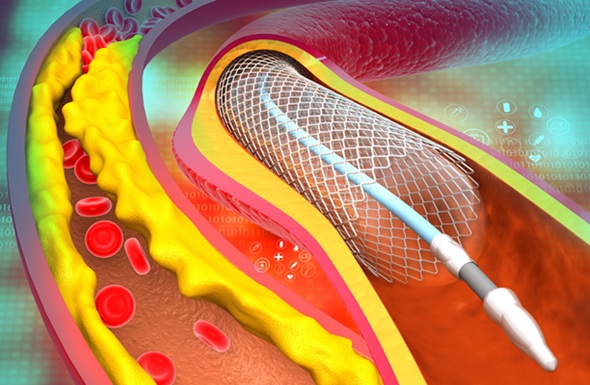
AI-Powered Imaging Technique Shows Promise in Evaluating Patients for PCI
Percutaneous coronary intervention (PCI), also known as coronary angioplasty, is a minimally invasive procedure where small metal tubes called stents are inserted into partially blocked coronary arteries... Read moreUltrasound
view channel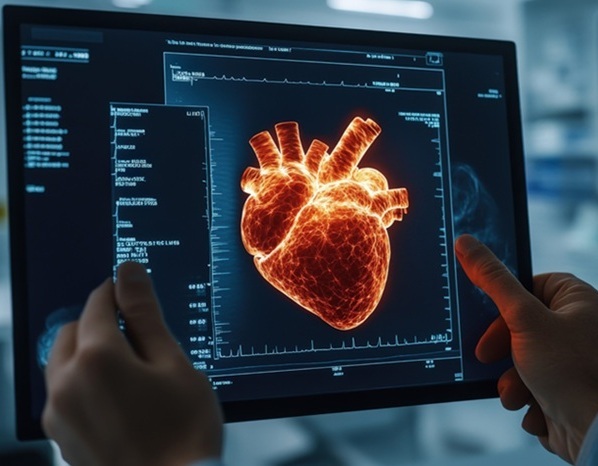
AI Identifies Heart Valve Disease from Common Imaging Test
Tricuspid regurgitation is a condition where the heart's tricuspid valve does not close completely during contraction, leading to backward blood flow, which can result in heart failure. A new artificial... Read more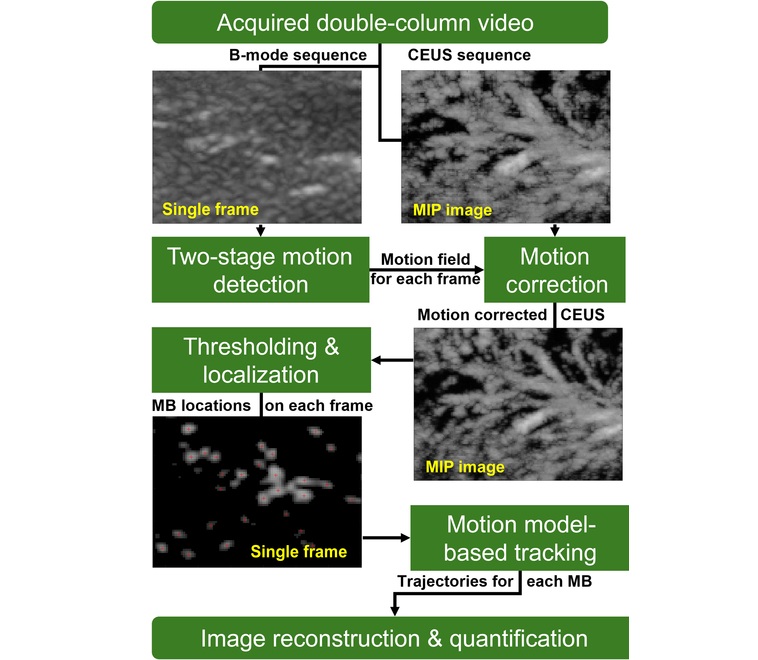
Novel Imaging Method Enables Early Diagnosis and Treatment Monitoring of Type 2 Diabetes
Type 2 diabetes is recognized as an autoimmune inflammatory disease, where chronic inflammation leads to alterations in pancreatic islet microvasculature, a key factor in β-cell dysfunction.... Read moreNuclear Medicine
view channel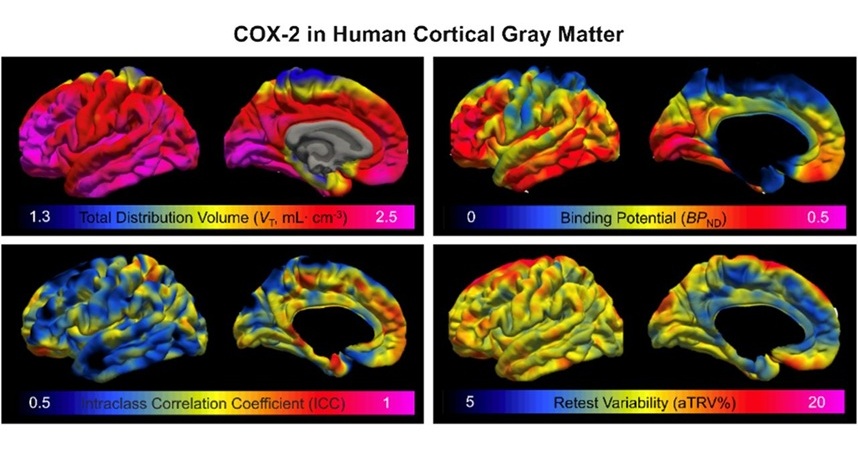
Novel PET Imaging Approach Offers Never-Before-Seen View of Neuroinflammation
COX-2, an enzyme that plays a key role in brain inflammation, can be significantly upregulated by inflammatory stimuli and neuroexcitation. Researchers suggest that COX-2 density in the brain could serve... Read more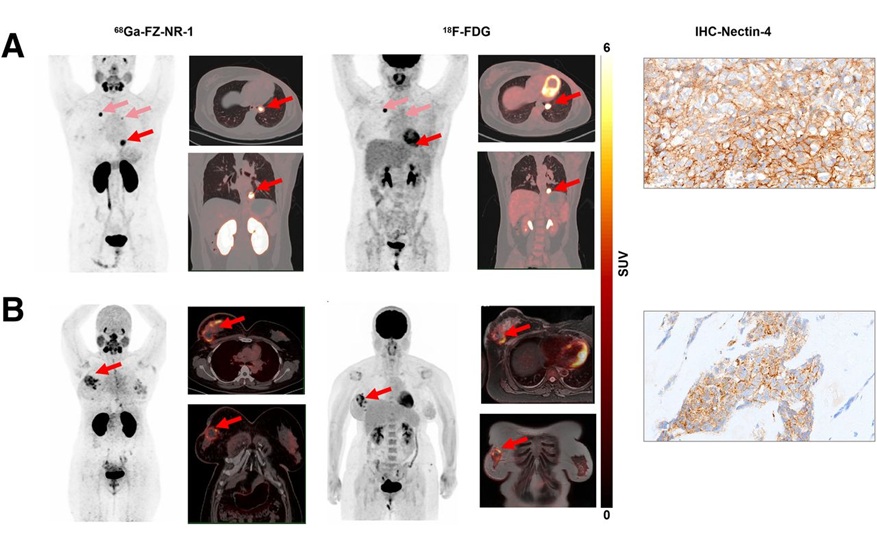
Novel Radiotracer Identifies Biomarker for Triple-Negative Breast Cancer
Triple-negative breast cancer (TNBC), which represents 15-20% of all breast cancer cases, is one of the most aggressive subtypes, with a five-year survival rate of about 40%. Due to its significant heterogeneity... Read moreGeneral/Advanced Imaging
view channel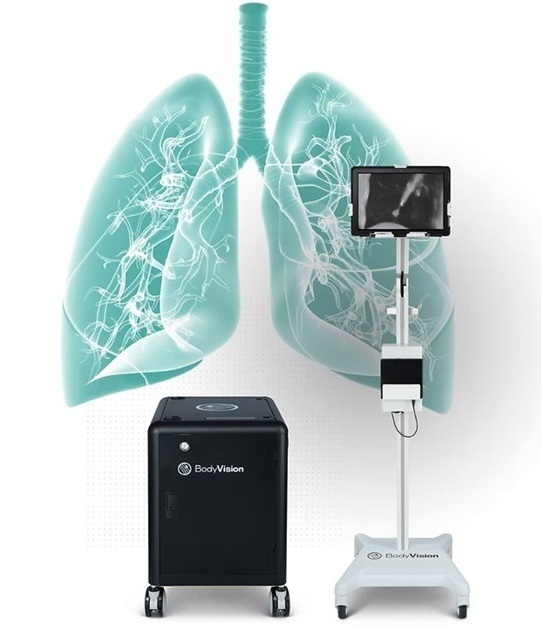
AI-Powered Imaging System Improves Lung Cancer Diagnosis
Given the need to detect lung cancer at earlier stages, there is an increasing need for a definitive diagnostic pathway for patients with suspicious pulmonary nodules. However, obtaining tissue samples... Read more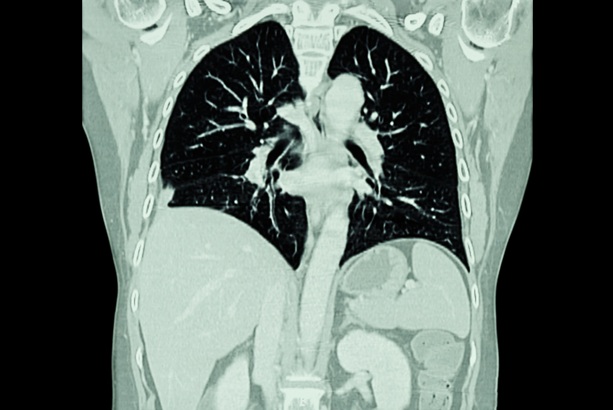
AI Model Significantly Enhances Low-Dose CT Capabilities
Lung cancer remains one of the most challenging diseases, making early diagnosis vital for effective treatment. Fortunately, advancements in artificial intelligence (AI) are revolutionizing lung cancer... Read moreImaging IT
view channel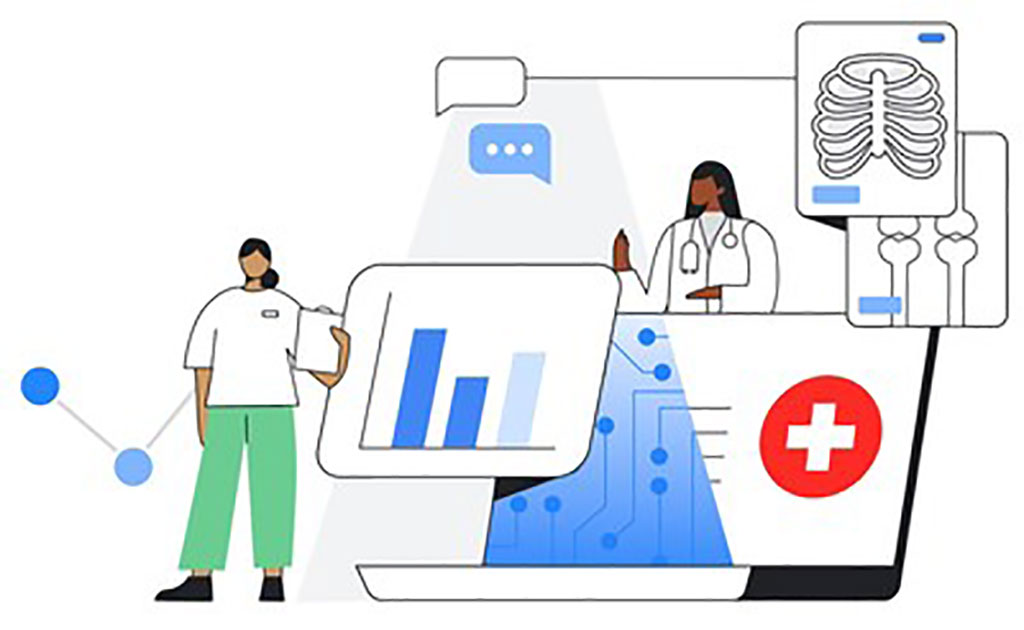
New Google Cloud Medical Imaging Suite Makes Imaging Healthcare Data More Accessible
Medical imaging is a critical tool used to diagnose patients, and there are billions of medical images scanned globally each year. Imaging data accounts for about 90% of all healthcare data1 and, until... Read more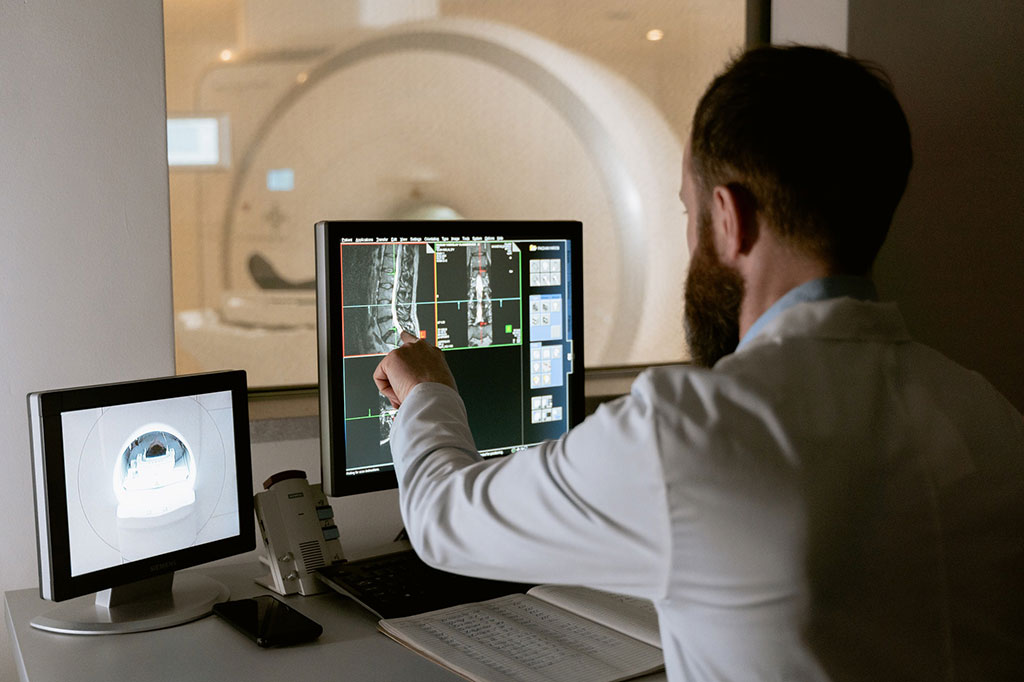
Global AI in Medical Diagnostics Market to Be Driven by Demand for Image Recognition in Radiology
The global artificial intelligence (AI) in medical diagnostics market is expanding with early disease detection being one of its key applications and image recognition becoming a compelling consumer proposition... Read moreIndustry News
view channel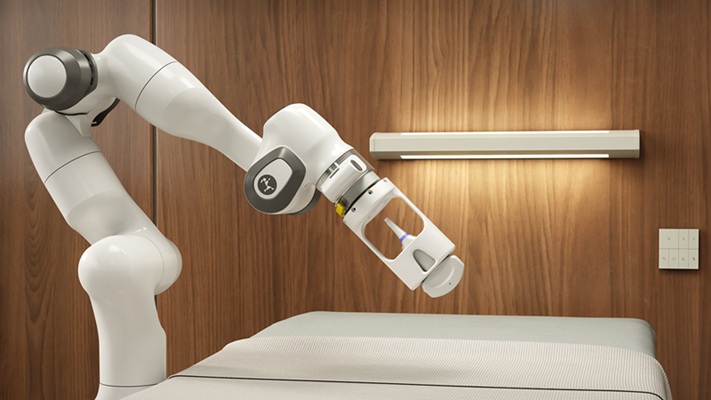
GE HealthCare and NVIDIA Collaboration to Reimagine Diagnostic Imaging
GE HealthCare (Chicago, IL, USA) has entered into a collaboration with NVIDIA (Santa Clara, CA, USA), expanding the existing relationship between the two companies to focus on pioneering innovation in... Read more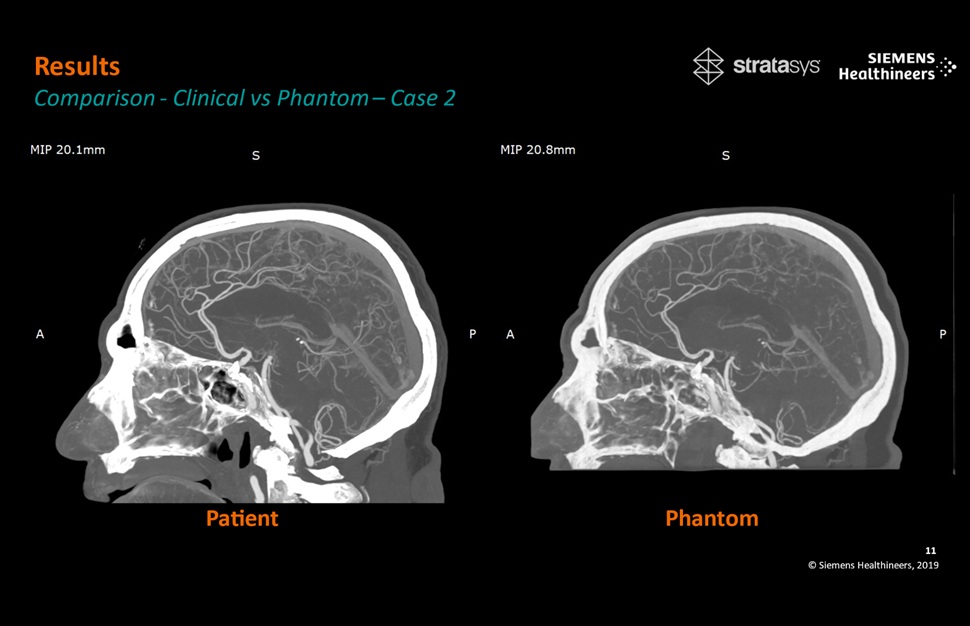
Patient-Specific 3D-Printed Phantoms Transform CT Imaging
New research has highlighted how anatomically precise, patient-specific 3D-printed phantoms are proving to be scalable, cost-effective, and efficient tools in the development of new CT scan algorithms... Read more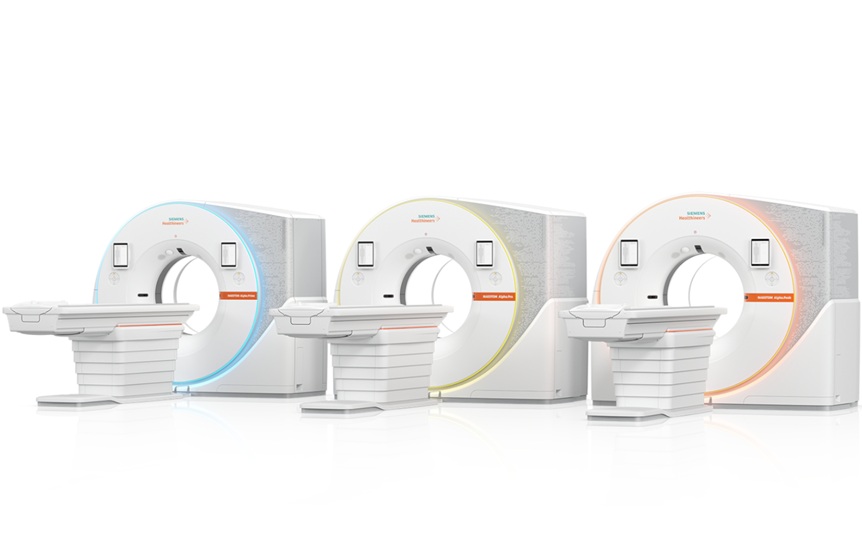
Siemens and Sectra Collaborate on Enhancing Radiology Workflows
Siemens Healthineers (Forchheim, Germany) and Sectra (Linköping, Sweden) have entered into a collaboration aimed at enhancing radiologists' diagnostic capabilities and, in turn, improving patient care... Read more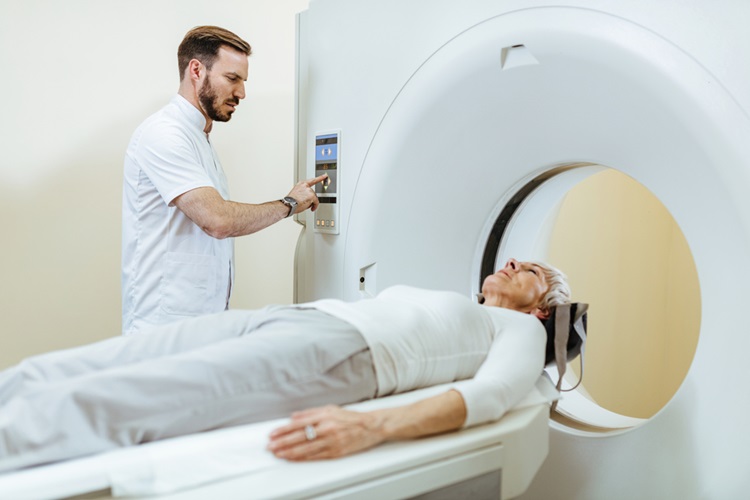