Machine Learning Method Can Help Women Avoid Unnecessary Breast Surgery
By MedImaging International staff writers Posted on 19 Mar 2019 |
A team of researchers from the Geisel School of Medicine at Dartmouth (Hanover, NH, USA) has developed a machine learning method to predict atypical ductal hyperplasia (ADH) upgrade to cancer.
ADH, a breast lesion associated with a four- to five-fold increase in the risk of breast cancer, is mainly found using mammography and identified on core needle biopsy. Despite multiple passes of the lesion during biopsy, only portions of the lesions are sampled. Other variable factors influence sampling and accuracy such that the presence of cancer may be underestimated by 10-45%. Currently, surgical removal is recommended for all ADH cases found on core needle biopsies to determine if the lesion is cancerous. About 20-30% of ADH cases are upgraded to cancer after surgical excision. However, this means that 70-80% of women undergo a costly and invasive surgical procedure for a benign (but high-risk) lesion.
The new machine learning method to predict ADH upgrade to cancer can potentially help clinicians and low-risk patients decide whether active surveillance and hormonal therapy is a reasonable alternative to surgical excision. An evaluation of the model by the researchers showed that the machine learning approach can identify 98% of all malignant cases prior to surgery while sparing from surgery 16% of women who otherwise would have undergone an unnecessary operation for a benign lesion. The researchers now plan to expand the scope of their model by including other high-risk breast lesions such as lobular neoplasia, papillomas, and radial scars. They also plan on further validating their approach on large external datasets using state and national breast cancer registries, and collaborating with other medical centers.
"Our results suggest there are robust clinical differences between women at low versus high risk for ADH upgrade to cancer based on core needle biopsy data that allowed our machine learning model to reliably predict malignancy upgrades in our dataset," said Saeed Hassanpour, PhD, who led the Dartmouth research team. "This study also identified important clinical variables involved in ADH upgrade risk."
"Our model can potentially help patients and clinicians choose an alternative management approach in low-risk cases," added Hassanpour. "In the era of personalized medicine, such models can be desirable for patients who value a shared decision-making approach with the ability to choose between surgical excision for certainty versus surveillance to avoid cost, stress, and potential side effects in women at low risk for upgrade of ADH to cancer."
Related Links:
Geisel School of Medicine at Dartmouth
ADH, a breast lesion associated with a four- to five-fold increase in the risk of breast cancer, is mainly found using mammography and identified on core needle biopsy. Despite multiple passes of the lesion during biopsy, only portions of the lesions are sampled. Other variable factors influence sampling and accuracy such that the presence of cancer may be underestimated by 10-45%. Currently, surgical removal is recommended for all ADH cases found on core needle biopsies to determine if the lesion is cancerous. About 20-30% of ADH cases are upgraded to cancer after surgical excision. However, this means that 70-80% of women undergo a costly and invasive surgical procedure for a benign (but high-risk) lesion.
The new machine learning method to predict ADH upgrade to cancer can potentially help clinicians and low-risk patients decide whether active surveillance and hormonal therapy is a reasonable alternative to surgical excision. An evaluation of the model by the researchers showed that the machine learning approach can identify 98% of all malignant cases prior to surgery while sparing from surgery 16% of women who otherwise would have undergone an unnecessary operation for a benign lesion. The researchers now plan to expand the scope of their model by including other high-risk breast lesions such as lobular neoplasia, papillomas, and radial scars. They also plan on further validating their approach on large external datasets using state and national breast cancer registries, and collaborating with other medical centers.
"Our results suggest there are robust clinical differences between women at low versus high risk for ADH upgrade to cancer based on core needle biopsy data that allowed our machine learning model to reliably predict malignancy upgrades in our dataset," said Saeed Hassanpour, PhD, who led the Dartmouth research team. "This study also identified important clinical variables involved in ADH upgrade risk."
"Our model can potentially help patients and clinicians choose an alternative management approach in low-risk cases," added Hassanpour. "In the era of personalized medicine, such models can be desirable for patients who value a shared decision-making approach with the ability to choose between surgical excision for certainty versus surveillance to avoid cost, stress, and potential side effects in women at low risk for upgrade of ADH to cancer."
Related Links:
Geisel School of Medicine at Dartmouth
Latest Industry News News
- GE HealthCare and NVIDIA Collaboration to Reimagine Diagnostic Imaging
- Patient-Specific 3D-Printed Phantoms Transform CT Imaging
- Siemens and Sectra Collaborate on Enhancing Radiology Workflows
- Bracco Diagnostics and ColoWatch Partner to Expand Availability CRC Screening Tests Using Virtual Colonoscopy
- Mindray Partners with TeleRay to Streamline Ultrasound Delivery
- Philips and Medtronic Partner on Stroke Care
- Siemens and Medtronic Enter into Global Partnership for Advancing Spine Care Imaging Technologies
- RSNA 2024 Technical Exhibits to Showcase Latest Advances in Radiology
- Bracco Collaborates with Arrayus on Microbubble-Assisted Focused Ultrasound Therapy for Pancreatic Cancer
- Innovative Collaboration to Enhance Ischemic Stroke Detection and Elevate Standards in Diagnostic Imaging
- RSNA 2024 Registration Opens
- Microsoft collaborates with Leading Academic Medical Systems to Advance AI in Medical Imaging
- GE HealthCare Acquires Intelligent Ultrasound Group’s Clinical Artificial Intelligence Business
- Bayer and Rad AI Collaborate on Expanding Use of Cutting Edge AI Radiology Operational Solutions
- Polish Med-Tech Company BrainScan to Expand Extensively into Foreign Markets
- Hologic Acquires UK-Based Breast Surgical Guidance Company Endomagnetics Ltd.
Channels
Radiography
view channel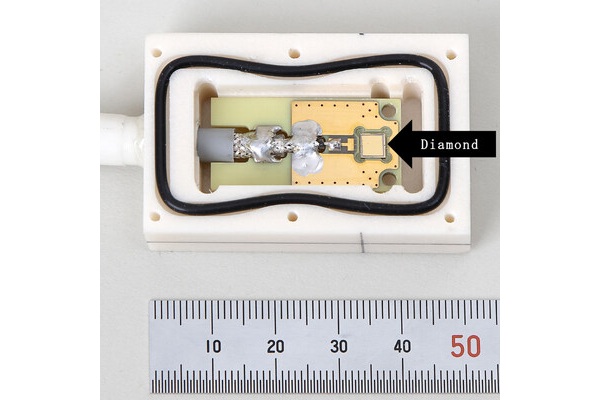
World's Largest Class Single Crystal Diamond Radiation Detector Opens New Possibilities for Diagnostic Imaging
Diamonds possess ideal physical properties for radiation detection, such as exceptional thermal and chemical stability along with a quick response time. Made of carbon with an atomic number of six, diamonds... Read more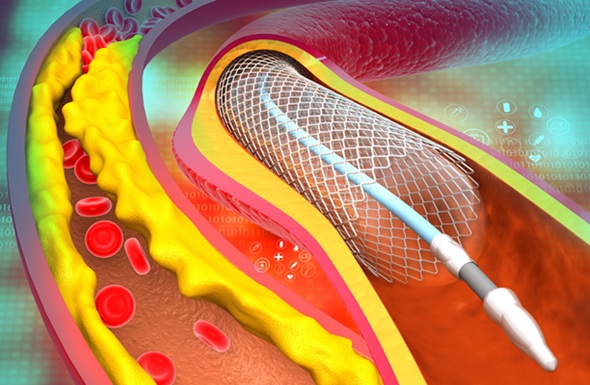
AI-Powered Imaging Technique Shows Promise in Evaluating Patients for PCI
Percutaneous coronary intervention (PCI), also known as coronary angioplasty, is a minimally invasive procedure where small metal tubes called stents are inserted into partially blocked coronary arteries... Read moreMRI
view channel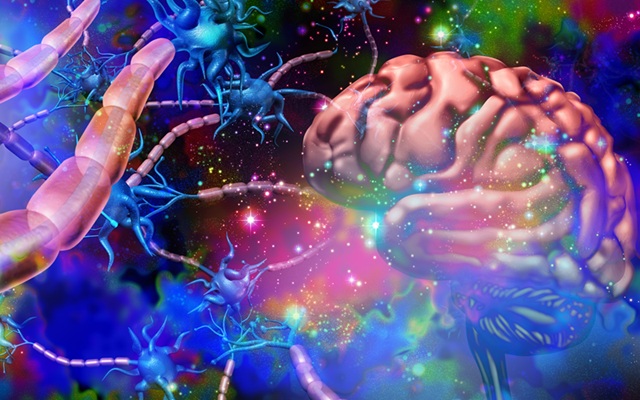
AI Tool Tracks Effectiveness of Multiple Sclerosis Treatments Using Brain MRI Scans
Multiple sclerosis (MS) is a condition in which the immune system attacks the brain and spinal cord, leading to impairments in movement, sensation, and cognition. Magnetic Resonance Imaging (MRI) markers... Read more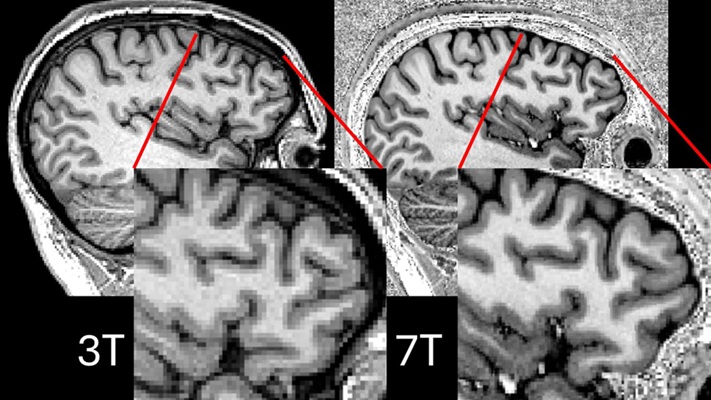
Ultra-Powerful MRI Scans Enable Life-Changing Surgery in Treatment-Resistant Epileptic Patients
Approximately 360,000 individuals in the UK suffer from focal epilepsy, a condition in which seizures spread from one part of the brain. Around a third of these patients experience persistent seizures... Read more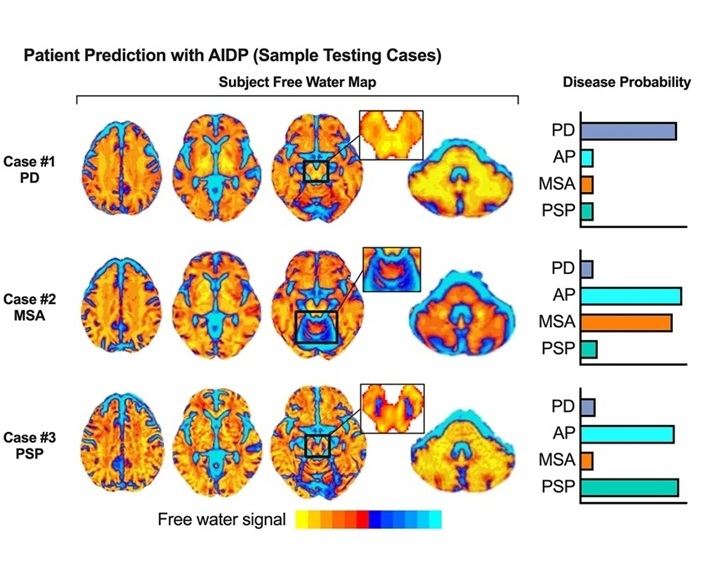
AI-Powered MRI Technology Improves Parkinson’s Diagnoses
Current research shows that the accuracy of diagnosing Parkinson’s disease typically ranges from 55% to 78% within the first five years of assessment. This is partly due to the similarities shared by Parkinson’s... Read more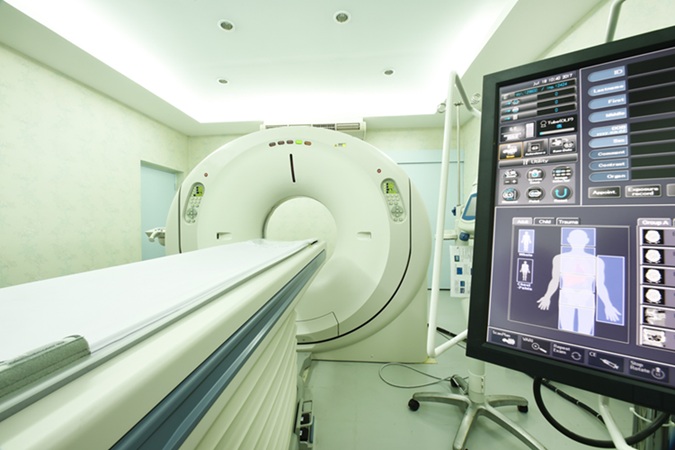
Biparametric MRI Combined with AI Enhances Detection of Clinically Significant Prostate Cancer
Artificial intelligence (AI) technologies are transforming the way medical images are analyzed, offering unprecedented capabilities in quantitatively extracting features that go beyond traditional visual... Read moreUltrasound
view channel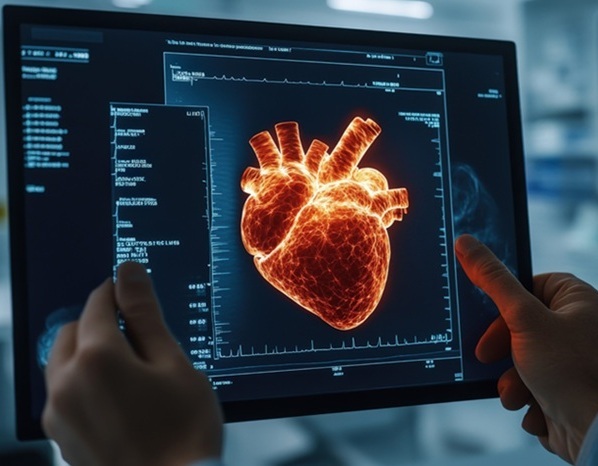
AI Identifies Heart Valve Disease from Common Imaging Test
Tricuspid regurgitation is a condition where the heart's tricuspid valve does not close completely during contraction, leading to backward blood flow, which can result in heart failure. A new artificial... Read more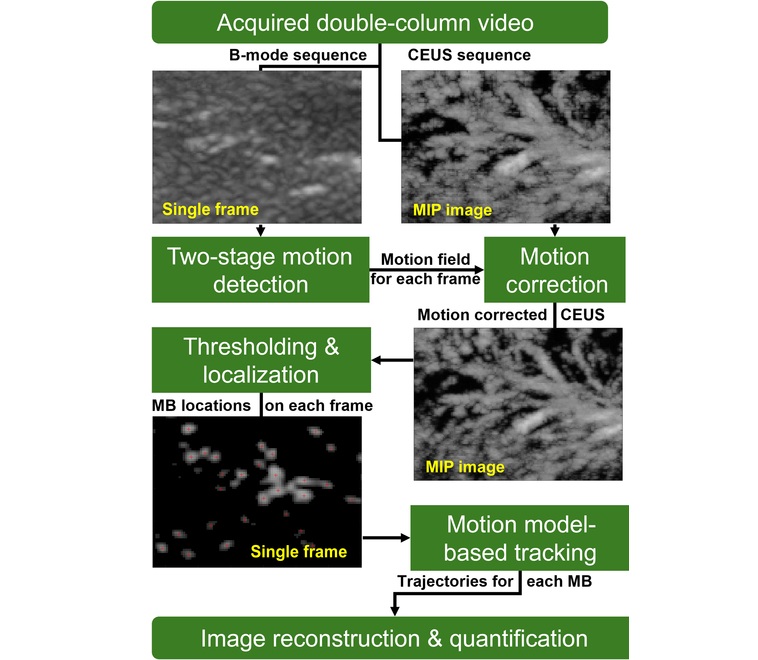
Novel Imaging Method Enables Early Diagnosis and Treatment Monitoring of Type 2 Diabetes
Type 2 diabetes is recognized as an autoimmune inflammatory disease, where chronic inflammation leads to alterations in pancreatic islet microvasculature, a key factor in β-cell dysfunction.... Read moreNuclear Medicine
view channel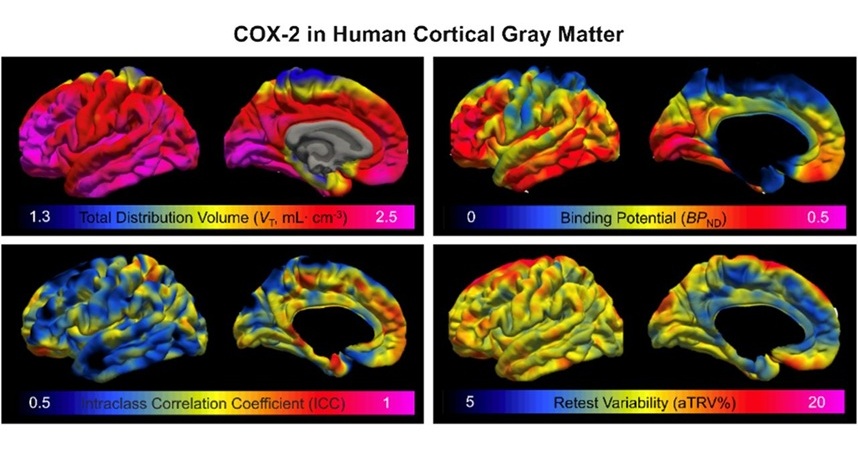
Novel PET Imaging Approach Offers Never-Before-Seen View of Neuroinflammation
COX-2, an enzyme that plays a key role in brain inflammation, can be significantly upregulated by inflammatory stimuli and neuroexcitation. Researchers suggest that COX-2 density in the brain could serve... Read more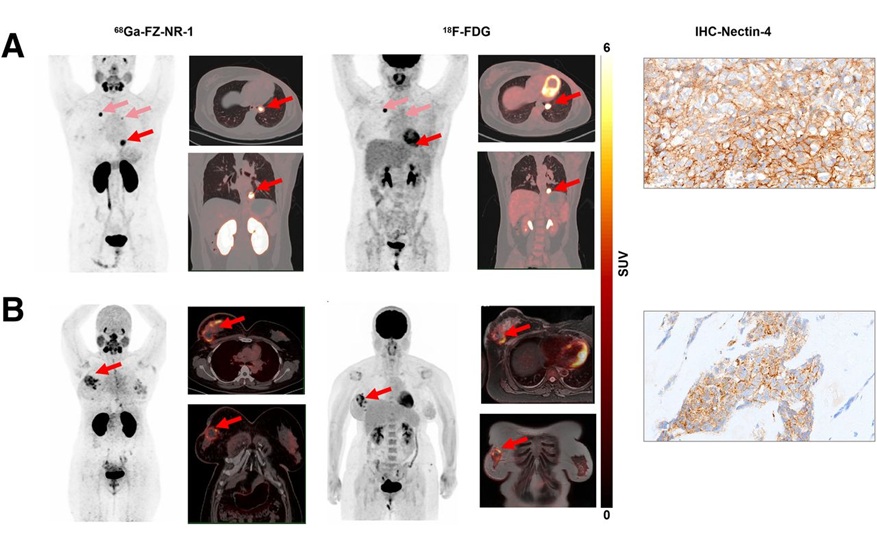
Novel Radiotracer Identifies Biomarker for Triple-Negative Breast Cancer
Triple-negative breast cancer (TNBC), which represents 15-20% of all breast cancer cases, is one of the most aggressive subtypes, with a five-year survival rate of about 40%. Due to its significant heterogeneity... Read moreGeneral/Advanced Imaging
view channel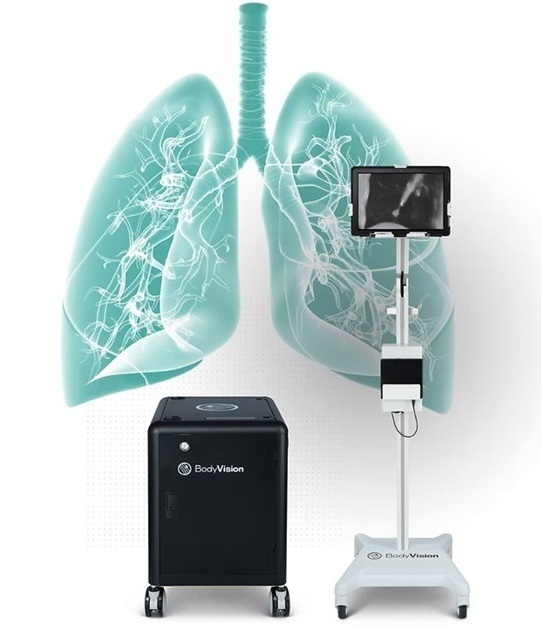
AI-Powered Imaging System Improves Lung Cancer Diagnosis
Given the need to detect lung cancer at earlier stages, there is an increasing need for a definitive diagnostic pathway for patients with suspicious pulmonary nodules. However, obtaining tissue samples... Read more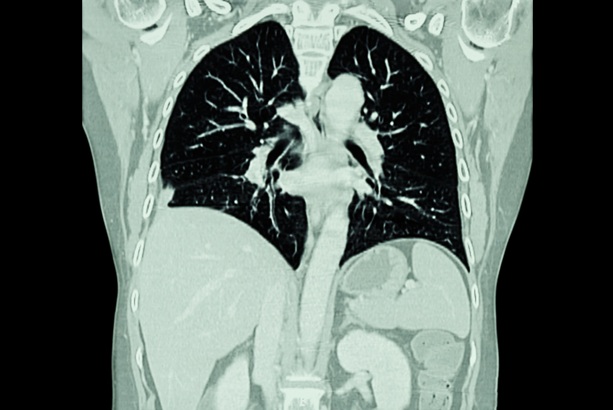
AI Model Significantly Enhances Low-Dose CT Capabilities
Lung cancer remains one of the most challenging diseases, making early diagnosis vital for effective treatment. Fortunately, advancements in artificial intelligence (AI) are revolutionizing lung cancer... Read moreImaging IT
view channel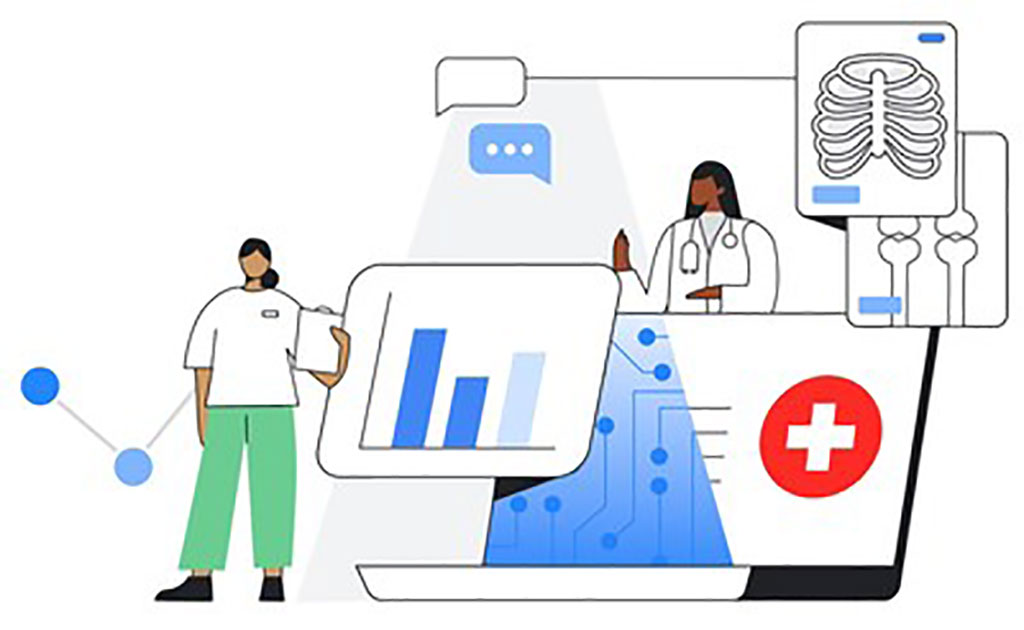
New Google Cloud Medical Imaging Suite Makes Imaging Healthcare Data More Accessible
Medical imaging is a critical tool used to diagnose patients, and there are billions of medical images scanned globally each year. Imaging data accounts for about 90% of all healthcare data1 and, until... Read more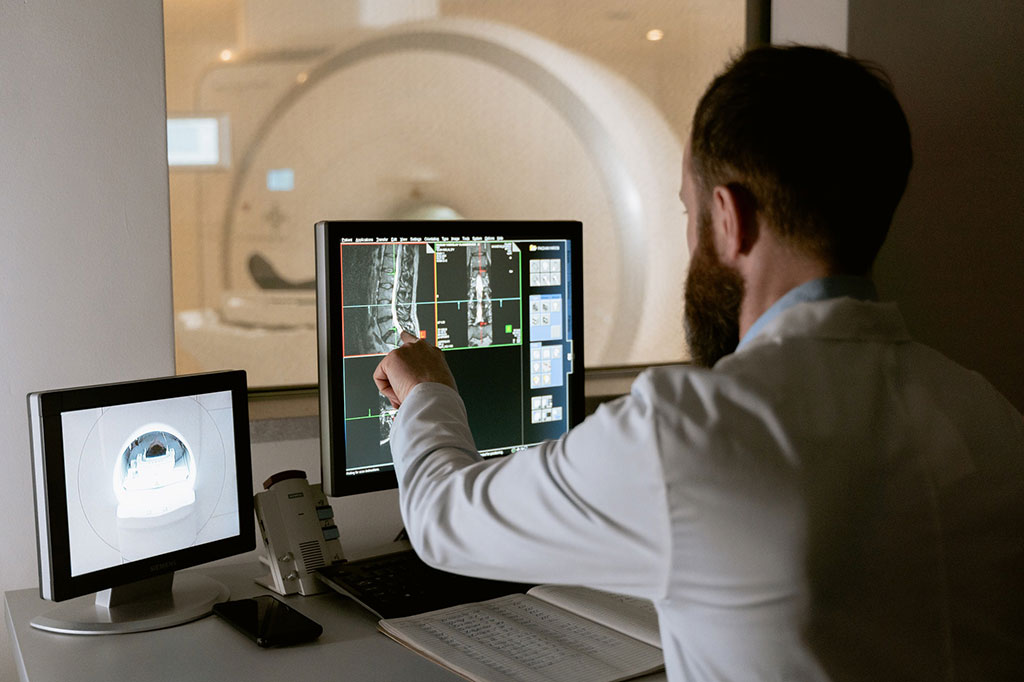