Artificial Intelligence Could Help Reduce Gadolinium Dose in MRI
By MedImaging International staff writers Posted on 18 Dec 2018 |
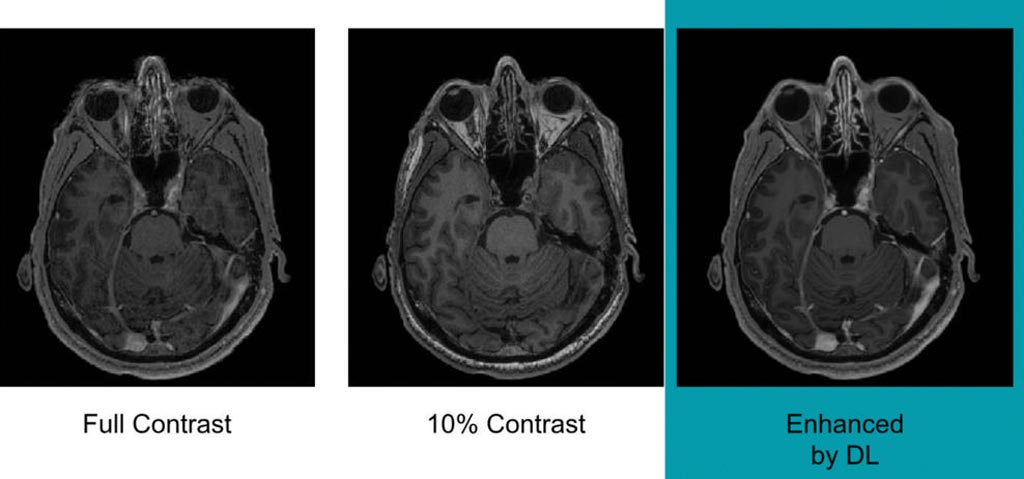
Image: Example of full-dose, 10 percent low-dose and algorithm-enhanced low-dose (Photo courtesy of RSNA).
Researchers from Stanford University (Stanford, CA, USA) are using artificial intelligence (AI) to reduce the dose of a contrast agent that may be left behind in the body after MRI exams.
Gadolinium is a heavy metal used in contrast material that enhances MRI images. Recent studies have found that trace amounts of the metal remain in the bodies of people who have undergone exams with certain types of gadolinium. The effects of this deposition are not yet known, although radiologists are working to optimize patient safety while simultaneously preserving the important information provided by gadolinium-enhanced MRI scans. The Stanford researchers have been doing this by studying deep learning, a sophisticated AI technique that teaches computers by examples. By using models called convolutional neural networks, computers can recognize images as well as find subtle distinctions among the imaging data that a human observer might be incapable of discerning.
In order to train the deep learning algorithm, the researchers used MR images from 200 patients who had received contrast-enhanced MRI exams for various indications. They collected three sets of images for each patient: pre-contrast scans, done prior to contrast administration and referred to as the zero-dose scans; low-dose scans, acquired after 10% of the standard gadolinium dose administration; and full-dose scans, acquired after 100% dose administration. The algorithm learned to approximate the full-dose scans from the zero-dose and low-dose images. Neuroradiologists then evaluated the images for contrast enhancement and overall quality.
The results showed that the image quality was not significantly different between the low-dose, algorithm-enhanced MR images and the full-dose, contrast-enhanced MR images. The initial results also demonstrated the potential for creating the equivalent of full-dose, contrast-enhanced MR images without any contrast agent use. These findings suggest the method’s potential for dramatically reducing gadolinium dose without sacrificing diagnostic quality. Future research in the clinical setting will focus on evaluation of the algorithm across a broader range of MRI scanners and with different types of contrast agents.
“Low-dose gadolinium images yield significant untapped clinically useful information that is accessible now by using deep learning and AI,” said study lead author Enhao Gong, Ph.D., researcher at Stanford University. “We’re not trying to replace existing imaging technology. We’re trying to improve it and generate more value from the existing information while looking out for the safety of our patients.”
Related Links:
Stanford University
Gadolinium is a heavy metal used in contrast material that enhances MRI images. Recent studies have found that trace amounts of the metal remain in the bodies of people who have undergone exams with certain types of gadolinium. The effects of this deposition are not yet known, although radiologists are working to optimize patient safety while simultaneously preserving the important information provided by gadolinium-enhanced MRI scans. The Stanford researchers have been doing this by studying deep learning, a sophisticated AI technique that teaches computers by examples. By using models called convolutional neural networks, computers can recognize images as well as find subtle distinctions among the imaging data that a human observer might be incapable of discerning.
In order to train the deep learning algorithm, the researchers used MR images from 200 patients who had received contrast-enhanced MRI exams for various indications. They collected three sets of images for each patient: pre-contrast scans, done prior to contrast administration and referred to as the zero-dose scans; low-dose scans, acquired after 10% of the standard gadolinium dose administration; and full-dose scans, acquired after 100% dose administration. The algorithm learned to approximate the full-dose scans from the zero-dose and low-dose images. Neuroradiologists then evaluated the images for contrast enhancement and overall quality.
The results showed that the image quality was not significantly different between the low-dose, algorithm-enhanced MR images and the full-dose, contrast-enhanced MR images. The initial results also demonstrated the potential for creating the equivalent of full-dose, contrast-enhanced MR images without any contrast agent use. These findings suggest the method’s potential for dramatically reducing gadolinium dose without sacrificing diagnostic quality. Future research in the clinical setting will focus on evaluation of the algorithm across a broader range of MRI scanners and with different types of contrast agents.
“Low-dose gadolinium images yield significant untapped clinically useful information that is accessible now by using deep learning and AI,” said study lead author Enhao Gong, Ph.D., researcher at Stanford University. “We’re not trying to replace existing imaging technology. We’re trying to improve it and generate more value from the existing information while looking out for the safety of our patients.”
Related Links:
Stanford University
Latest Industry News News
- GE HealthCare and NVIDIA Collaboration to Reimagine Diagnostic Imaging
- Patient-Specific 3D-Printed Phantoms Transform CT Imaging
- Siemens and Sectra Collaborate on Enhancing Radiology Workflows
- Bracco Diagnostics and ColoWatch Partner to Expand Availability CRC Screening Tests Using Virtual Colonoscopy
- Mindray Partners with TeleRay to Streamline Ultrasound Delivery
- Philips and Medtronic Partner on Stroke Care
- Siemens and Medtronic Enter into Global Partnership for Advancing Spine Care Imaging Technologies
- RSNA 2024 Technical Exhibits to Showcase Latest Advances in Radiology
- Bracco Collaborates with Arrayus on Microbubble-Assisted Focused Ultrasound Therapy for Pancreatic Cancer
- Innovative Collaboration to Enhance Ischemic Stroke Detection and Elevate Standards in Diagnostic Imaging
- RSNA 2024 Registration Opens
- Microsoft collaborates with Leading Academic Medical Systems to Advance AI in Medical Imaging
- GE HealthCare Acquires Intelligent Ultrasound Group’s Clinical Artificial Intelligence Business
- Bayer and Rad AI Collaborate on Expanding Use of Cutting Edge AI Radiology Operational Solutions
- Polish Med-Tech Company BrainScan to Expand Extensively into Foreign Markets
- Hologic Acquires UK-Based Breast Surgical Guidance Company Endomagnetics Ltd.
Channels
Radiography
view channel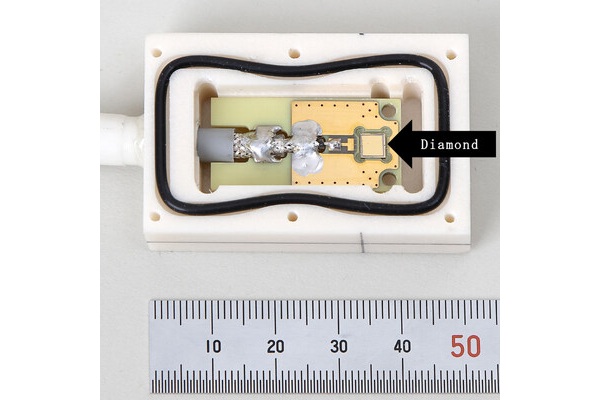
World's Largest Class Single Crystal Diamond Radiation Detector Opens New Possibilities for Diagnostic Imaging
Diamonds possess ideal physical properties for radiation detection, such as exceptional thermal and chemical stability along with a quick response time. Made of carbon with an atomic number of six, diamonds... Read more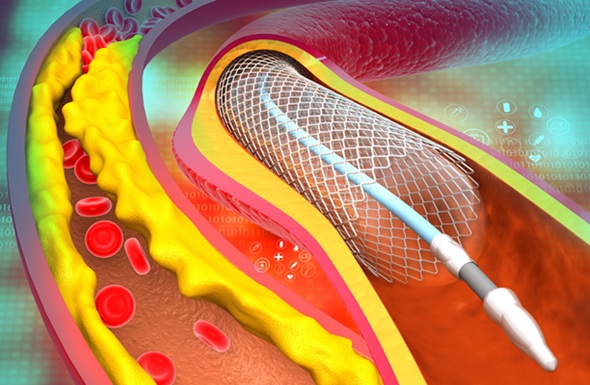
AI-Powered Imaging Technique Shows Promise in Evaluating Patients for PCI
Percutaneous coronary intervention (PCI), also known as coronary angioplasty, is a minimally invasive procedure where small metal tubes called stents are inserted into partially blocked coronary arteries... Read moreMRI
view channel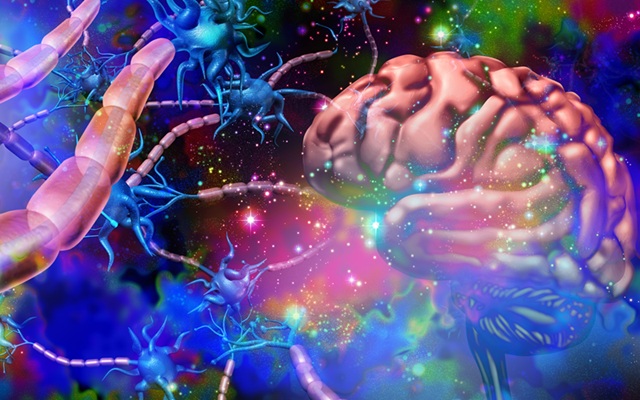
AI Tool Tracks Effectiveness of Multiple Sclerosis Treatments Using Brain MRI Scans
Multiple sclerosis (MS) is a condition in which the immune system attacks the brain and spinal cord, leading to impairments in movement, sensation, and cognition. Magnetic Resonance Imaging (MRI) markers... Read more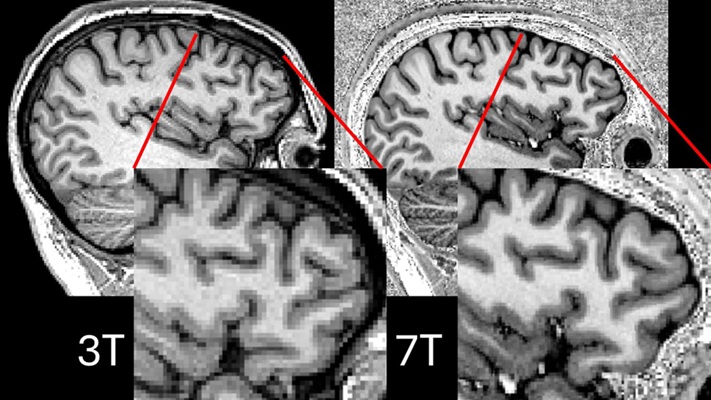
Ultra-Powerful MRI Scans Enable Life-Changing Surgery in Treatment-Resistant Epileptic Patients
Approximately 360,000 individuals in the UK suffer from focal epilepsy, a condition in which seizures spread from one part of the brain. Around a third of these patients experience persistent seizures... Read more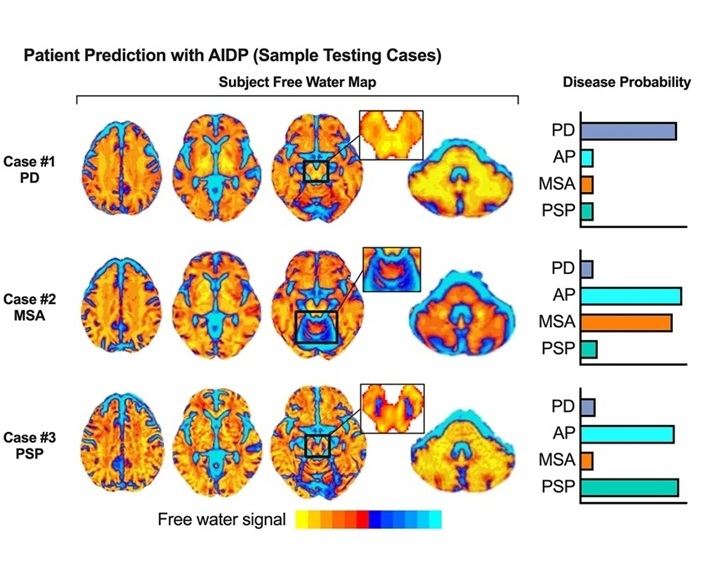
AI-Powered MRI Technology Improves Parkinson’s Diagnoses
Current research shows that the accuracy of diagnosing Parkinson’s disease typically ranges from 55% to 78% within the first five years of assessment. This is partly due to the similarities shared by Parkinson’s... Read more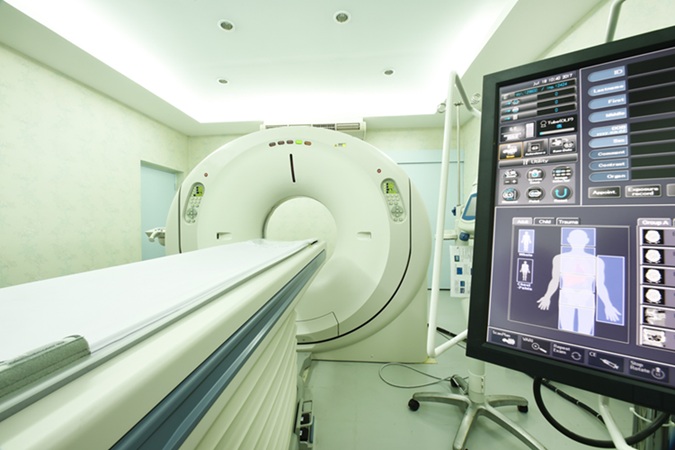
Biparametric MRI Combined with AI Enhances Detection of Clinically Significant Prostate Cancer
Artificial intelligence (AI) technologies are transforming the way medical images are analyzed, offering unprecedented capabilities in quantitatively extracting features that go beyond traditional visual... Read moreUltrasound
view channel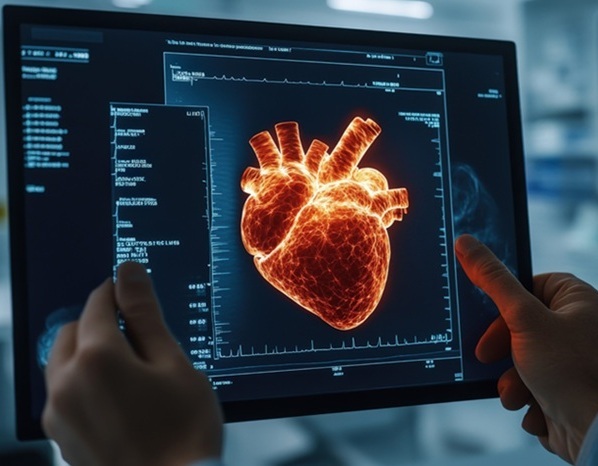
AI Identifies Heart Valve Disease from Common Imaging Test
Tricuspid regurgitation is a condition where the heart's tricuspid valve does not close completely during contraction, leading to backward blood flow, which can result in heart failure. A new artificial... Read more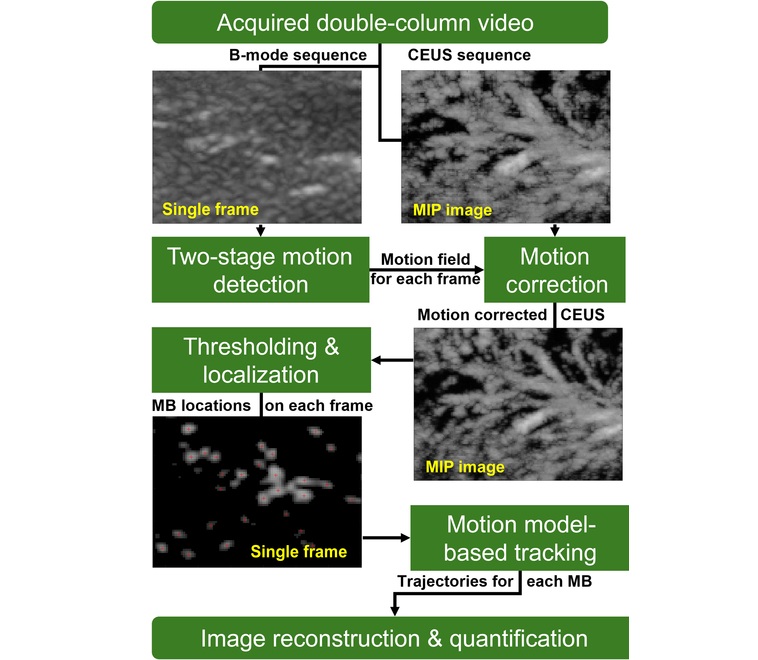
Novel Imaging Method Enables Early Diagnosis and Treatment Monitoring of Type 2 Diabetes
Type 2 diabetes is recognized as an autoimmune inflammatory disease, where chronic inflammation leads to alterations in pancreatic islet microvasculature, a key factor in β-cell dysfunction.... Read moreNuclear Medicine
view channel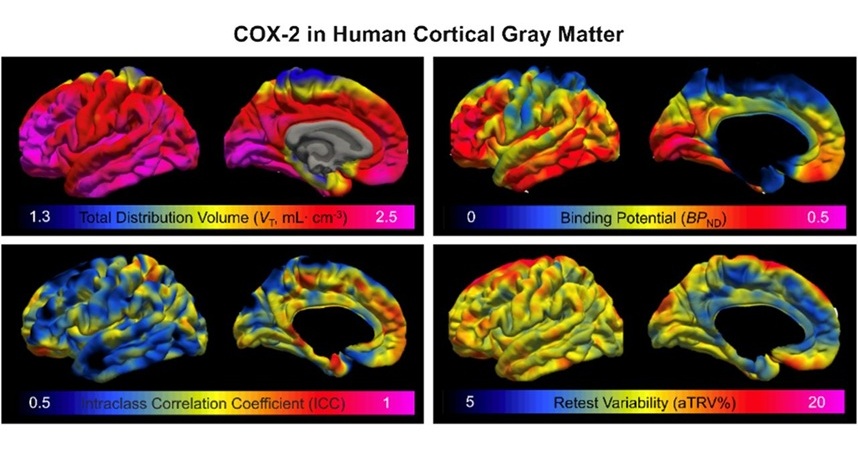
Novel PET Imaging Approach Offers Never-Before-Seen View of Neuroinflammation
COX-2, an enzyme that plays a key role in brain inflammation, can be significantly upregulated by inflammatory stimuli and neuroexcitation. Researchers suggest that COX-2 density in the brain could serve... Read more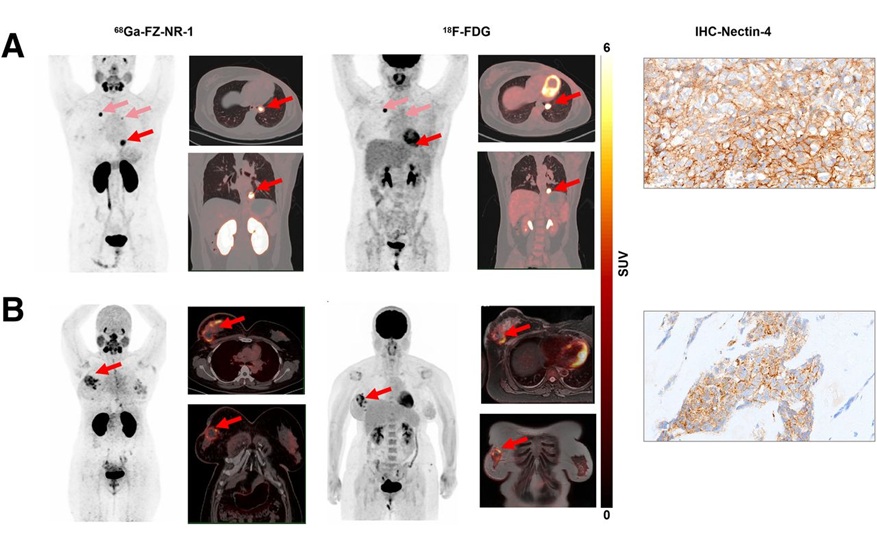
Novel Radiotracer Identifies Biomarker for Triple-Negative Breast Cancer
Triple-negative breast cancer (TNBC), which represents 15-20% of all breast cancer cases, is one of the most aggressive subtypes, with a five-year survival rate of about 40%. Due to its significant heterogeneity... Read moreGeneral/Advanced Imaging
view channel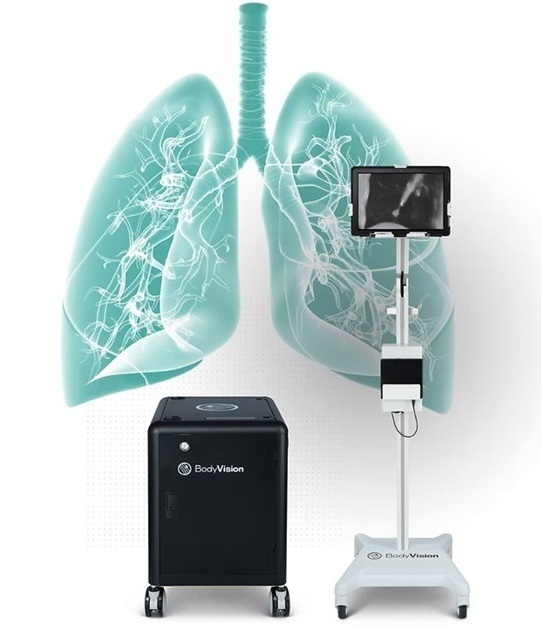
AI-Powered Imaging System Improves Lung Cancer Diagnosis
Given the need to detect lung cancer at earlier stages, there is an increasing need for a definitive diagnostic pathway for patients with suspicious pulmonary nodules. However, obtaining tissue samples... Read more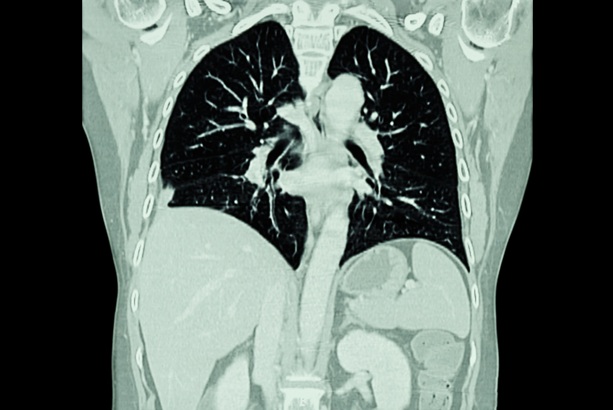
AI Model Significantly Enhances Low-Dose CT Capabilities
Lung cancer remains one of the most challenging diseases, making early diagnosis vital for effective treatment. Fortunately, advancements in artificial intelligence (AI) are revolutionizing lung cancer... Read moreImaging IT
view channel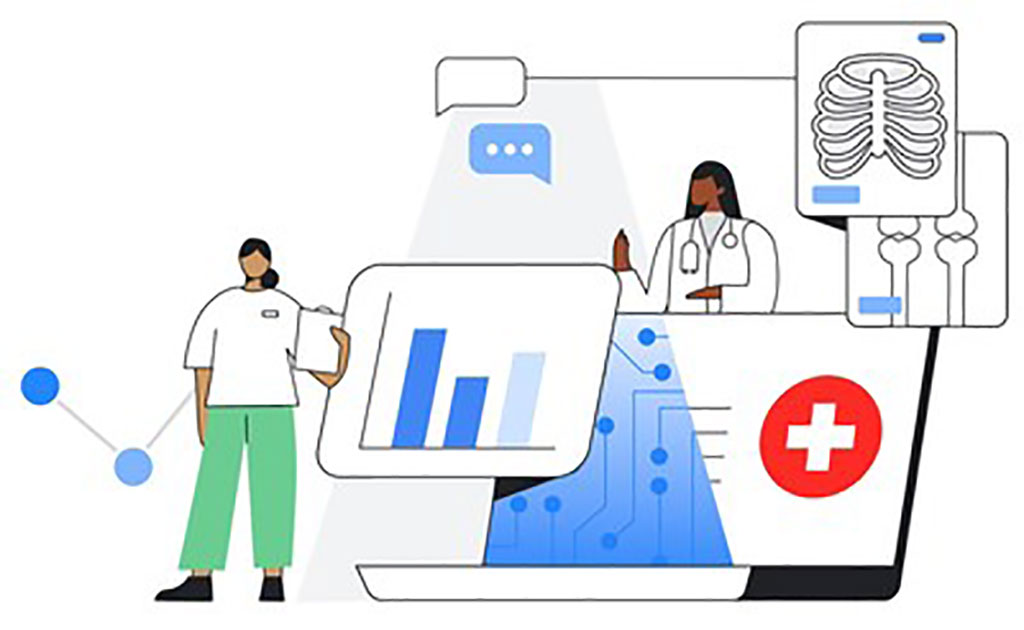
New Google Cloud Medical Imaging Suite Makes Imaging Healthcare Data More Accessible
Medical imaging is a critical tool used to diagnose patients, and there are billions of medical images scanned globally each year. Imaging data accounts for about 90% of all healthcare data1 and, until... Read more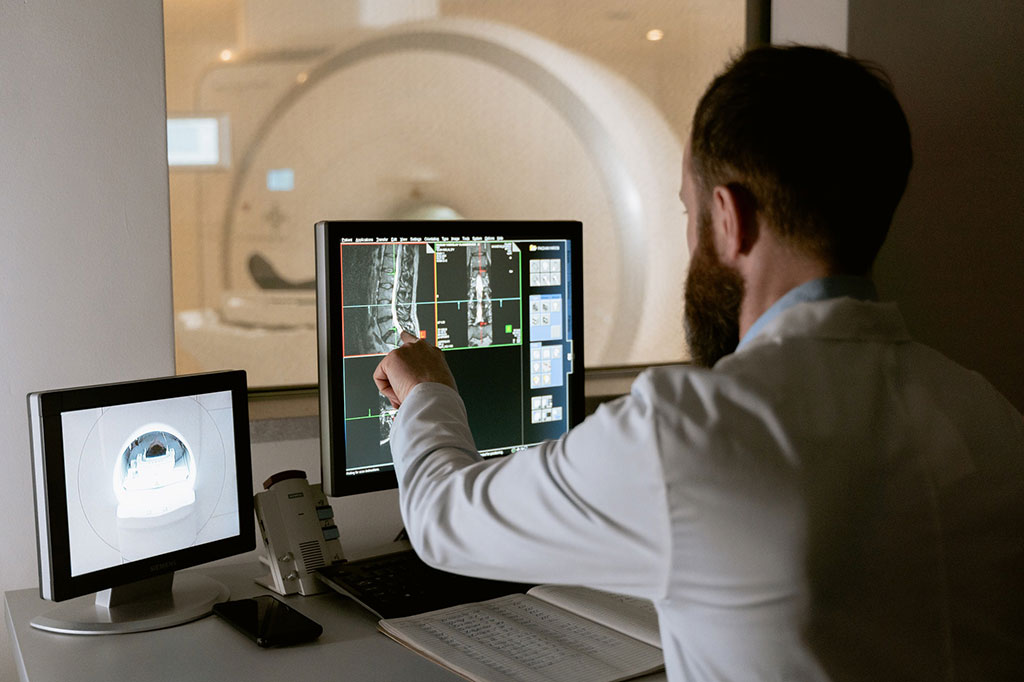