MIT Researchers Develop AI Tool That Compares 3D Scans
By MedImaging International staff writers Posted on 26 Jun 2018 |
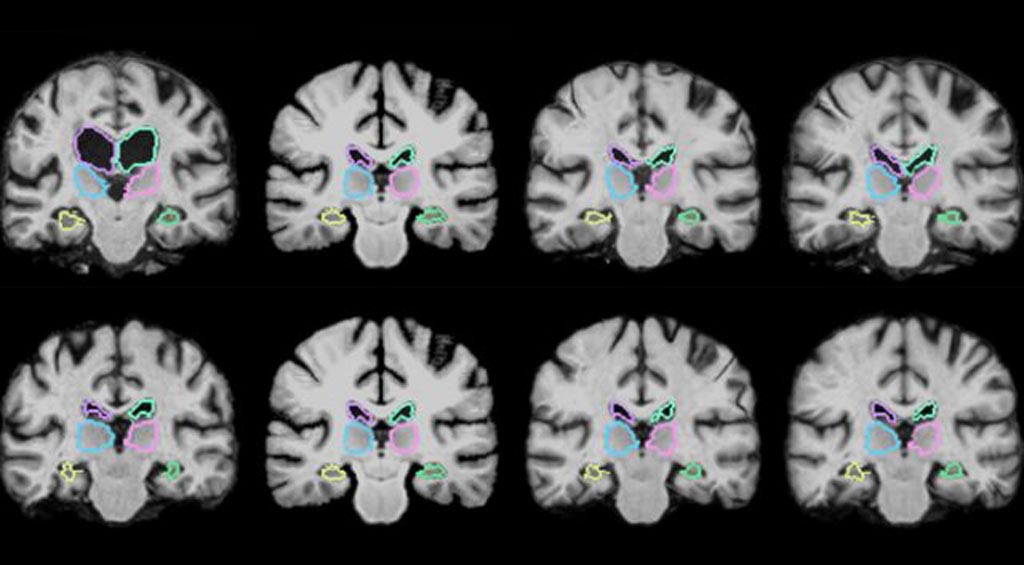
Image: The VoxelMorph algorithm is designed to register brain scans and other 3D images more than 1,000 times more quickly using novel learning techniques (Photo courtesy of MIT).
A team of researchers from the Massachusetts Institute of Technology (Cambridge, MA, USA) have submitted a pair of conference papers describing a machine-learning algorithm that can register brain scans and other 3D images more than 1,000 times more quickly using novel learning techniques. The algorithm works by “learning” while registering thousands of pairs of images and acquiring information during this process about how to align the images and estimating some optimal alignment parameters. After training, the algorithm uses those parameters to map all pixels of one image to another simultaneously. This reduces registration time to a minute or two using a normal computer, or less than a second using a GPU with accuracy similar to state-of-the-art systems.
In order to analyze variations in brain structures in patients with a particular disease or condition, neuroscientists often use the medical image registration technique. This involves overlaying two images, such as magnetic resonance imaging (MRI) scans, to compare and analyze the anatomical differences in great detail. However, this process usually takes two hours or more, as traditional systems meticulously align each of potentially a million pixels in the combined scans. Since MRI scans are basically hundreds of stacked 2D images that form massive 3D images, called “volumes,” containing a million or more 3D pixels, called “voxels,” it can be highly time-consuming to align all voxels in the first volume with those in the second. Additionally, scans coming from different machines and having different spatial orientations can make matching voxels even more computationally complex.
The researchers’ algorithm, called “VoxelMorph,” is powered by a convolutional neural network (CNN), a machine-learning approach commonly used for image processing. These networks consist of several nodes that process image and other information across various layers of computation. The researchers trained their algorithm on 7,000 publicly available MRI brain scans and then tested it on 250 additional scans. During training, the researchers fed the brain scans into the algorithm in pairs. Using a CNN and modified computation layer called a spatial transformer, the method captures similarities of voxels in one MRI scan with voxels in the other scan. This allows the algorithm to learn information about groups of voxels, such as anatomical shapes common to both scans, which it uses to calculate optimized parameters that can be applied to any scan pair.
When two new scans are fed into the algorithm, a simple mathematical “function” uses those optimized parameters to rapidly calculate the exact alignment of every voxel in both scans. Thus, the algorithm’s CNN component gains all the necessary information during training so that, during each new registration, the entire registration can be executed using one, easily computable function evaluation. The researchers found that their algorithm could accurately register all of their 250 test brain scans – those registered after the training set – within two minutes using a traditional central processing unit, and in under one second using a graphics-processing unit. What is particularly noteworthy is that the algorithm is “unsupervised,” which means it does not require additional information apart from image data. Some registration algorithms incorporate CNN models but require a “ground truth,” which means that another traditional algorithm is first run to compute accurate registrations. However, the algorithm developed by the MIT researchers maintains its accuracy without that data.
In addition to analyzing brain scans, the speedy algorithm could find a wide range of potential applications, according to the researchers. For instance, other researchers at MIT are currently running the algorithm on lung images. The algorithm could also allow for image registration during operations and allow surgeons to potentially register scans in near real-time, getting a much clearer picture on the progress of the surgery.
"This is a case where a big enough quantitative change [of image registration] – from hours to seconds – becomes a qualitative one, opening up new possibilities such as running the algorithm during a scan session while a patient is still in the scanner, enabling clinical decision making about what types of data needs to be acquired and where in the brain it should be focused without forcing the patient to come back days or weeks later," said Bruce Fischl, a professor in radiology at Harvard Medical School and a neuroscientist at Massachusetts General Hospital.
The papers were presented by the MIT researchers at the Conference on Computer Vision and Pattern Recognition (CVPR) and at the Medical Image Computing and Computer Assisted Interventions Conference (MICCAI).
Related Links:
Massachusetts Institute of Technology
In order to analyze variations in brain structures in patients with a particular disease or condition, neuroscientists often use the medical image registration technique. This involves overlaying two images, such as magnetic resonance imaging (MRI) scans, to compare and analyze the anatomical differences in great detail. However, this process usually takes two hours or more, as traditional systems meticulously align each of potentially a million pixels in the combined scans. Since MRI scans are basically hundreds of stacked 2D images that form massive 3D images, called “volumes,” containing a million or more 3D pixels, called “voxels,” it can be highly time-consuming to align all voxels in the first volume with those in the second. Additionally, scans coming from different machines and having different spatial orientations can make matching voxels even more computationally complex.
The researchers’ algorithm, called “VoxelMorph,” is powered by a convolutional neural network (CNN), a machine-learning approach commonly used for image processing. These networks consist of several nodes that process image and other information across various layers of computation. The researchers trained their algorithm on 7,000 publicly available MRI brain scans and then tested it on 250 additional scans. During training, the researchers fed the brain scans into the algorithm in pairs. Using a CNN and modified computation layer called a spatial transformer, the method captures similarities of voxels in one MRI scan with voxels in the other scan. This allows the algorithm to learn information about groups of voxels, such as anatomical shapes common to both scans, which it uses to calculate optimized parameters that can be applied to any scan pair.
When two new scans are fed into the algorithm, a simple mathematical “function” uses those optimized parameters to rapidly calculate the exact alignment of every voxel in both scans. Thus, the algorithm’s CNN component gains all the necessary information during training so that, during each new registration, the entire registration can be executed using one, easily computable function evaluation. The researchers found that their algorithm could accurately register all of their 250 test brain scans – those registered after the training set – within two minutes using a traditional central processing unit, and in under one second using a graphics-processing unit. What is particularly noteworthy is that the algorithm is “unsupervised,” which means it does not require additional information apart from image data. Some registration algorithms incorporate CNN models but require a “ground truth,” which means that another traditional algorithm is first run to compute accurate registrations. However, the algorithm developed by the MIT researchers maintains its accuracy without that data.
In addition to analyzing brain scans, the speedy algorithm could find a wide range of potential applications, according to the researchers. For instance, other researchers at MIT are currently running the algorithm on lung images. The algorithm could also allow for image registration during operations and allow surgeons to potentially register scans in near real-time, getting a much clearer picture on the progress of the surgery.
"This is a case where a big enough quantitative change [of image registration] – from hours to seconds – becomes a qualitative one, opening up new possibilities such as running the algorithm during a scan session while a patient is still in the scanner, enabling clinical decision making about what types of data needs to be acquired and where in the brain it should be focused without forcing the patient to come back days or weeks later," said Bruce Fischl, a professor in radiology at Harvard Medical School and a neuroscientist at Massachusetts General Hospital.
The papers were presented by the MIT researchers at the Conference on Computer Vision and Pattern Recognition (CVPR) and at the Medical Image Computing and Computer Assisted Interventions Conference (MICCAI).
Related Links:
Massachusetts Institute of Technology
Latest Industry News News
- GE HealthCare and NVIDIA Collaboration to Reimagine Diagnostic Imaging
- Patient-Specific 3D-Printed Phantoms Transform CT Imaging
- Siemens and Sectra Collaborate on Enhancing Radiology Workflows
- Bracco Diagnostics and ColoWatch Partner to Expand Availability CRC Screening Tests Using Virtual Colonoscopy
- Mindray Partners with TeleRay to Streamline Ultrasound Delivery
- Philips and Medtronic Partner on Stroke Care
- Siemens and Medtronic Enter into Global Partnership for Advancing Spine Care Imaging Technologies
- RSNA 2024 Technical Exhibits to Showcase Latest Advances in Radiology
- Bracco Collaborates with Arrayus on Microbubble-Assisted Focused Ultrasound Therapy for Pancreatic Cancer
- Innovative Collaboration to Enhance Ischemic Stroke Detection and Elevate Standards in Diagnostic Imaging
- RSNA 2024 Registration Opens
- Microsoft collaborates with Leading Academic Medical Systems to Advance AI in Medical Imaging
- GE HealthCare Acquires Intelligent Ultrasound Group’s Clinical Artificial Intelligence Business
- Bayer and Rad AI Collaborate on Expanding Use of Cutting Edge AI Radiology Operational Solutions
- Polish Med-Tech Company BrainScan to Expand Extensively into Foreign Markets
- Hologic Acquires UK-Based Breast Surgical Guidance Company Endomagnetics Ltd.
Channels
Radiography
view channel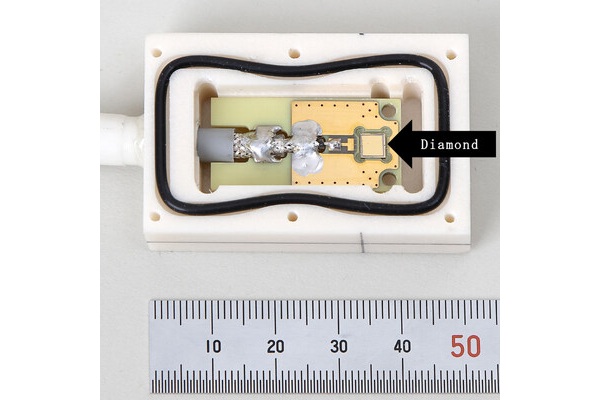
World's Largest Class Single Crystal Diamond Radiation Detector Opens New Possibilities for Diagnostic Imaging
Diamonds possess ideal physical properties for radiation detection, such as exceptional thermal and chemical stability along with a quick response time. Made of carbon with an atomic number of six, diamonds... Read more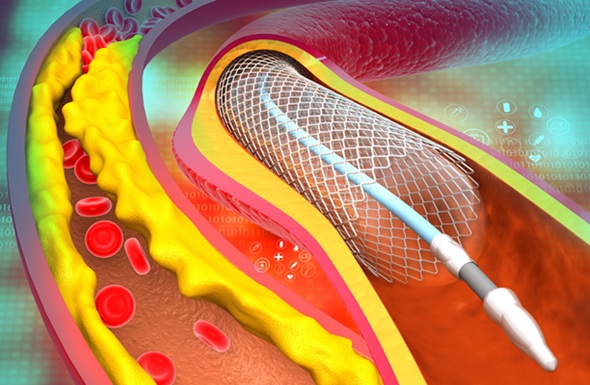
AI-Powered Imaging Technique Shows Promise in Evaluating Patients for PCI
Percutaneous coronary intervention (PCI), also known as coronary angioplasty, is a minimally invasive procedure where small metal tubes called stents are inserted into partially blocked coronary arteries... Read moreMRI
view channel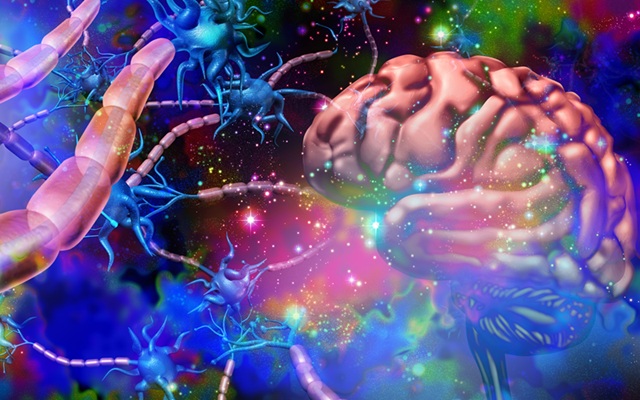
AI Tool Tracks Effectiveness of Multiple Sclerosis Treatments Using Brain MRI Scans
Multiple sclerosis (MS) is a condition in which the immune system attacks the brain and spinal cord, leading to impairments in movement, sensation, and cognition. Magnetic Resonance Imaging (MRI) markers... Read more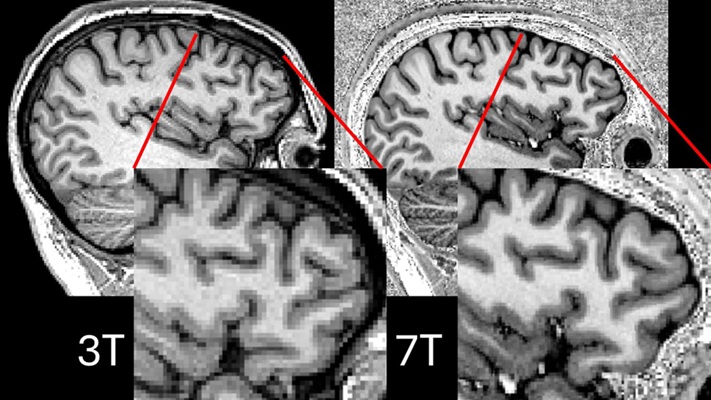
Ultra-Powerful MRI Scans Enable Life-Changing Surgery in Treatment-Resistant Epileptic Patients
Approximately 360,000 individuals in the UK suffer from focal epilepsy, a condition in which seizures spread from one part of the brain. Around a third of these patients experience persistent seizures... Read more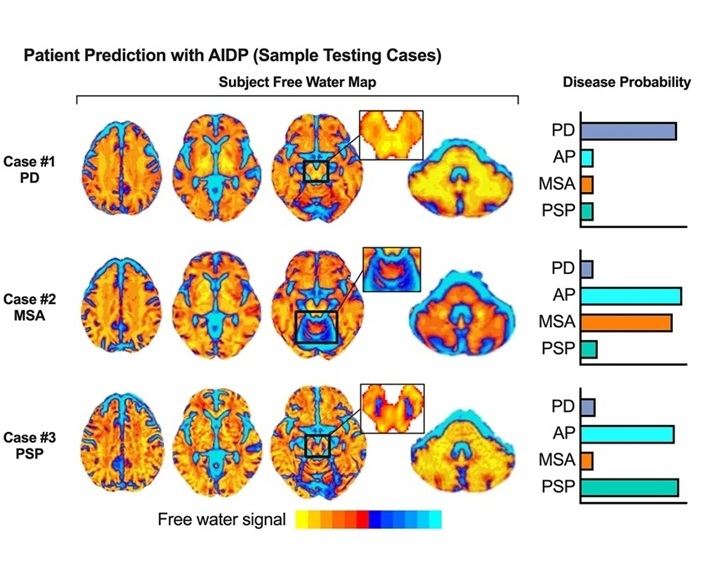
AI-Powered MRI Technology Improves Parkinson’s Diagnoses
Current research shows that the accuracy of diagnosing Parkinson’s disease typically ranges from 55% to 78% within the first five years of assessment. This is partly due to the similarities shared by Parkinson’s... Read more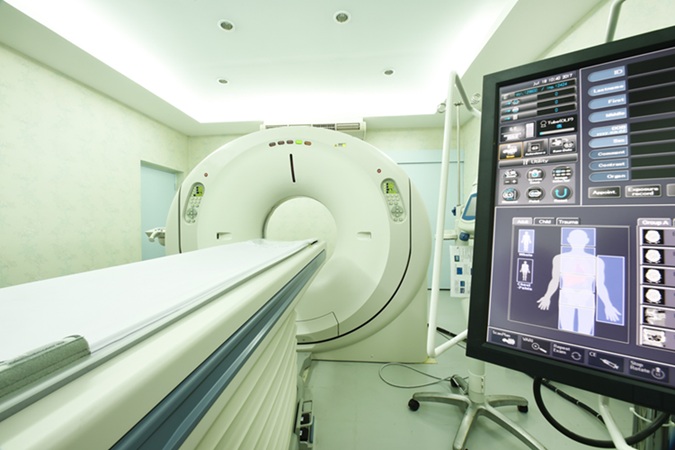
Biparametric MRI Combined with AI Enhances Detection of Clinically Significant Prostate Cancer
Artificial intelligence (AI) technologies are transforming the way medical images are analyzed, offering unprecedented capabilities in quantitatively extracting features that go beyond traditional visual... Read moreUltrasound
view channel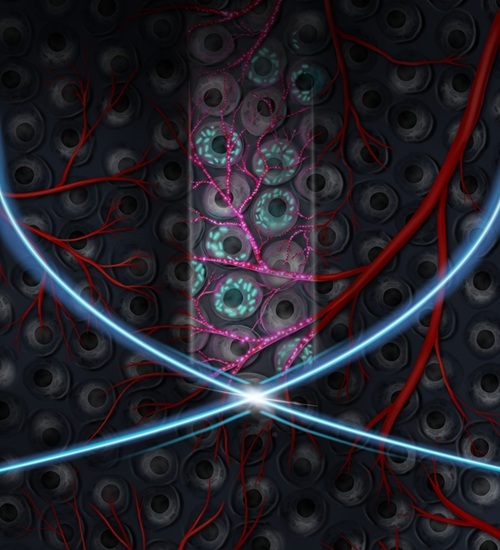
Ultrasound-Based Microscopy Technique to Help Diagnose Small Vessel Diseases
Clinical ultrasound, commonly used in pregnancy scans, provides real-time images of body structures. It is one of the most widely used imaging techniques in medicine, but until recently, it had little... Read more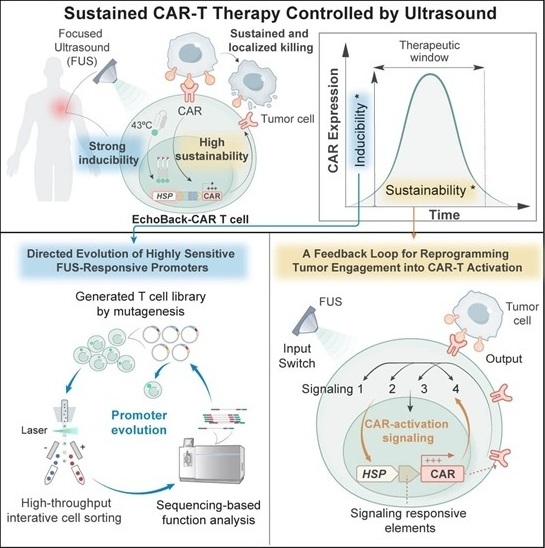
Smart Ultrasound-Activated Immune Cells Destroy Cancer Cells for Extended Periods
Chimeric antigen receptor (CAR) T-cell therapy has emerged as a highly promising cancer treatment, especially for bloodborne cancers like leukemia. This highly personalized therapy involves extracting... Read moreNuclear Medicine
view channel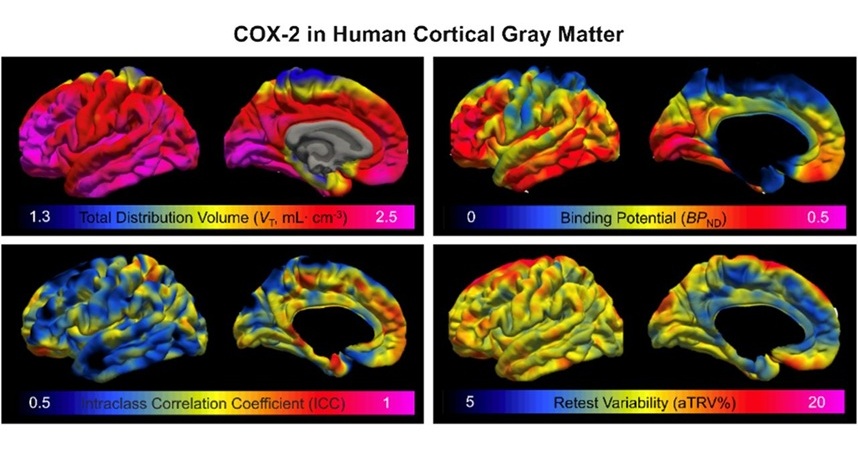
Novel PET Imaging Approach Offers Never-Before-Seen View of Neuroinflammation
COX-2, an enzyme that plays a key role in brain inflammation, can be significantly upregulated by inflammatory stimuli and neuroexcitation. Researchers suggest that COX-2 density in the brain could serve... Read more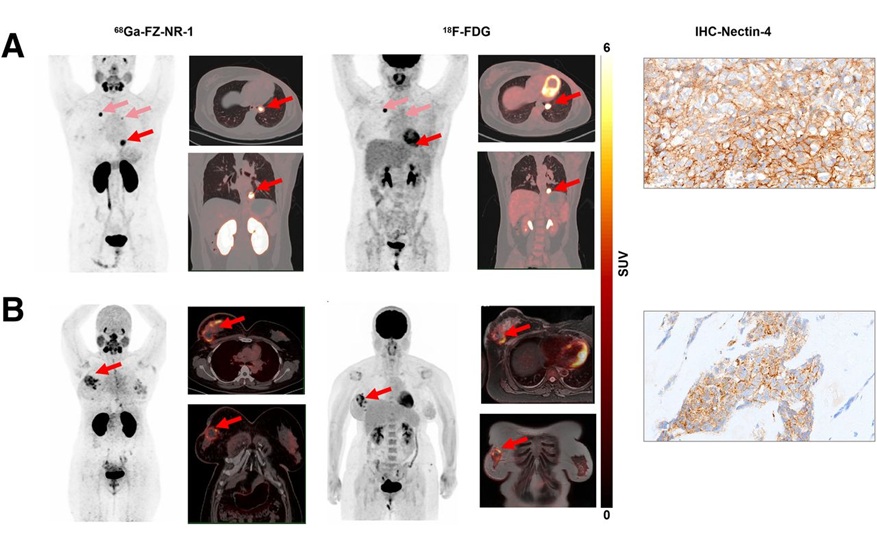
Novel Radiotracer Identifies Biomarker for Triple-Negative Breast Cancer
Triple-negative breast cancer (TNBC), which represents 15-20% of all breast cancer cases, is one of the most aggressive subtypes, with a five-year survival rate of about 40%. Due to its significant heterogeneity... Read moreGeneral/Advanced Imaging
view channel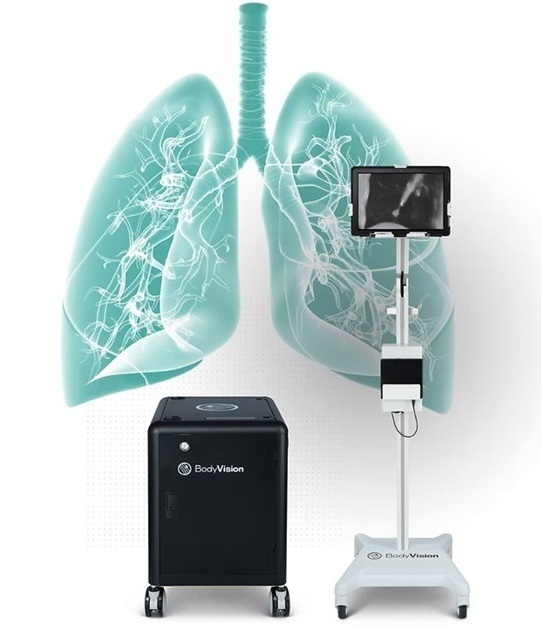
AI-Powered Imaging System Improves Lung Cancer Diagnosis
Given the need to detect lung cancer at earlier stages, there is an increasing need for a definitive diagnostic pathway for patients with suspicious pulmonary nodules. However, obtaining tissue samples... Read more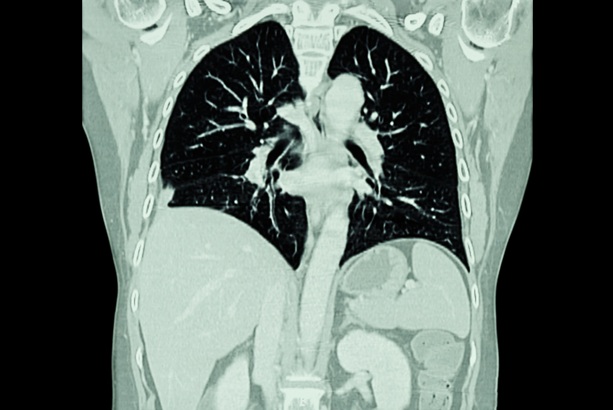
AI Model Significantly Enhances Low-Dose CT Capabilities
Lung cancer remains one of the most challenging diseases, making early diagnosis vital for effective treatment. Fortunately, advancements in artificial intelligence (AI) are revolutionizing lung cancer... Read moreImaging IT
view channel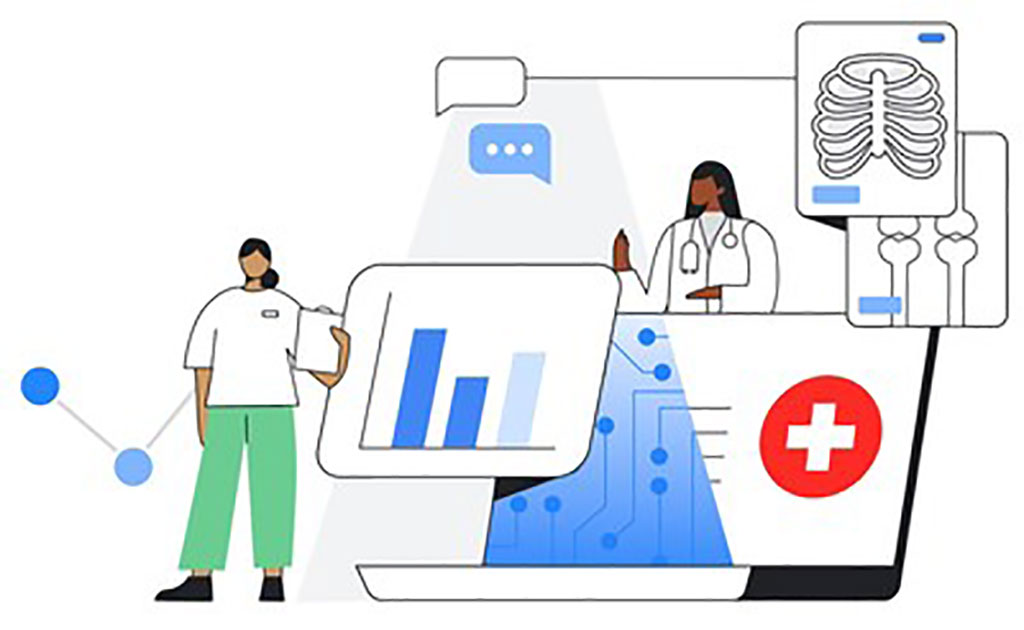
New Google Cloud Medical Imaging Suite Makes Imaging Healthcare Data More Accessible
Medical imaging is a critical tool used to diagnose patients, and there are billions of medical images scanned globally each year. Imaging data accounts for about 90% of all healthcare data1 and, until... Read more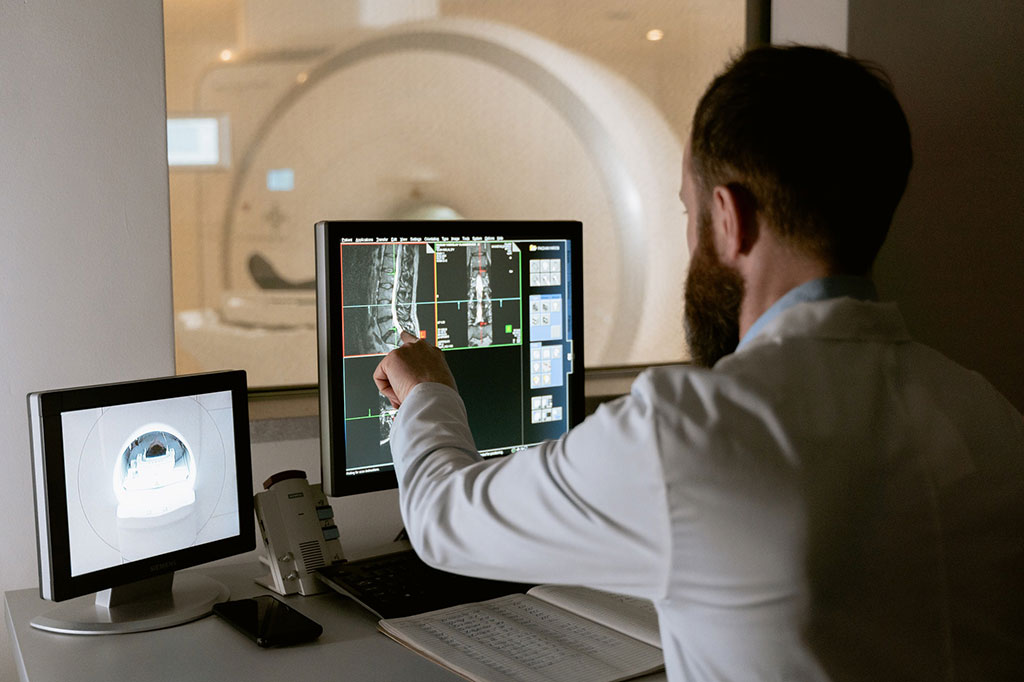