AI Model Draws Maps to Accurately Identify Tumors and Diseases in Medical Images
By MedImaging International staff writers Posted on 05 Mar 2024 |
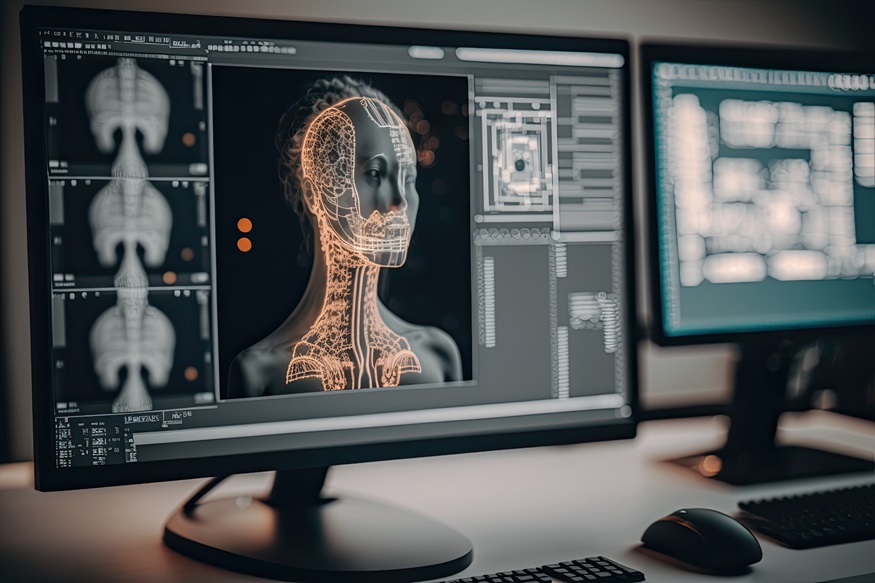
The interpretation of medical images varies across different regions of the world, particularly in developing countries where doctor shortages and long patient queues are common. Artificial Intelligence (AI) has emerged as a valuable aid in these settings. Automated medical image screening using AI can act as a supportive tool for doctors, pre-scanning images and highlighting unusual findings, such as tumors or early disease indicators (biomarkers), for further medical review. This approach not only saves time but can also enhance the accuracy of diagnoses. However, traditional AI models lack the capability to explain their findings, merely indicating the presence or absence of tumors without further elaboration.
Now, researchers at the Beckman Institute for Advanced Science and Technology (Urbana, IL, USA) have developed an innovative AI model that not only detects anomalies but also explains each decision it makes. This model, unlike standard AI tools, provides interpretive feedback rather than just identifying tumors. Conventionally, AI models assisting doctors are trained with numerous medical images, some showing abnormalities and others normal. These models, upon encountering a new image, assign a probability score indicating the likelihood of a tumor being present.
This novel AI model goes a step further by offering a visual explanation for its decision-making process through what's known as an "equivalency map" (E-map). This E-map transforms the original medical image, such as an X-ray or mammogram, assigning values to different regions based on their medical significance in predicting anomalies. The model aggregates these values to derive a final diagnostic score. This transparent approach allows doctors to see which areas of the map contributed more significantly to the diagnosis and to investigate these regions more closely, enhancing understanding and answering patient inquiries about the diagnostic process.
The research team trained this model on over 20,000 images across three different disease diagnostic tasks. The model was taught to identify early signs of tumors in simulated mammograms, to detect Drusen buildup in retinal images indicative of macular degeneration, and to recognize cardiomegaly in chest X-rays. When compared to traditional AI systems without self-explanation capabilities, this new model demonstrated comparable accuracy: 77.8% in mammograms, 99.1% in retinal OCT images, and 83% in chest X-rays, matching the existing models' accuracy. The success of this model, which employs a deep neural network mimicking the complexity of human neurons, is attributed to its design inspired by simpler, more interpretable linear neural networks. The researchers aim to extend this model's application to various body parts, with the ability to potentially distinguish between different anomalies in future developments.
"The idea is to help catch cancer and disease in its earliest stages — like an X on a map — and understand how the decision was made. Our model will help streamline that process and make it easier on doctors and patients alike,” said Sourya Sengupta, the study’s lead author and a graduate research assistant at the Beckman Institute.
“I am excited about our tool’s direct benefit to society, not only in terms of improving disease diagnoses, but also improving trust and transparency between doctors and patients,” added principal investigator Mark Anastasio, a Beckman Institute researcher and the Donald Biggar Willet Professor and Head of the Illinois Department of Bioengineering.
Related Links:
Beckman Institute
Latest General/Advanced Imaging News
- Bone Density Test Uses Existing CT Images to Predict Fractures
- AI Predicts Cardiac Risk and Mortality from Routine Chest CT Scans
- Radiation Therapy Computed Tomography Solution Boosts Imaging Accuracy
- PET Scans Reveal Hidden Inflammation in Multiple Sclerosis Patients
- Artificial Intelligence Evaluates Cardiovascular Risk from CT Scans
- New AI Method Captures Uncertainty in Medical Images
- CT Coronary Angiography Reduces Need for Invasive Tests to Diagnose Coronary Artery Disease
- Novel Blood Test Could Reduce Need for PET Imaging of Patients with Alzheimer’s
- CT-Based Deep Learning Algorithm Accurately Differentiates Benign From Malignant Vertebral Fractures
- Minimally Invasive Procedure Could Help Patients Avoid Thyroid Surgery
- Self-Driving Mobile C-Arm Reduces Imaging Time during Surgery
- AR Application Turns Medical Scans Into Holograms for Assistance in Surgical Planning
- Imaging Technology Provides Ground-Breaking New Approach for Diagnosing and Treating Bowel Cancer
- CT Coronary Calcium Scoring Predicts Heart Attacks and Strokes
- AI Model Detects 90% of Lymphatic Cancer Cases from PET and CT Images
- Breakthrough Technology Revolutionizes Breast Imaging
Channels
Radiography
view channel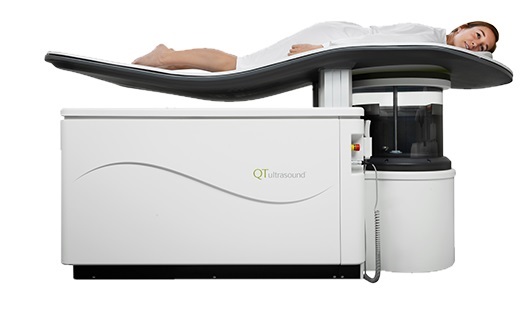
Novel Breast Imaging System Proves As Effective As Mammography
Breast cancer remains the most frequently diagnosed cancer among women. It is projected that one in eight women will be diagnosed with breast cancer during her lifetime, and one in 42 women who turn 50... Read more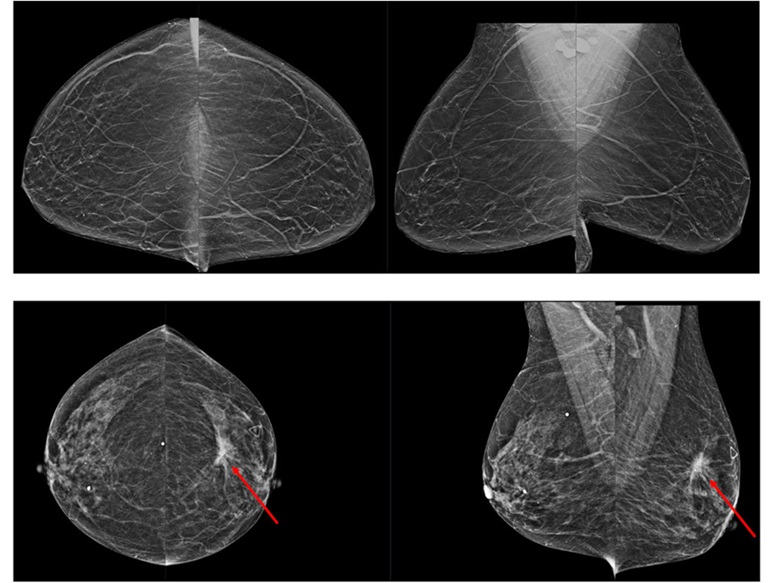
AI Assistance Improves Breast-Cancer Screening by Reducing False Positives
Radiologists typically detect one case of cancer for every 200 mammograms reviewed. However, these evaluations often result in false positives, leading to unnecessary patient recalls for additional testing,... Read moreMRI
view channel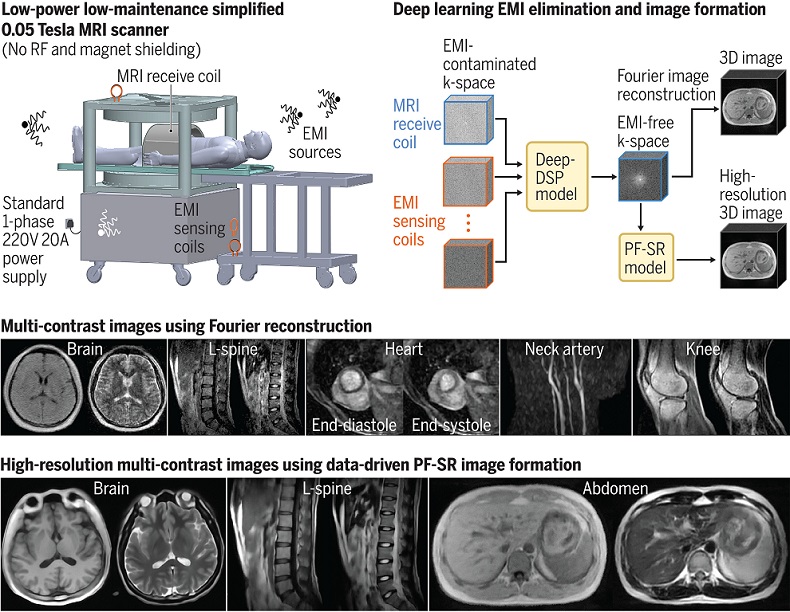
Low-Cost Whole-Body MRI Device Combined with AI Generates High-Quality Results
Magnetic Resonance Imaging (MRI) has significantly transformed healthcare, providing a noninvasive, radiation-free method for detailed imaging. It is especially promising for the future of medical diagnosis... Read more
World's First Whole-Body Ultra-High Field MRI Officially Comes To Market
The world's first whole-body ultra-high field (UHF) MRI has officially come to market, marking a remarkable advancement in diagnostic radiology. United Imaging (Shanghai, China) has secured clearance from the U.... Read moreUltrasound
view channel.jpg)
Diagnostic System Automatically Analyzes TTE Images to Identify Congenital Heart Disease
Congenital heart disease (CHD) is one of the most prevalent congenital anomalies worldwide, presenting substantial health and financial challenges for affected patients. Early detection and treatment of... Read more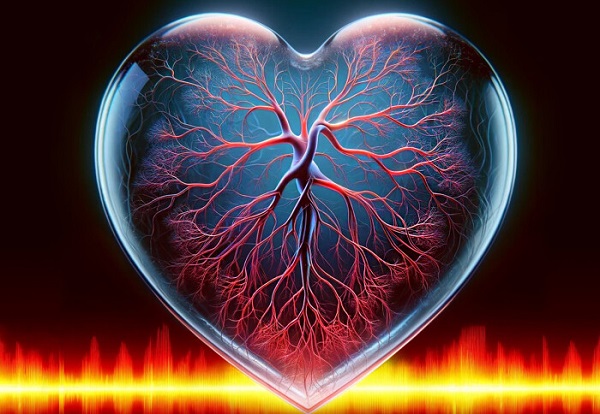
Super-Resolution Imaging Technique Could Improve Evaluation of Cardiac Conditions
The heart depends on efficient blood circulation to pump blood throughout the body, delivering oxygen to tissues and removing carbon dioxide and waste. Yet, when heart vessels are damaged, it can disrupt... Read more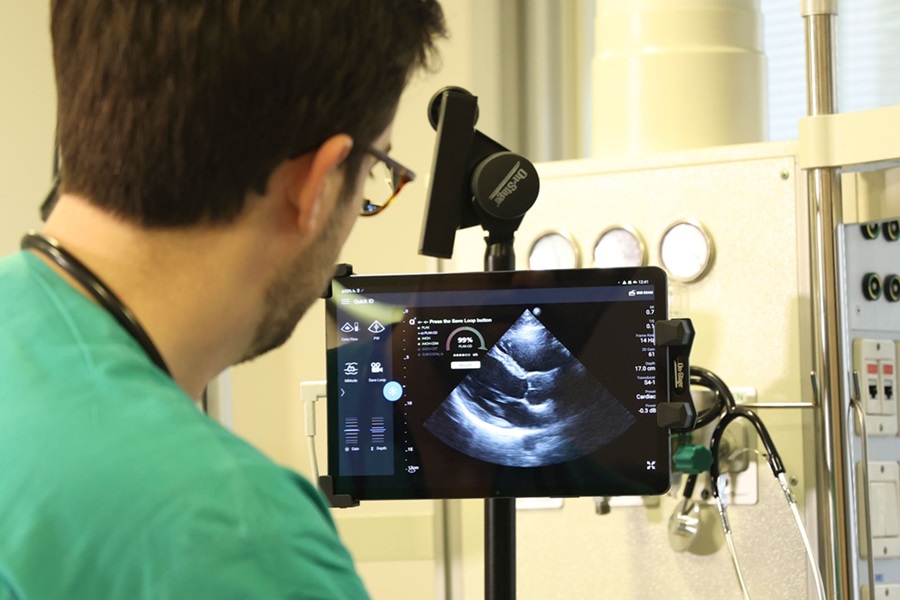
First AI-Powered POC Ultrasound Diagnostic Solution Helps Prioritize Cases Based On Severity
Ultrasound scans are essential for identifying and diagnosing various medical conditions, but often, patients must wait weeks or months for results due to a shortage of qualified medical professionals... Read moreNuclear Medicine
view channel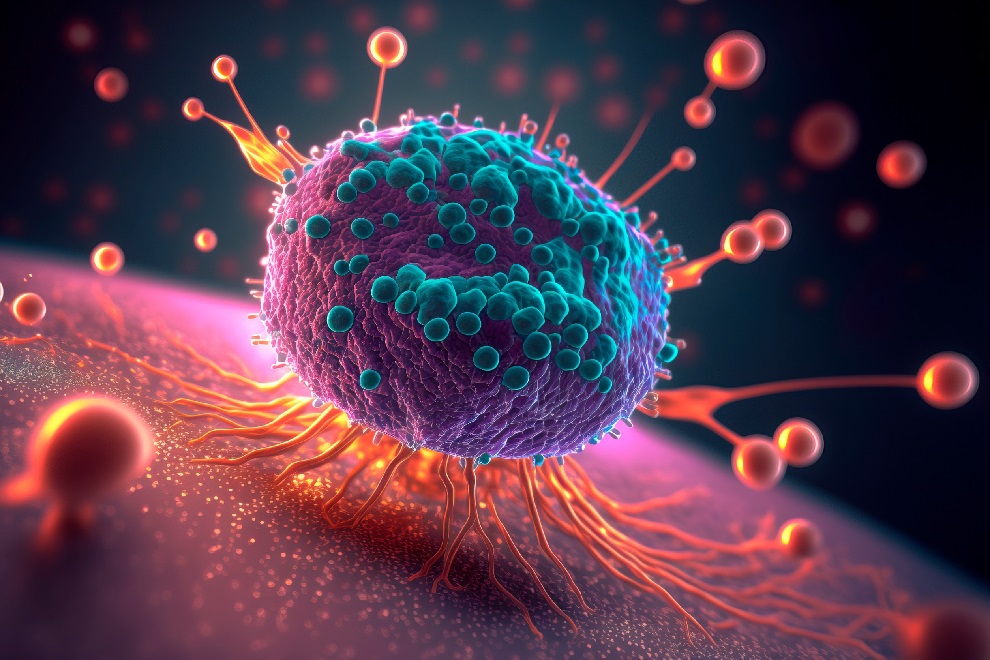
New PET Biomarker Predicts Success of Immune Checkpoint Blockade Therapy
Immunotherapies, such as immune checkpoint blockade (ICB), have shown promising clinical results in treating melanoma, non-small cell lung cancer, and other tumor types. However, the effectiveness of these... Read more![Image: Whole-body maximum-intensity projections over time after [68Ga]Ga-DPI-4452 administration (Photo courtesy of SNMMI) Image: Whole-body maximum-intensity projections over time after [68Ga]Ga-DPI-4452 administration (Photo courtesy of SNMMI)](https://globetechcdn.com/mobile_medicalimaging/images/stories/articles/article_images/2024-05-09/JNM May 2024 Hofman.jpg)
New PET Agent Rapidly and Accurately Visualizes Lesions in Clear Cell Renal Cell Carcinoma Patients
Clear cell renal cell cancer (ccRCC) represents 70-80% of renal cell carcinoma cases. While localized disease can be effectively treated with surgery and ablative therapies, one-third of patients either... Read more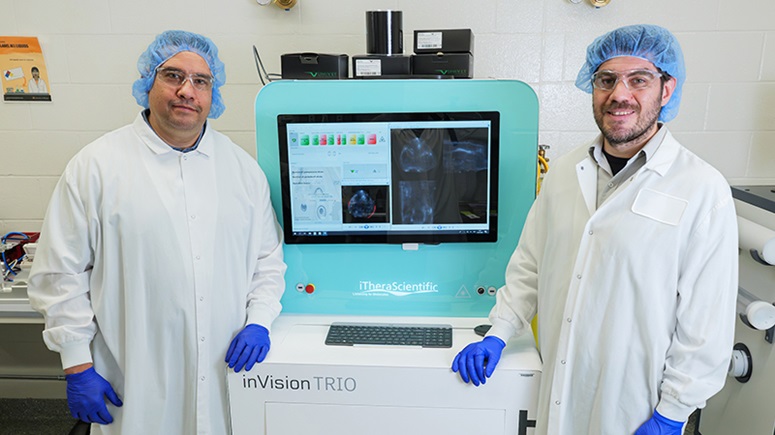
New Imaging Technique Monitors Inflammation Disorders without Radiation Exposure
Imaging inflammation using traditional radiological techniques presents significant challenges, including radiation exposure, poor image quality, high costs, and invasive procedures. Now, new contrast... Read more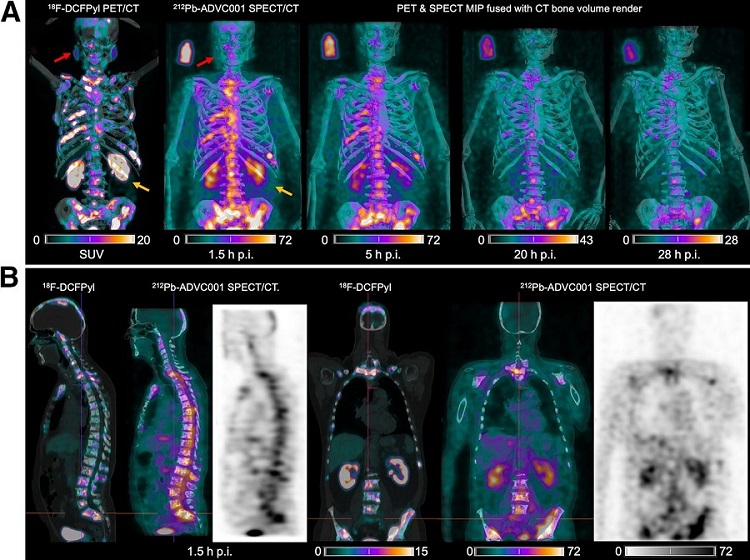
New SPECT/CT Technique Could Change Imaging Practices and Increase Patient Access
The development of lead-212 (212Pb)-PSMA–based targeted alpha therapy (TAT) is garnering significant interest in treating patients with metastatic castration-resistant prostate cancer. The imaging of 212Pb,... Read moreImaging IT
view channel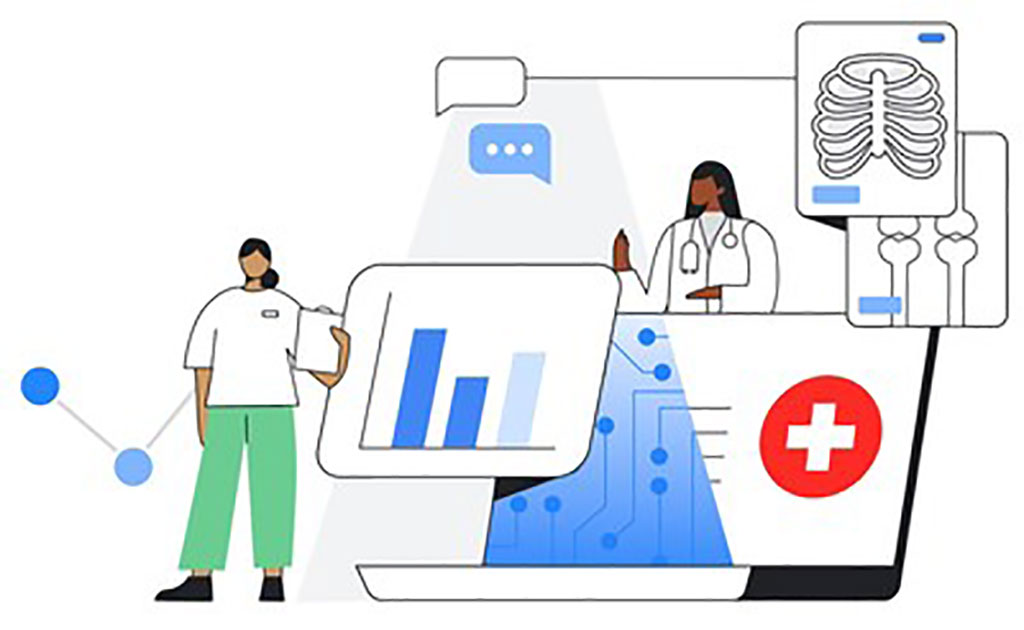
New Google Cloud Medical Imaging Suite Makes Imaging Healthcare Data More Accessible
Medical imaging is a critical tool used to diagnose patients, and there are billions of medical images scanned globally each year. Imaging data accounts for about 90% of all healthcare data1 and, until... Read more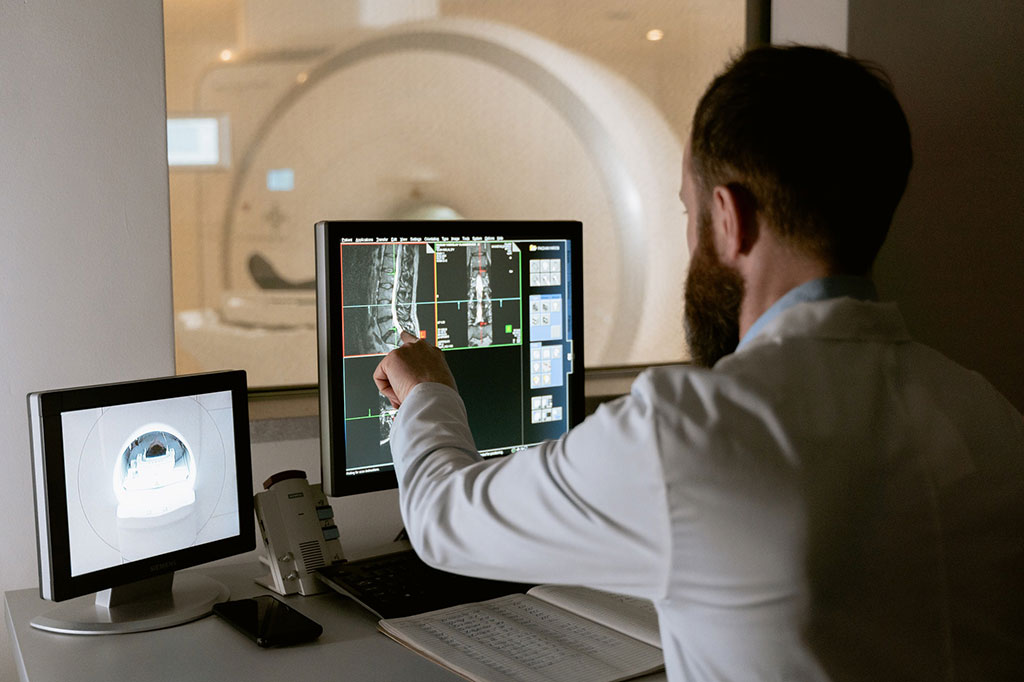
Global AI in Medical Diagnostics Market to Be Driven by Demand for Image Recognition in Radiology
The global artificial intelligence (AI) in medical diagnostics market is expanding with early disease detection being one of its key applications and image recognition becoming a compelling consumer proposition... Read moreIndustry News
view channel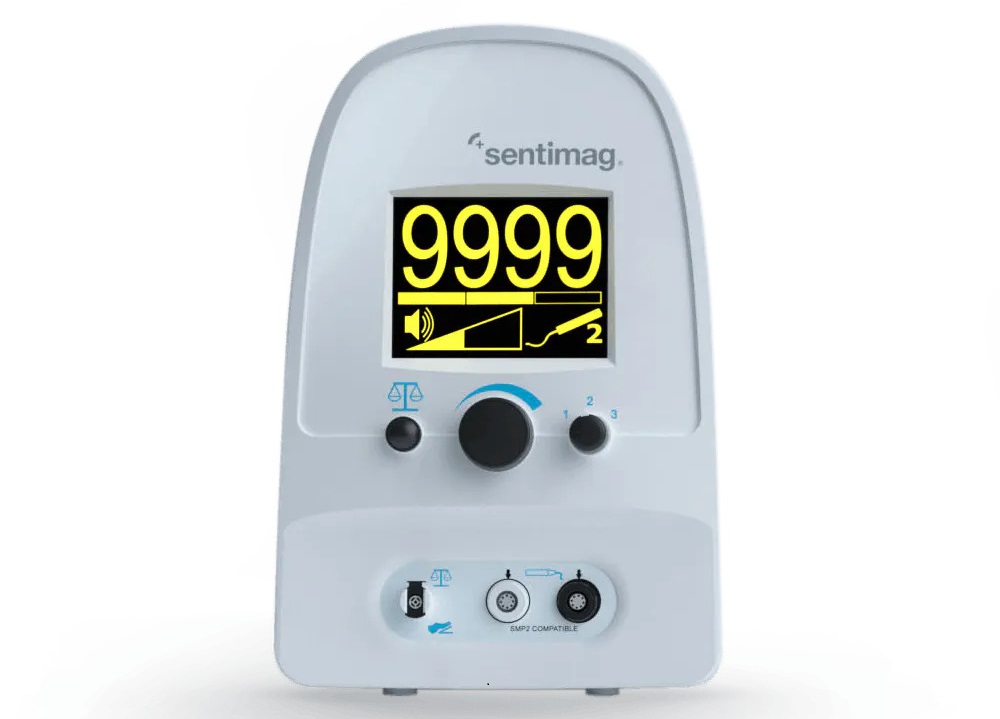
Hologic Acquires UK-Based Breast Surgical Guidance Company Endomagnetics Ltd.
Hologic, Inc. (Marlborough, MA, USA) has entered into a definitive agreement to acquire Endomagnetics Ltd. (Cambridge, UK), a privately held developer of breast cancer surgery technologies, for approximately... Read more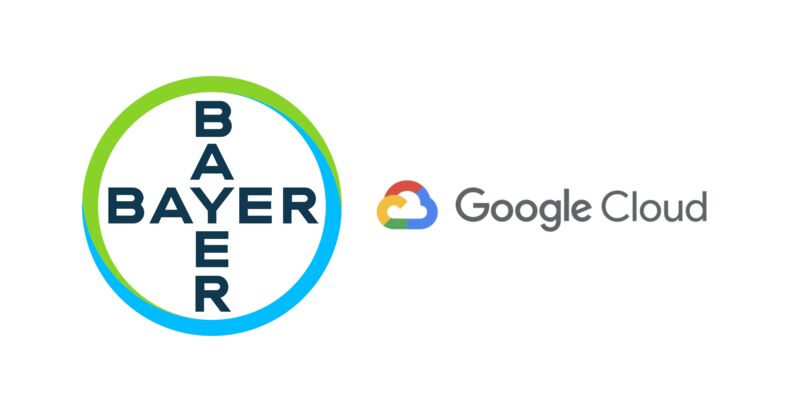
Bayer and Google Partner on New AI Product for Radiologists
Medical imaging data comprises around 90% of all healthcare data, and it is a highly complex and rich clinical data modality and serves as a vital tool for diagnosing patients. Each year, billions of medical... Read more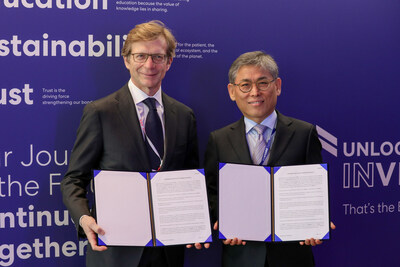