AI Outperforms Standard Risk Model for Predicting Breast Cancer
By MedImaging International staff writers Posted on 08 Jun 2023 |
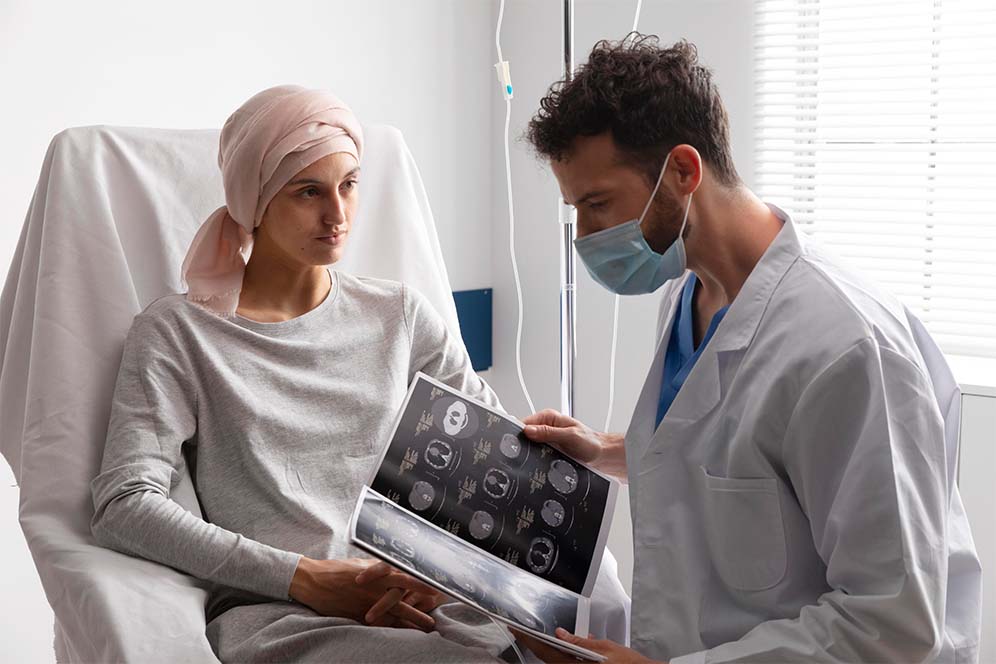
The risk of breast cancer in women is generally assessed using clinical models like the Breast Cancer Surveillance Consortium (BCSC) risk model. This model utilizes various patient data, including age, family history of breast cancer, childbirth history, and breast density, to produce a risk score. Now, a large study of thousands of mammograms has demonstrated that artificial intelligence (AI) algorithms can outperform this standard clinical risk model in predicting five-year breast cancer risk.
In the retrospective study, researchers at Kaiser Permanente Northern California (Oakland, CA, USA) used data from negative (indicating no visible signs of cancer) screening 2D mammograms carried out in 2016. From the 324,009 eligible women screened that year, a randomly selected subgroup of 13,628 women was examined. Additionally, all 4,584 patients who were diagnosed with cancer within five years of their 2016 mammogram were also included in the study. All women were monitored until 2021. The researchers split the five-year study duration into three separate time frames: interval cancer risk (diagnoses between 0 and 1 years), future cancer risk (diagnoses between 1 and 5 years), and all cancer risk (diagnoses between 0 and 5 years).
Five AI algorithms, including two used by researchers and three commercially available ones, were employed to generate breast cancer risk scores over the five-year period using the 2016 screening mammograms. These risk scores were then compared to each other and the BCSC clinical risk score. The study revealed that all five AI algorithms outperformed the BCSC risk model in predicting 0 to 5 year breast cancer risk. Some AI algorithms excelled in identifying high-risk patients for interval cancer, which can often be aggressive and may necessitate a second mammogram reading, additional screening, or follow-up imaging at short intervals. For instance, when assessing women with the top 10% risk, AI predicted up to 28% of cancers compared to the 21% predicted by the BCSC. Interestingly, even AI algorithms designed for shorter time horizons (as low as 3 months) could predict up to five years of future cancer risk when no cancer was clinically detected by the screening mammogram. When combined, the AI and BCSC risk models further enhanced cancer prediction.
"Clinical risk models depend on gathering information from different sources, which isn't always available or collected," said lead researcher Vignesh A. Arasu, M.D., Ph.D., a research scientist and practicing radiologist at Kaiser Permanente Northern California. "Recent advances in AI deep learning provide us with the ability to extract hundreds to thousands of additional mammographic features."
"This strong predictive performance over the five-year period suggests AI is identifying both missed cancers and breast tissue features that help predict future cancer development. Something in mammograms allows us to track breast cancer risk. This is the 'black box' of AI," added Arasu.
Related Links:
Kaiser Permanente
Latest General/Advanced Imaging News
- Radiation Therapy Computed Tomography Solution Boosts Imaging Accuracy
- PET Scans Reveal Hidden Inflammation in Multiple Sclerosis Patients
- Artificial Intelligence Evaluates Cardiovascular Risk from CT Scans
- New AI Method Captures Uncertainty in Medical Images
- CT Coronary Angiography Reduces Need for Invasive Tests to Diagnose Coronary Artery Disease
- Novel Blood Test Could Reduce Need for PET Imaging of Patients with Alzheimer’s
- CT-Based Deep Learning Algorithm Accurately Differentiates Benign From Malignant Vertebral Fractures
- Minimally Invasive Procedure Could Help Patients Avoid Thyroid Surgery
- Self-Driving Mobile C-Arm Reduces Imaging Time during Surgery
- AR Application Turns Medical Scans Into Holograms for Assistance in Surgical Planning
- Imaging Technology Provides Ground-Breaking New Approach for Diagnosing and Treating Bowel Cancer
- CT Coronary Calcium Scoring Predicts Heart Attacks and Strokes
- AI Model Detects 90% of Lymphatic Cancer Cases from PET and CT Images
- Breakthrough Technology Revolutionizes Breast Imaging
- State-Of-The-Art System Enhances Accuracy of Image-Guided Diagnostic and Interventional Procedures
- Catheter-Based Device with New Cardiovascular Imaging Approach Offers Unprecedented View of Dangerous Plaques
Channels
Radiography
view channel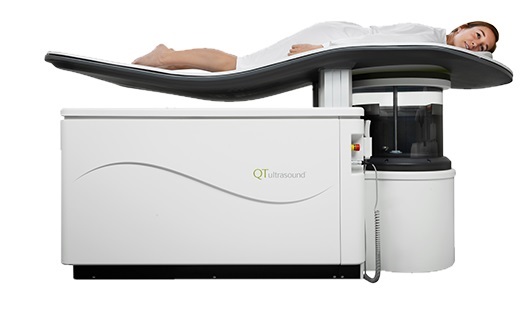
Novel Breast Imaging System Proves As Effective As Mammography
Breast cancer remains the most frequently diagnosed cancer among women. It is projected that one in eight women will be diagnosed with breast cancer during her lifetime, and one in 42 women who turn 50... Read more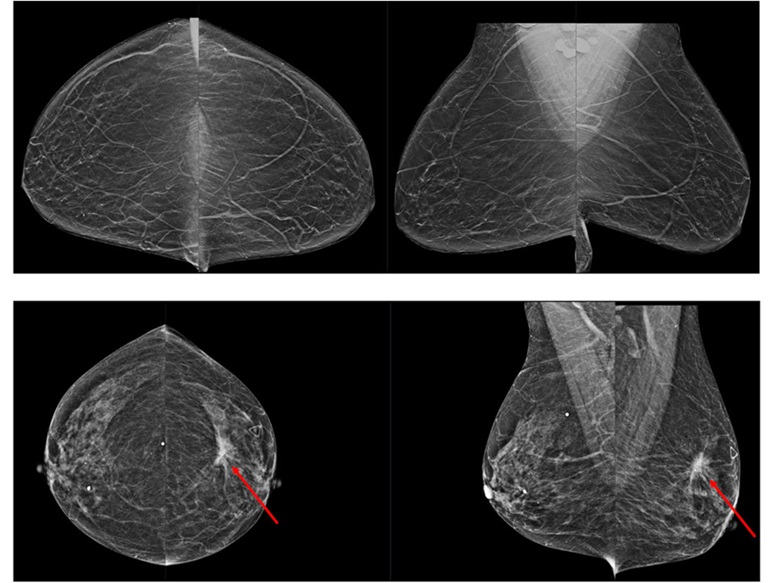
AI Assistance Improves Breast-Cancer Screening by Reducing False Positives
Radiologists typically detect one case of cancer for every 200 mammograms reviewed. However, these evaluations often result in false positives, leading to unnecessary patient recalls for additional testing,... Read moreMRI
view channel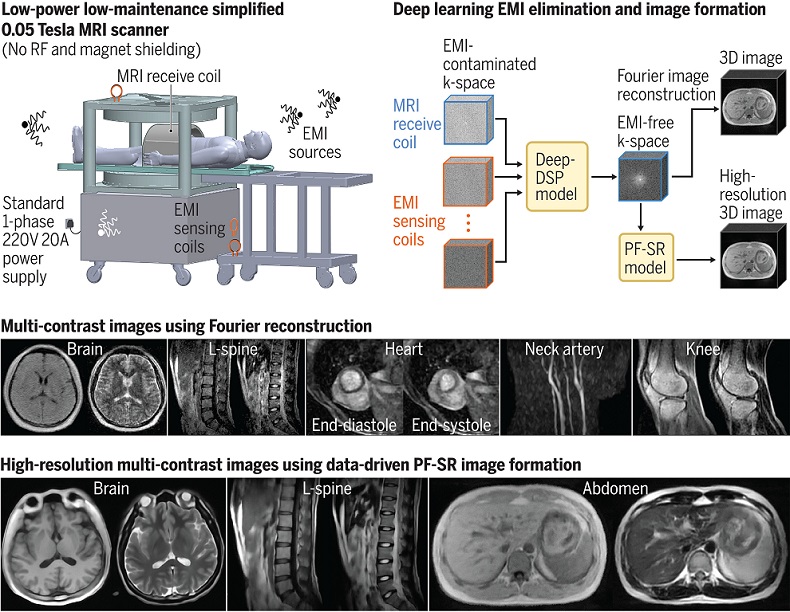
Low-Cost Whole-Body MRI Device Combined with AI Generates High-Quality Results
Magnetic Resonance Imaging (MRI) has significantly transformed healthcare, providing a noninvasive, radiation-free method for detailed imaging. It is especially promising for the future of medical diagnosis... Read more
World's First Whole-Body Ultra-High Field MRI Officially Comes To Market
The world's first whole-body ultra-high field (UHF) MRI has officially come to market, marking a remarkable advancement in diagnostic radiology. United Imaging (Shanghai, China) has secured clearance from the U.... Read moreUltrasound
view channel.jpg)
Diagnostic System Automatically Analyzes TTE Images to Identify Congenital Heart Disease
Congenital heart disease (CHD) is one of the most prevalent congenital anomalies worldwide, presenting substantial health and financial challenges for affected patients. Early detection and treatment of... Read more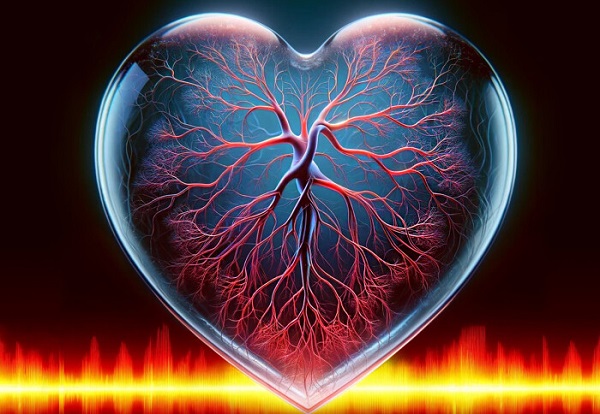
Super-Resolution Imaging Technique Could Improve Evaluation of Cardiac Conditions
The heart depends on efficient blood circulation to pump blood throughout the body, delivering oxygen to tissues and removing carbon dioxide and waste. Yet, when heart vessels are damaged, it can disrupt... Read more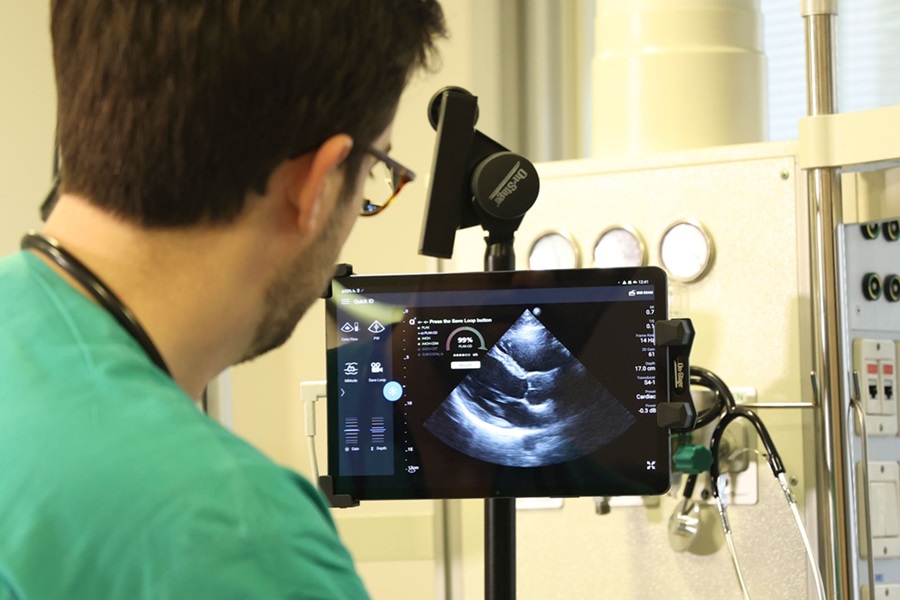
First AI-Powered POC Ultrasound Diagnostic Solution Helps Prioritize Cases Based On Severity
Ultrasound scans are essential for identifying and diagnosing various medical conditions, but often, patients must wait weeks or months for results due to a shortage of qualified medical professionals... Read moreNuclear Medicine
view channel![Image: Whole-body maximum-intensity projections over time after [68Ga]Ga-DPI-4452 administration (Photo courtesy of SNMMI) Image: Whole-body maximum-intensity projections over time after [68Ga]Ga-DPI-4452 administration (Photo courtesy of SNMMI)](https://globetechcdn.com/mobile_medicalimaging/images/stories/articles/article_images/2024-05-09/JNM May 2024 Hofman.jpg)
New PET Agent Rapidly and Accurately Visualizes Lesions in Clear Cell Renal Cell Carcinoma Patients
Clear cell renal cell cancer (ccRCC) represents 70-80% of renal cell carcinoma cases. While localized disease can be effectively treated with surgery and ablative therapies, one-third of patients either... Read more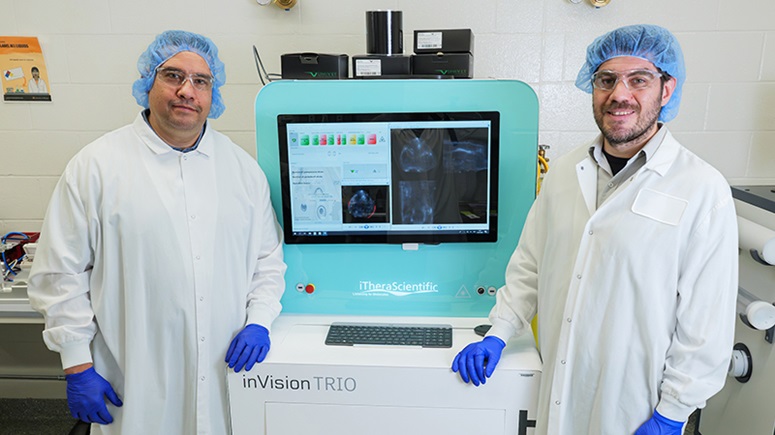
New Imaging Technique Monitors Inflammation Disorders without Radiation Exposure
Imaging inflammation using traditional radiological techniques presents significant challenges, including radiation exposure, poor image quality, high costs, and invasive procedures. Now, new contrast... Read more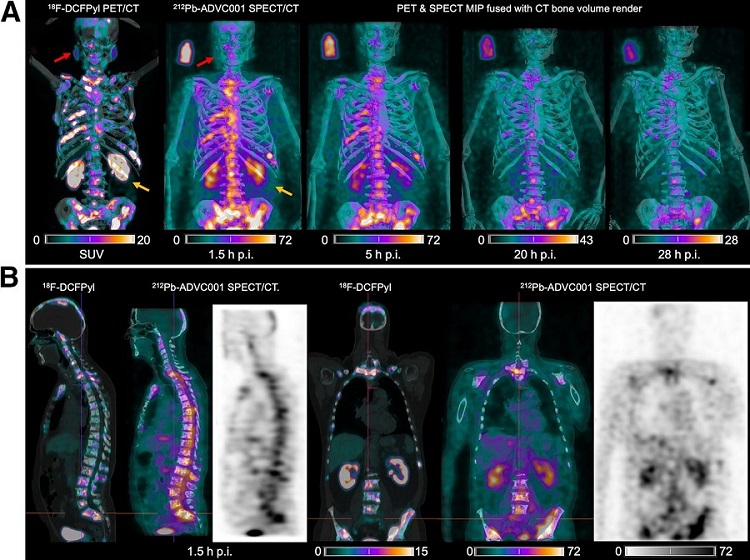
New SPECT/CT Technique Could Change Imaging Practices and Increase Patient Access
The development of lead-212 (212Pb)-PSMA–based targeted alpha therapy (TAT) is garnering significant interest in treating patients with metastatic castration-resistant prostate cancer. The imaging of 212Pb,... Read moreImaging IT
view channel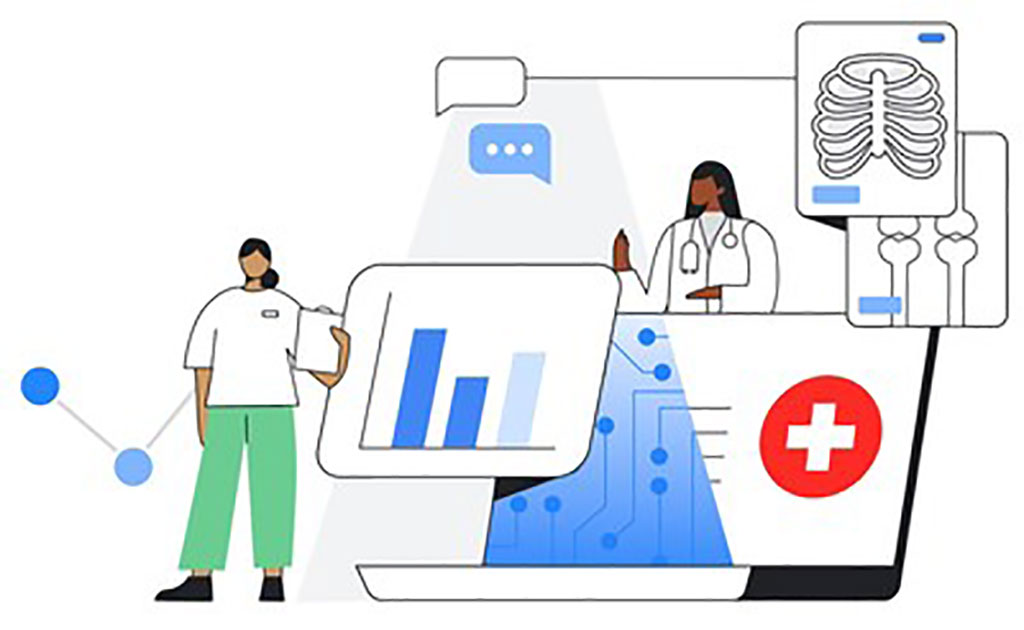
New Google Cloud Medical Imaging Suite Makes Imaging Healthcare Data More Accessible
Medical imaging is a critical tool used to diagnose patients, and there are billions of medical images scanned globally each year. Imaging data accounts for about 90% of all healthcare data1 and, until... Read more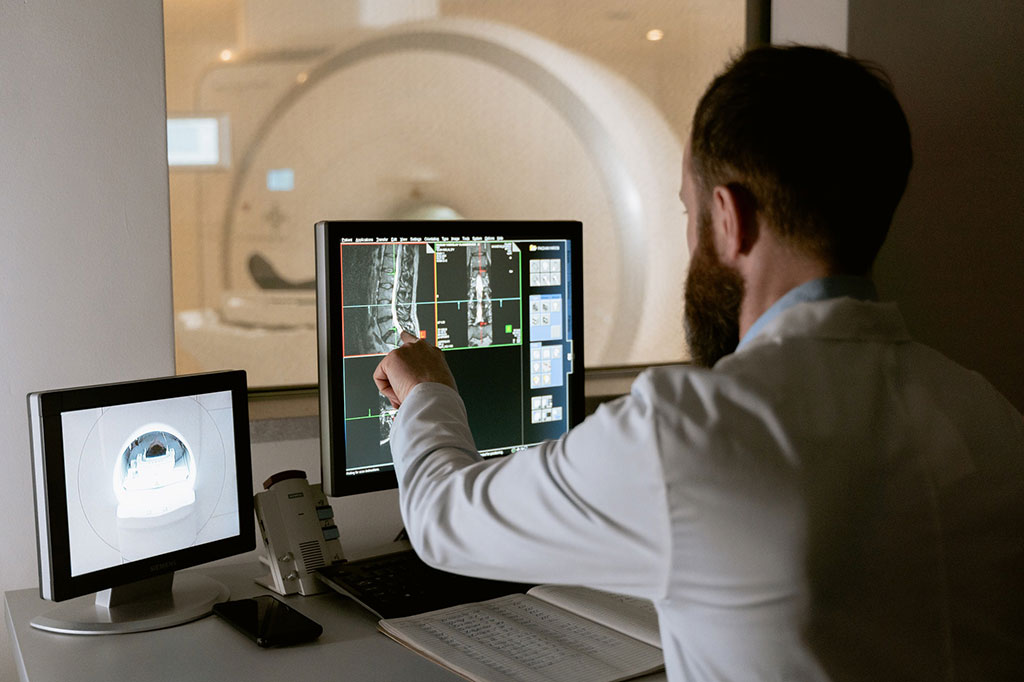
Global AI in Medical Diagnostics Market to Be Driven by Demand for Image Recognition in Radiology
The global artificial intelligence (AI) in medical diagnostics market is expanding with early disease detection being one of its key applications and image recognition becoming a compelling consumer proposition... Read moreIndustry News
view channel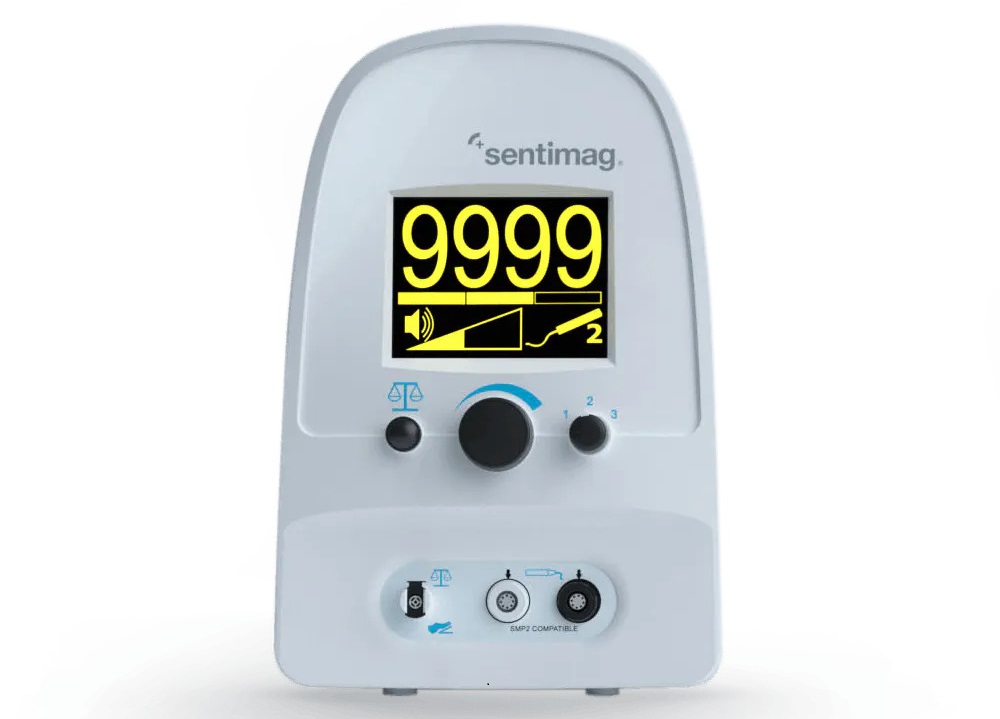
Hologic Acquires UK-Based Breast Surgical Guidance Company Endomagnetics Ltd.
Hologic, Inc. (Marlborough, MA, USA) has entered into a definitive agreement to acquire Endomagnetics Ltd. (Cambridge, UK), a privately held developer of breast cancer surgery technologies, for approximately... Read more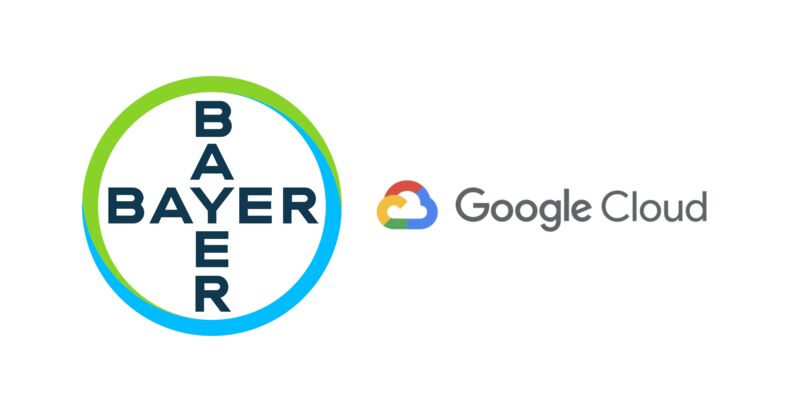
Bayer and Google Partner on New AI Product for Radiologists
Medical imaging data comprises around 90% of all healthcare data, and it is a highly complex and rich clinical data modality and serves as a vital tool for diagnosing patients. Each year, billions of medical... Read more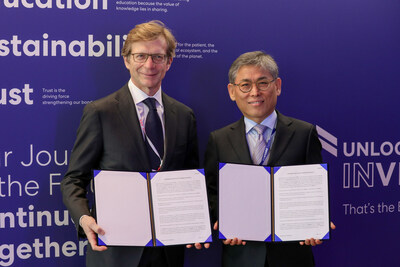