New Algorithm Allows Real-Time Reconstruction of Images Combining Optical and MRI Data to Improve Breast Cancer Detection
By MedImaging International staff writers Posted on 25 Feb 2022 |
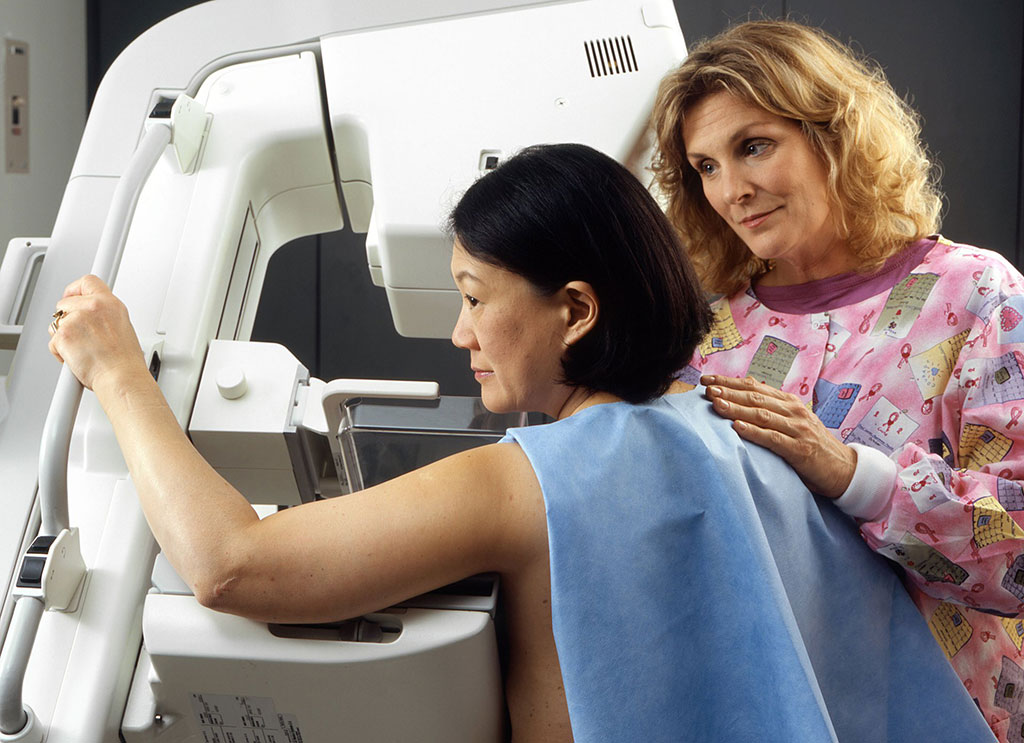
Researchers have developed a new image reconstruction approach that could contribute to better breast cancer detection.
The deep learning algorithm developed by a research team from Dartmouth College (Hanover, NH, USA) overcomes a major hurdle in multi-modality imaging by allowing images to be recovered in real time. The new algorithm, known as Z-Net, works with an imaging platform that combines optical spectral information with contrast-free magnetic resonance imaging (MRI) to improve detection of breast cancer. The new algorithm can distinguish between malignant and benign tumors using MRI-guided near infrared spectral tomography (NIRST) imaging data from patient breast exams.
Today, dynamic contrast-enhanced (DCE) MRI is recognized as the most sensitive breast cancer detection method. However, DCE MRI requires intravenous injection of a contrast agent and has a substantial false positive rate. Although non-contrast MRI-guided NIRST offers an alternative that doesn’t require contrast injection or ionizing radiation, reconstructing the combined images requires complicated light propagation models as well as time consuming MRI image analysis. The researchers used deep learning to make the image reconstruction process faster. Deep learning is a machine learning approach that creates connections among pieces of information in a way that is similar to how human brains operate, allowing the researchers to train their algorithm to recognize patterns and complex relationships.
After training the algorithm, the researchers used simulated data to confirm that the quality of the reconstructed images was not degraded by eliminating diffuse light propagation modeling or by not segmenting MRI images. They then applied the new algorithm prospectively to MRI-guided NIRST data collected from two breast imaging exams – one leading to a biopsy-confirmed cancer diagnosis, the other resulting in a benign abnormality. The new algorithm generated images that could tell the difference between the malignant and benign cases. The researchers are now working to adapt the new image reconstruction method to work with 3D data and plan to test it in a larger clinical trial in the near future.
“The near infrared spectral tomography (NIRST) and MRI imaging platform we developed has shown promise, but the time and effort involved in image reconstruction has prevented it from being translated into the day-to-day clinical workflow,” said Keith Paulsen, who led the research team. “Thus, we designed a deep-learning algorithm that incorporates anatomical image data from MRI to guide NIRST image formation without requiring complex modeling of light propagation in tissue.”
“Z-Net could allow NIRST to become an efficient and effective add-on to non-contrast MRI for breast cancer screening and diagnosis because it allows MRI-guided NIRST images to be recovered in nearly real time,” added Paulsen. “It can also be readily adapted for use with other cancers and diseases for which multi-modality imaging data are available.”
“The Z-Net algorithm reduces the time needed to generate a new image to a few seconds,” said Jinchao Feng, the study’s lead author. “Moreover, the machine learning network we developed can be trained with data generated by computer simulations rather than needing images from actual patient exams, which take a long time to collect and process into training information.”
Related Links:
Dartmouth College
Latest MRI News
- Low-Cost Whole-Body MRI Device Combined with AI Generates High-Quality Results
- World's First Whole-Body Ultra-High Field MRI Officially Comes To Market
- World's First Sensor Detects Errors in MRI Scans Using Laser Light and Gas
- Diamond Dust Could Offer New Contrast Agent Option for Future MRI Scans
- Combining MRI with PSA Testing Improves Clinical Outcomes for Prostate Cancer Patients
- PET/MRI Improves Diagnostic Accuracy for Prostate Cancer Patients
- Next Generation MR-Guided Focused Ultrasound Ushers In Future of Incisionless Neurosurgery
- Two-Part MRI Scan Detects Prostate Cancer More Quickly without Compromising Diagnostic Quality
- World’s Most Powerful MRI Machine Images Living Brain with Unrivaled Clarity
- New Whole-Body Imaging Technology Makes It Possible to View Inflammation on MRI Scan
- Combining Prostate MRI with Blood Test Can Avoid Unnecessary Prostate Biopsies
- New Treatment Combines MRI and Ultrasound to Control Prostate Cancer without Serious Side Effects
- MRI Improves Diagnosis and Treatment of Prostate Cancer
- Combined PET-MRI Scan Improves Treatment for Early Breast Cancer Patients
- 4D MRI Could Improve Clinical Assessment of Heart Blood Flow Abnormalities
- MRI-Guided Focused Ultrasound Therapy Shows Promise in Treating Prostate Cancer
Channels
Radiography
view channel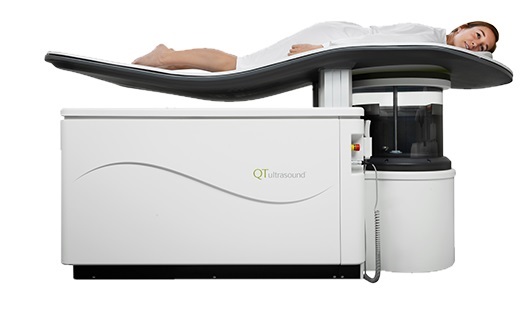
Novel Breast Imaging System Proves As Effective As Mammography
Breast cancer remains the most frequently diagnosed cancer among women. It is projected that one in eight women will be diagnosed with breast cancer during her lifetime, and one in 42 women who turn 50... Read more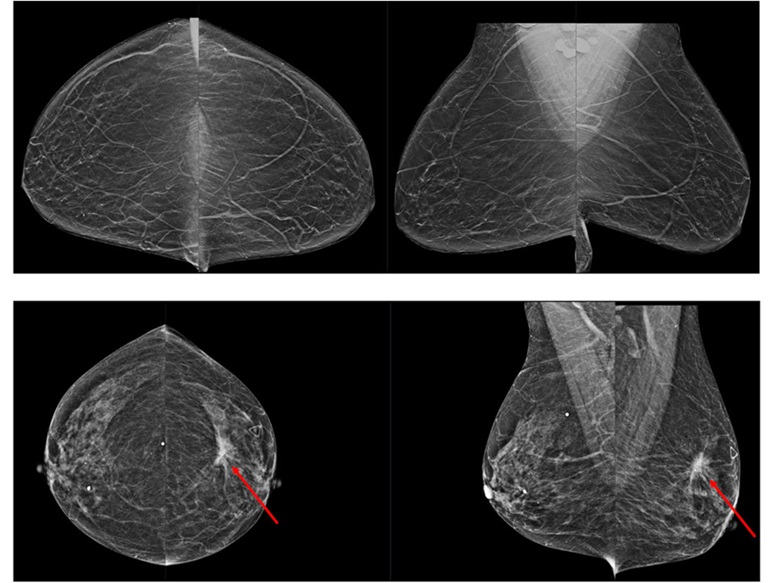
AI Assistance Improves Breast-Cancer Screening by Reducing False Positives
Radiologists typically detect one case of cancer for every 200 mammograms reviewed. However, these evaluations often result in false positives, leading to unnecessary patient recalls for additional testing,... Read moreUltrasound
view channel.jpg)
Diagnostic System Automatically Analyzes TTE Images to Identify Congenital Heart Disease
Congenital heart disease (CHD) is one of the most prevalent congenital anomalies worldwide, presenting substantial health and financial challenges for affected patients. Early detection and treatment of... Read more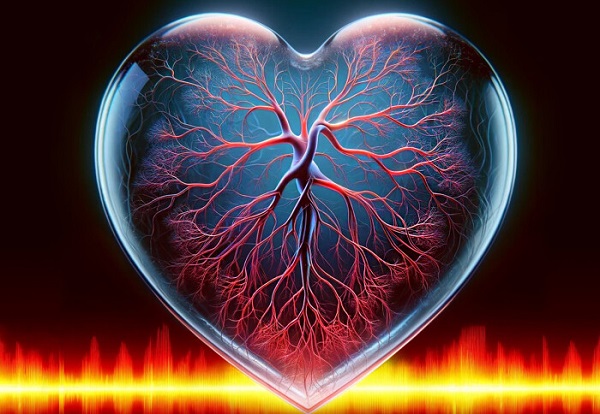
Super-Resolution Imaging Technique Could Improve Evaluation of Cardiac Conditions
The heart depends on efficient blood circulation to pump blood throughout the body, delivering oxygen to tissues and removing carbon dioxide and waste. Yet, when heart vessels are damaged, it can disrupt... Read more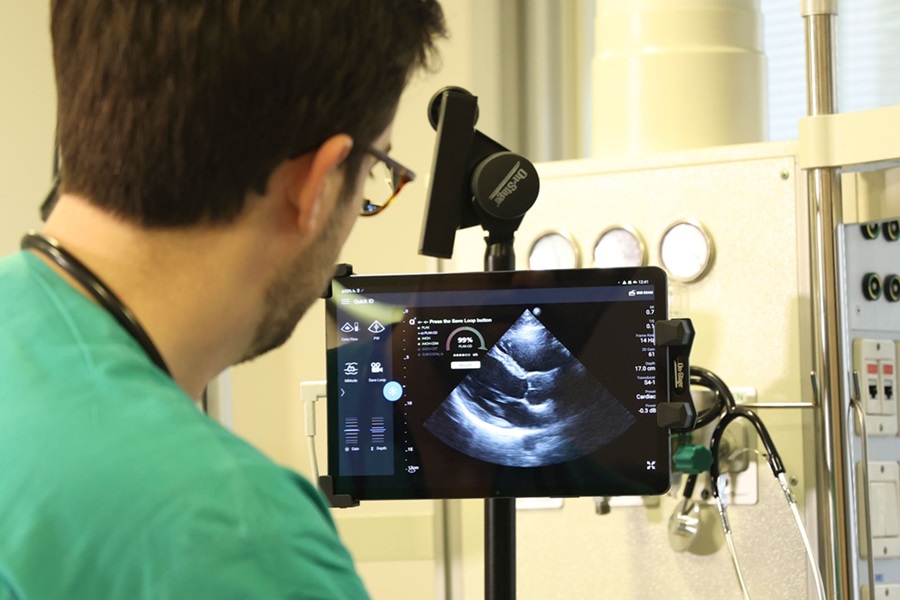
First AI-Powered POC Ultrasound Diagnostic Solution Helps Prioritize Cases Based On Severity
Ultrasound scans are essential for identifying and diagnosing various medical conditions, but often, patients must wait weeks or months for results due to a shortage of qualified medical professionals... Read moreNuclear Medicine
view channel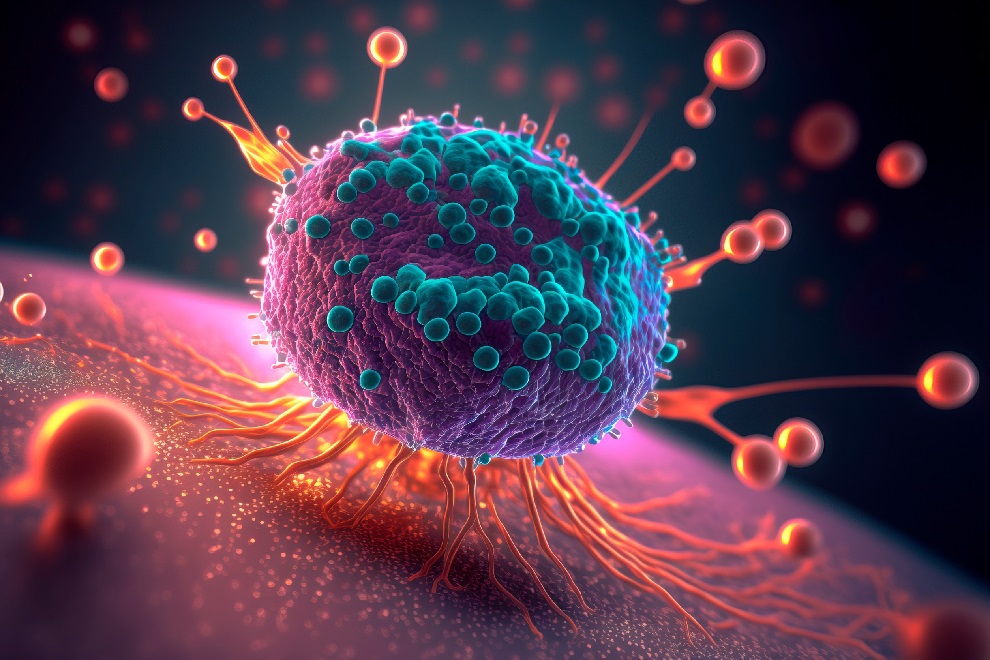
New PET Biomarker Predicts Success of Immune Checkpoint Blockade Therapy
Immunotherapies, such as immune checkpoint blockade (ICB), have shown promising clinical results in treating melanoma, non-small cell lung cancer, and other tumor types. However, the effectiveness of these... Read more![Image: Whole-body maximum-intensity projections over time after [68Ga]Ga-DPI-4452 administration (Photo courtesy of SNMMI) Image: Whole-body maximum-intensity projections over time after [68Ga]Ga-DPI-4452 administration (Photo courtesy of SNMMI)](https://globetechcdn.com/mobile_medicalimaging/images/stories/articles/article_images/2024-05-09/JNM May 2024 Hofman.jpg)
New PET Agent Rapidly and Accurately Visualizes Lesions in Clear Cell Renal Cell Carcinoma Patients
Clear cell renal cell cancer (ccRCC) represents 70-80% of renal cell carcinoma cases. While localized disease can be effectively treated with surgery and ablative therapies, one-third of patients either... Read more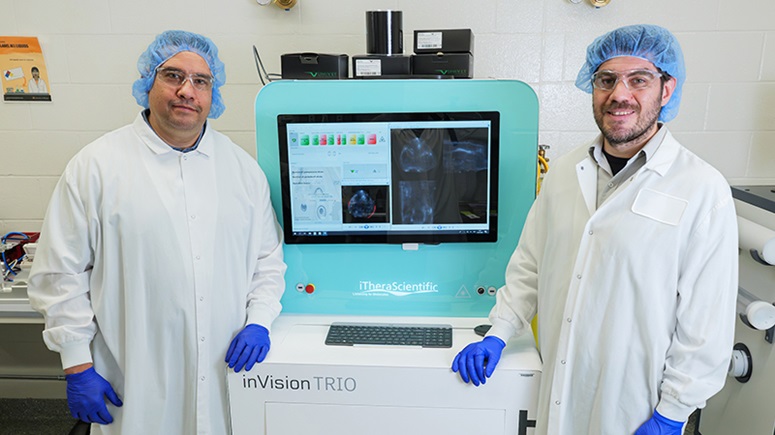
New Imaging Technique Monitors Inflammation Disorders without Radiation Exposure
Imaging inflammation using traditional radiological techniques presents significant challenges, including radiation exposure, poor image quality, high costs, and invasive procedures. Now, new contrast... Read more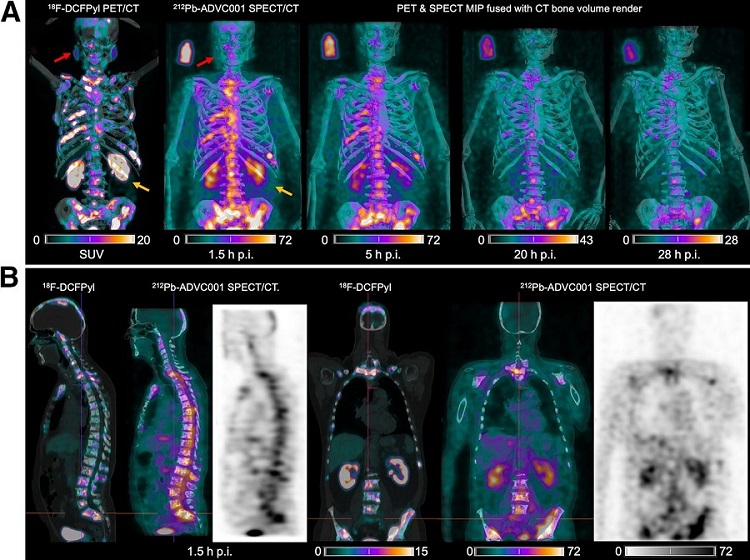
New SPECT/CT Technique Could Change Imaging Practices and Increase Patient Access
The development of lead-212 (212Pb)-PSMA–based targeted alpha therapy (TAT) is garnering significant interest in treating patients with metastatic castration-resistant prostate cancer. The imaging of 212Pb,... Read moreGeneral/Advanced Imaging
view channel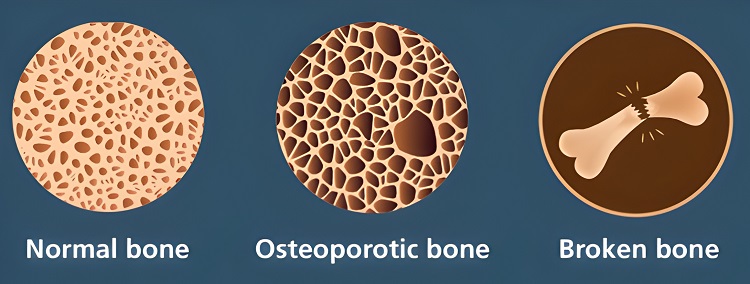
Bone Density Test Uses Existing CT Images to Predict Fractures
Osteoporotic fractures are not only devastating and deadly, especially hip fractures, but also impose significant costs. They rank among the top chronic diseases in terms of disability-adjusted life years... Read more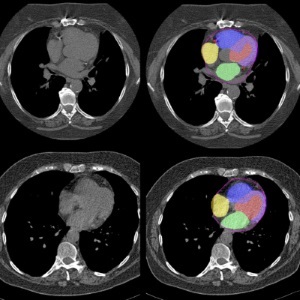
AI Predicts Cardiac Risk and Mortality from Routine Chest CT Scans
Heart disease remains the leading cause of death and is largely preventable, yet many individuals are unaware of their risk until it becomes severe. Early detection through screening can reveal heart issues,... Read moreImaging IT
view channel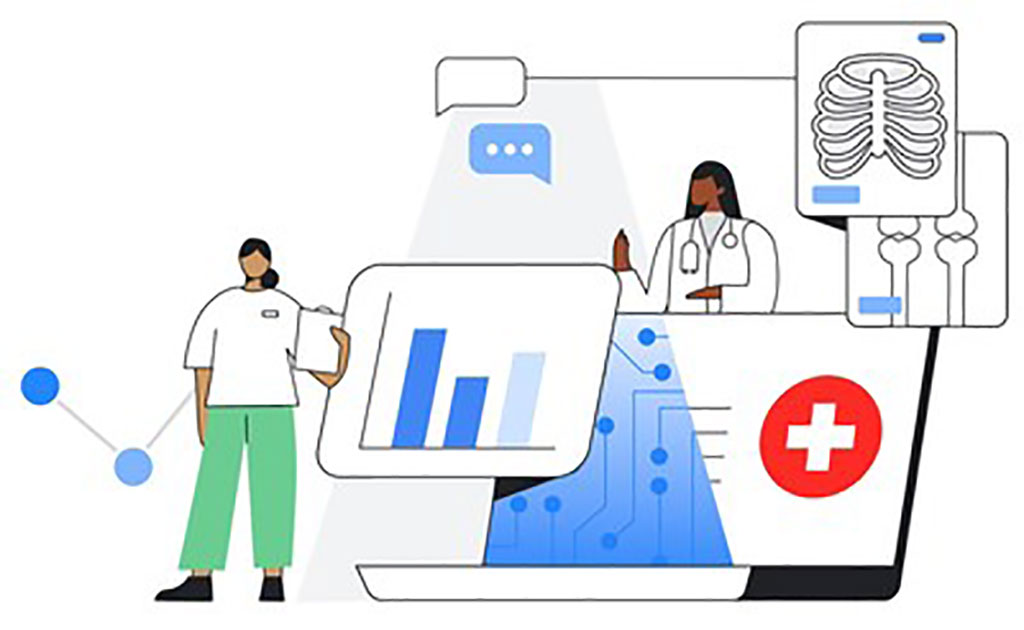
New Google Cloud Medical Imaging Suite Makes Imaging Healthcare Data More Accessible
Medical imaging is a critical tool used to diagnose patients, and there are billions of medical images scanned globally each year. Imaging data accounts for about 90% of all healthcare data1 and, until... Read more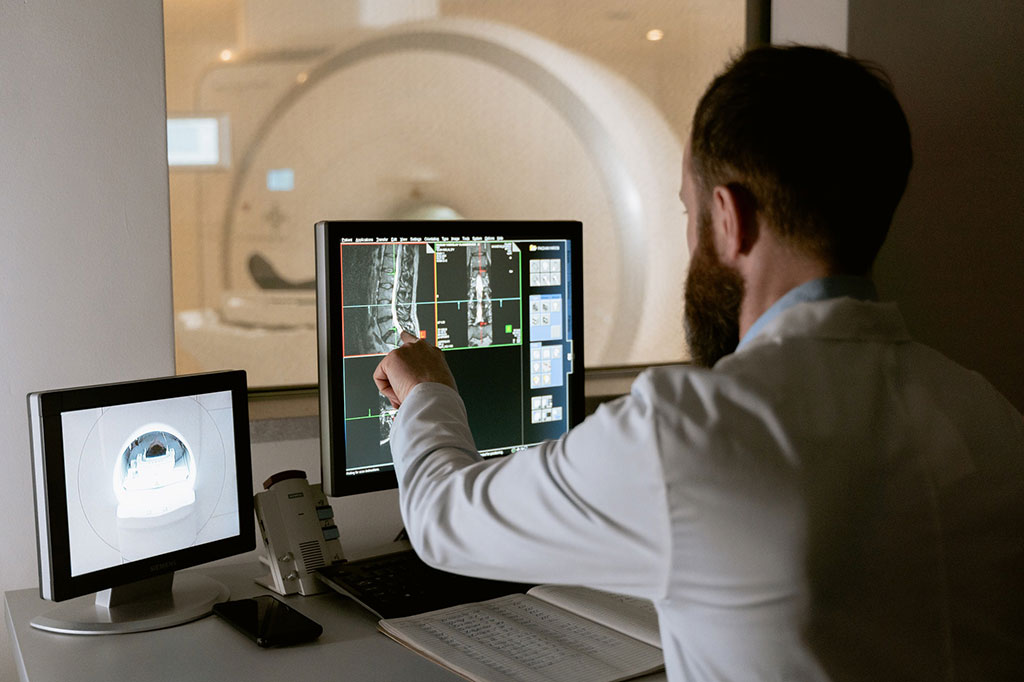
Global AI in Medical Diagnostics Market to Be Driven by Demand for Image Recognition in Radiology
The global artificial intelligence (AI) in medical diagnostics market is expanding with early disease detection being one of its key applications and image recognition becoming a compelling consumer proposition... Read moreIndustry News
view channel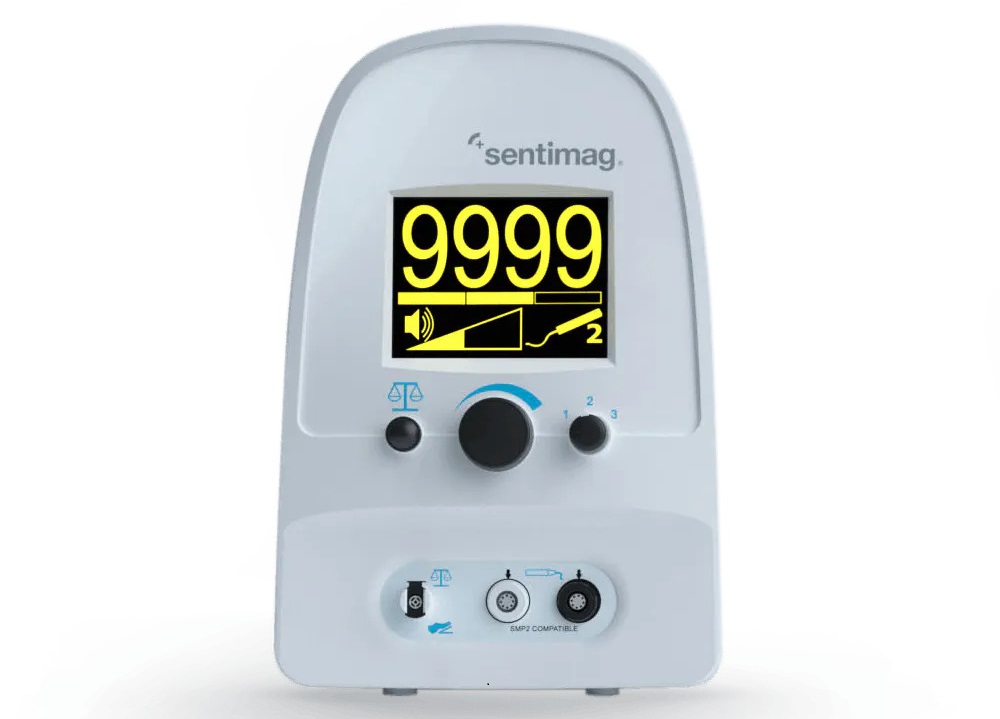
Hologic Acquires UK-Based Breast Surgical Guidance Company Endomagnetics Ltd.
Hologic, Inc. (Marlborough, MA, USA) has entered into a definitive agreement to acquire Endomagnetics Ltd. (Cambridge, UK), a privately held developer of breast cancer surgery technologies, for approximately... Read more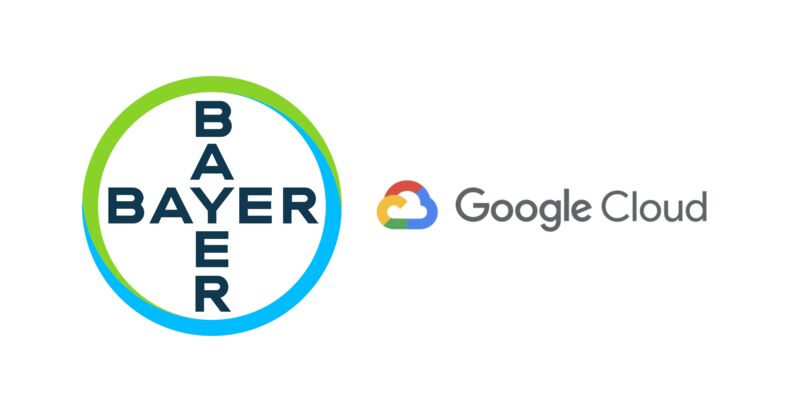
Bayer and Google Partner on New AI Product for Radiologists
Medical imaging data comprises around 90% of all healthcare data, and it is a highly complex and rich clinical data modality and serves as a vital tool for diagnosing patients. Each year, billions of medical... Read more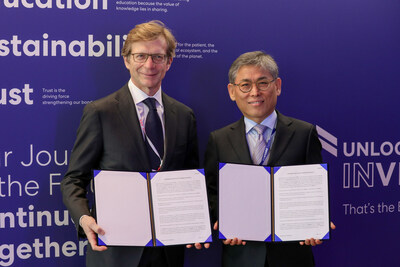