AI Outperforms Human Readers in Detecting Lung Nodules on X-Rays
Posted on 01 Feb 2024
Currently, over 150 artificial intelligence (AI)-based software products are available in the European market for radiology, with many addressing similar use cases. This makes it challenging for radiology departments to determine which software is most suitable for their needs. While software performance is a crucial factor in the procurement process, public data are scarce on the performance of these products. Clinical centers often lack the resources and personnel to thoroughly evaluate and compare multiple products before making a purchase. To address this issue, an initiative called Project AIR has been launched that aims to enhance market transparency for AI in radiology. Project AIR researchers have compiled a verified database of medical images for various clinical uses. This database allows for the comparative testing of multiple AI algorithms.
Now, in the first tests of the Project AIR concept, researchers discovered that out of seven AI algorithms trialed for detecting lung nodules in X-rays, four surpassed human readers in performance, while two algorithms for bone age prediction did not meet expectations. For testing the Project AIR concept, a team that included researchers from Radboud University (Nijmegen, the Netherlands) invited AI developers to participate. Between June 2022 and January 2023, nine products from eight vendors were validated: two for bone age prediction and seven for lung nodule assessment (one vendor participated in both categories). The team observed that the two algorithms for bone age analysis, Visiana, and Vuno, demonstrated excellent correlation with the reference standard, achieving r correlation coefficients of 0.987-0.989 (with 1 indicating perfect agreement). In lung nodule analysis, there was a more significant variation in performance, with human readers averaging an Area Under the Curve (AUC) of 0.81. The AI algorithms from Annalise.ai, Lunit, Milvue, and Oxipit showed superior performance, with AUCs of 0.90, 0.93, 0.86, and 0.88, respectively. The next tests of the Project AIR concept will focus on AI algorithms for fracture detection.
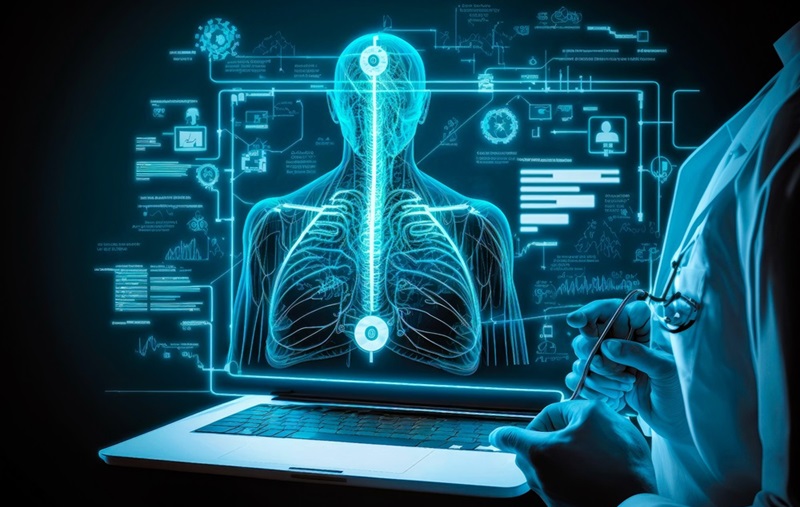
“We have shown the feasibility of the Project AIR methodology for external validation of commercial artificial intelligence (AI) products in medical imaging,” noted the researchers. “It is conceivable that in the future, radiology departments will require vendors to participate in transparent and comparative evaluations as a prerequisite for purchasing AI products.”
Related Links:
Radboud University