Radiologists Beat AI in Detecting Common Lung Diseases on Chest X-Rays
Posted on 27 Sep 2023
Chest X-rays are frequently used for diagnosis, but it takes a lot of training and expertise to read these images correctly. Although the Food and Drug Administration (FDA) has approved some artificial intelligence (AI) tools to help radiologists, the real-world effectiveness of these AI solutions in radiology is still relatively unexplored. Previous research has often focused on the AI's ability to detect a single disease, which is simpler than real-world situations where a patient might have multiple conditions. Now, a new study of over 2,000 chest X-rays has shown that human radiologists were generally more accurate than AI in identifying the presence and absence of three common lung diseases.
A team of researchers at Herlev and Gentofte Hospital (Copenhagen, Denmark) compared the performance of four commercially available AI tools against 72 radiologists. They evaluated 2,040 adult chest X-rays from four different hospitals, collected over two years in 2020. The patients' median age was 72, and about one-third of the X-rays had at least one key finding. The researchers focused on three common conditions: airspace disease (which could be due to pneumonia or lung edema), pneumothorax (collapsed lung), and pleural effusion (fluid around the lungs).
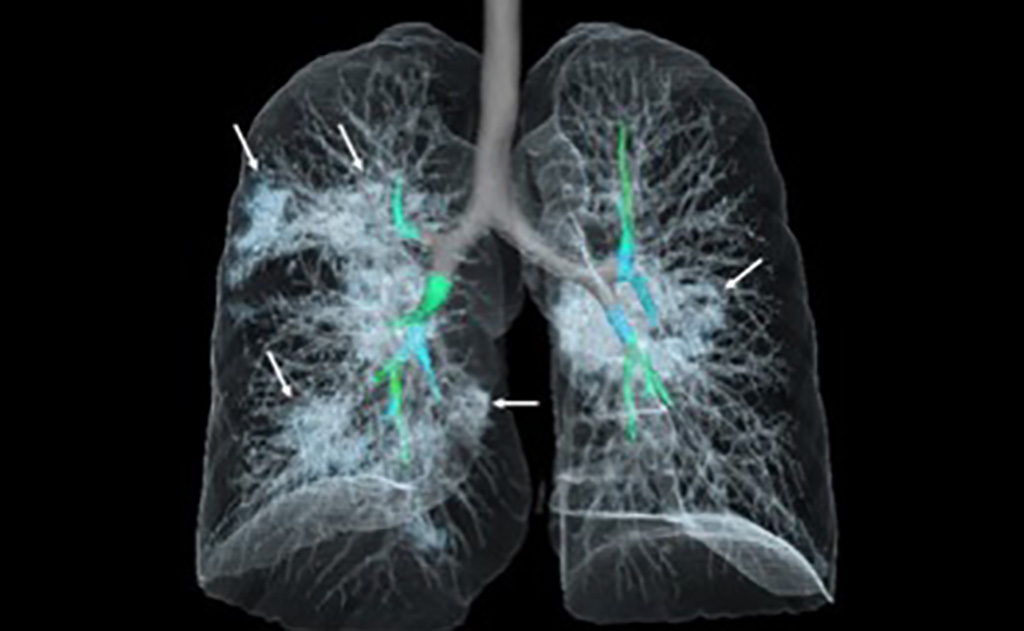
The AI tools showed sensitivity rates that varied: 72-91% for detecting airspace disease, 63-90% for pneumothorax, and 62-95% for pleural effusion. When it came to airspace disease, the AI's positive predictive values ranged from 40 to 50%. In this particular set of older and complex patients, the AI incorrectly flagged the presence of airspace disease in 50-60% of cases. While these AI tools had a moderate to high sensitivity comparable to human radiologists for detecting these conditions, they were more likely to produce false positives.
Moreover, their performance dipped when the X-rays showed multiple findings or smaller target areas. For instance, the probability of a correct pneumothorax diagnosis using AI ranged between 56% and 86%, while it was 96% for the radiologists. According to the research team, the ultimate goal for radiologists is to strike a balance between identifying diseases and avoiding false positives. These AI tools, although not perfect, could act as a secondary check for radiologists, potentially increasing their confidence in making diagnoses.
“AI systems seem very good at finding disease, but they aren’t as good as radiologists at identifying the absence of disease especially when the chest X-rays are complex. Too many false-positive diagnoses would result in unnecessary imaging, radiation exposure and increased costs,” said lead researcher Louis L. Plesner. “Our study demonstrates that radiologists generally outperform AI in real-life scenarios where there is a wide variety of patients. While an AI system is effective at identifying normal chest X-rays, AI should not be autonomous for making diagnoses.”
Related Links:
Herlev and Gentofte Hospital