High-Accuracy AI Model Can Improve Radiologists' Chest X-Ray Analysis Performance
Posted on 04 Jul 2023
A recent study presents compelling evidence that a high-accuracy AI model can significantly enhance radiologists' ability to analyze chest X-rays. The study reveals that only medical AI solutions with high diagnostic accuracy can markedly improve the diagnostic performance of radiologists.
Lunit (Seoul, Korea) has shared the results of a study investigating the impact of medical AI solutions' accuracy on radiologists' diagnostic decisions. The research involved a group of 30 physicians, consisting of 20 board-certified radiologists with 5 to 18 years of experience and 10 radiology residents with 2 to 3 years of training. The group assessed a total of 120 chest radiographs collected retrospectively, half from lung cancer patients and the rest showing no abnormalities. In the initial session, the readers were split into two groups and each group analyzed 120 chest X-rays without AI assistance. In the following session, they reexamined the images using either a high-accuracy or low-accuracy AI model.
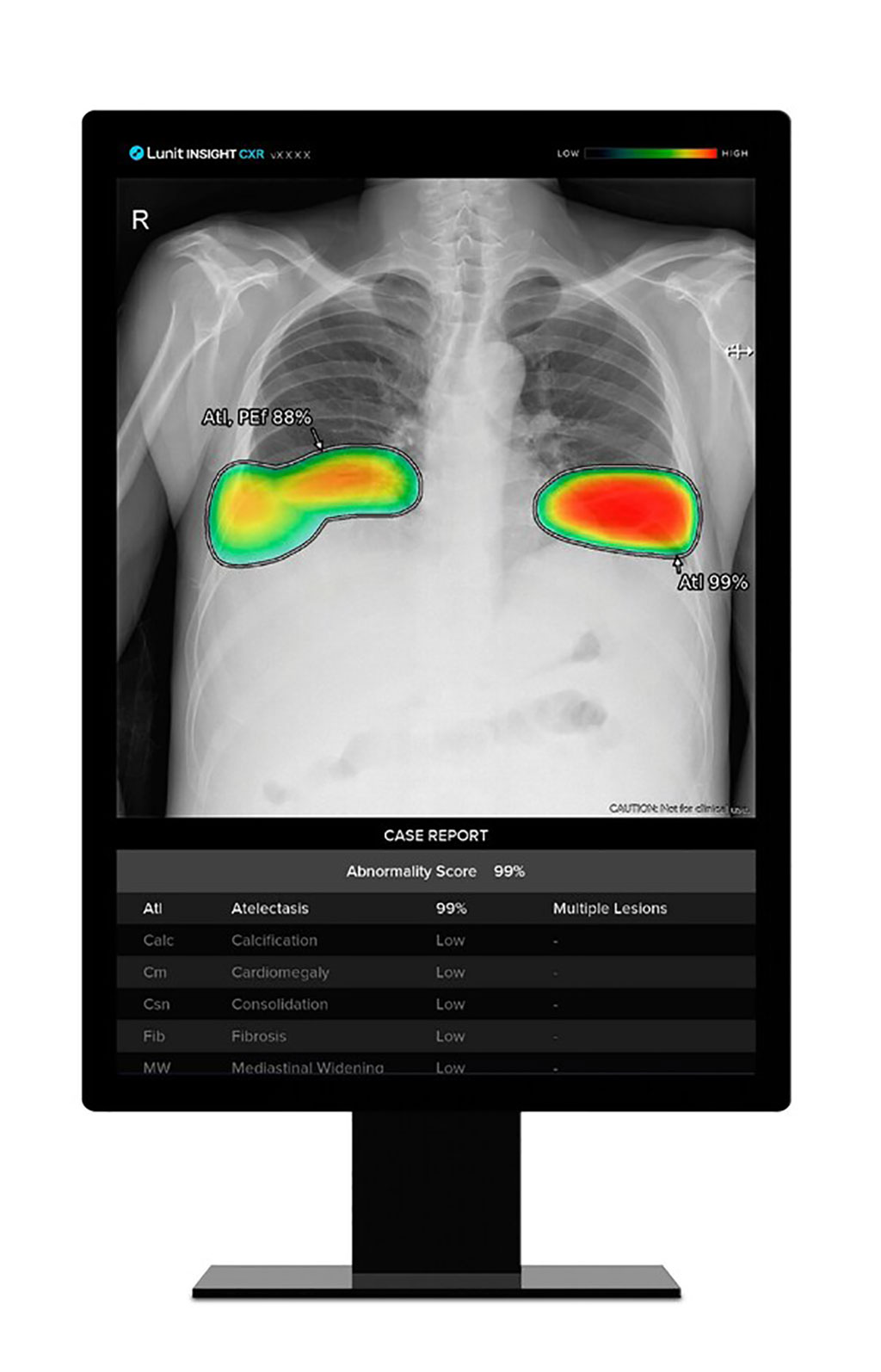
For the study, the researchers used Lunit INSIGHT CXR, a commercially available AI solution for chest X-ray analysis. The low-accuracy model, in contrast, was trained using only 10% of the data utilized for Lunit INSIGHT CXR. The AUROC (area under the receiver operating characteristic curve), a standard metric for diagnostic accuracy, for Lunit INSIGHT CXR was 0.88, while the low-accuracy AI model achieved only 0.77. The study findings showed that the use of the high-accuracy AI model, Lunit INSIGHT CXR, led to a significant enhancement in radiologists' performance. The AUROC improved remarkably from 0.77 to 0.82 when the high-accuracy AI model was used.
On the other hand, the group using the low-accuracy AI model did not witness any performance improvement, with the AUROC remaining at 0.75. Interestingly, the group utilizing the high-accuracy AI model exhibited a higher inclination to accept AI suggestions. They agreed with 67% of AI recommendations that conflicted with their initial interpretations, as opposed to a 59% acceptance rate from the group using the low-accuracy AI model. Moreover, the study concluded that individual factors such as the radiologists' expertise, their prior experience with AI, or attitudes toward AI had minimal effect on their diagnostic performance in the second session. Instead, the AI model's accuracy and the radiologists' initial diagnostic precision were identified as the primary influences on the final diagnostic outcomes. These results underscore the importance of the AI model's performance when used as a secondary reader by radiologists. They also show that such AI support can increase radiologists' receptiveness to AI suggestions, leading to more accurate diagnoses in the long run.
"The study backs that irrespective of radiologists' individual characteristics, the utilization of high-performance AI significantly enhances diagnostic accuracy and fosters a greater acceptance of AI within medical practices," said Brandon Suh, CEO of Lunit. "At Lunit, we are committed to developing AI-powered solutions that not only improve patient outcomes but also augment the expertise of healthcare professionals. This publication is a testament to our dedication to advancing the field of cancer diagnostics through cutting-edge technology."
Related Links:
Lunit