Artificial Intelligence Helps Radiologists Improve Chest X-Ray Interpretation, Finds New Study
By MedImaging International staff writers
Posted on 05 Jul 2021
A new diagnostic accuracy study has shown that radiologists can better interpret chest X-rays when assisted by a comprehensive deep-learning model that had a similar or better accuracy than the radiologists for most findings when compared with high-quality, gold standard assessment techniques.Posted on 05 Jul 2021
Chest X-rays are widely used in clinical practice; however, interpretation can be hindered by human error and a lack of experienced thoracic radiologists. Deep learning has the potential to improve the accuracy of chest X-ray interpretation. Therefore, the researchers aimed to assess the accuracy of radiologists with and without the assistance of a deep-learning model.
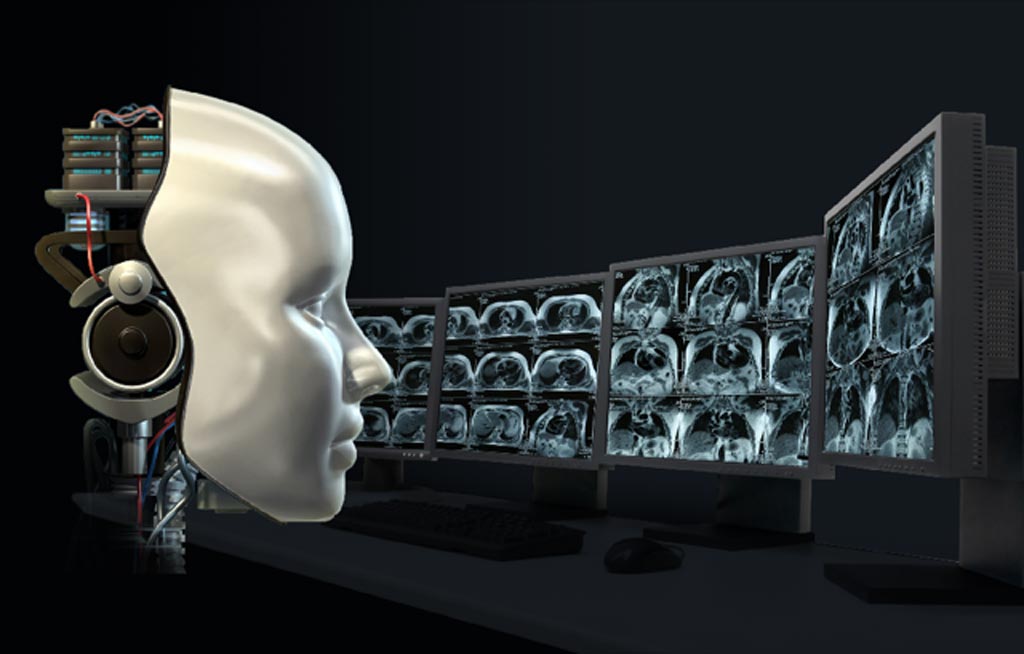
Illustration
In the retrospective study, a deep-learning model was trained on 821,681 images (284,649 patients) from five data sets from Australia, Europe, and the US. 2,568 enriched chest X-ray cases from adult patients who had at least one frontal chest X-ray were included in the test dataset; cases were representative of inpatient, outpatient, and emergency settings. 20 radiologists reviewed cases with and without the assistance of the deep-learning model with a three-month washout period. The researchers assessed the change in accuracy of chest X-ray interpretation across 127 clinical findings when the deep-learning model was used as a decision support by calculating area under the receiver operating characteristic curve (AUC) for each radiologist with and without the deep-learning model. The team also compared AUCs for the model alone with those of unassisted radiologists. If the lower bound of the adjusted 95% CI of the difference in AUC between the model and the unassisted radiologists was more than −0·05, the model was considered to be non-inferior for that finding. If the lower bound exceeded 0, the model was considered to be superior.
The researchers found that unassisted radiologists had a macroaveraged AUC of 0·713 (95% CI 0·645–0·785) across the 127 clinical findings, compared with 0·808 (0·763–0·839) when assisted by the model. The deep-learning model statistically significantly improved the classification accuracy of radiologists for 102 (80%) of 127 clinical findings, was statistically non-inferior for 19 (15%) findings, and no findings showed a decrease in accuracy when radiologists used the deep-learning model. Unassisted radiologists had a macroaveraged mean AUC of 0·713 (0·645–0·785) across all findings, compared with 0·957 (0·954–0·959) for the model alone. Model classification alone was significantly more accurate than unassisted radiologists for 117 (94%) of 124 clinical findings predicted by the model and was non-inferior to unassisted radiologists for all other clinical findings. Thus, the study demonstrated the potential of a comprehensive deep-learning model to improve chest X-ray interpretation across a large breadth of clinical practice.