Cardiac CT Algorithm Quantifies Aortic Valve Calcium
By MedImaging International staff writers
Posted on 22 Feb 2021
A new study shows that an artificial intelligence (AI) model can automatically detect aortic valve calcium (AVC) on cardiac CT, and is superior to visual grading by radiologists. Posted on 22 Feb 2021
Developed by researchers at the Catholic University of Korea (Seoul, South Korea), Yonsei University College of Medicine (Seoul, South Korea), and other institutions, the deep learning (DL)-based algorithm was initially trained and validated on 452 non-enhanced electrocardiogram-gated cardiac CT scans. It was then tested on a separate set of 137 cases, with each CT exam manually annotated by a radiologist with seven years of experience in cardiothoracic imaging, and AVC volume and Agatston scores were compared.
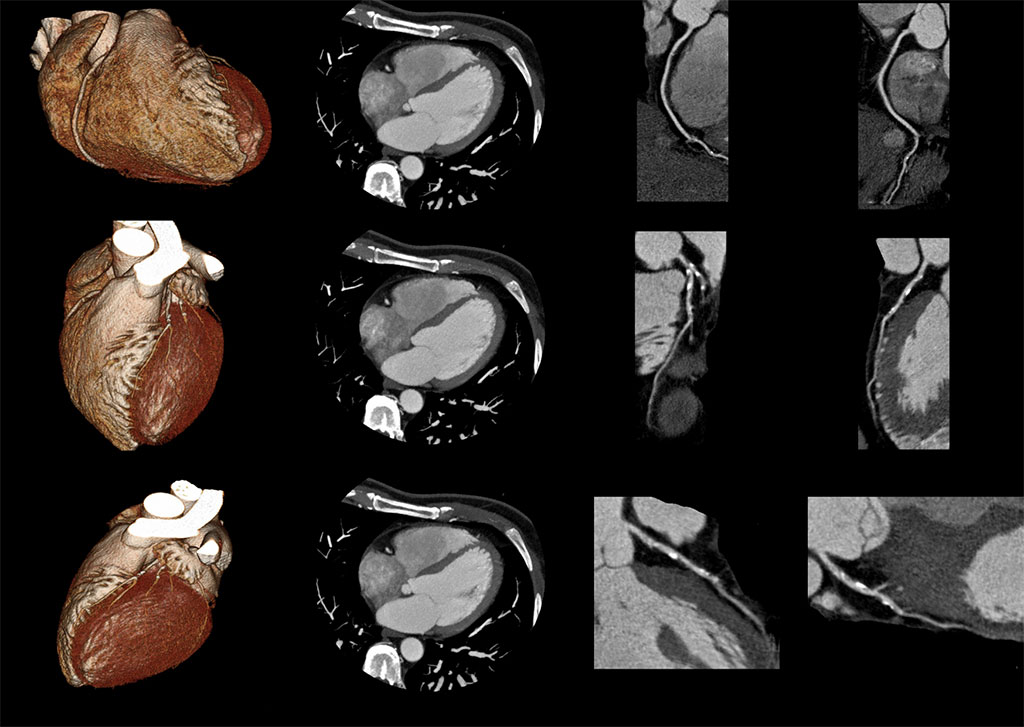
Image: Cardiac CT AI can detect calcium buildup on the aortic valve (Photo courtesy of Getty Images)
The results revealed that when manually measured AVC Agatston score was used as a benchmark, the accuracy of DL-measured AVC Agatston score for AVC volume grading was 97%, which was better than that of the four radiologist readers (77.8–89.9 %). The accuracy of DL algorithm for Agatston score was 92.9%. Overall, the DL model was deemed to be superior to all four radiologists for predicting severe aortic valve calcium cases. The study was published on February 6, 2021, in European Journal of Radiology.
“For observer performance testing, four radiologists determined AVC grade in two reading rounds. The diagnostic performance of DL-measured AVC volume and Agaston score for classifying severe AVC was compared with that of each reader's assessment,” explained lead author Suyon Chang, MD, of the Catholic University of Korea, and colleagues. “To validate AVC segmentation performance, the Dice coefficient [a statistic used to gauge the similarity of two samples] was calculated; after applying the DL algorithm, the Dice coefficient score was 0.807.”
The Agatston score is a semi-automated tool to calculate the extent of coronary artery calcification detected by an unenhanced low-dose CT scan, which is routinely performed in patients undergoing cardiac CT. It allows for early risk stratification as patients with a high Agatston score (over 160) have an increased risk for a major adverse cardiac event (MACE). Although it does not allow for the assessment of soft non-calcified plaques, it has shown a good correlation with contrast-enhanced CT coronary angiography.
Related Links:
Catholic University of Korea
Yonsei University College of Medicine