AI Algorithm Identifies Abnormal Chest X-Rays
By MedImaging International staff writers
Posted on 01 Oct 2018
A clinical validation study confirms that an artificial intelligence (AI) driven algorithm can differentiate between normal and abnormal x-rays with unprecedented accuracy.Posted on 01 Oct 2018
Researchers at Columbia Asia Hospitals (Kuala Lumpur, Malaysia) and Qure.ai (San Mateo, CA, USA) trained a deep learning system to identify abnormal x-rays using 1.2 million x-rays and their corresponding radiology reports. Specific x-ray abnormalities included blunted costophrenic angle, calcification, cardiomegaly, cavity, consolidation, fibrosis, hilar enlargement, opacity and pleural effusion, among others. The system was tested against a three-radiologist majority analysis based on an independent, retrospectively collected, and de-identified set of 2,000 x-rays.
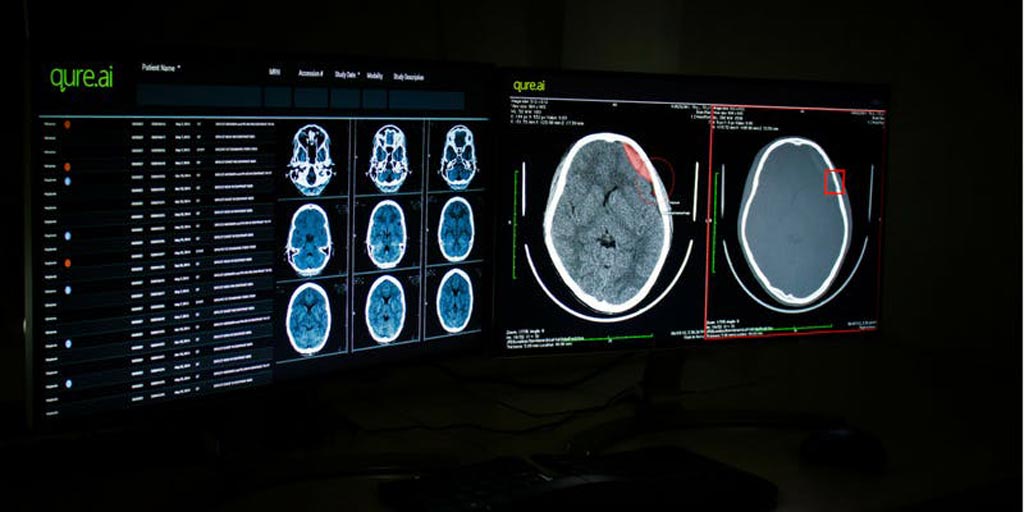
Image: AI algorithms match radiologists in detecting pathologies on chest X-rays and CT (Photo courtesy of Qure.ai).
The results showed that the deep learning AI system was highly accurate in detecting 15 abnormalities on chest x-rays at near-radiologist identification levels, with more than 90% accuracy. The chest clinical validation study joins a previous clinical study that confirmed the Qure.ai qER deep learning algorithm can identify bleeds, fractures, and other critical trauma in head computerized tomography (CT) scans, with more than 95% accuracy. The chest study was published on July 18, 2018, in arXiv.org.
“The chest x-ray is a valuable health screening tool and a vital component of public health programs worldwide. The enormous volume produced each year creates an ever-increasing demand for radiologists,” said study co-author Shalini Govil, MD, of the Columbia Asia Hospitals radiology group. “Unfortunately, numerous chest x-rays displaying significant pathology are left neglected in piles of backlogs due to a lack of available radiologists to report them. Through semi-automation of the reporting process, AI can significantly reduce a radiologist's workload, improve report accuracy, reduce turnaround time, and save lives.”
“This is the largest training dataset ever for a chest x-ray AI. It's also the largest validation study to date, measured against 2,000 x-rays, each read by three radiologists,” said senior author Prashant Warier, CEO and co-founder of Qure.ai. “This is an exciting time for deep learning technologies in medicine. As these systems increase in accuracy, so will the viability of using deep learning to extend the reach of chest x-ray interpretation, improve reporting efficiency, and save lives.”
Deep learning is part of a broader family of AI machine learning methods based on data representations, as opposed to task specific algorithms. It involves neural network algorithms that use a cascade of many layers of nonlinear processing units for feature extraction and transformation, with each successive layer using the output from the previous layer as input, forming a hierarchical representation.
Related Links:
Columbia Asia Hospitals
Qure.ai