AI Model for PET Imaging Determines Patient Response to Brain Tumor Treatments
Posted on 21 Aug 2023
The assessment of changes in metabolic tumor volume (MTV) through PET scans using specific radiotracers like F-18 fluoroethyl tyrosine (FET) plays a vital role in evaluating the treatment response in patients with brain tumors. Conventionally, MTV is determined through manual or semiautomatic processes, which are not only time-consuming but may also vary depending on the clinician performing the task. Now, a new AI model for PET imaging could greatly assist clinicians in evaluating how patients are responding to brain tumor treatments.
Researchers at Aachen University Hospital (Aachen, Germany) demonstrated that a deep learning-based AI model can automate the segmentation of MTV on brain PET images, potentially eliminating the need for human clinicians to preprocess these images. The goal of their study was to create a method for automated MTV segmentation and assess its effectiveness in gauging treatment responses in glioma patients. The research included a collection of 699 F-18 FET-PET scans from 555 brain tumor patients, either obtained at the time of initial diagnosis or during subsequent follow-up visits. Experienced nuclear-medicine physicians first segmented the MTV in these images, encompassing lesions with varying levels of F-18 FET radiotracer uptake.
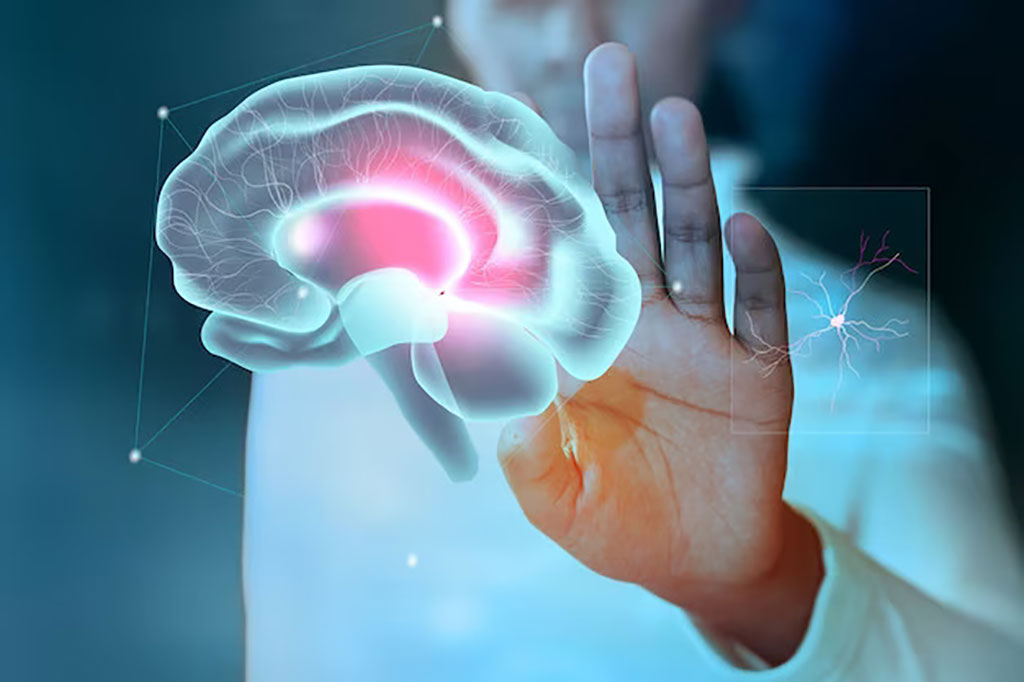
The team then utilized 476 of these images to train an AI neural network, named "no new U-Net," to perform MTV segmentation. To evaluate the model's accuracy, they applied it to an imaging dataset consisting of 223 scans from 156 different patients. Out of 205 lesions showing increased F-18 FET uptake in this dataset, the model correctly identified 189. Interestingly, the model did not mistakenly identify any anatomic regions with normal increased uptake of F-18 FET radiotracer, such as in the superior sagittal sinus, as tumors. The model's performance was quite remarkable, achieving a mean F1 score of 92%, a sensitivity of 93%, and a positive predictive value of 95% in detecting lesions. A key advantage of this model is its ability to fully automate 3D segmentation using just a single F-18 FET-PET scan. Moreover, it can perform this task in under two minutes using a standard graphics processing unit, without the need for any preprocessing.
"The main finding of our study is that our deep learning-based neural network allows reliable and fully automated detection and 3D segmentation of brain tumors investigated by F-18 FET PET," noted the researchers. "This finding highlights the value of the network for improvement and automatization of clinical decision-making based on the volumetric evaluation of amino acid PET."
Related Links:
Aachen University Hospital