AI-Driven Algorithm Uses fMRI Scans to Detect Autism and Predict Severity
Posted on 07 Apr 2022
Autism is one of the most common neurodevelopmental disorders but lacks objective biomarkers - telltale measurements that reveal a medical condition’s presence and sometimes severity -meaning there is no simple test for the disorder. Instead, diagnosis is based on observing patients’ behaviors, which are naturally highly variable and thus make diagnosis a challenge. Researchers have now developed an algorithm that may help discern if someone has autism by looking at brain scans.
The novel algorithm, developed by researchers at Stanford University (Stanford, CA, USA) and driven by recent advances in artificial intelligence (AI), also successfully predicts the severity of autism symptoms in individual patients. With further honing, the algorithm could lead to earlier diagnoses, more targeted therapies, and broadened understanding of autism’s origins in the brain.
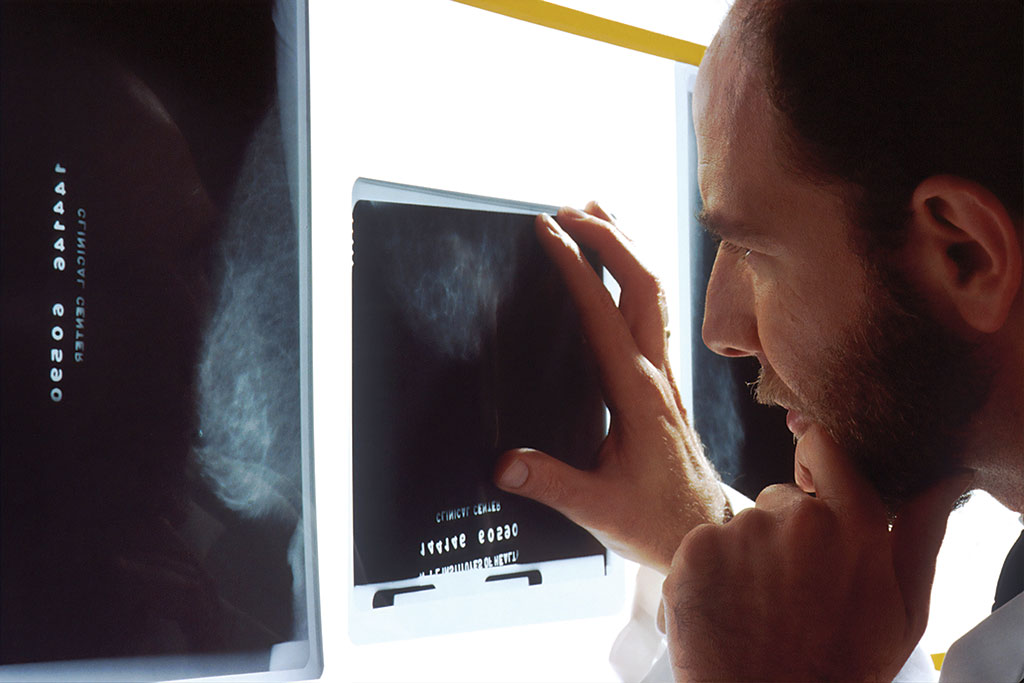
The algorithm pores over data gathered through functional magnetic resonance imaging (fMRI) scans. These scans capture patterns of neural activity throughout the brain. Scientists have long searched for biomarkers via fMRI scans. Yet studies to date with small populations have reported conflicting results, stemming from natural variability in patients’ brains and confounded further by differences in fMRI machines and testing methods. In deriving their image-recognition algorithms, the researchers sought to make the artificial intelligence explainable (or XAI), or understandable to human researchers.
By mapping this activity over time in the brain’s many regions, the algorithm generates neural activity “fingerprints.” Although unique for each individual just like real fingerprints, the brain fingerprints nevertheless share similar features, allowing them to be sorted and classified. In a new study, the algorithm assessed brain scans from a sample of approximately 1,100 patients. With 82% accuracy, the algorithm selected out a group of patients whom human clinicians had diagnosed with autism. The XAI algorithm alit upon three brain regions exhibiting significant differences in interconnectivity in a groupable portion of the dataset. Lending credibility to the XAI algorithm’s findings, those three brain regions have been previously implicated in autism pathology.
While the XAI algorithm performed admirably at this early stage of development, the researchers will need to improve its accuracy further still to raise brain fingerprinting to the level of a definitive biomarker. The researchers intend to explore the algorithm's efficacy in sibling studies, where one sibling has autism and the other does not, to hone the ability to detect fine-tuned, yet critical differences between potentially very similar brains. The researchers envision brain fingerprinting being used to assess the brains of very young children, perhaps as early as six months or a year old, who are at high risk of developing autism. Earlier diagnosis is critical in achieving better outcomes, with therapies proving more effective when introduced while patients are still toddler-aged versus later in childhood
“Although autism is one of the most common neurodevelopmental disorders, there is so much about it that we still don’t understand,” said lead author Kaustubh Supekar, a Stanford clinical assistant professor of psychiatry and behavioral sciences and Stanford HAI affiliate faculty. “In this study, we’ve shown that our AI-driven brain ‘fingerprinting’ model could potentially be a powerful new tool in advancing diagnosis and treatment.”
“We need to create objective biomarkers for autism,” added Supekar, “and brain fingerprints get us one step closer. We hope that the approach demonstrated in our study could diagnose autism during the window of opportunity when interventions are maximally most effective.”
Related Links:
Stanford University