AI-Based Models Outperform Human Experts at Identifying Ovarian Cancer in Ultrasound Images
Posted on 07 Jan 2025
Ovarian tumors are commonly found, often by chance. In many regions, there is a significant shortage of ultrasound specialists, which has raised concerns about unnecessary medical interventions and delayed cancer diagnoses. A new study, published in Nature Medicine, reveals that artificial intelligence (AI)-based models can outperform human experts in identifying ovarian cancer from ultrasound images.
In the international study led by Karolinska Institute (Solna, Sweden), researchers developed and validated neural network models that can distinguish between benign and malignant ovarian lesions. The AI was trained and tested on over 17,000 ultrasound images from 3,652 patients across 20 hospitals in eight countries. The researchers then compared the diagnostic performance of these AI models with a large group of both expert and less experienced ultrasound examiners. The results showed that the AI models achieved an accuracy rate of 86.3%, surpassing the experts' 82.6% and non-experts' 77.7%.
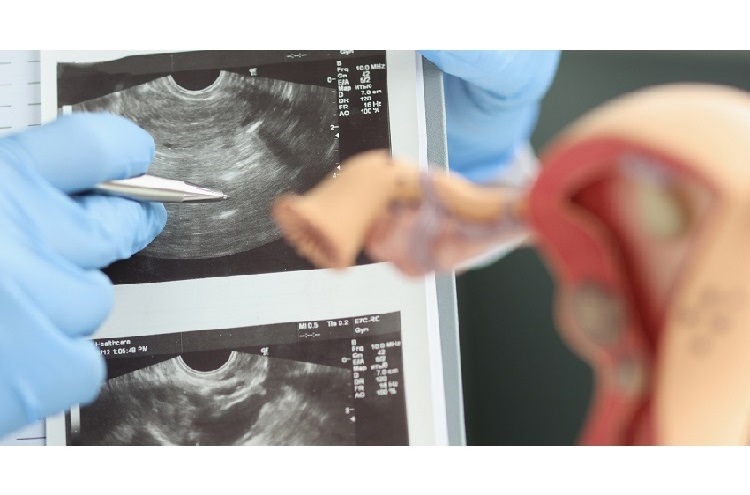
The AI models could also reduce the need for expert referrals. In a simulated triage scenario, the AI reduced the number of referrals by 63% and the misdiagnosis rate by 18%. This could lead to quicker and more cost-effective care for patients with ovarian lesions. Despite these promising results, the researchers emphasize the need for further studies to fully understand the clinical limitations and potential of the neural network models. They are currently conducting prospective clinical trials to assess the safety and effectiveness of the AI tool in daily clinical practice. Future research will also include a randomized multicenter study to evaluate its impact on patient management and healthcare costs.
“With continued research and development, AI-based tools can be an integral part of tomorrow’s healthcare, relieving experts and optimizing hospital resources, but we need to make sure that they can be adapted to different clinical environments and patient groups,” said Karolinska Institute’s Filip Christiansen who is the joint first author.