Non-Invasive Ultrasound Imaging Device Diagnoses Risk of Chronic Kidney Disease
Posted on 27 Mar 2024
Managing chronic kidney disease (CKD) efficiently is imperative for public health, as it represents a progressive condition affecting 10% of the worldwide population. The path from CKD to end-stage renal disease (ESRD) often involves renal fibrosis, making early detection and continuous monitoring critical for effective treatment and prognosis. Correctly identifying patients at elevated risk for advancing renal fibrosis remains a complex task in clinical settings. Researchers have now created a computer-based diagnostic tool that combines ultrasound (US) imagery with specific clinical indicators to evaluate the risk of moderate-to-severe renal fibrosis progression in individuals with CKD.
By leveraging advanced health technology, this innovative diagnostic tool Smart-CKD (S-CKD) developed by researchers from the Hong Kong Polytechnic University (PolyU, Hong Kong) can improve CKD management and patient monitoring. S-CKD integrates three essential clinical parameters: the patient's age, the ultrasonic renal length, and the end-diastolic velocity in the interlobar renal artery, all obtainable through regular medical follow-ups. Utilizing machine learning, S-CKD achieves a diagnostic accuracy of 80%. Available as an online web-based tool and an offline document, S-CKD offers a convenient, real-time, non-invasive method for clinicians to assess renal fibrosis risk, thus guiding therapeutic decisions, patient counseling, and follow-up scheduling.
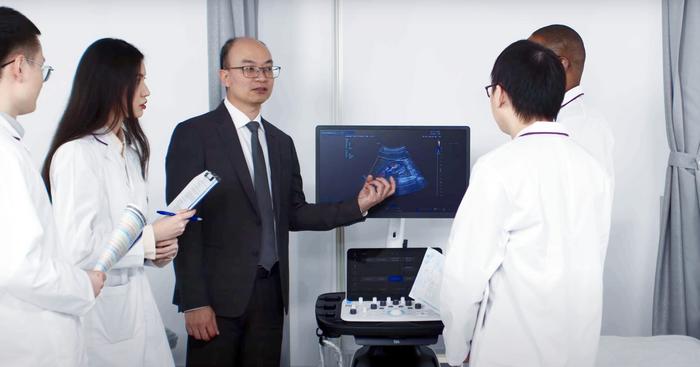
Additionally, the research group has developed a machine learning-based model using 2-D shear wave elastography (SWE) combined with CKD clinical data to assess renal fibrosis. Despite the advancements in ultrasound elastography for diagnosing renal fibrosis, the technique's effectiveness heavily relies on the operator's skill, posing challenges in settings with limited resources. S-CKD aims to facilitate the application of ultrasound elastography in various clinical environments, enabling accurate, low-cost risk stratification using data easily extracted from medical records and standard imaging assessments.
Further, the team has introduced an ultrasound radiomics analysis, progressing from clinical data to in-depth ultrasound image examination. Radiomics, a cutting-edge field, extracts a multitude of imaging features invisible to the human eye from medical images, building models for non-invasive renal fibrosis evaluations. This radiomics method combines ultrasound imaging with clinical data to create a diagnostic model visualized through a web-based calculator. Although current models require manual input from medical professionals, future efforts will explore artificial intelligence, including deep learning, to develop a fully automated diagnostic system.
“The implementation of S-CKD holds the potential to assist healthcare practitioners in tailoring medical judgments and optimizing post-treatment protocols for CKD patients,” said Prof. Michael Tin Cheung YING, Associate Head and Professor of Department of Health Technology and Informatics at PolyU. “By utilizing non-invasive medical imaging results and basic demographic data, this tool offers a cost-effective solution for guiding patient management, thereby contributing to notable clinical advantages.”
Related Links:
PolyU