New AI Model Helps Radiologists Identify Breast Cancer Lesions on Ultrasound Images
Posted on 30 Aug 2023
While ultrasound is frequently used for diagnosing breast cancer due to its availability and cost-effectiveness, its accuracy remains a challenge, often leading to high false-positive rates and unnecessary biopsies. Now, a novel artificial intelligence (AI) model could enhance the accuracy of radiologists in assessing ultrasound images for indications of breast cancer. This algorithm could be particularly beneficial for less-experienced readers who are still developing their skills.
Researchers at Nanjing Medical University (Nanjing, China) conducted a retrospective study to assess the diagnostic performance of a deep learning (DL) model for breast ultrasound and its utility for readers with varying levels of expertise. They utilized data from over 45,000 ultrasound images taken using 42 different machine types across four hospitals. The researchers developed and verified a dual attention-based convolutional neural network that can differentiate malignant tumors from benign ones using B-mode and color Doppler ultrasound images.
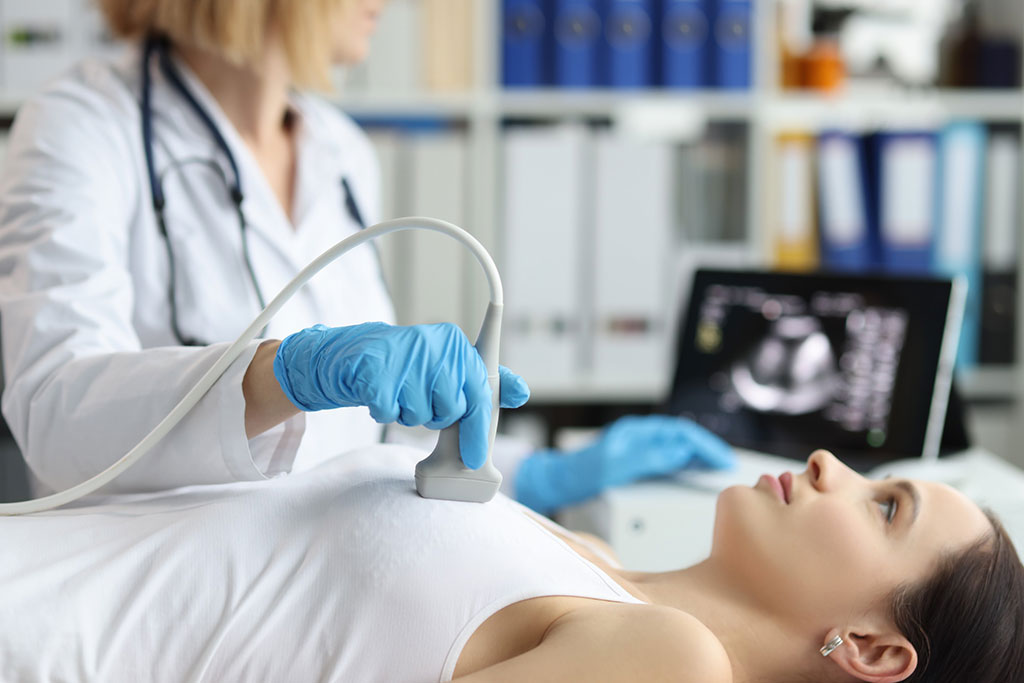
Using the DL model and without it, three novice readers with less than 5 years of ultrasound experience and two seasoned readers with 8 and 18 years of ultrasound experience each interpreted 1,024 randomly chosen lesions. The differences in areas under the receiver operating characteristic curves (AUCs) were analyzed using the DeLong test. The DL model showcased performance similar to experienced human readers, highlighting its potential as a reliable diagnostic tool. Specifically, the DL model's AUC closely matched that of seasoned radiologists. Novice radiologists with fewer than five years of ultrasound experience demonstrated notable enhancements when assisted by the DL model. The model increased their diagnostic precision, effectively elevating their performance to levels similar to those of experienced readers.
With the assistance of the DL model, both novice and experienced radiologists showed substantial improvements in diagnostic accuracy and interobserver agreement. Of particular significance was the noteworthy 7.6% decrease in the average false-positive rate. These findings suggest that DL-assisted diagnosis could be extremely beneficial for breast tumor diagnosis using ultrasound images. The model's accuracy, consistent results across different hospitals, and ability to support both novices and experts indicate a promising future for integrating DL technology into clinical practice. By boosting diagnostic accuracy and minimizing false-positive rates, the DL model could potentially streamline clinical processes and lower the risk of conducting unnecessary biopsies.
“This method is promising as an efficient and cost-effective tool for assisting radiologists, especially novice radiologists, in breast tumor diagnosis,” stated first author Huiling Xiang. “Further studies are warranted to characterize the feasibility of the model's widespread adoption.”
Related Links:
Nanjing Medical University