Novel AI Image Generation Tool Has Promising Future in Radiology
Posted on 20 Mar 2023
DALL-E 2, an artificial intelligence (AI) tool that was unveiled in April 2022 by OpenAI, generates novel photorealistic images or artwork based on textual input. Trained on billions of text-image pairs available existing on the internet, DALL-E 2 boasts powerful generative capabilities. Now, new research indicates that the DALL-E 2 deep learning model for text-to-image generation could have a bright future in health care, particularly for image generation, augmentation, and manipulation.
Researchers at Charité University Berlin (Berlin, Germany) set out to examine whether generative models have sufficient medical domain knowledge to provide accurate and useful results and to understand if the capabilities of DALL-E 2 can be transferred to the medical domain in order to create or augment data. They analyzed DALL-E 2’s radiological knowledge in creating and manipulating X-ray, computed tomography (CT), magnetic resonance imaging (MRI), and ultrasound images. The research team found that DALL-E 2 has learned relevant representations of X-ray images and has the potential for text-to-image generation. In particular, DALL-E 2 managed to create realistic X-ray images based on short text prompts, although it performed poorly when presented with specific CT, MRI, or ultrasound image prompts. Moreover, while it was able to reasonably reconstruct missing elements in radiological images, its ability to generate images with pathological abnormalities was limited. Additionally, DALL-E 2 could do much more, such as generating an entire, full-body radiograph using only one knee image.
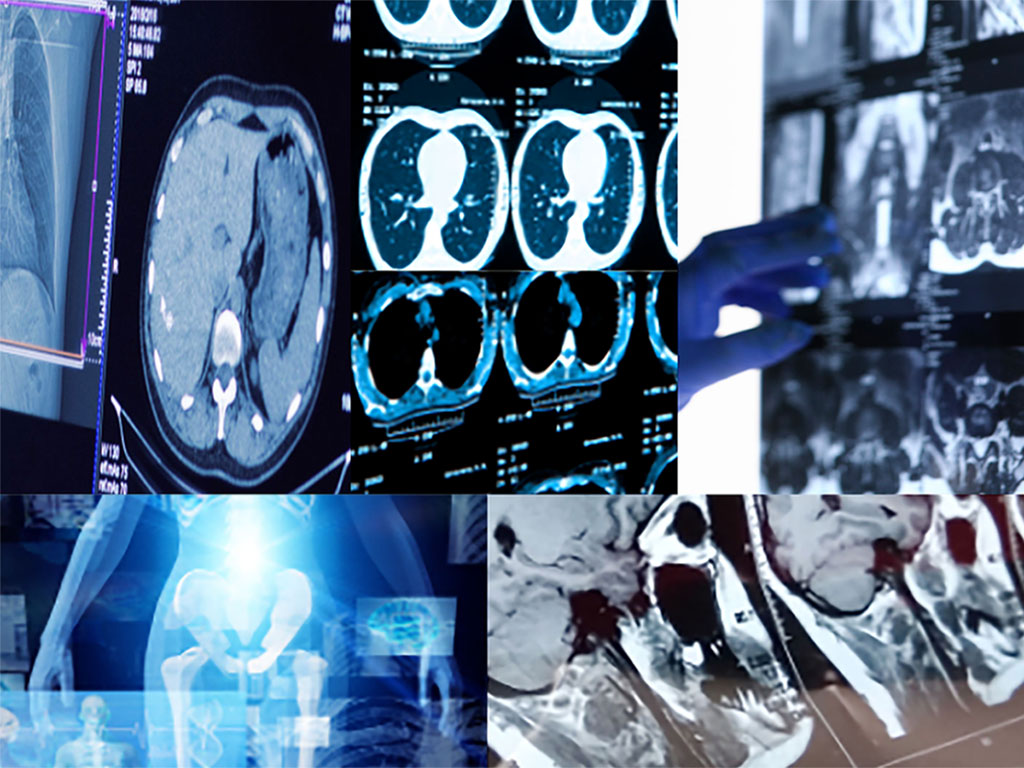
Synthetic data generated by DALL-E 2 can significantly speed up the development of new deep-learning tools for radiology while resolving privacy concerns over data sharing between institutions. The researchers have suggested that the generated images must be subjected to quality control by domain experts in order to minimize the risk of incorrect information being integrated into a generated data set. According to the researchers, there is also a need for further research to tweak these models to medical data and integrate medical terminology in order to create powerful models for data generation and augmentation in radiology research. DALL-E 2 is unavailable to the public for fine-tuning, although other generative models such as Stable Diffusion can be tweaked by the public and adapted to generate a variety of medical images. The study indicates that AI image generation in radiology has a promising future and further R&D could pave the way for exciting new tools for radiologists and medical professionals.
Related Links:
Charité University Berlin