New Approach Combining Ultrasound Imaging and AI Doubles Accuracy at Detecting Fetal Heart Flaws in the Womb
By MedImaging International staff writers
Posted on 28 May 2021
Researchers have found a way to double doctors’ accuracy in detecting the vast majority of complex fetal heart defects in utero by combining routine ultrasound imaging with machine-learning computer tools.Posted on 28 May 2021
The team of researchers from University of California, San Francisco (UCSF; San Francisco, CA, USA) trained a group of machine-learning models to mimic the tasks that clinicians follow in diagnosing complex congenital heart disease (CHD). Worldwide, humans detect as few as 30% to 50% of these conditions before birth. However, the combination of human-performed ultrasound and machine analysis allowed the researchers to detect 95% of CHD in their test dataset. Diagnosis of fetal heart defects, in particular, can improve newborn outcomes and enable further research on in utero therapies, the researchers said.
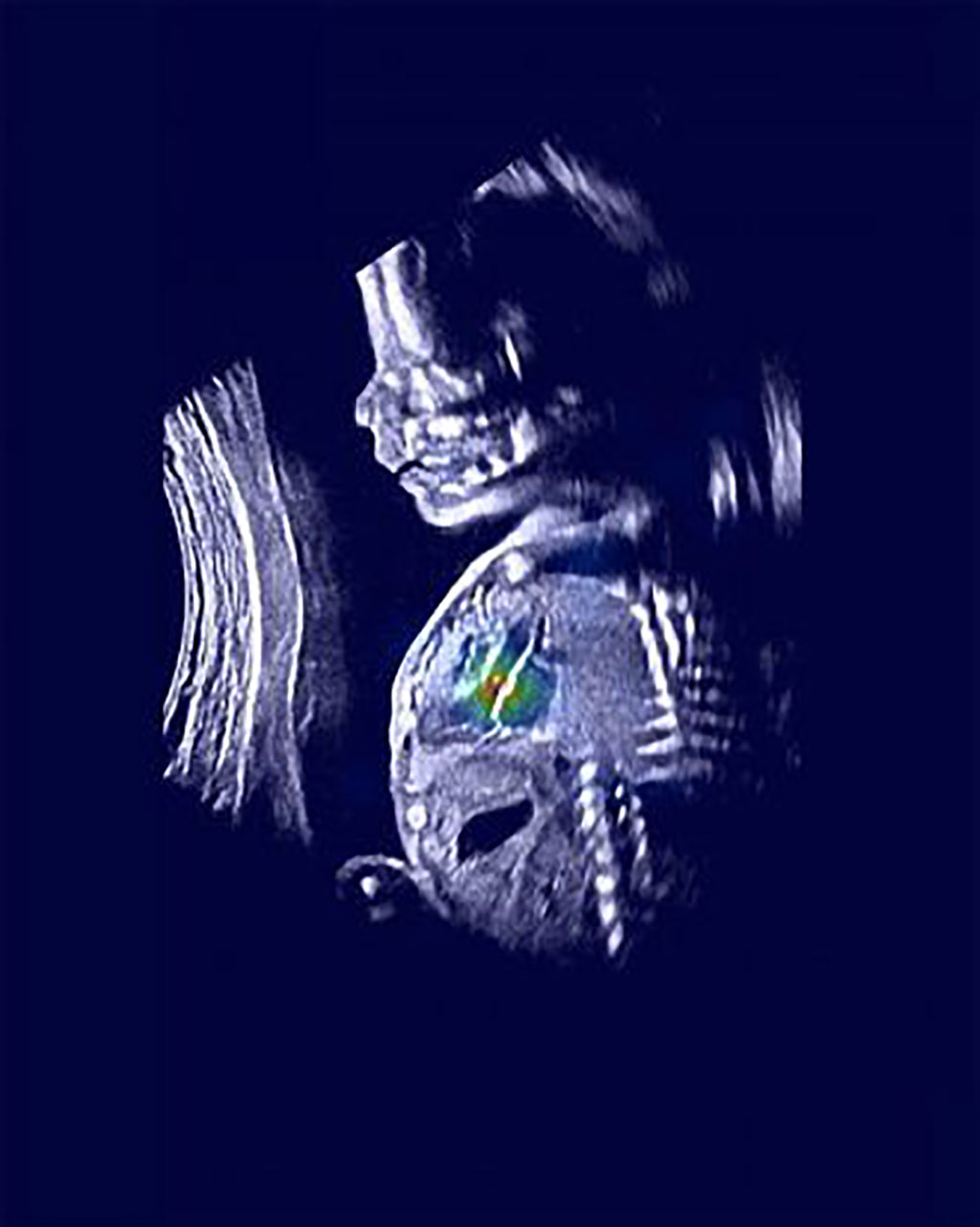
Image: An ultrasound image shows a normal fetus with relevant heart structures precisely highlighted (Photo courtesy of Rima Arnaout)
The UCSF team trained the machine tools to mimic clinicians’ work in three steps. First, they utilized neural networks to find five views of the heart that are important for diagnosis. Then, they again used neural networks to decide whether each of these views was normal or not. Then, a third algorithm combined the results of the first two steps to give a final result of whether the fetal heart was normal or abnormal.
“We hope this work will revolutionize screening for these birth defects,” said UCSF cardiologist Rima Arnaout, MD, a member of the UCSF Bakar Computational Health Sciences Institute, the UCSF Center for Intelligent Imaging, and a Chan Zuckerberg Biohub Intercampus Research Award Investigator. “Our goal is to help forge a path toward using machine learning to solve diagnostic challenges for the many diseases where ultrasound is used in screening and diagnosis.”
Related Links:
University of California, San Francisco