New Approach Combines Deep Learning and Physics to Fix Motion-Corrupted MRI Scans
Posted on 18 Aug 2023
MRI (Magnetic Resonance Imaging) is known for its superior ability to provide high-quality soft tissue contrast, making it a preferred choice over other imaging techniques like X-rays or CT scans. However, one of the challenges with MRI is its extreme sensitivity to even the smallest movements, leading to image artifacts. Such artifacts can obscure essential details, putting patients at risk of being misdiagnosed or receiving incorrect treatment. The process of an MRI scan varies in duration, ranging from a matter of minutes to an entire hour, depending on the specific images required. Even during the quickest scans, minor movements can greatly distort the resulting image. While in typical camera imaging, motion results in a localized blur, motion during an MRI scan can cause artifacts that corrupt the entire image. Some patients may be anesthetized or asked to control their breathing to minimize movement, but these solutions are not always feasible, especially in specific populations such as children or patients with psychiatric disorders.
Researchers at MIT (Cambridge, MA, USA) have risen to this challenge by creating a novel deep-learning model that can correct motion in brain MRI scans. This method combines physics-based modeling with deep learning techniques, enabling the construction of a motion-free image from corrupted data, without the need to alter the actual scanning process. The brilliance of this combined approach is its ability to ensure consistency between the image produced and the actual physical measurements of what is being depicted. If this balance is not maintained, the model could create "hallucinations"—images that look realistic but are physically and spatially incorrect. This could lead to even more misleading diagnoses. The application of an MRI free from motion artifacts would not only enhance patient outcomes but also has broad-reaching implications, especially for patients with neurological disorders causing involuntary movements, such as Alzheimer's or Parkinson's disease.
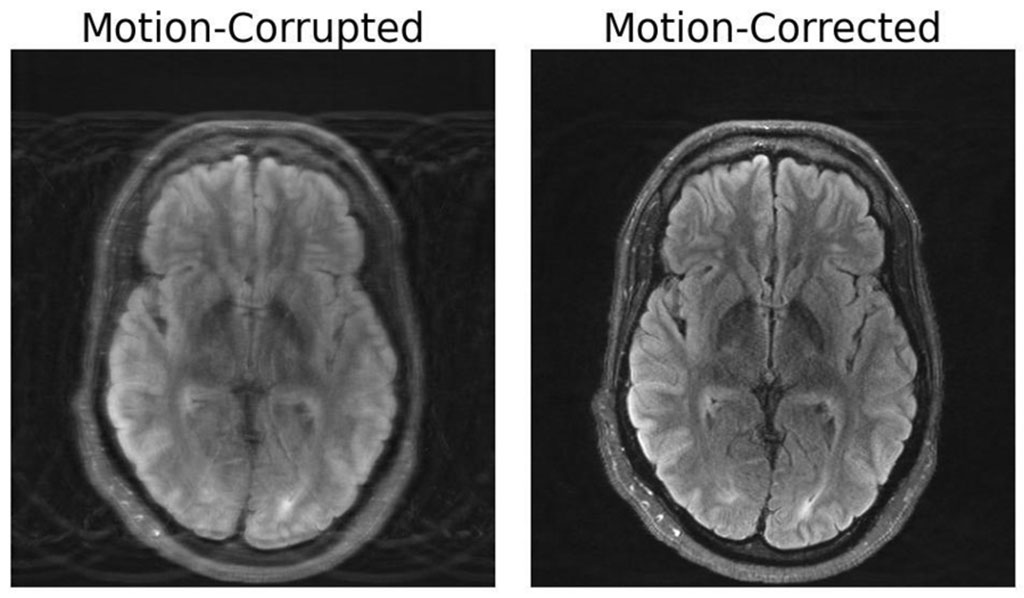
The impact of motion artifacts is not merely confined to patient diagnosis. An earlier study estimated that motion-related issues affect 15% of brain MRIs, leading to repeated scans or extended imaging sessions. This necessity for re-imaging translates into increased hospital costs per scanner. The researchers believe that their innovation could be further expanded. Future investigations might delve into more complex types of head movement or explore motion-related challenges in other parts of the body. For example, fetal MRI, which faces the problem of rapid and unpredictable motion, requires an approach that goes beyond simple translations and rotations. The development of a motion correction method for brain MRI scans marks a significant advancement in medical imaging that can improve diagnostic accuracy as well as reduce healthcare costs.
Related Links:
MIT