Study Reveals Value of Using Both Human and AI in Detecting Breast Cancer
Posted on 29 Apr 2022
Radiologists and artificial intelligence (AI) systems yield significant differences in breast-cancer screenings, according to a new study, revealing the potential value of using both human and AI methods in making medical diagnoses.
The analysis by a team of researchers at New York University (New York, NY, USA) centered on a specific AI tool: Deep neural networks (DNNs), which are layers of computing elements - “neurons” - simulated on a computer. A network of such neurons can be trained to “learn” by building many layers and configuring how calculations are performed based on data input - a process called “deep learning.” The scientists compared breast-cancer screenings read by radiologists with those analyzed by DNNs.
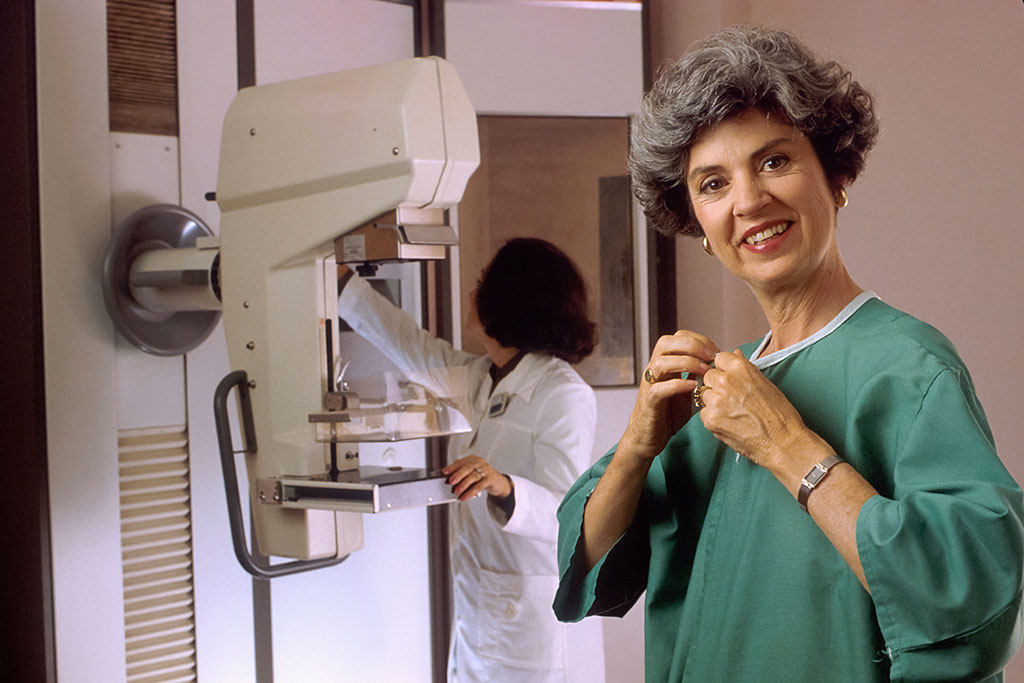
The researchers found that DNNs and radiologists diverged significantly in how they diagnose a category of malignant breast cancer called soft tissue lesions. While radiologists primarily relied on brightness and shape, the DNNs used tiny details scattered across the images. These details were also concentrated outside of the regions deemed most important by radiologists. By revealing such differences between human and machine perception in medical diagnosis, the researchers moved to close the gap between academic study and clinical practice.
“While AI may offer benefits in healthcare, its decision-making is still poorly understood,” explains Taro Makino, a doctoral candidate in NYU’s Center for Data Science and the paper’s lead author. “Our findings take an important step in better comprehending how AI yields medical assessments and, with it, offer a way forward in enhancing cancer detection.”
“The major bottleneck in moving AI systems into the clinical workflow is in understanding their decision-making and making them more robust,” added Makino. “We see our research as advancing the precision of AI’s capabilities in making health-related assessments by illuminating, and then addressing, its current limitations.”
“In these breast-cancer screenings, AI systems consider tiny details in mammograms that are seen as irrelevant by radiologists,” explained Krzysztof Geras, Ph.D., faculty in NYU Grossman School of Medicine’s Department of Radiology. “This divergence in readings must be understood and corrected before we can trust AI systems to help make life-critical medical decisions.”
Related Links:
New York University