AI-Powered Artefact Removal Can Identify Poor-Quality MRI Images with Near-Human Accuracy in Milliseconds
By MedImaging International staff writers
Posted on 05 Feb 2021
A new study has demonstrated the effective use of a retrospective artefact correction (RAC) neural network learned with unpaired data to disentangle and remove unwanted artefacts in magnetic resonance (MR) images.Posted on 05 Feb 2021
The findings of the study by researchers at the UNC School of Medicine (Chapel Hill, NC, USA) also revealed the capacity of the RAC network to retain anatomical details in MR images with different contrasts, improve magnetic resonance imaging (MRI) quality post acquisition, and enhance image usability.
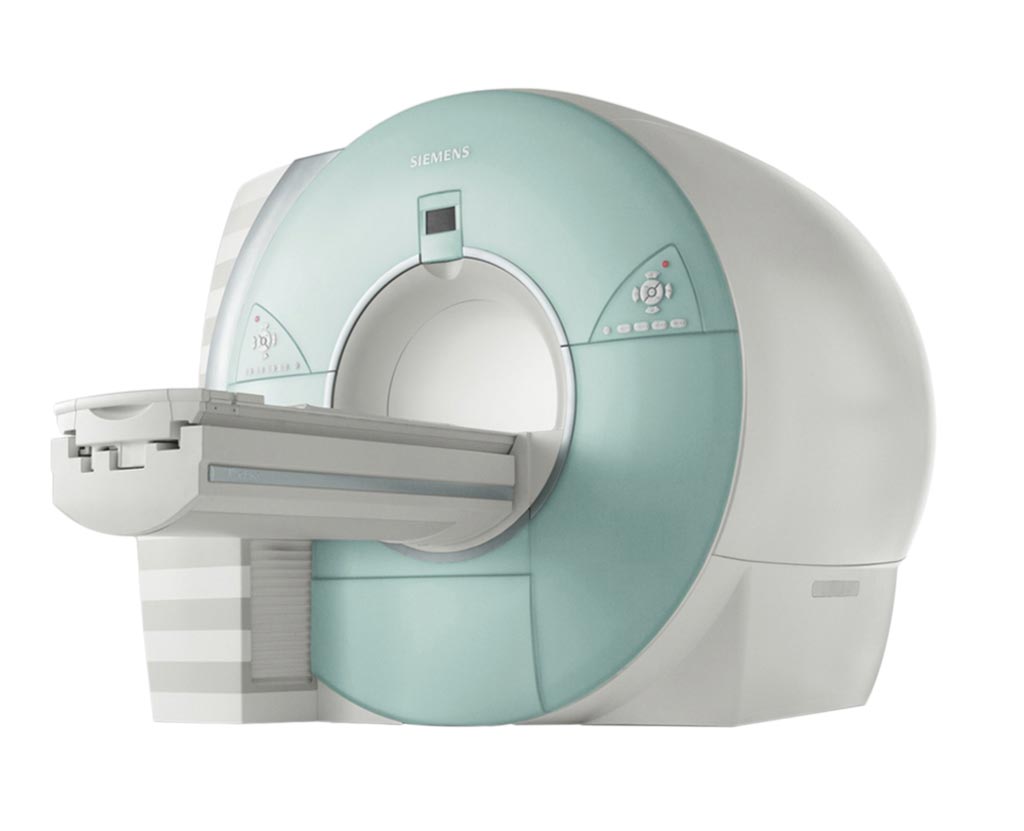
Image: Siemens Magnetom Espree 1.5T (Photo courtesy of Siemens)
MRI is susceptible to artefacts caused by motion that can render the images unusable and cause financial losses in imaging studies. At UNC’s Biomedical Research Imaging Center (BRIC), a team is exploring the use of deep learning to identify poor-quality images with near-human accuracy in milliseconds. Their investigative work is aimed at increasing timely decision-making in MRI re-scan. RAC is an increasingly investigated technique in MRI for the correction of motion-induced artefacts. Their study in applied imaging evidences superior motion correction via artificial intelligence (AI) techniques for RAC. Their investigation demonstrates further study of reliable AI techniques for RAC is warranted to benefit image correction and reconstruction in future MRI studies.
“AI-powered RAC can salvage innumerable images with motion artefacts to significantly boost the quantity of usable images and reduce financial losses for imaging studies,” said Pew-Thian Yap, PhD, Image Analysis Core Director at BRIC, who is leading the team.
Related Links:
UNC School of Medicine