AI Algorithm Identifies Patients Who Can Benefit from Clot Removal Surgery
By MedImaging International staff writers
Posted on 17 Oct 2019
Researchers at the University of Texas Health Science Center (Houston, TX, USA) have developed an algorithm that can help physicians outside of major stroke treatment centers assess if a patient suffering from ischemic stroke could benefit from an endovascular procedure for removing a clot blocking an artery.Posted on 17 Oct 2019
Endovascular thrombectomy involves threading a catheter through the femoral artery in the leg till it reaches the brain, where the clot can be removed mechanically. Previous studies have demonstrated that the procedure can improve outcomes for stroke patients only in cases of minimal injury to brain tissue during treatment. However, only advanced neuroimaging in the form of emergent magnetic resonance imaging or computed tomography (CT) perfusion makes it possible to detect if the treatment will be suitable for a patient. Such technology and expertise are usually unavailable at a majority of community hospitals and primary stroke centers.
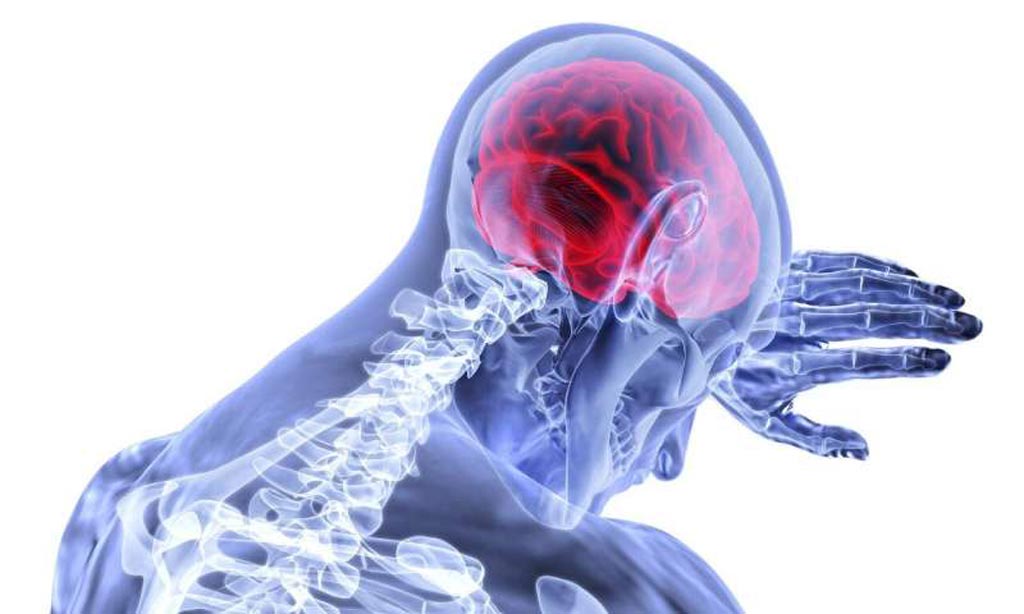
Image: The machine-learning algorithm, called DeepSymNet, learned to identify post-stroke blood vessel blockages from CT angiogram images (Photo courtesy of Medical Xpress).
To fill this gap, the team of researchers developed a machine-learning tool that can be used with the widely available CT angiogram imaging technique. The tool can analyze images by automatically "learning" subtle image patterns that can be used as a proxy for other more advanced, but not readily available, imaging modalities such as CT perfusion. The researchers tested the tool by identifying patients in their stroke registry who had suffered a stroke or had conditions that mimicked stroke. Out of the 224 patients who had a stroke, 179 had cerebral blood vessels that were blocked. The machine learning algorithm, called DeepSymNet learned to identify these blockages from the CT angiogram images, and trained the software to use those same images to define the area of brain that had died, using concurrent acquired CT perfusion scans as the "gold standard."
"The advantage is you don't have to be at an academic health center or a tertiary care hospital to determine whether this treatment would benefit the patient. And best of all, CT angiogram is already widely used for patients with stroke," said Sunil A. Sheth, MD, corresponding author and assistant professor of neurology with McGovern Medical School at UT Health.
Related Links:
University of Texas Health Science Center