Deep Learning with SPECT MPI Can Help Diagnose Coronary Heart Disease
By MedImaging International staff writers
Posted on 22 Jun 2019
Researchers from the Cedars-Sinai Medical Center (Los Angeles, CA, USA) have demonstrated for the first time that deep learning analysis of upright and supine single photon emission computed tomography (SPECT) myocardial perfusion imaging (MPI) can be used to improve the diagnosis of obstructive coronary artery disease.Posted on 22 Jun 2019
SPECT MPI is widely used for the diagnosis of coronary artery disease as it shows how well the heart muscle is pumping and examines blood flow through the heart during exercise and at rest. Two positions (semi-upright and supine) are routinely used to mitigate attenuation artifacts on new cameras with a patient imaged in sitting position. The current quantitative standard for analyzing MPI data is to calculate the combined total perfusion deficit (TPD) from these two positions. Visually, physicians need to reconcile information available from the two views.
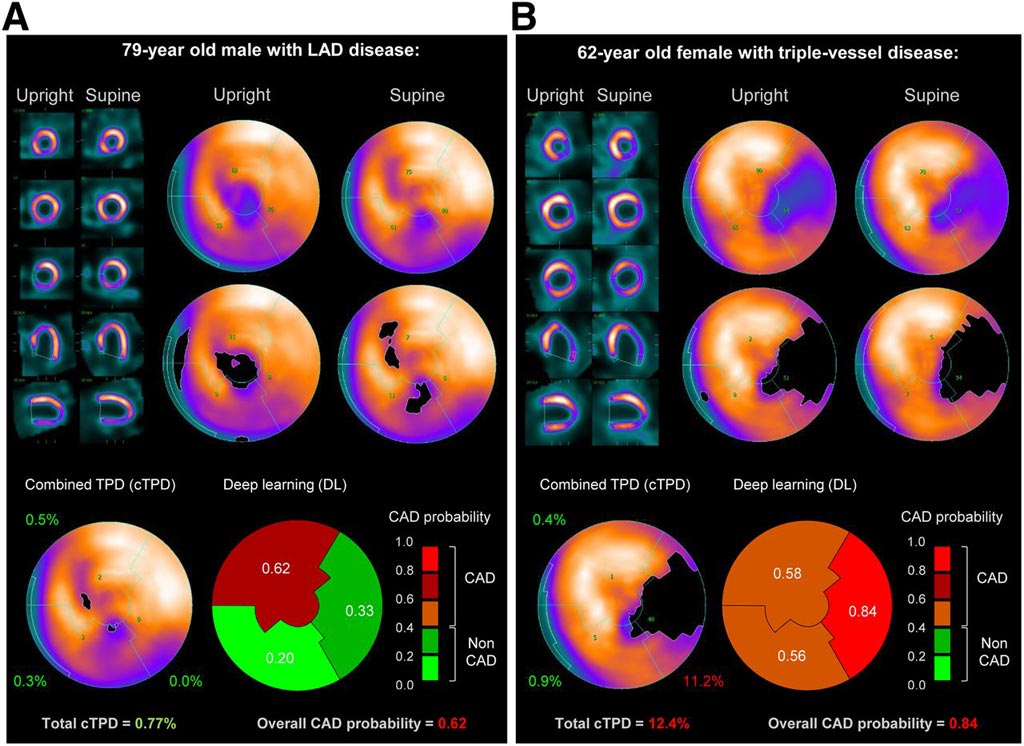
Image: A prediction of obstructive CAD from upright and supine stress MPI. Short/long axis views, polar maps depicting normalized radiotracer count distribution and perfusion defects (top), and predictions by cTPD and DL (bottom) are shown for two patients with obstructive CAD (Photo courtesy of SNMMI).
Deep convolutional neural networks, or deep learning, go beyond machine learning using algorithms. They directly analyze visual data, learn from them, and make intelligent findings based on the image information. For their study, the researchers compared deep learning analysis of data from the two-position stress MPI with the standard TPD analysis of 1,160 patients without known coronary artery disease. All patients underwent stress MPI and had on-site clinical reads and invasive coronary angiography correlations within six months of MPI. During the validation procedure, the researchers trained four different deep learning models.
The researchers found that 62% patients and 37% of the arteries had obstructive disease. Per-patient sensitivity improved from 61.8% with TPD to 65.6% with deep learning, and per-vessel sensitivity improved from 54.6% with TPD to 59.1% with deep learning. Additionally, deep learning had a sensitivity of 84.8%, as compared to 82.6% for an on-site clinical read. These results clearly demonstrate that deep learning improves MPI interpretation over the current methods.
Related Links:
Cedars-Sinai Medical Center