Researchers Publish Chest X-Ray Dataset to Train AI Models
By MedImaging International staff writers
Posted on 20 Feb 2019
Researchers from the Stanford University School of Medicine (Stanford, CA, USA) have published CheXpert, a large dataset of chest X-rays and competition for automated chest X-ray interpretation, which features uncertainty labels and radiologist-labeled reference standard evaluation sets. Automated chest radiograph interpretation at the level of practicing radiologists could provide substantial benefit in many medical settings, from improved workflow prioritization and clinical decision support to large-scale screening and global population health initiatives. Posted on 20 Feb 2019
CheXpert consists of 224,316 chest radiographs of 65,240 patients collected from Stanford Hospital that were performed between October 2002 and July 2017 in both inpatient and outpatient centers, along with their associated radiology reports. The dataset was co-released with MIMIC-CXR, a large dataset of 371,920 chest X-rays associated with 227,943 imaging studies sourced from the Beth Israel Deaconess Medical Center between 2011-2016.
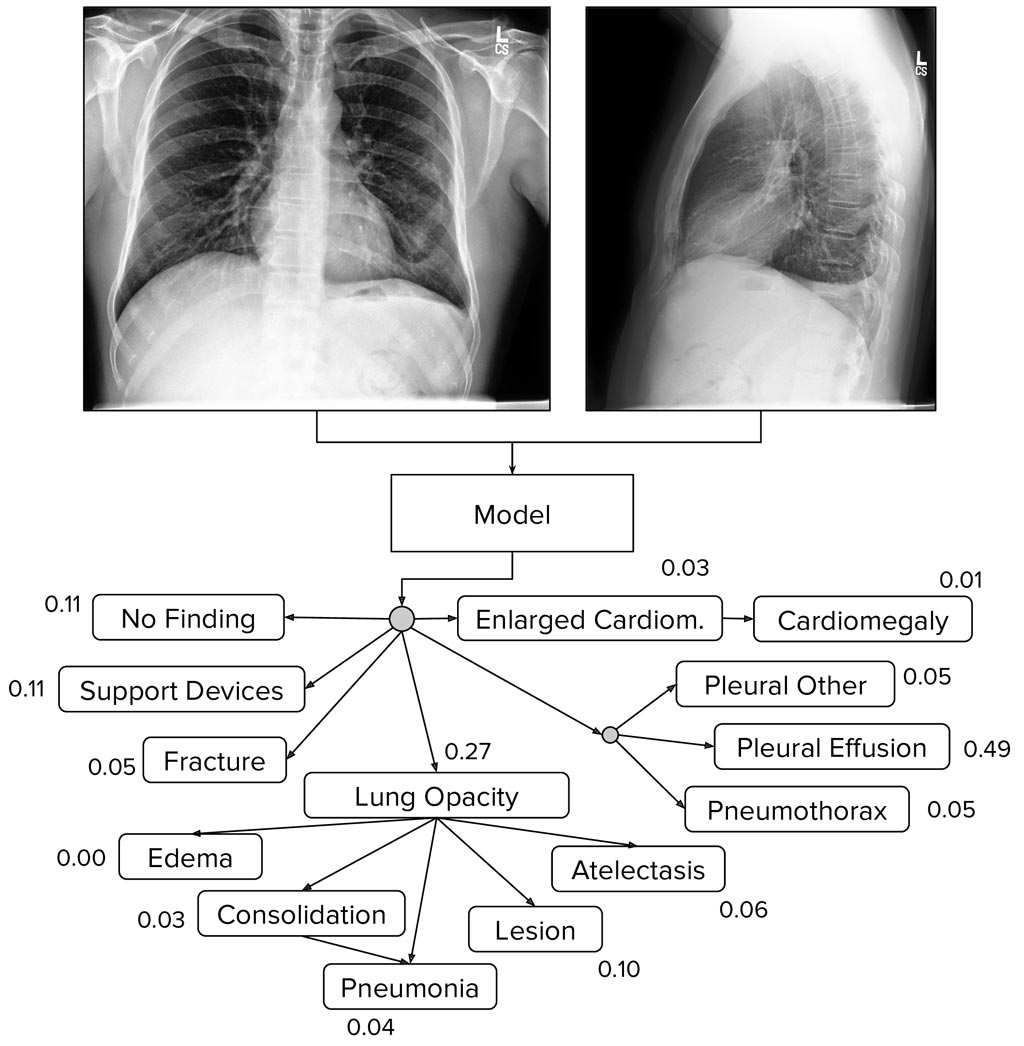
Image: The CheXpert dataset of chest X-rays is designed for automated chest X-ray interpretation (Photo courtesy of Stanford University School of Medicine).
One of the main obstacles in the development of chest radiograph interpretation models has been the lack of datasets with strong radiologist-annotated groundtruth and expert scores against which researchers can compare their models. CheXpert is expected to address that gap, making it easy to track the progress of models over time on a clinically important task.
The researchers have also developed and open-sourced the CheXpert labeler, an automated rule-based labeler to extract observations from the free text radiology reports to be used as structured labels for the images. This is expected to help other institutions extract structured labels from their reports and release other large repositories of data that will allow for cross-institutional testing of medical imaging models. The dataset is expected to help in the development and validation of chest radiograph interpretation models towards improving healthcare access and delivery worldwide.
Related Links:
Stanford University School of Medicine